the Creative Commons Attribution 4.0 License.
the Creative Commons Attribution 4.0 License.
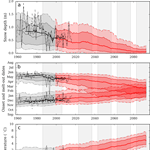
Multi-component ensembles of future meteorological and natural snow conditions for 1500 m altitude in the Chartreuse mountain range, Northern French Alps
Deborah Verfaillie
Matthieu Lafaysse
Michel Déqué
Nicolas Eckert
Yves Lejeune
This article investigates the climatic response of a series of indicators for characterizing annual snow conditions and corresponding meteorological drivers at 1500 m altitude in the Chartreuse mountain range in the Northern French Alps. Past and future changes were computed based on reanalysis and observations from 1958 to 2016, and using CMIP5–EURO-CORDEX GCM–RCM pairs spanning historical (1950–2005) and RCP2.6 (4), RCP4.5 and RCP8.5 (13 each) future scenarios (2006–2100). The adjusted climate model runs were used to drive the multiphysics ensemble configuration of the detailed snowpack model Crocus. Uncertainty arising from physical modeling of snow accounts for 20 % typically, although the multiphysics is likely to have a much smaller impact on trends. Ensembles of climate projections are rather similar until the middle of the 21st century, and all show a continuation of the ongoing reduction in average snow conditions, and sustained interannual variability. The impact of the RCPs becomes significant for the second half of the 21st century, with overall stable conditions with RCP2.6, and continued degradation of snow conditions for RCP4.5 and 8.5, the latter leading to more frequent ephemeral snow conditions. Changes in local meteorological and snow conditions show significant correlation with global temperature changes. Global temperature levels 1.5 and 2 ∘C above preindustrial levels correspond to a 25 and 32 % reduction, respectively, of winter mean snow depth with respect to the reference period 1986–2005. Larger reduction rates are expected for global temperature levels exceeding 2 ∘C. The method can address other geographical areas and sectorial indicators, in the field of water resources, mountain tourism or natural hazards.
- Article
(4627 KB) - Full-text XML
-
Supplement
(745 KB) - BibTeX
- EndNote
Snow on the ground is one of the most climate-sensitive components of the mountain environment. Indeed, temperature changes drive shifts of the partitioning between rain and snow precipitation and are strongly linked with the magnitude of ablation processes (e.g., melt, sublimation). Scientific studies carried out over the past decades have demonstrated that large-scale climate change has a profound impact on past and future snow conditions in alpine regions throughout the world (Beniston, 1997; Brown and Mote, 2009; Martin et al., 1994; Marty et al., 2017b; Mote et al., 2005, 2018; Reid et al., 2015) and in particular in the European Alps (Beniston et al., 2018; Gobiet et al., 2014).
Besides its emotional and cultural visual role in the winter mountain landscape, snow is a critical water resource component (Bosshard et al., 2014; Lafaysse et al., 2014; Olsson et al., 2015) including hydropower (Francois et al., 2015). Furthermore, snow conditions exert major controls over winter mountain tourism (Abegg et al., 2007; Spandre et al., 2016a). Snow on the ground and its climate fluctuations are highly relevant for mountain ecosystem functioning (Boulangeat et al., 2014; Thuiller et al., 2014) and are strongly tied with the frequency and magnitude of mountain hazards such as snow avalanches (Castebrunet et al., 2014; Martin et al., 2001) and debris flows (Jomelli et al., 2015).
While a wealth of studies have addressed, with various levels of complexity, the unequivocal projected decrease in mean multi-annual snow amount along with corresponding temperature increase predicted by all existing climate change scenarios available for the European Alps (Gilaberte-Burdalo et al., 2014; Gobiet et al., 2014; Lafaysse et al., 2014; Marty et al., 2017a; Piazza et al., 2014; Rousselot et al., 2012; Schmucki et al., 2014; Steger et al., 2013), there remains a need for quantitative and authoritative information spanning various lead times at the scale of the 21st century appropriate for socioeconomic stakeholders at the local, regional and national scale. This originates from the unavailability hitherto of required input information as well as a suitable methodological framework to identify and convey the information to their potential users in the most relevant and appropriate way. Indeed, many existing studies addressing future snow conditions in the European Alps rely on climate scenarios which have formed the basis of the 4th IPCC Assessment Report (AR4). While their conclusions were not contradicted by the subsequent report (IPCC, 2013, 2014a, b, c), various methodological changes and updates warrant the necessity to generate renewed estimates of the impact of future climate change on meteorological and natural snow conditions in the Alps, consistent with AR5 material and conclusions (IPCC, 2013). Firstly, IPCC global-scale socioeconomic/greenhouse gas emission scenarios have seen major changes from AR4 to AR5, from the SRES approach to RCP (Moss et al., 2010). Secondly, global climate models have evolved from the Coupled Model Intercomparison Project Phase 3 (CMIP3) to CMIP5 (Taylor et al., 2012) and generated novel ensembles of global climate projections (Taylor et al., 2012). Last, regional climate model outputs have recently been generated using CMIP5 climate projections as boundary conditions, providing ensemble model runs spanning the entire chronology of climate fluctuations using historical model runs and RCP-driven projections. This concerns the time period from 1950 to 2100 in the case of the EURO-CORDEX project (Jacob et al., 2014; Kotlarski et al., 2014). Existing recent literature addressing the impact of climate change on wintertime snow conditions has only in a few cases used these latest-generation model results (Frei et al., 2018; Hanzer et al., 2018; Terzago et al., 2017).
Using latest-generation climate models as input for impact assessments because they are newer is not per se a sufficient motivation for updating existing climate impact studies (Knutti et al., 2010). Improved methodological approaches also have the potential to lever critical limitations of existing studies. For example, several recent studies (Castebrunet et al., 2014; Marty et al., 2017a; Rousselot et al., 2012; Schmucki et al., 2014) were based on so-called, more or less sophisticated, delta-change approaches applied to meteorological conditions, employed to drive snowpack models. Using such methods, as recognized by Marty et al. (2017a), implies “that the variability does not change over time”, in particular the seasonality of meteorological conditions, such as the frequency of precipitation events and their time distribution. Indeed, such approaches consist in applying a pre-determined difference (delta) of temperature and/or precipitation values to an observation record, based on changes computed using climate models (either global or regional). This cannot capture combined changes in temperature, precipitation and other meteorological factors, in terms of magnitude of the fluctuations and their seasonal-scale and interannual variability. Given that snow conditions for a given season depend on the unfolding of meteorological conditions driving accumulation (precipitation events) and ablation of the snowpack, realistic predictions of the impact of climate change on mountain meteorological and snow conditions should instead be based on the chronology of the climate model outputs at the daily or sub-daily time resolution. However, this requires the use of downscaling and adjustment methods operating at these timescales (Déqué, 2007; Gobiet et al., 2015; Themeßl et al., 2011), in order to bridge the elevation gap induced by the difference between the spatial resolution of the regional or global climate model and the topography of the target area (Piazza et al., 2014), and to mitigate inevitable biases held by the raw climate model outputs (Christensen et al., 2008; Kotlarski et al., 2014; Rauscher et al., 2010). Last, solid assessment of the impact of climate change on snow conditions requires carefully handling uncertainty and variability sources, in order to provide balanced and relevant information to the end users (Brasseur and Gallardo, 2016). This can be achieved by selecting relevant indicators along with their time and space aggregation principles, relying on ensembles addressing the largest possible range of uncertainty and variability sources, and employing a robust statistical analysis framework, in order to focus not only on changes in mean conditions (Marty et al., 2017a) but also higher-order moments of the distribution of possible futures (Vasseur et al., 2014) and the statistical significance level of the computed trends (Castebrunet et al., 2014).
In this study, we introduce recent developments in the field of climate information related to meteorological and natural snow conditions, applied to the French mountain areas. The approach draws on the use of the ADAMONT statistical adjustment method (Verfaillie et al., 2017) applied to multiple historical (1950–2005) and future (2006–2100) EURO-CORDEX regional climate model runs spanning all relevant RCPs (RCP2.6, RCP4.5 and RCP 8.5). The 13 GCM–RCM EURO-CORDEX pairs available in April 2017 (and for which the geopotential data for the corresponding CMIP5 GCMs were available) were used. These are expected to span the overall uncertainty resulting from GCM errors, RCM errors and climate internal variability. We used one of the longest meteorological reanalyses available in the French mountain regions – the SAFRAN reanalysis (Durand et al., 2009b) – as the reference observational dataset. Continuous hourly resolution meteorological time series derived from RCM output by the ADAMONT statistical adjustment method are then used as input of the SURFEX/ISBA-Crocus snowpack model (Vionnet et al., 2012). Its default configuration and also, for the first time to the best of our knowledge, a recently developed multiphysics ensemble system (Lafaysse et al., 2017) are used, making it possible to quantify snowpack model errors in the context of climate change impact assessment. We define a series of indicators for meteorological and natural snow conditions at the annual scale based on daily temperature, precipitation, snow depth and snow water equivalent data. The multi-ensemble datasets are analyzed using two specific statistical frameworks, addressing either individual annual values or multi-annual averages, which provide complementary information depending on the application. While the framework developed here can be applied as such in all areas where the SAFRAN system has been implemented (Durand et al., 2009b; Maris et al., 2009; Quintana-Seguí et al., 2017), we focus in this article on results obtained for the Chartreuse massif in the Northern French Alps at an altitude of 1500 m. This altitude level is particularly sensitive to climate change (Gobiet et al., 2015; Lafaysse et al., 2014; Martin et al., 1994; Marty et al., 2017a; Rousselot et al., 2012; Schmucki et al., 2014; Steger et al., 2013) and it corresponds roughly to the setting of the mid-altitude long-term observational site Col de Porte (1325 m altitude; 45.3∘ N, 5.77∘ E), which has long been used to monitor and showcase the impact of climate change on mountain snowpack and provides appropriate observational records, making it possible to place the modeling results in context.
2.1 Geographical setup
This study uses meteorological data from the SAFRAN reanalysis (Durand et al., 2009a, b), which provides meteorological data for different regions in the French Alps but also in the French and Spanish Pyrenees and Corsica. Unlike traditional reanalyses, SAFRAN does not operate on a grid but rather on mountain regions subdivided into different polygons known as massifs (Durand et al., 1993, 1999), which correspond to regions of 500 to 2000 km2 for which meteorological conditions are assumed spatially homogeneous but varying only with altitude. SAFRAN data are thus available for each massif and for elevation bands with a resolution of 300 m. While all the developments and results introduced below can be generically applied to all the French mountain regions, we focus solely, for the sake of brevity, on the Chartreuse massif at an altitude of 1500 m, on flat terrain and without accounting for specific topographical masks. This corresponds roughly to the configuration of the Col de Porte observatory (CDP, 1325 m above sea level (a.s.l.); 45.3∘ N, 5.77∘ E), located in the Chartreuse massif in the French Alps (Morin et al., 2012).
2.2 Observations
2.2.1 SAFRAN reanalysis
The SAFRAN system is a regional-scale meteorological downscaling and surface analysis system (Durand et al., 1993), providing hourly data of temperature, precipitation amount and phase, specific humidity, wind speed, and shortwave and longwave radiation. SAFRAN refers here to the original mountain region implementation (Durand et al., 1993). SAFRAN was later expanded to wider geographical areas in France (Vidal et al., 2010) and Spain (Quintana-Seguí et al., 2017). In this study, we use data from 1958 to 2016 for the single-site setup of the Chartreuse massif at 1500 m a.s.l., on flat terrain.
2.2.2 Col de Porte observations
This study uses long-term observations from the Col de Porte observatory (Morin et al., 2012). Daily snow depth and meteorological measurements (temperature and precipitation) are available from 1960 to 2016. At this site, the snow season generally extends from December to April, with occasional occurrences of snowmelt and rainfall events, and usually low wind speed. Note that the Col de Porte meteorological observations are not used in the SAFRAN reanalysis.
