the Creative Commons Attribution 4.0 License.
the Creative Commons Attribution 4.0 License.
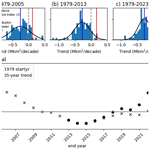
Brief communication: New perspectives on the skill of modelled sea ice trends in light of recent Antarctic sea ice loss
Thomas J. Bracegirdle
Paul R. Holland
Julienne Stroeve
Jeremy Wilkinson
Most climate models do not reproduce the 1979–2014 increase in Antarctic sea ice cover. This was a contributing factor in successive Intergovernmental Panel on Climate Change reports allocating low confidence to model projections of sea ice over the 21st century. We show that recent rapid declines bring observed sea ice area trends back into line with the models and confirm that discrepancies exist for earlier periods. This demonstrates that models exhibit different skill for different timescales and periods. We discuss possible interpretations of this linear trend assessment given the abrupt nature of recent changes and discuss the implications for future research.
- Article
(2139 KB) - Full-text XML
- BibTeX
- EndNote
The early years of the 21st century revealed a puzzling conundrum in Antarctic sea ice (Turner and Comiso, 2017; National Academies of Sciences, Engineering, and Medicine, 2017). Observations of Antarctic sea ice extent (SIE) showed a small increase during the satellite era (which began in late 1978), with annual mean values reaching a maximum in 2014, but most climate models simulated SIE declines over the same period. Various studies examined possible reasons for this discrepancy (Turner and Comiso, 2017). Specifically, the community discussed whether it could be explained by internal variability masking the anthropogenic forced signal in observations (Gagné et al., 2015; Rosenblum and Eisenman, 2017; Roach et al., 2020) and the extent to which it revealed model deficiencies in sea ice processes (Fox-Kemper, 2021). Some studies found that the observed pan-Antarctic trends lay within the distribution of modelled trends (Polvani and Smith, 2013; Zunz et al., 2013) and that only regional trends could robustly be deemed inaccurate in the models (Hobbs et al., 2015). However, these studies considered 1979–2005 trends only, and over this 27-year period the relative role of internal variability in the models is larger than it is for longer periods with more recent end dates. Others suggested that trends in sea ice, particularly SIE, may not be a robust metric of model performance, particularly when the observational time series is too short to separate internal variability from anthropogenic forcing (Notz, 2014). Even so, the poorly understood discrepancy between models and observations has been a contributing factor in a widespread lack of confidence in projections of 21st-century Antarctic sea ice decline and, consequently, in many aspects of projected climate change around Antarctica which are underpinned by projections of substantial sea ice decline (Bracegirdle et al., 2015, 2018).
Recently, Antarctic sea ice has exhibited a starkly different pattern of behaviour. Following the pre-2015 era of slightly increasing ice extent, rapid ice loss beginning in early 2015 culminated in a dramatic drop in spring 2016–2017 (Turner et al., 2017). This led to several years of record-low SIE, which has been framed as a “new sea ice state” (Purich and Doddridge, 2023; Hobbs et al., 2024). This situation shows no sign of abating, with further declines since 2021 leading to monthly mean SIE records being broken in 8 months of 2023 (Fetterer et al., 2017; Siegert et al., 2023). The initial decline showed strong linkages to patterns of intrinsic atmospheric variability (Turner et al., 2017; Schlosser et al., 2018; Zhang et al., 2022) which have high internal variability on short (subannual) timescales. However, growing evidence of the contribution of warming in the subsurface ocean (Zhang et al., 2022; Purich and Doddridge, 2023) and the magnitude and spatial homogeneity of the sea ice reductions since 2016/17 point to more sustained declines.
We are therefore interested in the fundamental question as to whether this new data showing rapid decline should change our judgement of the models' skill. To do so, in the context of previous assessments and based on the approximate linearity of the modelled time series, we assess linear trends. Specifically, we reconsider whether the distribution of linear trends simulated by the current generation of climate models, from the Coupled Model Intercomparison Project Phase 6 (CMIP6; Eyring et al., 2016) dataset, allows for a trend of the observed magnitude and thus whether observed trends are consistent with the multi-model ensemble. Key previous studies have considered trends to 2005 (Hobbs et al., 2015; Polvani and Smith, 2013; Zunz et al., 2013) or 2013 (Rosenblum and Eisenman, 2017) based on CMIP5 models and to 2018 based on CMIP6 (Roach et al., 2020). We might expect the situation to have changed, for two reasons. First, being able to assess trends in longer time series (due to the longer observational record) potentially reduces the impact of short-term internal variability on trend calculations (Notz, 2014). Second, and more specifically, these data now include the recent years of observed rapid decline of sea ice, decreasing long-term trends. Therefore, we perform an analysis of all trends with end dates between 2005 and 2023, to place our results in the context of previous studies and show how the results change over time due to these two factors while using a consistent set of CMIP6 model data (such that the changes are not attributable to changes in model components or resolution). Our discussion of these results focusses on the changing assessment of skill depending on the timescale and period considered, the implications for our confidence in the models, and the interpretation of linear trend assessments considering the abrupt nature of recent changes.
2.1 Sea ice metric
Sea ice cover is calculated as either sea ice extent, SIE (the total area of all grid boxes where sea ice concentration SIC exceeds a 15 % threshold), or sea ice area, SIA (the sum of grid box areas multiplied by grid box SIC). SIA has larger observational uncertainties, as it is more sensitive to differences in SIC. However, SIE is a nonlinear measure and so can give misleading results when comparing models and observations or when calculating trends (Notz, 2014). Therefore, in contrast to some previous assessments, but following community precedent (Roach et al., 2020), we assess SIA. SIA and SIE have similar trends (Fig. A1).