2.3 Climate projections
This study uses the EURO-CORDEX dataset (Jacob et al., 2014; Kotlarski et al., 2014) available in April 2017, consisting of six regional climate models (RCMs) forced by five different global climate models (GCMs) from the CMIP5 ensemble (Taylor et al., 2012) over Europe, for the historical, RCP 2.6, RCP 4.5 and RCP 8.5 scenarios (Moss et al., 2010). Only the GCM–RCM pairs for which the geopotential data for the CMIP5 GCMs were available were used. Historical runs generally cover the period 1950–2005 and RCPs cover the period 2006–2100, with some exceptions due to the availability of either the RCM or the GCM. Table 1 provides the different GCM–RCM combinations used in this study. In total, 43 different 0.11∘ resolution (EUR 11, ≈ 12.5 km) time series of daily minimum and maximum temperature, total precipitation, longwave and shortwave incoming radiation, zonal and meridian near-surface wind speed, and specific humidity were used. In order to analyze continuous long-term series (generally from 1950 to 2100 with a few exceptions), historical (HIST) and each RCP time series were concatenated (named RCP2.6, RCP4.5 and RCP8.5 in the following). The spread of this ensemble for a given RCP is due to three distinct factors: the different responses among the GCMs to a given RCP, the different responses among RCMs to a given GCM forcing, and the internal variability of climate at different timescales affecting the response of one specific model run. As in most impact studies based on EURO-CORDEX scenarios, we assume here that the 13 GCM–RCM pairs reasonably sample the overall uncertainty resulting from these three sources, even though not all GCM–RCM combinations are available.
Table 1EURO-CORDEX GCM–RCM combinations used in this study (rows: RCMs; columns: GCMs), with the time period available for the HIST and RCP 4.5 and 8.5 scenarios (RCPs). Model combinations additionally using RCP 2.6 are displayed in bold. Contributing institutes are indicated inside parentheses – CLMcom: CLM Community with contributions by BTU, DWD, ETHZ, UCD,WEGC; CNRM: Météo France; IPSL-INERIS: Institut Pierre Simon Laplace, CNRS, France – Laboratoire des Sciences du Climat et de l'Environnement, IPSL, CEA/CNRS/UVSQ – Institut National de l'Environnement Industriel et des Risques, Verneuil en Halatte, France; KNMI: Royal Netherlands Meteorological Institute, Ministry of Infrastructure and the Environment; MPI-CSC: Climate Service Center (CSC), Hamburg, Germany; SMHI: Rossby Centre, Swedish Meteorological and Hydrological Institute, Norrköping Sweden.
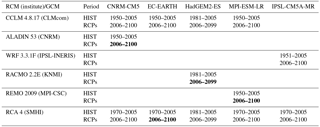
The EURO-CORDEX raw surface fields were adjusted using the ADAMONT method, which is a quantile mapping and disaggregation method taking into account weather regimes to provide multi-variable hourly adjusted climate projections (Verfaillie et al., 2017). The method uses a meteorological observational dataset at hourly time resolution (here the SAFRAN meteorological reanalysis from 1980 to 2011), and regional climate model outputs covering the geographical domain of interest (here the EURO-CORDEX dataset). Raw RCM outputs for the grid point closest to the middle of the Chartreuse massif were used (see Verfaillie et al., 2017 for details). The altitude values of the RCM grid points used range from 612 to 1085 m, with a mean value across all RCMs of 880 m. Note that Verfaillie et al. (2017) have demonstrated that the ADAMONT method provides adequate results under this setting with several hundreds of meters difference between RCM and the target altitude, and that selecting RCM grid points with a larger geographical distance but lower altitude difference does not necessarily improve the outcome of the adjustment procedure.
2.4 Snowpack model
We used the Crocus (Vionnet et al., 2012) unidimensional multilayer snowpack model to predict snow conditions based on meteorological input data (both reanalysis and adjusted climate projections). Crocus computes the exchanges of energy and mass between the snow surface and the atmosphere and between the snowpack and the ground underneath. It requires sub-diurnal (ideally hourly) meteorological forcing data and is able to simulate the evolution of the snowpack over time, by accounting for several processes occurring in the snowpack, such as thermal diffusion, phase changes and metamorphism. In this study, we used the ESCROC (Ensemble System CROCus) multiphysics approach described in Lafaysse et al. (2017), which consists in using multiple combinations of different physical options of the model to build an ensemble of model configurations. We specifically use ensemble E2 as defined in Lafaysse et al. (2017), which includes a subset of 35 configurations selected to be equiprobable at CDP. The spread of this ensemble has been optimized at CDP and is able to explain about two-thirds of total error in simulations driven by meteorological measurements at CDP, which is a realistic contribution of snowpack model error to the total simulation error (Lafaysse et al., 2017; Raleigh et al., 2015). An additional configuration corresponding to the default Crocus configuration run was also used, totalling 36 model configurations.
2.5 Indicators and post-processing
2.5.1 Definition of indicators
Based on meteorological and snow-related variables at daily time resolution, we computed and analyzed different indicators defined at the annual timescale, using an indicator-oriented approach described in Strasser et al. (2014). Defining “winter” as the period from December to April inclusive (5 months long), the following snow condition indicators were computed: mean winter snow depth (), exceedance duration over a snow depth threshold for thresholds values of 5 cm, 50 cm and 1 m (STED5, STED50, STED100, expressed in days). In terms of meteorological indicators, given the focus of the present study on wintertime processes and snow conditions, we considered mean winter temperature (), cumulated winter total (rain and snow) precipitation () and mean winter ratio between snow and total precipitation (). Relaxing the focus on the winter time period, we also computed the maximum annual snow water equivalent () as well as the snowpack onset and melt-out dates (SOD and SMOD), which correspond to the earliest/latest time bounds of the longest period of time with snow depth values exceeding 5 cm, which can be interpreted as the longest period of time with continuous snow cover. These indicators are meant to represent the most significant features of natural snow on the ground at the annual scale (Schmucki et al., 2014), although they are not immediately relevant for snow conditions in ski resorts (Spandre et al., 2016a; Steiger et al., 2017) and should not be the sole source of information to be used in this context. Figure 1 provides an overview of the snow-related indicators introduced above.
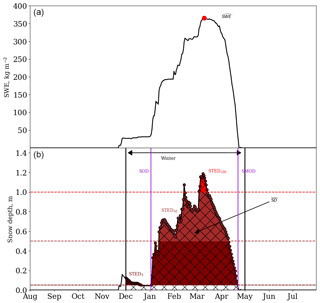
Figure 1Overview of the snow-related indicators introduced in Sect. 2.5, using an arbitrary SWE and snow depth time series over the course of a given year. (a) SWE time series, displaying the maximum value . (b) Snow depth time series, displaying graphically the related indicators. See text for details.
2.5.2 Statistical post-processing of indicators
The entire model chain provides estimates of a series of annual indicators continuously spanning the historical period from 1950 to 2005, typically to the end of the 21st century. A total of 13 GCM–RCM pairs were considered in the case of RCP4.5 and RCP8.5, out of which 4 are also available for RCP2.6. We generally used a 15-year window to assess the statistical distribution of the indicators considered. For a given GCM–RCM pair and a given RCP, statistics corresponding to a given year can be computed using indicator values for the 15 years surrounding it (7 before, the central year, and 7 after). In what follows, we assume that all GCM–RCM pairs bear equal probability (Knutti et al., 2010). We post-processed the distribution of annual indicator values in two ways.
-
Quantiles of annual values: in this case, for a given RCP, all annual values of the indicators spanning the 15-year time window for all the corresponding GCM–RCM pairs were pooled together (195 in the case of RCP4.5 and RCP8.5, 60 in the case of RCP2.6). The quantiles of the distribution of the annual values were determined using a kernel smoothing approach. We computed the 5, 17, 50, 83 and 95 % values (Q5, Q17, Q50, Q83, Q95), consistent with IPCC (2013). This approach provides statistical estimates for annual values of the indicator, although it mixes together the effects of interannual variability and intermodel variability.
-
Moments of multi-year averages: a running average of annual indicator values was computed using the 15-year sample window, for a given RCP and for each GCM–RCM pair. For a given RCP, mean (μ) and standard deviation (σ) values were computed for the ensemble of multi-annual averages of all GCM–RCM pairs. This approach provides information on the statistical distribution of each indicator for a given RCP on a multi-annual average perspective. In practice, we compute , corresponding to the 17 and 83 % quantiles in the case of a normal distribution, so that this approach becomes more comparable to the annual quantiles approach described earlier. In the case of the multiphysics Crocus model implementation, we mostly used the multi-year averages approach, and applied it to all Crocus members.
The spread of the distributions of these two approaches can be assessed in rather similar ways. In the multi-year average approach, the coefficient of variation (CV) can be determined as CV . In the annual quantiles approach, the spread can be assessed by dividing Q83–Q17 by Q50 to form a formal equivalent to the coefficient of variation, defined using quantile values instead of mean and standard deviation (referred to as quantile-based coefficient of variation – QCV – hereafter).
2.5.3 Changes between reference and future time periods
For both methods, results over the historical period are contextualized with temporal median or mean of the annual indicators computed for the SAFRAN-Crocus reanalysis and for observations at CDP.
The values of the post-processed indicators were computed using sliding 15-year windows spanning the entire climate dataset available, i.e., from 1950 to 2100 in the case of EURO-CORDEX data (although some GCM–RCM pairs do not span the full historical period), from 1958 to 2016 in the case the SAFRAN-Crocus reanalysis, and from 1960 to 2016 in the case of CDP observations. In order to compute differences between conditions of the recent past and future changes, the reference period 1986–2005 (Ref) was selected, which contains all (and only) historical EURO-CORDEX model runs and was used as a baseline period of the IPCC AR5. Specific values of the post-processed indicators were computed for a series of representative future 15-year time windows t centered on 2030, 2050, 2070 and 2090. For the snowpack indicators, values are provided for the reference time period as well as for the future. Changes were computed in the case of meteorological indicators , and . For each GCM–RCM pair m the mean value over the period 1986–2005 () was calculated, as well as mean values for 15-year windows around each future time period t for the RCP r (). For temperature and the ratio between snow and total precipitation, corresponds to the difference between and , while for precipitation, corresponds to the percentage increase or decrease compared to the reference period, i.e., . Finally, values of all or for a given r and a given t were determined. These calculations were performed for each RCP using all available GCM–RCM pairs. For the reference period 1986–2005 and future time periods, the multi-model calculations were performed using either all the GCM–RCM pairs providing RCP2.6, RCP4.5 and RCP8.5 model runs (4) or all the GCM–RCM pairs providing RCP4.5 and RCP8.5 model runs (13).
2.5.4 Relationships between local indicators and global air temperature between reference and future time periods
For the reference period 1986–2005 and for three 30-year periods during the 21st century (beginning of century (BOC), 2011–2040, middle of century (MOD) 2041–2070 and end of century (EOC), 2071–2100), we computed interannual mean values corresponding to a given GCM–RCM pair for the meteorological and snow indicators introduced above, for all RCPs available for a given GCM–RCM pair (either RCP4.5 and RCP8.5 only, or all three RCP2.6, RCP4.5 and RCP8.5 scenarios). For each GCM–RCM run under each available RCP configuration, the global temperature difference between future time periods (BOC, MOC and EOC, respectively) and the preindustrial period (1851–1880), referred to as , and , respectively, for the corresponding GCM and RCP was calculated (Taylor et al., 2012). In addition, the global temperature difference was also computed between future periods (BOC, MOC and EOC) and the reference (Ref) period 1986–2005 , and , respectively. Based on these datasets, we computed linear regressions curves (intercept forced to 0) between interannual means of the local meteorological and snow indicators during BOC, MOC and EOC, and the corresponding global annual temperature difference between the corresponding time period and the Ref period. Linear regressions were also computed using all future time periods together (ALL). In addition, the future values of the local meteorological and snow indicators of all future time periods were binned according to the corresponding global temperature by steps of 0.5 ∘C (± 0.25 ∘C), and the mean and standard deviation of all values within a given bin were computed.
2.5.5 Comparison between results of numerical simulations and observations
On the basis of the annual values of the indicators , and for the time period from 1986 to 2005, statistics of the differences between reanalysis data and Col de Porte observations were computed, in terms of mean bias, root mean square deviation (RMSD) and correlation (only , ). This is not meant to represent an evaluation of the SAFRAN-Crocus reanalysis, because the SAFRAN dataset used in this study was not optimized to correspond exactly to the geographical setting of the Col de Porte observation site (appropriate altitude, specific terrain masks impacting solar radiation time distribution). However, the geographical setting of the observations and simulations are sufficiently close to each other that the two can be analyzed concurrently and provide reasonable information pertaining to the ability of the model chain to represent meteorological conditions in such a mountainous area. A better statistical match between observation and reanalysis would, however, be expected using meteorological data more applicable to the observation configuration, which is not the purpose of this article and was addressed in previous publications (Durand et al., 2009b; Lafaysse et al., 2013).