2.2 Model data
We use data from 39 CMIP6 models, from multiple modelling centres. Across the ensemble, there are multiple different model components and resolutions of each component. Monthly SIA is obtained from the University of Hamburg (UHH) CMIP6 Sea Ice Area Directory V02 (Notz and Kern, 2023) and aggregated into weighted annual means. This is supplemented by SIA for the two NorESM models, which are not available in the UHH dataset due to a bug in an earlier version of NorESM-released SIA data. We merge historical simulations ending in December 2014 with the ssp585 forcing scenario run for 2015 to 2023; ssp585 indicates a global average radiative forcing of 8.5 W m−2 by 2100 (O'Neill et al., 2016). This is a high-emissions forcing scenario; however, emissions scenarios have little bearing on results for the time period considered here. The resulting historical–ssp585 merger constitutes 188 ensemble members from 39 models (Table A1), each contributing between 1 and 57 members of an initial condition ensemble.
By using a large number of ensemble members of the historical multi-model ensemble, we sample internal variability under historical anthropogenic forcing. However, since only four models contain more than six members, we use a maximum of six members from each model to avoid weighting the results too heavily towards models with large ensembles. Thus, the final ensemble analysed has 98 members (Fig. B1) from 39 models (Table B1). The sensitivity of our results to this treatment of model ensembles and to the emission scenario is discussed in Appendix C.
Since many models have drifts in their pre-industrial runs, we calculated linear trends over the full pre-industrial period available (in the range 150 to 500 years across the 32 models with data available in the UHH dataset; Table B1), henceforward referred to as “drift”. In all cases, drifts are an order of magnitude smaller than the trends for years 1979–2023, and there is no significant inter-model relationship between the drift in a model's pre-industrial simulation and the ensemble mean of linear trends in that model (p=0.48). This implies drifts are negligible in the context of historical trends, consistent with results for CMIP5 (Gupta et al., 2013), so they are not considered further.
2.3 Observational data
For an estimate of observed sea ice cover, NSIDC Sea Ice Index v3.0 SIA (Fetterer et al., 2017) is used, available from January 1979–September 2023. We investigate the role of observational uncertainty by also using other observational estimates for 1979–2019 from the UHH SIA dataset (Dörr et al., 2021). Data for missing months (December 1987–January 1988 for the Sea Ice Index v3.0) are infilled by interpolating between the same month in the previous and following year (Rosenblum and Eisenman, 2017).
2.4 Trend evaluation methodology
Our evaluation methodology is an extension of that previously used for CMIP5 (Rosenblum and Eisenman, 2017). Linear trends are calculated for all periods of at least 35 years overlapping with the satellite record (January 1979–September 2023) using the OLS (ordinary least squares) method of the Python package statsmodels.api. For comparison with the earlier studies mentioned in the Introduction, we additionally calculate trends for periods 1979–y2, where y2 is between 2005 and 2012. We calculate the mean and standard deviation of the trends from the model ensemble and use these to fit a Gaussian distribution, with cumulative distribution function F(X), to the distribution of these modelled trends. To estimate the probability that a trend at least as large as observed would occur in the climate model population, we calculate the p value for a one-tailed test as 1−F(x), where x is the observed trend. The extent to which a linear trend is an appropriate metric for evaluating SIA, given the evidence for a recent regime change, is considered in the Discussion below.
3.1 Trend evaluation
The recent decade of data has reduced the significant positive trend (Parkinson, 2019) in observed annual mean and monthly SIA, which peaked in the period ending 2015, to near-zero (Fig. 1a–c, red lines; Figs. C1a, A1). For some months and in the annual mean, the trend since 1979 is now weakly negative, and trends are statistically insignificant in all months (Fig. A1). Meanwhile, adding the extra years of data hardly changed the multi-model mean trend at all (Fig. 1a–c, blue lines). The mean trend remains strongly negative, although a few simulations have weakly positive trends. The simulated trends are less influenced by internal climate variability as more years are added, and therefore the standard deviation of the modelled trends for a fixed start year of 1979 decreases over time (Fig. C1c).
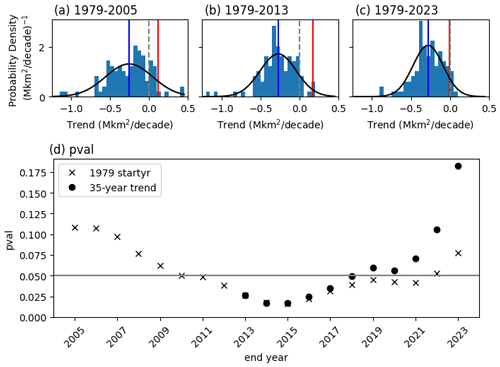
Figure 1(a–c) Linear trends in annual mean SIA in satellite observations (red) and CMIP6 models (blue histogram) and Gaussian fit to CMIP6 distribution (black) for the periods (a) 1979–2005, (b) 1979–2013 and (c) 1979–2023. The dashed vertical line indicates zero trend, and the blue line indicates the multi-model mean. (d) The probability of observing a trend at least as large as observed (a one-tailed test) under the null hypothesis that observations are taken from the same population as the CMIP6 multi-model ensemble, for varying end dates and either a fixed start date of 1979 as in panels (a) and (b) (crosses) or fixed trend length of 35 years (dots); pval stands for p value.