This study introduces multi-component ensembles of past and future simulations of meteorological and snow conditions in the Chartreuse mountain range in the Northern French Alps at 1500 m altitude. As described previously, simulations encompass multiple RCPs, multiple GCM–RCM pairs from the EURO-CORDEX database adjusted using the ADAMONT method, and multiple Crocus snowpack model runs using the ESCROC ensemble system. This section describes the wealth of information generated through this process, focussing on meteorological and snow indicators described previously and addressing various components of the uncertainty and variability sources affecting the simulations.
3.1 Full ensemble configuration and uncertainty apportionment
Figures 2–3 provide an overview of all sources of uncertainty and variability accounted for in this study, in terms of snow conditions (using the indicator as an example) for the period from 1950 to 2100, for RCP4.5 and RCP8.5 climate projection data, respectively.
Figures 2a and 3a show continuous time series of annual values of mean winter snow depth data (), either observed or generated by the default snowpack model configuration fed by meteorological data from a reanalysis or an adjusted RCM for RCP4.5 and 8.5. They highlight the significant interannual variability in observed, reanalyzed and climate model datasets. For the time period 1986–2005, the mean observed value is 0.64 m. Using the default Crocus configuration fed by the SAFRAN reanalysis at 1500 m altitude yields bias and RMSD values of annual values of 0.10 m and 0.18 m, respectively, against the Col de Porte observational record, which falls within the commonly accepted range of snowpack modeling errors at observing stations when models are driven by meteorological observations (Essery et al., 2013; Lafaysse et al., 2017). The mean observed value over the same period is 0.9 ∘C, with bias and RMSD values of −0.1 and 0.6 ∘C, respectively, when comparing SAFRAN with the Col de Porte observational record. The coefficient of determination between SAFRAN and the observations is equal to 0.85. For , the mean observed value is 777 kg m−2, with a bias value of 7 kg m−2 and a RMSD value of 149 kg m−2. The coefficient of determination is equal to 0.74. The interannual fluctuations among GCM–RCM are only correlated between RCMs forced by the same GCM but decorrelated between the different GCMs, as expected.
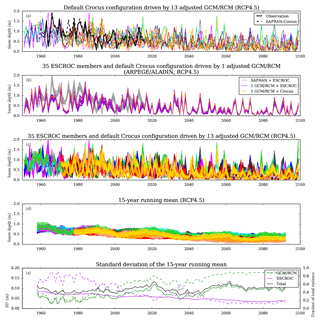
Figure 2Observed and simulated time series of . (a) Continuous time series of annual values of mean winter snow depth data (), either observed or generated by the default snowpack model configuration fed by meteorological data from a reanalysis or an adjusted RCM. (b) values obtained using the ensemble of Crocus model configurations ESCROC. (c) Ensemble of Crocus model configurations driven by the 13 RCP 4.5 GCM–RCM pairs; each GCM–RCM pair is displayed with a different color. (d) Fifteen-year running average values of all simulation members presented in (c). (e) Estimate of absolute and relative contribution of uncertainty components arising from GCM–RCM intermodel variability and multiphysics snowpack model uncertainty (ESCROC).
Figures 2b and 3b show, both using meteorological reanalysis and adjusted climate model data (here one given GCM–RCM pair under RCP4.5 and RCP8.5 climate conditions), the spread of values which can be obtained using the ESCROC E2 ensemble of snowpack model configurations (Lafaysse et al., 2017). The interannual fluctuations are highly correlated between members because they are mainly driven by the GCM–RCM used as input. The plots show by how much the snowpack modeling uncertainty affects the results in terms of mean annual snow depth under two specific climate scenarios (two different RCPs, one GCM–RCM pair).
Figures 2c and 3c show the ensemble of Crocus model configurations driven by the 13 GCM–RCM pairs in the case of RCP4.5 and RCP8.5, each GCM–RCM pair being displayed with a given color. These figures show the large multi-component ensemble of individual annual data which can be generated when combining all available information, which highlights the need for appropriate data synthesis methods. Indeed, it is not possible to draw conclusions or make decisions on the sole basis of such a raw ensemble of individual scenarios.
Figures 2d and 3d present the 13×35 15-year running average values spanning all simulation members of Figs. 2c and 3c, respectively. This corresponds to the second statistical post-processing described in Sect. 2.5.2, which removes the interannual variability and allows an easier quantification of each source of uncertainty.
Figures 2e and 3e aim to apportion the uncertainty in the time series of Figs. 2d and 3d, respectively, between the uncertainty arising from GCM–RCM intermodel variability (including model uncertainty and internal variability of climate at different timescales) and the uncertainty arising from the multiphysics snowpack model. For that purpose, the standard deviations of the 455 values of Figs. 2d and 3d were computed for each 15-year window, and these correspond to the total standard deviations of the . This is shown by the black solid line in Figs. 2e and 3e. Figure 2e displays values on the order of 0.08 to 0.11 m with decadal variability but no temporal trend from 1950 to 2100. Figure 3e, on the other hand, shows a decline of standard deviation with time, as becomes smaller. This standard deviation can be viewed as the total quantified uncertainty level for a given RCP affecting individual values of 15-year averages of . The snowpack multiphysics (referred to as ESCROC) and GCM–RCM uncertainty components were computed based on a further post-processing of the 455 15-year averages for each 15-year window. The ESCROC component was quantified as the mean value of the 13 values (one for each GCM–RCM pair) of the standard deviation of the 35 multiphysics configurations. Similarly, the GCM–RCM component was quantified as the mean value of the 35 values (one for each multiphysics configuration) of the standard deviation of the 13 GCM–RCM pairs. Time series of these individual values are displayed in Figs. 2e and 3e. The ESCROC component shows values ranging from 0.02 to 0.07 m depending on the RCP scenario considered, exhibiting rather smooth fluctuations from 1950 to 2100 and a general decreasing trend, along with the general decreasing trend of over the considered time period (see below). In contrast, the GCM–RCM component shows significant spread, with values from 0.02 to 0.11 m. Note that the assessment of this component for the historical period is affected by the varying number of available GCM–RCM before 1980 and by a potentially artificial reduction of spread over the 1980–2011 calibration period of the ADAMONT statistical adjustment method. This could partly explain why the uncertainty of GCM–RCM appears lower than the multiphysics uncertainty during the historical period, in combination with the deeper snowpack in the historical period. The relative proportion of these two components was estimated as the simple ratio of the corresponding variance values to the total variance value. The variance is used in this comparison because the variances of both factors would be additive if they were independent (the interaction term is neglected here). It shows that the ESCROC component plays in the future period a smaller role than the GCM–RCM component, decreasing over time. This shows that the uncertainty arising from snowpack modeling errors plays a significant (always more than 15 % of variance), although secondary, role for future climate projections. Furthermore, we anticipate that the impact of snowpack modeling uncertainties plays an even smaller role when focussing on relative changes in simulated snow conditions because for one given GCM–RCM the different ESCROC members are usually ranked in a similar order all along the simulation period. For these reasons, we focus below on modeling results solely using the default Crocus model configuration and not the multiphysics ensemble. This is further discussed in the Discussion section.
3.2 Projections of multi-RCP annual quantile values
Fifteen-year sliding quantiles for annual indicators of snow and meteorological conditions are displayed in Fig. 4. Figures for each RCP taken separately are available in the Supplement (Figs. S1–S3). Values for specific time periods (highlighted in Fig. 4) are provided in Table 2 and in Table S1 of the Supplement.
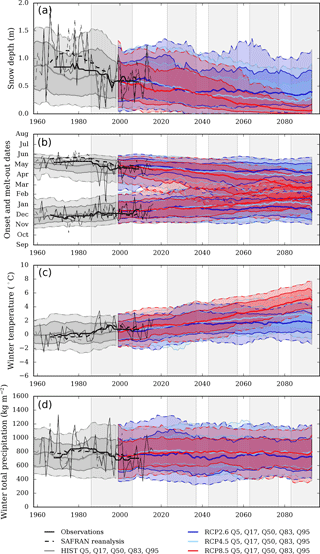
Figure 4Quantile values (5, 17, 50, 83 and 95 %) over 15-year windows of all GCM–RCM pairs (HIST, RCP2.6, RCP4.5 and RCP8.5), along with annual values of observations (1960–2016) and SAFRAN-Crocus runs (1958–2016) and their respective 15-year running medians (bold full and dotted lines, respectively), for (a) , (b) SOD and SMOD, (c) , and (d) . Light grey bars indicate the reference period 1986–2005 and the time slots used in Tables 2–4 and S1–S2.
Table 2Quantile values (Q17=17 %, Q50=50 %, Q83=83 %) over 15-year windows, for the reference period 1986–2005 (Ref) in observations (OBS, only Q50), SAFRAN-Crocus (S-C, only Q50) and historical scenario (HIST, * 13 GCM–RCM pairs, ** 4 GCM–RCM pairs corresponding to the ones in RCP2.6), and around the time slots 2030, 2050, 2070 and 2090 for each future scenario (RCP2.6: 4 pairs; RCP4.5 and RCP8.5: 13 pairs), for , and SOD – SMOD (mm/dd – mm/dd; for RCPs, number of days earlier or later compared to HIST* SOD and SMOD).
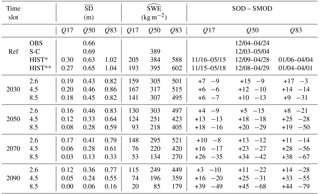
Figure 4 shows the significant interannual variability in snow and meteorologically related indicators in the observations and SAFRAN reanalysis. The observation and reanalysis indicators for snow and meteorological conditions exhibit fluctuations which span the entire range covered by climate projections, under both historical and early 21st century RCPs (the transition between historical and RCP occurs in 2005, which current observations and reanalysis overcross). This indicates that the historical and early 21st century RCPs are consistent with the observed range and interannual variability at the considered location, which corroborates the use of the EURO-CORDEX regional climate simulations together with the ADAMONT method and the Crocus snowpack model to address past and future changes in snow conditions in this mountainous area.
For the reference period 1986–2005, the median of annual values of , snow onset date (SOD) and snow melt-out date (SMOD) is consistent between observations, reanalysis and simulations driven by adjusted historical climate model simulations (HIST using 13 GCM–RCM pairs), with some differences. For example, as can be observed in Table 2, while the SOD median value is similar between observations and simulations (within 1 day), the SMOD median value occurs approximately 10 days later in the reanalysis than in observations, consistent with the 3 cm deviation between the median value of reanalysis-driven and observed . Simulations driven by adjusted historical climate model runs indicate slightly less snow than observations and reanalysis. Similar features can be identified in terms of STED values in Table S1 of the Supplement.
In the case where a smaller number of GCM–RCM pairs are considered for the same time period, HIST – i.e., when only the 4 GCM–RCM pairs for which RCP2.6 model runs are available and not the 13 GCM–RCM pairs for which RCP4.5 and RCP8.5 are available, the indicators calculated for the reference period only taking into account the 4 model pairs available in RCP2.6 (HIST** in Tables 2 and S1) show very small deviation to the values obtained with 13 GCM–RCM pairs. Quantile values differ by up to 3 cm for (≈ 10 %), 14 kg m−2 for (≈ 6 %) and 3 days for SOD and SMOD. For STED quantile values, the largest difference is 5 days (≈ 15 %). This shows that in terms of statistical distributions of annual values of the indicators, the sub-ensemble of four GCM–RCM pairs for which RCP2.6 are available exhibits similar statistical features than the full ensemble of 13 GCM–RCM pairs, in terms of mean trends and spread.
At the scale of 20-year spaced future intervals provided in Tables 2 and S1, all snow-related indicators exhibit a trend towards gradually increased snow scarcity. and quantile values sampled every 20 years generally decrease, SOD increases (later snow onset) and SMOD decreases (earlier snow melt-out date), and STED values decrease. In most cases, climate projections for the 15-year periods centered around 2030 and 2050 depend only slightly, if at all, on the RCP. The periods centered around 2070 and 2090 show significant deviations between RCPs, with reinforced downwards trends for RCP8.5-based indicators, pursued decrease under RCP4.5 and stabilization or reduced decreasing trend for RCP2.6. In comparison to the historical model runs during the reference period 1986–2005, not only the median but also the individual quantile Q17 and Q83 values decrease. However the interquantile Q83–Q17 value remains rather constant throughout the century, in comparison with the reference period, except in the late 21st century under RCP8.5 where snow conditions become increasingly ephemeral. For example, in the case of , the Q83–Q17 value of 0.72 m for the reference period varies for future conditions between 0.62 and 0.67 m for RCP2.6, 0.50 and 0.66 m for RCP4.5 and 0.16 and 0.64 m for RCP8.5 (lowest value at the end of the century). The variability of snow conditions is therefore projected to remain significant, as large as currently encountered as long as snow conditions remain comparable.