In light of these findings, we test the null hypothesis that observed sea ice trends are consistent with trends simulated across the CMIP6 multi-model ensemble and consider how additional years of data affect the outcome of this test. We consider trends calculated with both a fixed start date (1979) and fixed duration (35 years) to aid our interpretation. Until 2010 inclusive, the probability of a CMIP6 model trend matching or exceeding the observed trend exceeds 0.05, so we would not reject the null hypothesis that modelled and observed trends are consistent (as concluded in Zunz et al., 2013; Hobbs et al., 2015; Polvani and Smith, 2013). However, in the period 2005 through 2015, the multi-model mean trend and observed trend diverge while the modelled trend distribution narrows (Fig. C1), reducing the likelihood that the observed trend falls within the modelled distribution. As a result, between 2011 and 2018, the probability of a CMIP6 model trend matching or exceeding the observed trend is very low (p<0.05; Fig. 1d), so the null hypothesis is rejected, and the model trends may be deemed inconsistent with observations. This test provides a clear result; the short time period of under 40 years should allow for a generous range of modelled trends due to internal variability, but this range still fails to accommodate the observations.
From 2015, the probability of CMIP6 trends matching or exceeding the observed trend starts to increase, as the ice loss brings observations into line with the models (Fig. 1d). However, if trends are calculated with a fixed 1979 start date, progressively lengthening the trend under consideration decreases the modelled trend standard deviation while hardly affecting the model mean trend (Fig. C1). This makes it less likely that the observed trends will fall within the distribution of modelled trends. Only in 2022 does the recent rapid decline in observations counteract this effect and finally bring observed trends into line with the models (null hypothesis not rejected at p=0.05; Fig. 1d). In contrast, for “fixed duration” trends, the standard deviation of modelled trends remains large, while the observed trend more rapidly declines and becomes negative due to the neglect of early low-SIA years (Gagné et al., 2015; Schroeter et al., 2023) in addition to the inclusion of the recent low-SIA years. Therefore, the null hypothesis is no longer rejected at p=0.05 as early as 2019 under this measure.
3.2 Relationship of trends with mean state
It is known that, seasonally and especially in summer, there is a relationship between sea ice area climatology and future trends, which is to be expected as, for example, very low sea ice constrains trends (Holmes et al., 2022). Therefore, we investigated the relevance of this for our trend assessment. The relationship between both summer and annual mean climatology and the annual mean trends is highly statistically significant but has a very weak slope (Fig. C2a, b). Since there are two models (MIROC6 and MIROC-ES2L; Table B1) which are clear outliers, having far too little sea ice in the annual mean (Fig. C2b; Shu et al., 2020), we test the sensitivity to removing these models. This does not change our conclusion that trends are consistent for an end date of 2023 (Fig. C2c). Therefore, while there is some evidence that the models with trends closest to observations tend to be biased low (Fig. C2a, b), this does not appear to dominate our conclusion that observed and modelled trends are now consistent.
Our results show that, if we consider linear trends in models and observations, then we find that the level of agreement varies over time. Firstly, for early end dates (prior to 2011) there is no evidence of inconsistency between observed and modelled trends, as noted by earlier studies (Hobbs et al., 2015; Zunz et al., 2013; Polvani and Smith, 2013). Secondly, there is a mismatch between observed and modelled trends for the period up to around 2018, as discussed in the Introduction. This suggests that modelled anthropogenic trends are too strong relative to modelled variability during that period. Finally, our study shows the novel result that the persistent low Antarctic SIA of 2022 and 2023 brings observed trends over the full satellite era back into line with the ensemble of modelled trends. Trends on the shorter 35-year timescale also fall within the model ensemble for the five most recent 35-year periods (Fig. 1d), showing that the evaluation depends on the exact period analysed, at least on this shorter timescale.
We approach our interpretation of the changing assessment of skill as follows: conceptually, for any time period there is a distribution of model trends and also a distribution of possible real trends that could have occurred (depending upon the evolution of internal climate variability). The observed trend is a single realisation of the distribution of possible real trends. The observed trends with end dates between 2011 and 2021 were outside the model trend distribution. Now, the latest observed trends fall within the distribution of modelled trends, as do observed trends for periods ending before 2011. In other words, the observed trends over the middle period lie in the region where the modelled and real trend distributions do not overlap, and observed trends in the earlier and most recent periods lie in the region where they do overlap.
The nonoverlapping region could arise from a difference in the spread of the modelled and real trend distributions (due to inaccurate modelled variability) or in their mean (due to a modelled anthropogenic forced trend that is too strong). Therefore, inaccurate variability, particularly on multidecadal timescales, could explain the changing assessment of skill. Indeed, modelled variability exceeds observed variability and varies greatly between models (Zunz et al., 2013; Roach et al., 2020; Diamond et al., 2024), with some models containing large centennial variability (Zhang et al., 2019). Alternatively, it could be that the modelled anthropogenic trends are too strong (Schneider and Deser, 2018) or emerge too early. For example, this is consistent with the hypothesis that models underestimate the timescale or magnitude of the cooling phase of the “two-timescale” response to stratospheric ozone forcing, whereby increasing westerlies cause a cooling (sea ice increase) on “short” timescales and warming (decline) on “long” timescales (Ferreira et al., 2015; Kostov et al., 2017). However, other evidence from models suggests this mechanism is unlikely to be a primary driver of the model–observation mismatch (Seviour et al., 2019).
We can then consider what our results imply for our question as posed in the Introduction, namely whether recent rapid declines observed in satellite data change our judgement of model skill and ultimately our confidence in the models. This paragraph considers the answer to this question based on the linear trend assessment, and the following paragraphs take the broader view of how a linear trend assessment should be interpreted in the light of the possible step-change nature of recent decline. Our results permit the interpretation that modelled forced trends and variability are realistic on 45-year timescales (the full length of the modern satellite record). However, the existing discrepancy for earlier, shorter time periods points to fundamental issues remaining, either on shorter timescales in general or specifically for the earlier time period. If this discrepancy is, as discussed above, linked to multidecadal variability or to ozone forcing, then one interpretation may be that we can have some level of greater confidence in projections of substantial centennial decline (Roach et al., 2020; Holmes et al., 2022) under strong forcing, since model performance on longer (45-year) timescales is of the greatest relevance to centennial projections of climate change. However, our confidence would remain low under weak forcings or in the near term, where multidecadal variability and ozone forcing retain relative importance. If, however, the discrepancy is because the forced greenhouse gas response is too strong, models will produce ice loss that is too strong even on centennial timescales. Confidence in which of these interpretations is most appropriate will require both more years of data and further analysis. Further, processes lacking from models, such as increasing freshwater input from accelerating ice sheet melt (Swart et al., 2023), may provide further complications in the relative evolution of modelled and observed sea ice over the 21st century.
This study uses linear trend analysis as a metric for evaluation. Linear trends are a limited parametric assessment, and the observed time series when the years 2017–2023 are included arguably looks strikingly nonlinear in time (Fig. B1). Indeed, the recent abrupt change has been interpreted by some as a regime shift (Purich and Doddridge, 2023; Hobbs et al., 2024), which points to limitations in applying a linear trend evaluation. Nevertheless, an update to the linear trend evaluation has significant value. Firstly, the use of linear trends in many previous assessments (as cited in the Introduction) merits a careful examination of whether the conclusions of those studies still hold. Secondly, many models have approximately linear evolution in time (Fig. B1), which justifies a comparison of linear trends, although the time evolution of SIA in many models also exhibits nonlinear features so that the apparent observed nonlinearity itself is not a reason to conclude there is a discrepancy between models and observations.
A third justification of the linear trend assessment is that a regime shift is not the only interpretation of the observations, and multidecadal variability superimposed on a forced linear trend (e.g. Zhang et al., 2019) could cause the abrupt change seen since 2016. This interpretation is consistent with evidence of steady sea ice decline in the 20th century before the satellite era (Fogt et al., 2022) and with early satellite data, which suggest that the ice area was more variable in the 1960s (Meier et al., 2013; Gallaher et al., 2013) and dropped rapidly immediately before the onset of continuous coverage in 1979 (Cavalieri et al., 2003). Under this “multidecadal variability” interpretation, evaluating linear trends on increasingly long timescales would capture more of the underlying forced trend. In this context, it is a key novel result that our results show that models no longer fail the fundamental test of being able to simulate observed linear trends over the full 45-year modern satellite era. However, capturing the underlying forced trends would require a period much longer than the timescale of multidecadal variability.
Nevertheless, we must interpret the results of the linear evaluation in the light of the recently observed abrupt decline, whereby the linear model looks increasingly less valid for observations. This again implies the emerging agreement on linear trends should not necessarily imply more confidence in model projections. From this perspective, the rapid decline provides a new context for comparing observations and models (Diamond et al., 2024) and adds evidence for which characteristics of sea ice variability the models are unable to simulate and should therefore be a focus of future studies. Therefore, while it is a tenable view that the observed rapid decline could be the first indication that the declines projected in the models could occur, there is now a need to probe the nature of this recent change, specifically the contribution of multiple timescales, and its representation in models. This will be challenging, since extremes and multidecadal variability are difficult to assess due to limited observational data. Moreover, the recent declines are still short-lived, so further years of data will add clarity to the nature of recent change. More broadly, there are many measures by which modelled sea ice may be assessed and found to have deficiencies, including seasonal and interannual variability (Zunz et al., 2013), spatial patterns (Hobbs et al., 2015), physical processes (Holmes et al., 2019), and relationships between trends and other variables (e.g. global warming; Rosenblum and Eisenman, 2017, or mean state, as discussed in Sect. 3.2). Improving knowledge on the strengths and weaknesses of climate models in representing sea ice is important for understanding wider implications for both marine ecosystem function and Southern Hemisphere climate – including Southern Ocean heat and carbon uptake, circumpolar winds (Bracegirdle et al., 2018), and melting of the Antarctic Ice Sheet. This understanding in turn underpins decisions about the mitigation of future greenhouse gas emissions and about ecosystem management.
Our new evidence shows that the level of agreement between modelled and observed linear trends in Antarctic sea ice varies over time. Specifically, we show two new results. Firstly, trends over a fixed 35-year timescale have changing agreement depending on the time period analysed. Secondly, trends over the full satellite era 1979–2023 do not disagree between observations and models. This could imply that models are better able to represent changes over longer timescales. Alternatively, it could be the case that even on this 45-year timescale, the specific time period analysed (i.e. the inclusion of the low-sea-ice years 2017–2023) determines the level of agreement between observations and models. Increasing confidence in these interpretations will require an increased understanding of the recent decline and of variability on different timescales in models and observations. Moreover, understanding the applicability of our linear trend assessment requires an increased knowledge of the extent to which the observed trend can be interpreted as a linear trend superimposed with interannual to multidecadal variability. Nevertheless, our new evidence shows that the recent years of observed low sea ice have changed the picture of model–observation agreement in linear trends in Antarctic sea ice.
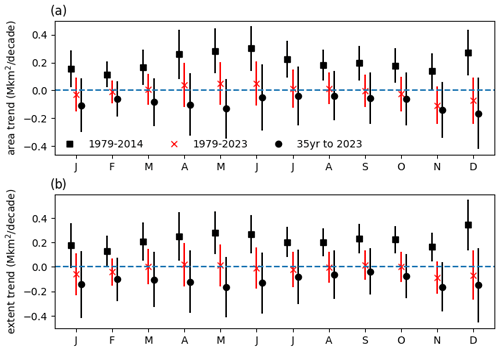
Figure A1Observed sea ice trends for (a) sea ice area and (b) sea ice extent in individual months for 1979–2014 (squares), the full 45-year trend 1979–2023 (crosses) and the 35-year trend to 2023 (circles). The 1979–2023 trends are highlighted in shades of red as this period is the focus of the paper. The 5th–95th percentile uncertainties are indicated by vertical lines. Data are from the NSIDC Sea Ice Index v3.0 (see Methods).