The quantile-based coefficient of variation (QCV =(Q83–Q17)∕Q50) for the reference period is equal to 1.14, which means that the spread between the Q17 and Q83 quantile values, which comprise two-thirds of the values potentially obtained for a given winter, exceeds the median value itself, highlighting quantitatively how variable snow conditions can be from one winter to the next. For future conditions, QCV values are never found to be lower than the reference value, and vary between 1.46 and 1.81 for RCP2.6, 1.43 and 2.08 for RCP4.5, and 1.42 and 2.67 for RCP8.5. This indicates that, with the gradual decrease in median and other quantile values for , the interannual/intermodel variability is projected to remain significant and even increase in relative terms (compared to the median value). Very similar results can be obtained when considering . In the case of STED values, however, the situation is different especially for STED50 and STED100 because the number of snow-scarce winters increase will directly lower the Q83 quantile value, while the Q17 quantile value is bounded by 0 and already equal to this value in the early 21st century for all RCPs for STED100 and approaching it by the middle of the 21st century for all RCPs (including RCP2.6) in the case of STED50.
3.3 Projections of multi-RCP multi-annual mean values
Figure 5 represents the mean for the same indicators as Fig. 4. Figures for each RCP taken separately are available in the Supplement (Figs. S4–S6). Table 3 and Table S2 of the Supplement also contain values for specific time slots and for additional indicators. Table 4 lists the relative change in , and for the same time slots compared to the reference period 1986–2005.
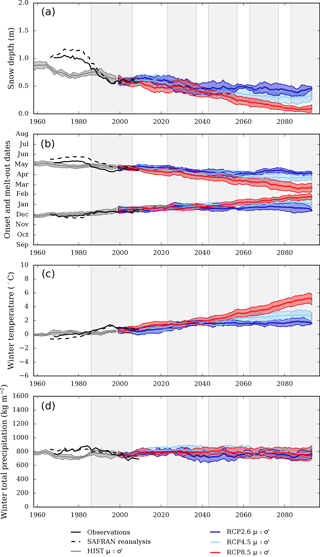
Figure 5Ensemble spread in 15-year running mean (μ ± σ′) of all GCM–RCM pairs for each scenario (HIST, RCP2.6, RCP4.5 and RCP8.5), along with 15-year running means of observations (1960–2016) and SAFRAN-Crocus runs (1958–2016) at CDP, for (a) , (b) SOD and SMOD, (c) , and (d) . Light grey bars indicate the reference period 1986–2005 and the time slots used in Tables 2–4 and S1–S2.
Table 3Values for the mean (μ) of 15-year running means, for the reference period 1986–2005 (Ref) in observations (OBS, only μ), SAFRAN-Crocus (S-C, only μ) and historical scenario (HIST, * 13 GCM–RCM pairs, ** 4 GCM–RCM pairs corresponding to the ones in RCP2.6), and around the time slots 2030, 2050, 2070 and 2090 for each future scenario (RCP2.6: 4 pairs; RCP4.5 and RCP8.5: 13 pairs), for , and SOD – SMOD (mm/dd – mm/dd; for RCPs, number of days earlier or later compared to HIST* SOD and SMOD).
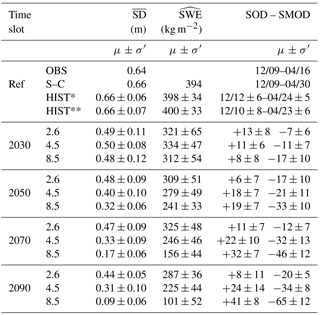
Table 4Reference values of , and for the period 1986–2005 (Ref) from observations (OBS, only μ), SAFRAN (SAF, only μ) and the historical scenario (HIST, * 13 GCM–RCM pairs, ** 4 GCM–RCM pairs corresponding to the ones in RCP2.6). Change () in those indicators (, and ) for the same time slots and RCPs as in previous tables, compared to the reference period 1986–2005 in HIST*.
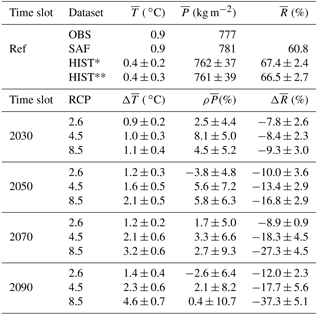
In contrast to Fig. 4, by design Fig. 5 suppresses most of the effects of the interannual variability, focussing on long-term trends and highlighting the uncertainty components originating from global and regional climate models. As illustrated in Tables 3 and S2, the uncertainty pertaining to multi-annual/multi-model averages is computed based on the standard deviation of the mean of the multi-model multi-annual averages over sliding time periods, as described above. Values for σ′ (=0.95 σ) are generally lower for the HIST 1986–2005 period than for the future periods centered on 2030, 2050, 2070 and 2090. For example, σ′ for over the HIST 1986–2005 period is equal to 0.06 m, while for all future periods it is rather on the order of 0.06–0.12 m, except for RCP8.5 towards the end of the century, with σ′ values 0.06 m, but associated with significantly lower μ values on the order of 0.09–0.17 m. A similar observation can be made for , SOD, SMOD and STED values.
In terms of absolute values, as illustrated in Fig. 5, and indicated in Tables 3 and S2, the historical model runs for the reference period 1986–2005 are characterized by about the same amounts of snow on average as in the observations and reanalysis data. This is consistent with the only slight deviation observed between median values in the previous section. As shown in Fig. 5a, the decadal dynamics however differs, with snow conditions (observed and reanalyzed) showing rather stable conditions in the 1970s followed by abrupt change in the mid-1980s, followed by another period of relative stability. Simulations driven by climate model data show a different pattern of changes, with an earlier reduction in the 1970s, followed by a relative increase in the 1980s followed by another reduction in the 1990s onwards. The length of the observation, reanalysis and historical climate records is too small to generalize, but all three sources of information point towards low-frequency fluctuations at the decadal timescale, superimposing on a long-term trend of general snow reduction.
At the scale of 20-year spaced future intervals provided in Tables 3 and S2, similarly to the results of the annual quantiles approach, all snow-related indicators exhibit a trend towards gradually increased snow scarcity. Also similarly, in most cases, climate projections for the 15-year periods centered around 2030 and 2050 depend only slightly, if at all, on the RCP, the periods centered around 2070 and 2090 show significant deviations between RCPs, with reinforced downwards trends for RCP8.5-based indicators, pursued decrease under RCP4.5 and stabilization or reduced decreasing trend for RCP2.6.
Similarly to the previous section, the values of the indicators are calculated for the reference period either taking into account the 4 model pairs available in RCP2.6 (HIST**) or the 13 pairs for which RCP4.5 and RCP8.5 are available; see in Tables 3–4 and S2. Mean values are only slightly impacted for some indicators (e.g., for or ). This shows that at the interannual timescales, the sub-ensemble of four GCM–RCM pairs for which RCP2.6 are available exhibits similar statistical features than the full ensemble of 13 GCM–RCM pairs, in terms of mean trends and spread.
The coefficient of variation (CV ) for the reference period is equal to 0.18, which illustrates well the suppression of the interannual variability effect. This corresponds to only 16 % of the QCV (see above), which indicates that for the reference period and for this case, the interannual variability of annual indicator values plays a stronger role than the intermodel spread for a given year. For future conditions, CV tends to increase, but this is more due to a decrease in μ in all cases than to σ′ differences, as shown above. CV always remains smaller than QCV, which indicates that, regardless of the scenario and the time period in the future, the variability/uncertainty related to the intermodel spread (for a given RCP and time period) always remains lower than the interannual fluctuations.
Table 4 provides a summary of the meteorological conditions associated with the past and future snow conditions addressed in this study, in terms of multi-annual means. While the mean winter temperature value for the reference period 1986–2005 is on the order of 0.4–0.9 ∘C in the Chartreuse mountain range at 1500 m depending on whether the SAFRAN reanalysis or the historical climate runs are considered, the 15-year period centered on 2030 already exhibits a mean increase of ∘C regardless of the RCP. The results for the three RCPs already differentiate for the 2050 lead time, and the difference continues to widen until the end of the century with ∘C for RCP2.6, ∘C for RCP4.5 and ∘C for RCP8.5. While the temperature trends are unequivocal, there is no significant trend for total winter precipitation, as shown in Table 4. The snow / rain precipitation ratio is projected to evolve markedly along with the temperature rise, with a maximum reduction by 37.3 ± 5.1 % of the snow precipitation share over the total winter precipitation.
3.4 Relationship between global temperature trends and local snow and meteorological conditions
Figure 6 shows the relationships between computed changes in the snow and meteorological indicators between 1986–2005 (reference period for this study) and three future time periods (beginning of century (BOC), 2011–2040; middle of century (MOD), 2041–2070; and end of century (EOC), 2071–2100), and the corresponding global temperature changes simulated by the driving GCM. This figure uses as a reference (lower axis). The corresponding relationship to is also shown (upper axis), which consists in a shift of 0.62 ∘C (), although individual values range from 0.19 to 0.84 ∘C depending on the GCM. Regressions were, however, computed using the values of , and for each GCM, as well as all three future periods taken together. Table 5 shows the slope (per global ∘C difference with the Ref value) of the change of the local indicator, as well as the coefficient of determination. With the notable exception of the cumulated winter precipitation , all indicators show a consistent relationship with ΔTg. The slope of the regression curve is very similar for all three future time periods – BOC, MOC and EOC – as well as when all future time periods are pooled together. The maximum correlation is found for the snow precipitation ratio with a coefficient of determination of 0.90, followed by local air temperature with a coefficient of determination of 0.86. The worst correlation is found for STED100 (R2=0.48 for all time periods). All snow-related indicators R2 values range between 0.76 and 0.83 (for all future time periods together), with a trend to lower values for BOC only time period, and higher values for EOC and all time periods together. The slope of the regression curve, in terms of % change per global ∘C difference with the Ref value, is larger for (about −25 % ∘C−1) than for (−20 % ∘C−1). Similarly to previous sections, the SOD and SMOD changes are not symmetrical, i.e., the date of snowpack onset exhibits a lower relative reduction (12 days per global ∘C difference with the Ref value) than the date of snowpack melt out (17 days per global ∘C difference with the Ref value). Taking the sum of absolute values of SOD and SMOD as a measure of the changes in total snow season length, it is found that the total snow season length is decreased by 29 days, i.e., about one month, per global ∘C difference with the Ref value. The slope of the local temperature regression curve is 1.1 ∘C ∘C−1, which indicates that the local rate of warming only slightly exceeds the global warming rate during the 21st century, using this method.
Relating to specific target values of global surface air temperature changes since the preindustrial period, Fig. 6 and the data provided in Table 6 show for example that for a global temperature increase of 1.5 ∘C compared to the preindustrial period, the mean change of mean snow depth at 1500 m altitude in the Chartreuse mountain range is on the order of −25 %, and this value increases very rapidly with increasing global temperature changes, reaching reductions of 65 % for 3 ∘C global temperature rise, and even 80 % reduction passed 4 ∘C temperature rise. However, for a given value, model runs spanning several tens of % reduction rate can be sampled (e.g., around 2 ∘C), showing that the relationship between global temperature values and local impacts is not unequivocal. This is materialized by the standard deviation provided in Table 6. The same applies in terms of trends to all local meteorological and snow indicators (except total precipitation, as noted before).
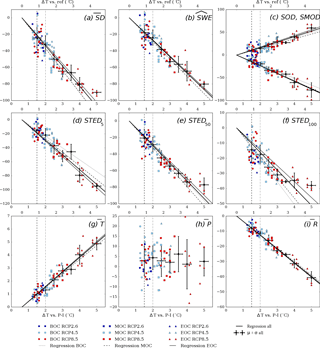
Figure 6Response of local meteorological and snow indicators to global warming level. Indicator response is computed as the difference of multi-annual means between end of century (EOC, 2071–2100), middle of century (MOC, 2041–2070), or beginning of century (BOC, 2011–2040) and the reference period (Ref, 1986–2005). Global warming level is computed as the difference in global mean surface air temperature between EOC, MOC or BOC and either the reference period (top axes) or the preindustrial period (P-I, 1851–1880) (lower axes). Each point corresponds to a snow or meteorological indicator computed using a given RCP and one GCM–RCM pair, for which the global surface air temperature change is inferred from the corresponding GCM run: (a) (%), (b) (%), (c) SOD and SMOD (days), (d) STED5 (days), (e) STED50 (days), (f) STED100 (days), (g) (∘C), (h) (%), and (i) (%). Warming levels of 1.5 and 2 ∘C compared to preindustrial are shown with the vertical dashed lines. Regression lines are shown for the response at EOC, MOC, BOC or all three periods (ALL) (except for ). Mean values and standard deviations among ALL changes in each indicator for 0.5 ∘C intervals (±0.25 ∘C) are displayed as error bars.