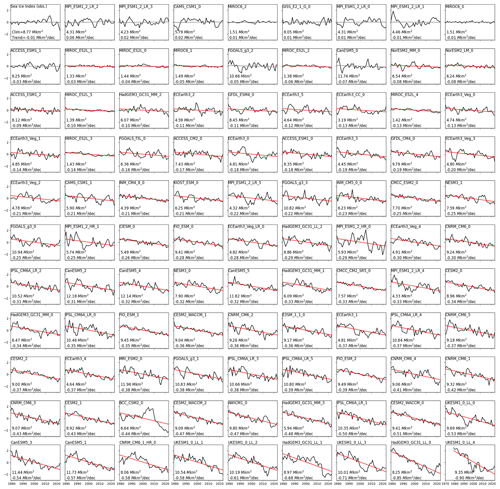
Figure B1The 1979–2023 annual mean sea ice area in observations (NSIDC Sea Ice Index v3.0, top left) and in all CMIP6 model ensemble members considered in the analysis. Panels are sorted by their linear trend over 1979–2023. Linear trends are shown and indicated in red (statistically significant at p<0.05) or grey (statistically insignificant). Each panel includes annotation showing the simulation's 1979–2023 climatology and trend. The y axis shows the SIA anomaly from the 1979–2023 climatology (106 km2).
Table B1The models available for the study and summary values: the number of ensemble members number used (as well as the number available where this differs); the ensemble mean trend (106 km2 per decade) and the climatology (106 km2) across the ensemble members used only for the period specified and the trend in the pre-industrial simulation “pi control” (106 km2 per decade). NorESM values were calculated by the authors from SIC data; all other values were obtained from the CMIP6 Sea Ice Area Directory V02 (Notz and Kern, 2023) made available by the University of Hamburg, and methods are fully detailed there. Model names appear first as formatted in the CMIP6 SIA Directory V02 (Notz and Kern, 2023) and then by their official names.
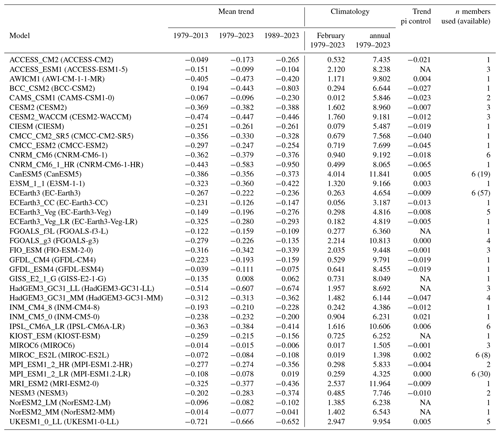
NA: not available
C1 Sensitivity to observational dataset
Observational uncertainty in SIA is particularly high prior to winter 1987 (not shown) due to missing SIC data. Trends in the other datasets, in particular OSI SAF 450/430b (Fig. C1, green; Lavergne et al., 2019), are in general more strongly positive than those in the NSIDC Sea Ice Index v3.0 (Fig. C1a). Therefore, for the “1979 start date” trends, these might exhibit consistency with model-simulated trends at later end dates than 2022 (Fig. C1d, crosses); note that all datasets already display consistency for the 35-year trends ending in 2019 onwards (Fig. C1d, dots).
C2 Sensitivity to treatment of CMIP6 models
We also tested the sensitivity of our conclusions to our treatment of CMIP6 models. First, we tested the sensitivity to treatment of individual model ensembles. As stated in the main text, the choice of using a maximum of six ensemble members per model was to sample internal variability adequately without weighting towards models with large ensembles. By including all ensemble members (instead of a maximum of six per model), we largely add simulations from models with weak negative average trends (Table B1) and therefore increase consistency with observations (not shown). However, the evolution with end year of the model–observation comparison (Fig. 1d) and the broad timings of threshold crossings are unchanged. On the other hand, since curtailment to a maximum of six members per model still constitutes uneven sampling across models which have different internal variabilities, we also verified that when using one ensemble member per model, results remain on average the same for 2023 end dates (Fig. C2d).
Second, we tested sensitivity to using the weaker forcing scenario ssp245 instead of ssp585 for the extension of modelled trends after 2014. The effect of forcing scenario is small early in the 21st century (Hawkins and Sutton, 2012) so that any difference arising is due to internal variability or structural differences between the models with simulations available. For the overlapping subset of 147 model–realisation combinations, ssp245 has marginally stronger trends and so is slightly less consistent with observations. In contrast, using the full ssp245 ensemble (with all available members) means including a larger ensemble of MIROC6 than in the overlapping subset or in the ssp585 ensemble; MIROC6 implausibly has virtually no sea ice year-round (Shu et al., 2020) and therefore zero trends (Holmes et al., 2022), leading to weaker mean trends and slightly greater consistency with observations. In summary, these effects are small, so our conclusions are robust to these sensitivity tests.
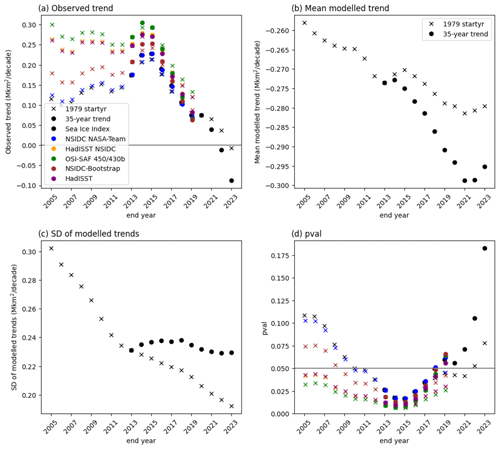
Figure C1Contributions to the p value shown in Fig. 1d. (a) Observed trend, with NSIDC Sea Ice Index v3.0 in black as in the main text and other datasets as indicated. (b) Mean of modelled trends, (c) standard deviation of modelled trends and (d) p value (pval; as main text Fig. 1d but with alternative observational estimates (Dörr et al., 2021)).
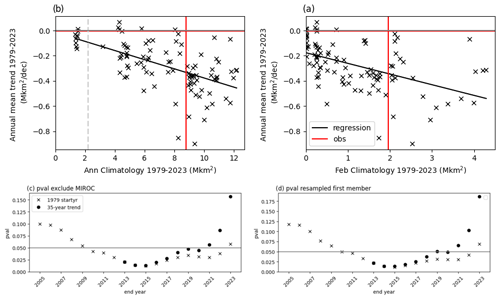
Figure C2The role of ice-free conditions in explaining model spread (a and b) and result sensitivity to ensemble treatment (c and d). (a) Scatterplot of summer (February) sea ice climatology for 1979–2023 against the annual mean trend over 1979–2023. (b) As (a) but for annual mean climatology against the trend, with a cutoff threshold (a quarter of the observed climatology) to exclude MIROC models indicated by a dashed grey line. Panels (a) and (b) show a maximum of six ensemble members per model. (c) As Fig. 1d but excluding MIROC models. (d) As Fig. 1d but the mean of p values (pval) from 10 000 resamples each using one random ensemble member from each model.
The code for calculating trends, performing the evaluation and preparing the figures is available from the corresponding author on request.
Sea ice area from the CMIP6 models is available from the University of Hamburg (UHH) CMIP6 Sea Ice Area Directory V02 (https://www.cen.uni-hamburg.de/en/icdc/data/cryosphere/cmip6-sea-ice-area.html; Notz and Kern, 2023). The NSIDC Sea Ice Index v3.0 SIA (Fetterer et al., 2017) is available from https://doi.org/10.7265/N5K072F8 (Fetterer et al., 2017). Other observational estimates of sea ice area (Dörr et al., 2021) are available from https://doi.org/10.25592/uhhfdm.8559.
CRH, TJB and PRH conceived the study. CRH conducted the analysis and prepared the figures. All authors discussed the results and reviewed the manuscript.
The contact author has declared that none of the authors has any competing interests.
Publisher’s note: Copernicus Publications remains neutral with regard to jurisdictional claims made in the text, published maps, institutional affiliations, or any other geographical representation in this paper. While Copernicus Publications makes every effort to include appropriate place names, the final responsibility lies with the authors.
The World Climate Research Programme's (WCRP) Working Group on Coupled Modelling, which is responsible for CMIP, and the climate modelling groups are thanked for producing and making available their model output.
All authors were supported by the National Environment Research Council (NERC) (grant no. NE/W004747/1). Julienne Stroeve also received funding from the Canada 150 Research Chairs programme (C150, grant no. 50296) and from the European Union's Horizon 2020 research and innovation programme (grant no. 101003826).
This paper was edited by Chris Derksen and reviewed by William Hobbs and two anonymous referees.
Bracegirdle, T. J., Stephenson, D. B., Turner, J., and Phillips, T.: The importance of sea ice area biases in 21st century multimodel projections of Antarctic temperature and precipitation, Geophys. Res. Lett., 42, 10832–10839, https://doi.org/10.1002/2015GL067055, 2015.
Bracegirdle, T. J., Hyder, P., and Holmes, C. R.: CMIP5 diversity in southern westerly jet projections related to historical sea ice area: Strong link to strengthening and weak link to shift, J. Climate, 31, 195–211, https://doi.org/10.1175/JCLI-D-17-0320.1, 2018.
Cavalieri, D., Parkinson, C., and Vinnikov, K. Y.: 30-Year satellite record reveals contrasting Arctic and Antarctic decadal sea ice variability, Geophys. Res. Lett., 30, 1970, https://doi.org/10.1029/2003GL018031, 2003.
Diamond, R., Sime, L. C., Schroeder, D., and Holmes, C. R.: CMIP6 models rarely simulate Antarctic winter sea-ice anomalies as large as observed in 2023, Geophys. Res. Lett., 51, e2024GL109265, https://doi.org/10.1029/2024GL109265, 2024.
Dörr, J., Dirk, N., and Kern, S.: UHH sea-ice area product, 1850–2019 (v2019_fv0.01), UHH [data set], https://doi.org/10.25592/uhhfdm.8559, 2021.
Eyring, V., Bony, S., Meehl, G. A., Senior, C. A., Stevens, B., Stouffer, R. J., and Taylor, K. E.: Overview of the Coupled Model Intercomparison Project Phase 6 (CMIP6) experimental design and organization, Geosci. Model Dev., 9, 1937–1958, https://doi.org/10.5194/gmd-9-1937-2016, 2016.
Ferreira, D., Marshall, J., Bitz, C. M., Solomon, S., and Plumb, A.: Antarctic Ocean and sea ice response to ozone depletion: A two-time-scale problem, J. Climate, 28, 1206–1226, https://doi.org/10.1175/JCLI-D-14-00313.1, 2015.
Fetterer, F., Knowles, K., Meier, W. N., Savoie, M., and Windnagel, A. K.: Sea Ice Index. (G02135, Version 3), Boulder, Colorado USA, National Snow and Ice Data Center [data set], https://doi.org/10.7265/N5K072F8 (last access: 26 January 2024), 2017.