Table 5Slope (α, unit indicated inside brackets) and determination coefficient (R2, no unit) of linear regressions of the changes in indicators between BOC, MOC, EOC or ALL and the reference period (1986–2005) and corresponding global temperature rise since 1986–2005. is not shown. The number of values used for each regression is indicated inside brackets.
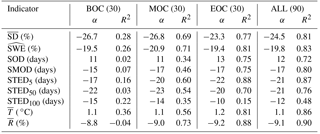
This study is based on a multi-component ensemble framework in order to provide future values of meteorological and snow conditions at a typical mid-altitude (1500 m) mountain range in the Northern French Alps, accounting for these uncertainty and variability sources in the most consistent and rigorous possible manner. To this end, a multi-component ensemble framework was designed and built, addressing various sources of uncertainty and variability, i.e., several RCPs (RCP 2.6, RCP 4.5 and RCP8.5), feeding several GCM runs from the CMIP5 intercomparison exercise, which themselves feed various RCM runs as part of the EURO-CORDEX downscaling exercise, which are adjusted using the ADAMONT method against the meteorological reanalysis product SAFRAN, making it possible to drive a multi-physical version of the energy balance multi-layer snowpack model Crocus. Here we discuss the results obtained for the period from 1950 to 2100, in comparison to reanalysis and comparable observation data for the past period, and with other existing scientific studies for future conditions.
4.1 On the comparability between adjusted historical climate model runs and observations and reanalyses
As shown in Sect. 3.1, SAFRAN and Crocus (either multiphysics or default configuration) results show acceptable performance metrics compared to in situ observations of meteorological conditions and snow conditions, respectively. By definition no performance metrics pertaining to annual fluctuations can be computed between the adjusted climate output and either observations or reanalysis data, because the two are not designed to exhibit synchronous fluctuations. Only multi-annual statistics may be compared, under certain assumptions, which is done in Sect. 3.2 and 3.3, for the snow indicators defined in this study. Indeed, even over a timescale of 20 years, it is likely and even expected that low-frequency variability in the climate, in nature and as it is represented in GCMs, leads to deviations at this timescale, which the statistical adjustment method can only partially mitigate. For the reference period 1986–2005, the match between observation and reanalysis data and historical GCM–RCM runs is nevertheless satisfying. However, it is also clear from Figs. 4 and 5 that the match is not as good for a period extending back into the past, with a tendency for adjusted climate model data to provide reduced snow conditions compared to observed and reanalyzed data for the period before 1985. While the reasons for such a behavior are likely multiple, it is certainly influenced by the fact that this period is almost independent from the time period for calibration of the ADAMONT adjustment method (1980–2011), and during which major climate shifts occurred (Reid et al., 2015). This could also be due to the fact that Crocus model outputs result from the interaction between various meteorological variables, both in terms of mean values and their day-to-day fluctuations, especially precipitation and temperature conditions which together yield either to rain or snow precipitation. By design, the ADAMONT method adjusts the variables independently from each other (Verfaillie et al., 2017). Even if special care is taken to minimize the disadvantages of this approach, such as the use of weather regimes for the quantile mapping statistical adjustment method, or applying the final quantile mapping separately to rain and snow precipitation in order to mitigate detrimental interactions between temperature and precipitation (Verfaillie et al., 2017), some interaction terms probably remain uncorrected. The adjustment method also probably exerts an influence on the variability during the historical period, which may be responsible for the overall lower spread (expressed in terms of either quantile-based coefficient of variation in annual values or the coefficient of variation of the interannual means) compared to future projections. Indeed, by design the adjustment method attempts to bring reanalysis meteorological data and historical model runs to the same ground in terms of quantile distributions, which inevitably reduces the spread between different GCM–RCM pairs. This is visible in the analyzed results, because the reference time period used (1986–2005) is included in the period used for the statistical adjustment method. In addition, the lower spread, compared to future periods of 15 years, could also be due to the fact that the reference period is longer than the future time periods considered, so that a wider range of climate conditions are sampled in the multi-annual mean, thereby bringing closer the values originating from the various RCMs.
4.2 Uncertainty and variability sources
The study uses multi-component ensembles to address uncertainty and variability sources, which are analyzed through indicators computed using various sub-ensembles. Based on the results shown above, it clearly appears that snowpack modeling errors, due to uncertain physical knowledge of processes at play and their imperfect implementation in the model, can be responsible for a significant fraction of the uncertainty pertaining to future climate projections, consistent with previous results obtained based on observations at instrumented sites (Essery et al., 2013; Lafaysse et al., 2017). While this must be taken into account for a fully comprehensive assessment, evidence from this study suggests that, under the conditions of the Northern French Alps and after the middle of the 21st century, the uncertainty component attributed to the snowpack modeling errors alone is on the order of 20 %, which is significant but of second order compared to the spread originating from multiple climate models.
Because the number of GCM–RCM pairs was different for RCP2.6 (4) and RCP4.5 and RCP8.5 (13), we compared the statistics for indicators during the historical period based on the 4 RCP2.6 pairs alone, as well as the full ensemble of 13 GCM–RCM pairs. Both in terms of statistics distributions of annual values for a period of 20 years (1986–2005) or in terms of multi-model spread of multi-annual average values, results were extremely close for the full and sub-ensemble. While it remains desirable, when possible, to use the largest possible number of different GCM–RCM pairs in order to mitigate the impact of multi-model variability and climate internal variability, this tends to show that, in this case, robust results can be obtained using a subset of a few models dealt with appropriately. However, as shown in Fig. 6, individual GCM–RCM pairs only sample the range of possible future climate conditions imperfectly, so that choosing, randomly or not, too small a number of GCM–RCM pairs would inevitably lead to biased results. This is consistent with the fact that the variability of snow conditions is primarily dominated by interannual variability, over which intermodel spread superimposes an additional uncertainty component. It is very likely that the 4 GCM–RCM pairs used in this study, which feature RCP2.6, RCP4.5 and RCP8.5 model results, possess appropriate interannual variability properties and overall no major deviation from the average behavior of the full ensemble of 13 GCM–RCM pairs, which leads to the fact that similar statistics are found for these 4 model pairs as for the full ensemble of 13. It is not certain that a similar result would be obtained by picking randomly four GCM–RCM pairs within the full ensemble available (see Fig. 6 for contrasted individual model behavior).
4.3 General trends and added value of the approach developed
That natural snow conditions at 1500 m in the Northern French Alps are projected to decrease under ongoing climate change is an expected result, which deserves, however, to be put in perspective with other existing studies on the matter. Figures 4–5 and Tables 2 and 3 indicate a general decreasing trend in towards the end of the century (≈ −0.8 cm per decade for RCP2.6, −3.2 cm per decade for RCP4.5 and −6.5 cm per decade for RCP8.5 over the period 2030–2090), accompanied by a shortening of the snow season (later SOD and earlier SMOD). This is consistent with previous results from Steger et al. (2013) for the 1000–1500 m a.s.l. range in the European Alps. The magnitude of the decrease is similar to the one found by Marty et al. (2017a) for the Aare and Grisons regions in Switzerland, although their GCM–RCMs and future scenarios differ from ours. This trend is visible for all scenarios, but stronger for RCP8.5. At the end of the century, simulations carried out under this scenario predict an increasingly ephemeral snow cover (multi-annual mean value of 9±6 cm for the 2090 time slot; see Table 3) and more frequent seasons with barely any snow on the ground (Figs. 4–5 and Tables 2 and 3). The shortening of the snow season is projected to become asymmetric towards the end of the century, with a stronger reduction in spring than in autumn (Tables 2 and 3), similar to findings from Steger et al. (2013) and Marty et al. (2017a). The decreasing trend is also combined with a decreasing trend (≈ −6 kg m−2 per decade for RCP2.6, −18 kg m−2 per decade for RCP4.5 and −35 kg m−2 per decade for RCP8.5 over the period 2030–2090, Table 3) and decreasing trends of STED5 (as in Marty et al., 2017a), STED50 and STED100 (Table S2).
Figures 4–5 also indicate a strong increasing trend in for the 21st century (≈ +0.08 ∘C decade−1 for RCP2.6, +0.22 ∘C decade−1 for RCP4.5 and +0.58 ∘C decade−1 for RCP8.5 over the period 2030–2090), but no significant trend in . Compared to the reference period 1986–2005, increases by 1.4 ± 0.4 ∘C in 2090 for scenario RCP2.6, 2.3 ± 0.6 ∘C for scenario RCP4.5 and 4.6 ± 0.7 ∘C for scenario RCP8.5 (Table 4). Values for the change in and are comparable to Steger et al. (2013) and Marty et al. (2017a), even though their GCM–RCMs and future scenarios differ from ours. The insignificant trend in and its variable sign depending on the projections are fully consistent with previous studies identifying the internal variability of climate as the main uncertainty component for precipitation in the Alpine region all along the 21st century (Fatichi et al., 2014; Lafaysse et al., 2014). Table 4 further shows a strong decrease in (by 2090, −12.0 ± 2.3 % for RCP2.6, −17.7 ± 5.6 % for RCP4.5 and −37.3 ± 5.1 % for RCP8.5, compared to 1986–2005), with values very similar to Frei et al. (2018).
The comparison of trends of meteorological indicators (temperature, total precipitation and ratio of snow to total precipitation) and indicators characterizing the state of snow on the ground provides insights into the physical mechanisms responsible for changes in snow conditions. The snowpack is progressively initiated and complemented by precipitation events during the wintertime, and it is thus unsurprising and consistent with previous evidence that the decline in snow precipitation is one of the main factors responsible for the decline in snow conditions, even if total precipitation does not exhibit any significant trend (Beniston et al., 2018; Castebrunet et al., 2014; Gobiet et al., 2014; Lafaysse et al., 2014; Schmucki et al., 2014; Steger et al., 2013). That the reduction of the snow season is asymmetrical with a stronger reduction in the spring than in autumn is consistent with the fact that snow precipitation amounts drive not only the response of the snowpack to climate change but also the intensity of the melt rate, which also depends on atmospheric conditions and is enhanced under warmer conditions (Pierce and Cayan, 2013; Steger et al., 2013). The datasets underpinning the present study could be used to address in a more quantitative manner the physical processes responsible for the results of the simulations; however, this falls beyond the scope of this study (Pierce and Cayan, 2013).
Beyond the general trends, which provide an unsurprising – yet required – update of previous assessments based on older climate scenarios applied to the French Alps (Castebrunet et al., 2014; Piazza et al., 2014; Rousselot et al., 2012), the main added value of the approach developed here lies in its ability to capture high-order moments of possible snow futures. For example, that the year-to-year variability of snow conditions on the ground remains as large as currently, and even increases in relative terms (until the middle of the century for all RCPs, and towards the end of the century for all RCPs except RCP8.5), may be of equal, if not higher, significance to stakeholders operating in the alpine environment than the long-term trends. Such results can only be attained making use of a sufficiently large number of independent global and regional climate models, the EURO-CORDEX database corresponding to a significant achievement of the climate modeling community enabling such impact studies to take place.
Many of the results discussed above indicate a strong consistency between our results and results obtained using delta-change methods, in French mountain regions as well as in Switzerland (Castebrunet et al., 2014; Schmucki et al., 2014). This consistency is shown for multi-annual multi-model trends on snow depth or snow water equivalent mean values, but it cannot be assessed regarding the interannual variability because this is generally not addressed in these studies. The model chain implemented here, explicitly making use of the intra-seasonal and interseasonal RCM chronology, inherently captures more appropriately potential changes in timing of meteorological conditions, in particular precipitation. Differences between the current study and studies based on delta-change approaches would be expected under a situation where the chronology of precipitation would differ significantly in the future, because the delta-change approach would only modify the air temperature and rain–snow partitioning, and not the timing of the events. These changes in the multivariate chronology of meteorological events in the Alpine region have not been investigated in details until now to the best of our knowledge, although their stationarity is a requirement for the validity of the delta-change method. Furthermore, although our results do not exhibit significant changes in the interannual variability of the snow indicators, this is a result of our projections, whereas it is only an assumption when applying a delta-change method. More in-depth comparisons between outputs of delta-change approaches and direct adjustments to RCM output could be carried out in the future but are beyond the scope of this article.