Fogt, R. L., Sleinkofer, A. M., Raphael, M. N., and Handcock, M. S.: A regime shift in seasonal total Antarctic sea ice extent in the twentieth century, Nat. Clim. Change, 12, 54–62, https://doi.org/10.1038/s41558-021-01254-9, 2022.
Fox-Kemper, B., Hewitt, H. T., Xiao, C., Aðalgeirsdóttir, G., Drijfhout, S. S., Edwards, T. L., Golledge, N. R., Hemer, M., Kopp, R. E., Krinner, G., Mix, A., Notz, D., Nowicki, S., Nurhati, I. S., Ruiz, L., Sallée, J.-B., Slangen, A. B. A., and Yu, Y.: Ocean, Cryosphere and Sea Level Change, in: In Climate Change 2021 – The Physical Science Basis: Working Group I Contribution to the Sixth Assessment Report of the Intergovernmental Panel on Climate Change, Cambridge University Press, 1211–1362, https://doi.org/10.1017/9781009157896.011, 2021.
Gagné, M. È., Gillett, N., and Fyfe, J.: Observed and simulated changes in Antarctic sea ice extent over the past 50 years, Geophys. Res. Lett., 42, 90–95, https://doi.org/10.1002/2014GL062231, 2015.
Gallaher, D. W., Campbell, G. G., and Meier, W. N.: Anomalous variability in Antarctic sea ice extents during the 1960s with the use of Nimbus data, IEEE J. Sel. Top. Appl. Earth Obs., 7, 881–887, https://doi.org/10.1109/JSTARS.2013.2264391, 2013.
Gupta, A. S., Jourdain, N. C., Brown, J. N., and Monselesan, D.: Climate Drift in the CMIP5 Models, J. Climate, 26, 8597–8615, https://doi.org/10.1175/JCLI-D-12-00521.1, 2013.
Hawkins, E. and Sutton, R.: Time of emergence of climate signals, Geophys. Res. Lett., 39, L01702, https://doi.org/10.1029/2011GL050087, 2012.
Hobbs, W. R., Bindoff, N. L., and Raphael, M. N.: New Perspectives on Observed and Simulated Antarctic Sea Ice Extent Trends Using Optimal Fingerprinting Techniques, J. Climate, 28, 1543–1560, https://doi.org/10.1175/JCLI-D-14-00367.1, 2015.
Hobbs, W., Spence, P., Meyer, A., Schroeter, S., Fraser, A., Reid, P., Tian, T., Wang, Z., Liniger, G., Doddridge, E. and Boyd, P.: Observational Evidence for a Regime Shift in Summer Antarctic Sea Ice, J. Climate, 37, 2263–2275, https://doi.org/10.1175/JCLI-D-23-0479.1, 2024.
Holmes, C., Bracegirdle, T., and Holland, P.: Antarctic sea ice projections constrained by historical ice cover and future global temperature change, Geophys. Res. Lett., 49, e2021GL097413, https://doi.org/10.1029/2021GL097413, 2022.
Holmes, C. R., Holland, P. R., and Bracegirdle, T. J.: Compensating biases and a noteworthy success in the CMIP5 representation of Antarctic sea ice processes, Geophys. Res. Lett., 46, 4299–4307, https://doi.org/10.1029/2018GL081796, 2019.
Kostov, Y., Marshall, J., Hausmann, U., Armour, K. C., Ferreira, D., and Holland, M. M.: Fast and slow responses of Southern Ocean sea surface temperature to SAM in coupled climate models, Clim. Dynam., 48, 1595–1609, https://doi.org/10.1007/s00382-016-3162-z, 2017.
Lavergne, T., Sørensen, A. M., Kern, S., Tonboe, R., Notz, D., Aaboe, S., Bell, L., Dybkjær, G., Eastwood, S., Gabarro, C., Heygster, G., Killie, M. A., Brandt Kreiner, M., Lavelle, J., Saldo, R., Sandven, S., and Pedersen, L. T.: Version 2 of the EUMETSAT OSI SAF and ESA CCI sea-ice concentration climate data records, The Cryosphere, 13, 49–78, https://doi.org/10.5194/tc-13-49-2019, 2019.
Meier, W. N., Gallaher, D., and Campbell, G. G.: New estimates of Arctic and Antarctic sea ice extent during September 1964 from recovered Nimbus I satellite imagery, The Cryosphere, 7, 699–705, https://doi.org/10.5194/tc-7-699-2013, 2013.
National Academies of Sciences, Engineering, and Medicine: Antarctic Sea Ice Variability in the Southern Ocean-Climate System, Proceedings of a Workshop, Washington, DC, The National Academies Press, https://doi.org/10.17226/24696, 2017.
Notz, D.: Sea-ice extent and its trend provide limited metrics of model performance, The Cryosphere, 8, 229–243, https://doi.org/10.5194/tc-8-229-2014, 2014.
Notz, D. and Kern, S.: CMIP6 Sea Ice Area Directory, version V02, University of Hamburg, https://www.cen.uni-hamburg.de/en/icdc/data/cryosphere/cmip6-sea-ice-area.html (last access: 17 August 2023), 2023.
O'Neill, B. C., Tebaldi, C., van Vuuren, D. P., Eyring, V., Friedlingstein, P., Hurtt, G., Knutti, R., Kriegler, E., Lamarque, J.-F., Lowe, J., Meehl, G. A., Moss, R., Riahi, K., and Sanderson, B. M.: The Scenario Model Intercomparison Project (ScenarioMIP) for CMIP6, Geosci. Model Dev., 9, 3461–3482, https://doi.org/10.5194/gmd-9-3461-2016, 2016.
Parkinson, C. L.: A 40-y record reveals gradual Antarctic sea ice increases followed by decreases at rates far exceeding the rates seen in the Arctic, P. Natl. Acad. Sci. USA, 116, 14414–14423, https://doi.org/10.1073/pnas.1906556116, 2019.
Polvani, L. M. and Smith, K. L.: Can natural variability explain observed Antarctic sea ice trends? New modeling evidence from CMIP5, Geophys. Res. Lett., 40, 3195–3199, https://doi.org/10.1002/grl.50578, 2013.
Purich, A. and Doddridge, E. W.: Record low Antarctic sea ice coverage indicates a new sea ice state, Commun. Earth Environ., 4, 314, https://doi.org/10.1038/s43247-023-00961-9, 2023.
Roach, L. A., Dörr, J., Holmes, C. R., Massonnet, F., Blockley, E. W., Notz, D., Rackow, T., Raphael, M. N., O'Farrell, S. P., Bailey, D. A., and Bitz, C. M.: Antarctic Sea Ice Area in CMIP6, Geophys. Res. Lett., 47, e2019GL086729, https://doi.org/10.1029/2019GL086729, 2020.
Rosenblum, E. and Eisenman, I.: Sea ice trends in climate models only accurate in runs with biased global warming, J. Climate, 30, 6265–6278, https://doi.org/10.1175/JCLI-D-16-0455.1, 2017.
Schlosser, E., Haumann, F. A., and Raphael, M. N.: Atmospheric influences on the anomalous 2016 Antarctic sea ice decay, The Cryosphere, 12, 1103–1119, https://doi.org/10.5194/tc-12-1103-2018, 2018.
Schneider, D. P. and Deser, C.: Tropically driven and externally forced patterns of Antarctic sea ice change: Reconciling observed and modeled trends, Clim. Dynam., 50, 4599–4618, https://doi.org/10.1007/s00382-017-3893-5, 2018.
Schroeter, S., O'Kane, T. J., and Sandery, P. A.: Antarctic sea ice regime shift associated with decreasing zonal symmetry in the Southern Annular Mode, The Cryosphere, 17, 701–717, https://doi.org/10.5194/tc-17-701-2023, 2023.
Seviour, W., Codron, F., Doddridge, E. W., Ferreira, D., Gnanadesikan, A., Kelley, M., Kostov, Y., Marshall, J., Polvani, L., and Thomas, J.: The Southern Ocean sea surface temperature response to ozone depletion: A multimodel comparison, J. Climate, 32, 5107–5121, https://doi.org//10.1175/JCLI-D-19-0109.1, 2019.
Shu, Q., Wang, Q., Song, Z., Qiao, F., Zhao, J., Chu, M., and Li, X.: Assessment of sea ice extent in CMIP6 with comparison to observations and CMIP5, Geophys. Res. Lett., 47, e2020GL087965, https://doi.org/10.1029/2020GL087965, 2020.
Siegert, M. J., Bentley, M. J., Atkinson, A., Bracegirdle, T. J., Convey, P., Davies, B., Downie, R., Hogg, A. E., Holmes, C., and Hughes, K. A.: Antarctic extreme events, Front. Environ. Sci., 11, 1229283, https://doi.org/10.3389/fenvs.2023.1229283, 2023.
Swart, N. C., Martin, T., Beadling, R., Chen, J.-J., Danek, C., England, M. H., Farneti, R., Griffies, S. M., Hattermann, T., Hauck, J., Haumann, F. A., Jüling, A., Li, Q., Marshall, J., Muilwijk, M., Pauling, A. G., Purich, A., Smith, I. J., and Thomas, M.: The Southern Ocean Freshwater Input from Antarctica (SOFIA) Initiative: scientific objectives and experimental design, Geosci. Model Dev., 16, 7289–7309, https://doi.org/10.5194/gmd-16-7289-2023, 2023.
Turner, J. and Comiso, J.: Solve Antarctica's sea-ice puzzle, Nature, 547, 275–277, https://doi.org/10.1038/547275a, 2017.
Turner, J., Phillips, T., Marshall, G. J., Hosking, J. S., Pope, J. O., Bracegirdle, T. J., and Deb, P.: Unprecedented springtime retreat of Antarctic sea ice in 2016, Geophys. Res. Lett., 44, 6868–6875, https://doi.org/10.1002/2017GL073656, 2017.
Zhang, L., Delworth, T. L., Cooke, W., and Yang, X.: Natural variability of Southern Ocean convection as a driver of observed climate trends, Nat. Clim. Change, 9, 59–65, https://doi.org/10.1038/s41558-018-0350-3, 2019.
Zhang, L., Delworth, T. L., Yang, X., Zeng, F., Lu, F., Morioka, Y., and Bushuk, M.: The relative role of the subsurface Southern Ocean in driving negative Antarctic Sea ice extent anomalies in 2016–2021, Commun. Earth Environ., 3, 302, https://doi.org/10.1038/s43247-022-00624-1, 2022.
Zunz, V., Goosse, H., and Massonnet, F.: How does internal variability influence the ability of CMIP5 models to reproduce the recent trend in Southern Ocean sea ice extent?, The Cryosphere, 7, 451–468, https://doi.org/10.5194/tc-7-451-2013, 2013.
- Abstract
- Introduction
- Data and methods
- Results
- Discussion
- Conclusions
- Appendix A: Monthly trends
- Appendix B: CMIP6 models
- Appendix C: Sensitivity tests
- Code availability
- Data availability
- Author contributions
- Competing interests
- Disclaimer
- Acknowledgements
- Financial support
- Review statement
- References
- Abstract
- Introduction
- Data and methods
- Results
- Discussion
- Conclusions
- Appendix A: Monthly trends
- Appendix B: CMIP6 models
- Appendix C: Sensitivity tests
- Code availability
- Data availability
- Author contributions
- Competing interests
- Disclaimer
- Acknowledgements
- Financial support
- Review statement
- References