4.4 Link with global temperature increase
The international framework for climate negotiations, culminating at the yearly Conferences Of Parties (COP), and basing the technical part of its decision process on IPCC assessments, shows a strong tendency to focus on global temperature changes. In recent years, there has been increasing societal demand for quantifying the local impacts of global warming levels since the preindustrial time period of 1.5, 2 ∘C and beyond. While for a number of reasons this approach is limited and only partially represents climate change (James et al., 2017; Millar et al., 2017; Rogelj et al., 2015), its infusion in the public debate at all levels, from the international, national and even local level, makes it relevant to discuss and illustrate local impacts of global climate change. With Fig. 6 and Tables 5 and 6 we provide such a link, thereby highlighting the specific sensitivity of the mountain meteorological and snow conditions to global climate conditions. Such figures allow stakeholders interested in snow and meteorological conditions at the local scale to directly infer the consequences of climate policies in their socioeconomic domain (James et al., 2017; Marty et al., 2017a). However, using only such an approach with a focus on the end of the 21st century may lower the impact of the results and the motivation of stakeholders if the consequences appear too distant in time. The power of the approach shown in this article is that it not only makes it possible to infer EOC impacts of climate change but also provides a continuous vision of past and current climate context, and its most likely evolution according to state-of-the-art GCM–RCM pairs driven by RCPs. Furthermore, our results indicate that the response of local meteorological and snow conditions is essentially the same regardless of whether data from the beginning or end of the century are sampled. This indicates that the seasonal snowpack at this location and altitude level responds in a linear and reversible way to global-scale climate change, and the near-term and mid-term responses can be used, in addition to the end-of-century information, to infer the relationship between local and global conditions using a larger dataset, thereby providing more robust assessments of the influence of the global air temperature on local snow and meteorological data. This is all the more relevant in that none of the GCMs used for this study predict EOC warming below 1.5 ∘C compared to preindustrial levels, so that using less distant future time periods makes it possible to assess the response of the local snow conditions to 1.5 and 2 ∘C difference in a more robust way than EOC only (see Table 6) (James et al., 2017). Even for the lowest level of global warming, none of the model results predict that local snow conditions will be unaffected by climate change, the minimum level of decrease in mean winter snow depth being on the order of 25 % for 1.5 ∘C global increase since the preindustrial period.
In more details, these results highlight several discussion points. First of all, it is remarkable that the regression line of the local mean winter temperature with global temperature increase shows a slope of 1.1 ∘C ∘C−1, which represents a low additional warming of the mountain environment in contrast to previous studies (Durand et al., 2009a; Pepin et al., 2015). This result may stem in part from the fact that although elevation dependent warming is generally maximal in the fall and springtime, our target period mostly covers wintertime. Alternatively, this low enhancement factor could be due to the fact that the RCM grid points used for our analysis are at lower altitudes, from 612 to 1085 m, with a mean value across all RCMs of 880 m. Snow conditions at such altitude levels are generally limited already at present time, so that the local snow albedo feedback which considerably drives the elevation-dependent warming (Pepin et al., 2015) may be limited at such a low elevation. Addressing this issue in more detail is left open for future research, as it may imply that the temperature trends identified in this study are underestimated for this reason. Second, it is interesting to note that the relationship between snow conditions and global air temperature is different for winter mean snow depth and peak SWE. The latter shows a lower sensitivity (−20 % ∘C−1) than mean snow depth (−25 % ∘C−1); see Table 5. While this is first due to the different nature of the indicators (peak SWE value vs. mean winter snow depth value), this may also be due to the fact that rain-on-snow events (whose frequency is projected to increase) can positively contribute to SWE, through refreezing of the precipitation water in the snowpack, while not contributing to increasing snow depth. This shows that the difference of response of the snow-related indicators must be carefully assessed depending on the target environmental or socioeconomic domain of interest, because specific snow-related variables may provide distinct messages regarding their impact (Pierce and Cayan, 2013). While global temperature is well correlated to the snow indicators, the slope of the regression curve is not the same for all indicators, illustrating the usefulness of using a detailed snowpack model to predict the impact of climate change of snow conditions, accounting for a maximum amount of processes operating at the boundaries and within the snowpack. Nevertheless, the significant correlation between 30-year average global temperature difference to preindustrial levels of the GCMs and the local effects on air temperature and snow conditions simulated using the same driving GCMs processed by means of a cascade of physically based (RCM) and statistical (ADAMONT) downscaling and adjustment methods, followed by the use a multi-layer energy and mass balance snowpack model (Crocus), is consistent with the fact that (i) 30-year average regional and local temperature in the European Alps is strongly and directly influenced by the global climate and (ii) the multi-annual mean response of the snowpack at 1500 m altitude is substantially governed by and responds to multi-annual mean local air temperature.
This study introduced a multi-component ensemble framework in order to provide future values of meteorological and snow conditions in mountainous regions, exemplified for a typical mid-altitude (1500 m) mountain range in the Northern French Alps. The multi-component ensemble framework makes it possible to account for the various sources of uncertainty and variability that affect future climate projections, some of which are neglected in both previous and ongoing climate change impact studies. The multi-ensemble framework developed here draws on several RCPs (RCP 2.6, RCP 4.5 and RCP8.5), feeding several GCM runs from the CMIP5 intercomparison exercise, which themselves feed various RCP model runs from the EURO-CORDEX downscaling exercise. Those are adjusted using the refined quantile mapping method ADAMONT against the meteorological reanalysis SAFRAN, making it possible to drive a multi-physical version of the energy balance multi-layer snowpack model Crocus. The method defines a series of annual snow and meteorological indicators that represent various aspects of the winter season (mean annual snow depth, peak Snow Water Equivalent, date of inception and melt out of the snowpack, mean air temperature, cumulated winter precipitation etc.), which are computed from daily values of the variables representing meteorological and snow conditions (here temperature, precipitation, snow depth and SWE).
Based on an analysis of various sub-ensembles of past, current and future observations and simulations, spanning the period from 1950 to 2100, and focussing on this particular yet representative geographical setting, the main conclusions of this study are as follows:
-
Uncertainty arising from physical modeling of snow after the middle of the century can account for up to typically 20 % of the simulation results, although the multiphysics is likely to have a much smaller impact on trends, because of the systematic nature of a large fraction of the error sources considered.
-
The ADAMONT method appropriately adjusts the output of the EURO-CORDEX GCM–RCM runs, making it possible to drive an energy balance land surface model such as Crocus based on the chronology of the driving climate model, thereby leveraging the caveats of using delta-change methods applied to past observations, which do not make it possible to take into account differences in seasonality or climatically variable weather patterns (blocking, extreme precipitation events, etc.). The method can be readily applied to the next generation of climate model runs, generated using refined greenhouse gas emission scenarios and/or improved model components (Millar et al., 2017; Rogelj et al., 2015). This should make it possible to update climate change impact assessments more quickly than previously, thereby reducing the phase lag between the production of assessments of global, regional and local climate change and of its impacts.
-
The four GCM–RCMs within the EURO-CORDEX ensemble, which provided not only RCP4.5 and RCP8.5 but also RCP2.6 model runs, exhibit similar statistics at the interannual and multi-annual scale as the full 13-member ensemble, making results obtained for RCP2.6 comparable with results obtained for RCP4.5 and RCP8.5 even though they are not based on the same number of models. This result may not generalize to any sub-ensemble of the available GCM–RCM runs of EURO-CORDEX; therefore, we consider it preferable to use as many as possible GCM–RCM runs in ensemble-based assessments.
-
Projections of meteorological and snow conditions corresponding to RCP2.6, RCP4.5 and RCP8.5 show similar behavior until the middle of the 21st century. They all exhibit significant interannual variability, and a long-term trend of increasing snow scarcity. Our study shows that, for this location, the interannual variability is larger than intermodel spread for a given RCP.
-
The impact of the RCP becomes significant for the second half of the 21st century, with overall stable conditions under the RCP2.6 scenario, and continued degradation of snow conditions along with increased air temperature for RCP4.5 and 8.5, the latter leading to frequent occurrence of ephemeral or nearly snow-free conditions at the end of the century.
-
Changes in local meteorological and snow conditions show significant correlations with global temperature levels (using 30-year means), with respect to preindustrial levels. For example, the change in mean snow depth at 1500 m altitude in the Chartreuse mountain range is on the order of −25 and −32 % for a 1.5 and 2 ∘C global temperature rise, respectively, with respect to preindustrial levels, and the magnitude of the impact consistently increases along with global mean temperature reaching reductions of 80 % for 4 ∘C of global warming.
While this work provides scientific results directly exploitable for snow and meteorological conditions at 1500 m altitude in the Chartreuse mountain range, our results do not directly allow extrapolation of the conclusions in other mountain regions in France or other elevations. It is, however, expected that the response of neighboring mountain ranges may be comparable at the same altitude level, because their behavior in the past (Durand et al., 2009a, b) and in previous studies addressing future changes (Castebrunet et al., 2014; Rousselot et al., 2012) was generally rather similar. This remains to be explored more quantitatively and will be the topic of upcoming studies, based on the methodological framework introduced here and the data available in the SAFRAN reanalysis for the French Alps and Pyrenees (Durand et al., 2009a, b; Maris et al., 2009). The method can obviously be applied beyond French borders, provided that an adequate long-term observational dataset can be used as a basis for RCM output adjustment using the ADAMONT method (Verfaillie et al., 2017).
Beyond the geographical scope, which can be extended to address a wider diversity of territorial climate-related challenges, sector-specific further applications can now be considered. For example, the adjusted climate scenarios can be projected on sloping surfaces, making it possible to address the impact of climate change on avalanche hazard using Crocus model runs, thereby upgrading and consolidating the results of Castebrunet et al. (2014). Also, the adjusted climate scenarios could be employed to simulate snow conditions on ski slopes in French ski resorts, drawing on the method developed by François et al. (2014) to be applied using the version of Crocus accounting explicitly for snowmaking and grooming (Spandre et al., 2016b). This method has shown significant potential to account simultaneously for the impact of natural snow precipitation and temperature conditions (driving the capability to produce snow) on the operating capabilities of alpine ski resorts over the past decades (Spandre et al., 2018). It is now ready to be applied for future conditions, drawing on the framework developed in this study. It must be emphasized that while the projected changes in meteorological and natural snow conditions shown in this work are likely to affect operating conditions of ski resorts, no quantitative conclusions can be drawn on this topic. Indeed, snow management practices, especially snowmaking, play an essential role in their operations, and they should be accounted for in studies specifically addressing the impact of climate change on this socioeconomic sector (Hanzer et al., 2014; Spandre et al., 2016b; Steiger et al., 2017). Beyond these applications to seasonal snow, the method is ready to use for a wide range of environmental impact studies addressing various mountain features potentially affected by climate change, such as natural hazards, cryospheric components (glaciers and permafrost), water resources (including hydropower), ecosystems functioning and the impact of their changes on human societies.
Data used in this research are accessible upon request to the authors. Note that the climate projection data are progressively being uploaded and made available on the French Climate Services portal Drias http://www.drias-climat.fr/.
The supplement related to this article is available online at: https://doi.org/10.5194/tc-12-1249-2018-supplement.
The authors declare that they have no conflict of interest.
We thank CEN staff members for contributing to observational data acquisition
at the Col de Porte site (Marie Dumont, Bernard Lesaffre, Philippe Lapalus,
Jean-Michel Panel, Daniel Poncet, Pierre David and Marcel Sudul) and the
SAFRAN reanalysis (Yves Durand, Gérald Giraud, Laurent Mérindol and
Matthieu Vernay). Fruitful discussions and interactions with colleagues at
Météo-France (CNRM, Samuel Somot, Serge Planton and Hervé Douville, and
DCSC, Jean-Michel Soubeyroux) were beneficial for the present study, too.
This study benefited from funding from the French Ministry for Ecology (MTES)
through the GICC program and ONERC, in the framework of the ADAMONT project,
from the Interreg project POCTEFA/Clim'Py and from the IDEX Univ. Grenoble
Alpes Cross Disciplinary Project “Trajectories”. CNRM/CEN and Irstea are
part of LabEX OSUG@2020 (ANR10 LABX56). We thank the two anonymous reviewers
and the editor, Ross Brown, for useful and constructive
comments.
Edited by: Ross Brown
Reviewed by: two anonymous referees
Abegg, B., Agrawala, S., Crick, F., and de Montfalcon, A.: Climate change impacts and adaptation in winter tourism, in: Climate Change in the European Alps, edited by: Agrawala, S., OECD Paris, 25–60, https://doi.org/10.1787/9789264031692-en, 2007. a
Beniston, M.: Variations of snow depth and duration in the Swiss Alps over the last 50 years: links to changes in large-scale climatic forcings, Climatic Change, 36, 281–300, https://doi.org/10.1023/A:1005310214361, 1997. a
Beniston, M., Farinotti, D., Stoffel, M., Andreassen, L. M., Coppola, E., Eckert, N., Fantini, A., Giacona, F., Hauck, C., Huss, M., Huwald, H., Lehning, M., López-Moreno, J.-I., Magnusson, J., Marty, C., Morán-Tejéda, E., Morin, S., Naaim, M., Provenzale, A., Rabatel, A., Six, D., Stötter, J., Strasser, U., Terzago, S., and Vincent, C.: The European mountain cryosphere: a review of its current state, trends, and future challenges, The Cryosphere, 12, 759–794, https://doi.org/10.5194/tc-12-759-2018, 2018. a, b
Bosshard, T., Kotlarski, S., Zappa, M., and Schär, C.: Hydrological climate-impact projections for the Rhine River: GCM–RCM uncertainty and separate temperature and precipitation effects, J. Hydrometeorol., 15, 697–713, https://doi.org/10.1175/JHM-D-12-098.1, 2014. a
Boulangeat, I., Georges, D., Dentant, C., Bonet, R., Van Es, J., Abdulhak, S., Zimmermann, N., and Thuiller, W.: Anticipating the spatio-temporal response of plant diversity and vegetation structure to climate and land use change in a protected area, Ecography, 37, 1230–1239, https://doi.org/10.1111/ecog.00694, 2014. a
Brasseur, G. and Gallardo, L.: Climate services: Lessons learned and future prospects, Earth's Future, 4, 79–89, https://doi.org/10.1002/2015EF000338, 2016. a
Brown, R. D. and Mote, P. W.: The response of Northern Hemisphere snow cover to a changing climate, J. Climate, 22, 2124–2145, https://doi.org/10.1175/2008JCLI2665.1, 2009. a
Castebrunet, H., Eckert, N., Giraud, G., Durand, Y., and Morin, S.: Projected changes of snow conditions and avalanche activity in a warming climate: the French Alps over the 2020–2050 and 2070–2100 periods, The Cryosphere, 8, 1673–1697, https://doi.org/10.5194/tc-8-1673-2014, 2014. a, b, c, d, e, f, g, h
Christensen, J., Boberg, F., Christensen, O., and Lucas-Picher, P.: On the need for bias correction of regional climate change projections of temperature and precipitation, Geophys. Res. Lett., 35, L20709, https://doi.org/10.1029/2008GL035694, 2008. a
Déqué, M.: Frequency of precipitation and temperature extremes over France in an anthropogenic scenario: Model results and statistical correction according to observed values, Global Planet. Change, 57, 16–26, https://doi.org/10.1016/j.gloplacha.2006.11.030, 2007. a
Durand, Y., Brun, E., Mérindol, L., Guyomarc'h, G., Lesaffre, B., and Martin, E.: A meteorological estimation of relevant parameters for snow models, Ann. Glaciol., 18, 65–71, 1993. a, b, c
Durand, Y., Giraud, G., Brun, E., Mérindol, L., and Martin, E.: A computer-based system simulating snowpack structures as a tool for regional avalanche forecasting, J. Glaciol., 45, 469–484, 1999. a
Durand, Y., Giraud, G., Laternser, M., Etchevers, P., Mérindol, L., and Lesaffre, B.: Reanalysis of 47 Years of Climate in the French Alps (1958–2005): Climatology and Trends for Snow Cover, J. Appl. Meteorol. Clim., 48, 2487–2512, https://doi.org/10.1175/2009JAMC1810.1, 2009a. a, b, c, d
Durand, Y., Giraud, G., Laternser, M., Etchevers, P., Mérindol, L., and Lesaffre, B.: Reanalysis of 44 Yr of Climate in the French Alps (1958–2002): Methodology, Model Validation, Climatology, and Trends for Air Temperature and Precipitation, J. Appl. Meteorol. Clim., 48, 429–449, https://doi.org/10.1175/2008JAMC1808.1, 2009b. a, b, c, d, e, f
Essery, R., Morin, S., Lejeune, Y., and Menard, C. B.: A comparison of 1701 snow models using observations from an alpine site, Adv. Water Resour., 55, 131–148, https://doi.org/10.1016/j.advwatres.2012.07.013, 2013. a, b
Fatichi, S., Rimkus, S., Burlando, P., and Bordoy, R.: Does internal climate variability overwhelm climate change signals in streamflow? The upper Po and Rhone basin case studies, Sci. Total Environ., 493, 1171–1182, https://doi.org/10.1016/j.scitotenv.2013.12.014, 2014. a
Francois, B., Hingray, B., Creutin, J., and Hendrickx, F.: Estimating water system performance under climate change: influence of the management strategy modeling, Water Resour. Manag., 29, 4903–4918, https://doi.org/10.1007/s11269-015-1097-5, 2015. a
François, H., Morin, S., Lafaysse, M., and George-Marcelpoil, E.: Crossing numerical simulations of snow conditions with a spatially-resolved socio-economic database of ski resorts: A proof of concept in the French Alps, Cold Reg. Sci. Technol., 108, 98–112, https://doi.org/10.1016/j.coldregions.2014.08.005, 2014. a
Frei, P., Kotlarski, S., Liniger, M. A., and Schär, C.: Future snowfall in the Alps: projections based on the EURO-CORDEX regional climate models, The Cryosphere, 12, 1–24, https://doi.org/10.5194/tc-12-1-2018, 2018. a, b
Gilaberte-Burdalo, M., Lopez-Martin, F. M. R., Pino-Otin, M., and Lopez-Moreno, J.: Impacts of climate change on ski industry, Environ. Sci. Policy, 44, 51–61, https://doi.org/10.1016/j.envsci.2014.07.003, 2014. a
Gobiet, A., Kotlarski, S., Beniston, M., Heinrich, G., Rajczak, J., and Stoffel, M.: 21st century climate change in the European Alps, Sci. Total Environ., 493, 1138–1151, https://doi.org/10.1016/j.scitotenv.2013.07.050, 2014. a, b, c
Gobiet, A., Suklitsch, M., and Heinrich, G.: The effect of empirical-statistical correction of intensity-dependent model errors on the temperature climate change signal, Hydrol. Earth Syst. Sci., 19, 4055–4066, https://doi.org/10.5194/hess-19-4055-2015, 2015. a, b
Hanzer, F., Marke, T., and Strasser, U.: Distributed, explicit modeling of technical snow production for a ski area in the Schladming region (Austrian Alps), Cold Reg. Sci. Technol., 108, 113–124, https://doi.org/10.1016/j.coldregions.2014.08.003, 2014. a
Hanzer, F., Förster, K., Nemec, J., and Strasser, U.: Projected cryospheric and hydrological impacts of 21st century climate change in the Ötztal Alps (Austria) simulated using a physically based approach, Hydrol. Earth Syst. Sci., 22, 1593–1614, https://doi.org/10.5194/hess-22-1593-2018, 2018. a
IPCC: Climate Change 2013: The Physical Science Basis. Contribution of Working Group I to the Fifth Assessment Report of the Intergovernmental Panel on Climate Change, edited by: Stocker, T. F., Qin, D., Plattner, G.-K., Tignor, M., Allen, S. K., Boschung, J., Nauels, A., Xia, Y., Bex, V., and Midgley, P. M., Cambridge University Press, Cambridge, United Kingdom and New York, NY, USA, 1535 pp., 2013. a, b, c
IPCC: Climate Change 2014: Impacts, Adaptation, and Vulnerability. Part A: Global and Sectoral Aspects. Contribution of Working Group II to the Fifth Assessment Report of the Intergovernmental Panel on Climate Change, edited by: Field, C. B., Barros, V. R., Dokken, D. J., Mach, K. J., Mastrandrea, M. D., Bilir, T. E., Chatterjee, M., Ebi, K. L., Estrada, Y. O., Genova, R. C., Girma, B., Kissel, E. S., Levy, A. N., MacCracken, S., Mastrandrea, P. R., and White, L. L., Cambridge University Press, Cambridge, United Kingdom and New York, NY, USA, 1132 pp., 2014a. a
IPCC: Climate Change 2014: Impacts, Adaptation, and Vulnerability. Part B: Regional Aspects. Contribution of Working Group II to the Fifth Assessment Report of the Intergovernmental Panel on Climate Change, edited by: Barros, V. R., Field, C. B., Dokken, D. J., Mastrandrea, M. D., Mach, K. J., Bilir, T. E., Chatterjee, M., Ebi, K. L., Estrada, Y. O., Genova, R. C., Girma, B., Kissel, E. S., Levy, A. N., MacCracken, S., Mastrandrea, P. R., and White, L. L., Cambridge University Press, Cambridge, United Kingdom and New York, NY, USA, 688 pp., 2014b. a
IPCC: Climate Change 2014: Mitigation of Climate Change. Contribution of Working Group III to the Fifth Assessment Report of the Intergovernmental Panel on Climate Change, edited by: [Edenhofer, O., Pichs-Madruga, R., Sokona, Y., Farahani, E., Kadner, S., Seyboth, K., Adler, A., Baum, I., Brunner, S. Eickemeier, P., Kriemann, B., Savolainen, J., Schlömer, S., von Stechow, C., Zwickel, T., and Minx, J. C., Cambridge University Press, Cambridge, United Kingdom and New York, NY, USA, 2014c. a
Jacob, D., Petersen, J., Eggert, B., Alias, A., Christensen, O. B., Bouwer, L. M., Braun, A., Colette, A., Deque, M., Georgievski, G., Georgopoulou, E., Gobiets, A., Menut, L., Nikulin, G., Haensler, A., Hempelmann, N., Jones, C., Keuler, K., Kovats, S., Kroner, N., Kotlarski, S., Kriegsmann, A., Martin, E., Meijgaard, E. V., Moseley, C., Pfeifer, S., Preuschmann, S., Radermacher, C., Radtke, K., Rechid, D., Rounsevell, M., Samuelsson, P., Somot, S., Soussana, J.-F., Teichmann, C., Valentini, R., Vautard, R., Weber, B., and Yiou, P.: EURO-CORDEX: new high-resolution climate change projections for European impact research, Reg. Environ. Change, 14, 563–578, https://doi.org/10.1007/s10113-013-0499-2, 2014. a, b
James, R., Washington, R., Schleussner, C.-F., Rogelj, J., and Conway, D.: Characterizing half-a-degree difference: a review of methods for identifying regional climate responses to global warming targets, Wires Clim. Change, 8, e457, https://doi.org/10.1002/wcc.457, 2017. a, b, c
Jomelli, V., Pavlova, I., Eckert, N., Grancher, D., and Brunstein, D.: A new hierarchical Bayesian approach to analyse environmental and climatic influences on debris flow occurrence, Geomorphology, 250, 407–421, https://doi.org/10.1016/j.geomorph.2015.05.022, 2015. a
Knutti, R., Abramowitz, G., Collins, M., Eyring, V., Gleckler, P., Hewitson, B., and Mearns, L.: Good practice guidance paper on assessing and combining multi model climate projections, in: IPCC Expert meeting on assessing and combining multi model climate projections, edited by: Stocker, T., Dahe, Q., Plattner, G.-K., Tignor, M., and Midgley, P., p. 15, 2010. a, b
Kotlarski, S., Keuler, K., Christensen, O. B., Colette, A., Déqué, M., Gobiet, A., Goergen, K., Jacob, D., Lüthi, D., van Meijgaard, E., Nikulin, G., Schär, C., Teichmann, C., Vautard, R., Warrach-Sagi, K., and Wulfmeyer, V.: Regional climate modeling on European scales: a joint standard evaluation of the EURO-CORDEX RCM ensemble, Geosci. Model Dev., 7, 1297–1333, https://doi.org/10.5194/gmd-7-1297-2014, 2014. a, b, c
Lafaysse, M., Morin, S., Coleou, C., Vernay, M., Serca, D., Besson, F., Willemet, J.-M., Giraud, G., and Durand, Y.: Towards a new chain of models for avalanche hazard forecasting in French mountain ranges, including low altitude mountains, in: Proceedings of International Snow Science Workshop Grenoble–Chamonix Mont-Blanc, CEN, 162–166, 2013. a
Lafaysse, M., Hingray, B., Mezghani, A., Gailhard, J., and Terray, L.: Internal variability and model uncertainty components in future hydrometeorological projections: The Alpine Durance basin, Water Resour. Res., 50, 3317–3341, https://doi.org/10.1002/2013WR014897, 2014. a, b, c, d, e
Lafaysse, M., Cluzet, B., Dumont, M., Lejeune, Y., Vionnet, V., and Morin, S.: A multiphysical ensemble system of numerical snow modelling, The Cryosphere, 11, 1173–1198, https://doi.org/10.5194/tc-11-1173-2017, 2017. a, b, c, d, e, f, g
Maris, M. N., Giraud, G., Durand, Y., Navarre, J.-P., and Mérindol, L.: Results of 50 years of climate reanalysis in the French Pyrenees (1958–2008) using the SAFRAN and CROCUS models, in: Proceedings of the International Snow Science Workshop, Davos 2009, 219–223, 2009. a, b
Martin, E., Brun, E., and Durand, Y.: Sensitivity of the French Alps snow cover to the variation of climatic variables, Ann. Geophys., 12, 469–477, https://doi.org/10.1007/s00585-994-0469-6, 1994. a, b
Martin, E., Giraud, G., Lejeune, Y., and Boudart, G.: Impact of a climate change on avalanche hazard, Ann. Glaciol., 32, 163–167, 2001. a
Marty, C., Schögl, S., Bavay, M., and Lehning, M.: How much can we save? Impact of different emission scenarios on future snow cover in the Alps, The Cryosphere, 11, 517–529, https://doi.org/10.5194/tc-11-517-2017, 2017a. a, b, c, d, e, f, g, h, i, j
Marty, C., Tilg, A.-M., and Jonas, T.: Recent Evidence of Large-Scale Receding Snow Water Equivalents in the European Alps, J. Hydrometeorol., 18, 1021–1031, https://doi.org/10.1175/JHM-D-16-0188.1, 2017b. a
Millar, R., Fuglestvedt, J., Friedlingstein, P., Rogelj, J., Grubb, M., Matthews, H. D., Skeie, R. B., Forster, P. M., Frame, D. J., and Allen, M. R.: Emission budgets and pathways consistent with limiting warming to 1.5∘C, Nat. Geosci., 10, 741–747, https://doi.org/10.1038/ngeo3031, 2017. a, b
Morin, S., Lejeune, Y., Lesaffre, B., Panel, J.-M., Poncet, D., David, P., and Sudul, M.: An 18-yr long (1993–2011) snow and meteorological dataset from a mid-altitude mountain site (Col de Porte, France, 1325 m alt.) for driving and evaluating snowpack models, Earth Syst. Sci. Data, 4, 13–21, https://doi.org/10.5194/essd-4-13-2012, 2012. a, b
Moss, R. H., Edmonds, J. A., Hibbard, K. A., Manning, M. R., Rose, S. K., Van Vuuren, D. P., Carter, T. R., Emori, S., Kainuma, M., Kram, T., Meehl, G. A., Mitchell, J. F. B., Nakicenovic, N., Riahi, K., Smith, S. J., Stouffer, R. J., Thomson, A. M., Weyant, J. P., and Wilbanks, T. J.: The next generation of scenarios for climate change research and assessment, Nature, 463, 747–756, https://doi.org/10.1038/nature08823, 2010. a, b
Mote, P., Hamlet, A., Clark, M., and Lettenmaier, D.: Declining mountain snowpack in western North America, B. Amer. Meteorol. Soc., 86, 39–49, https://doi.org/10.1175/BAMS-86-1-39, 2005. a
Mote, P. W., Li, S., Lettenmaier, D. P., Xiao, M., and Engel, R.: Dramatic declines in snowpack in the western US, Climate and Atmospheric Science, 1, https://doi.org/10.1038/s41612-018-0012-1, 2018. a
Olsson, T., Jakkila, J., Veijalainen, N., Backman, L., Kaurola, J., and Vehviläinen, B.: Impacts of climate change on temperature, precipitation and hydrology in Finland – studies using bias corrected Regional Climate Model data, Hydrol. Earth Syst. Sci., 19, 3217–3238, https://doi.org/10.5194/hess-19-3217-2015, 2015. a
Pepin, N., Bradley, R., Diaz, H., Baraer, M., Caceres, E., Forsythe, N., Fowler, H., Greenwood, G., Hashmi, M., Liu, X., Miller, J., Ning, L., Ohmura, A., Palazzi, E., Rangwala, I., Schöner, W., Severskiy, I., Shahgedanova, M., Wang, M., Williamson, S., and Yang, D.: Elevation-dependent warming in mountain regions of the world, Nat. Clim. Change, 5, 424–430, https://doi.org/10.1038/nclimate2563, 2015. a, b
Piazza, M., Boe, J., Terray, L., Page, C., Sanchez-Gomez, E., and Deque, M.: Projected 21st century snowfall changes over the French Alps and related uncertainties, Climatic Change, 122, 583–594, https://doi.org/10.1007/s10584-013-1017-8, 2014. a, b, c
Pierce, D. W. and Cayan, D. R.: The Uneven Response of Different Snow Measures to Human-Induced Climate Warming, J. Climate, 26, 4148–4167, https://doi.org/10.1175/JCLI-D-12-00534.1, 2013. a, b, c
Quintana-Seguí, P., Turco, M., Herrera, S., and Miguez-Macho, G.: Validation of a new SAFRAN-based gridded precipitation product for Spain and comparisons to Spain02 and ERA-Interim, Hydrol. Earth Syst. Sci., 21, 2187–2201, https://doi.org/10.5194/hess-21-2187-2017, 2017. a, b
Raleigh, M. S., Lundquist, J. D., and Clark, M. P.: Exploring the impact of forcing error characteristics on physically based snow simulations within a global sensitivity analysis framework, Hydrol. Earth Syst. Sci., 19, 3153–3179, https://doi.org/10.5194/hess-19-3153-2015, 2015. a
Rauscher, S. A., Coppola, E., Piani, C., and Giorgi, F.: Resolution effects on regional climate model simulations of seasonal precipitation over Europe, Clim. Dynam., 35, 685–711, https://doi.org/10.1007/s00382-009-0607-7, 2010. a
Reid, P., Hari, R., Beaugrand, G., Livingstone, D., Marty, C., Straile, D., Barichivich, J., Goberville, E., Adrian, R., Aono, Y., Brown, R., Foster, J., Groisman, P., Hélaouet, P., Hsu, H.-H., Kirby, R., Knight, J., Kraberg, A., Li, J., Lo, T.-T., Myneni, R., North, R., Pounds, J., Sparks, T., Stubi, R., Tian, Y., Wiltshire, K., Xiao, D., and Zhu, Z.: Global impacts of the 1980s regime shift, Glob. Change Biol., 22, 682–703, https://doi.org/10.1111/gcb.13106, 2015. a, b
Rogelj, J., Luderer, G., Pietzcker, R., Kriegler, E., Schaeffer, M., Krey, V., and Riahi, K.: Energy system transformations for limiting end-of-century warming to below 1.5 ∘C, Nat. Clim. Change, 5, 519–527, https://doi.org/10.1038/nclimate2572, 2015. a, b
Rousselot, M., Durand, Y., Giraud, G., Mérindol, L., Dombrowski-Etchevers, I., Déqué, M., and Castebrunet, H.: Statistical adaptation of ALADIN RCM outputs over the French Alps – application to future climate and snow cover, The Cryosphere, 6, 785–805, https://doi.org/10.5194/tc-6-785-2012, 2012. a, b, c, d, e
Schmucki, E., Marty, C., Fierz, C., and Lehning, M.: Simulations of 21st century snow response to climate change in Switzerland from a set of RCMs, Int. J. Climatol., 35, 3185–3384, https://doi.org/10.1002/joc.4205, 2014. a, b, c, d, e, f
Spandre, P., François, H., George-Marcelpoil, E., and Morin, S.: Panel based assessment of snow management operations in French ski resorts, Journal of Outdoor Recreation and Tourism, 24–36, https://doi.org/10.1016/j.jort.2016.09.002, 2016a. a, b
Spandre, P., Morin, S., Lafaysse, M., George-Marcelpoil, E., François, H., and Lejeune, Y.: Integration of snow management in a detailed snowpack model, Cold Reg. Sci. Technol., 125, 48–64, https://doi.org/10.1016/j.coldregions.2016.01.002, 2016b. a, b
Spandre, P., François, H., Morin, S., George, E., and Lafaysse, M.: Investigations on socio economic indicators of French Alps ski industry from an explicit spatial modelling of managed snow on ski slopes, Journal of Tourism Management, in revision, 2018. a
Steger, C., Kotlarski, S., Jonas, T., and Schär, C.: Alpine snow cover in a changing climate: a regional climate model perspective, Clim. Dynam., 41, 735–754, https://doi.org/10.1007/s00382-012-1545-3, 2013. a, b, c, d, e, f, g
Steiger, R., Scott, D., Abegg, B., Pons, M., and Aall, C.: A critical review of climate change risk for ski tourism, Curr. Issues Tour., 37 pp., https://doi.org/10.1080/13683500.2017.1410110, 2017. a, b
Strasser, U., Vilsmaier, U., Prettenhaler, F., Marke, T., Steiger, R., Damm, A., Hanzer, F., Wilcke, R., and Stotter, J.: Coupled component modelling for inter-and transdisciplinary climate change impact research: Dimensions of integration and examples of interface design, Environ. Modell. Softw., 60, 180–187, https://doi.org/10.1016/j.envsoft.2014.06.014, 2014. a
Taylor, K. E., Stouffer, R. J., and Meehl, G. A.: An overview of CMIP5 and the experiment design, B. Am. Meteorol. Soc., 93, 485–498, https://doi.org/10.1175/BAMS-D-11-00094.1, 2012. a, b, c, d
Terzago, S., von Hardenberg, J., Palazzi, E., and Provenzale, A.: Snow water equivalent in the Alps as seen by gridded data sets, CMIP5 and CORDEX climate models, The Cryosphere, 11, 1625–1645, https://doi.org/10.5194/tc-11-1625-2017, 2017. a
Themeßl, M. J., Gobiet, A., and Leuprecht, A.: Empirical-statistical downscaling and error correction of daily precipitation from regional climate models, Int. J. Climatol., 31, 1530–1544, https://doi.org/10.1002/joc.2168, 2011. a
Thuiller, W., Gueguen, M., Georges, D., Bonet, R., Chalmandrier, L., Garraud, L., Renaud, J., Roquet, C., Van Es, J., Zimmermann, N., and Lavergne, S.: Are different facets of plant diversity well protected against climate and land cover changes? A test study in the French Alps, Ecography, 37, 1254–1266, https://doi.org/10.1111/ecog.00670, 2014. a
Vasseur, D., DeLong, J., Gilbert, B., Greig, H., Harley, C., McCann, K., Savage, V., Tunney, T., and O'Connor, M.: Increased temperature variation poses a greater risk to species than climate warming, P. R. Soc. Lond. B Bio., 281, 20132612, https://doi.org/10.1098/rspb.2013.2612, 2014. a
Verfaillie, D., Déqué, M., Morin, S., and Lafaysse, M.: The method ADAMONT v1.0 for statistical adjustment of climate projections applicable to energy balance land surface models, Geosci. Model Dev., 10, 4257–4283, https://doi.org/10.5194/gmd-10-4257-2017, 2017. a, b, c, d, e, f, g
Vidal, J.-P., Martin, E., Franchistéguy, L., Baillon, M., and Soubeyroux, J.-M.: A 50 year high resolution atmospheric reanalysis over France with the Safran system, Int. J. Climatol., 30, 1627–1644, https://doi.org/10.1002/joc.2003, 2010. a
Vionnet, V., Brun, E., Morin, S., Boone, A., Faroux, S., Le Moigne, P., Martin, E., and Willemet, J.-M.: The detailed snowpack scheme Crocus and its implementation in SURFEX v7.2, Geosci. Model Dev., 5, 773–791, https://doi.org/10.5194/gmd-5-773-2012, 2012. a, b