the Creative Commons Attribution 4.0 License.
the Creative Commons Attribution 4.0 License.
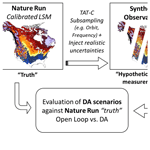
Evaluating the utility of active microwave observations as a snow mission concept using observing system simulation experiments
Carrie M. Vuyovich
Sujay V. Kumar
Melissa L. Wrzesien
Rhae Sung Kim
Satellite-based synthetic aperture radar (SAR) sensors have the potential to provide the first global measure of snow water equivalent (SWE), with key advantages compared to existing satellite observations (e.g., passive microwave sensors) such as high spatial resolution and capability in mountainous areas. While recent studies have shown some capability in challenging conditions, such as deep snow and forested areas, there is still work to be done to understand the limitations and benefits of these observations in an assimilation system. In this study, we develop an observing system simulation experiment (OSSE) to characterize the expected error levels of active microwave-based volume-scattering SWE retrievals over a western Colorado domain. We found that for a hypothetical SAR snow mission, the root mean square error (RMSE) of SWE improves by about 20 % in the mountainous environment if the retrieval algorithm can estimate SWE up to 600 mm and the tree cover fraction up to 40 %. Results also demonstrate that the potential SWE retrievals have larger improvements in the tundra (43 %) snow class, followed by boreal forest (22 %) and montane forest (17 %). Even though active microwave sensors are known to be limited by liquid water in the snowpack, they still reduced errors by up to 6 %–16 % of domain-averaged SWE in the melting period, suggesting that the SWE retrievals can add value to meltwater estimations and hydrological applications. Overall, this work provides a quantitative benchmark of the utility of a potential snow mission concept in a mountainous domain, helping to prioritize future algorithm development and field validation activities.
- Article
(8827 KB) - Full-text XML
-
Supplement
(1675 KB) - BibTeX
- EndNote
Global distribution of seasonal snow is a critical component of the Earth's water and energy cycles (Barnett et al., 2005; Pulliainen et al., 2020; Sturm et al., 2017). Seasonal snow covers up to 50×106 km2 of the Northern Hemisphere in winter, and about 17 % of the world's population relies on meltwater from seasonal snow that replenishes reservoir storage and groundwater for natural and human systems (Bormann et al., 2018; Li et al., 2017; Immerzeel et al., 2019). However, spatially distributed information on snow water equivalent (SWE; the amount of water stored in the snowpack) across the globe is limited, particularly in complex terrain such as mountainous regions, where a large portion of the snowpack is commonly distributed. In general, mountains and remote regions lack in situ SWE networks across the globe (Dozier et al., 2016). Even if there are relatively dense ground measurement networks, the in situ observations have limited spatial representativeness (e.g., automated snow pillow stations in the snow telemetry (SNOTEL) network represent a ∼ 3 m by 3 m area), providing limited information on the spatial distribution of SWE, particularly in heterogeneous terrain (Molotch and Bales, 2005).
Historically, a series of satellite-based passive microwave radiometers have been used to develop spatially distributed snow depth and SWE information (Cho et al., 2017; Derksen et al., 2005; Foster et al., 2005; Vuyovich et al., 2014). However, the passive microwave satellite-based SWE retrievals have a coarse spatial resolution (∼ 25 km) and large SWE uncertainties in various snow and land conditions, which often limit their utility for water supply assessments and operational weather prediction applications (Lettenmaier et al., 2015; Carroll et al., 1999). The passive microwave retrieval algorithms do not perform well under a deep snowpack greater than approximately 200 mm SWE (the so-called “saturation effect”) because the microwave radiation at a higher frequency does not decrease with increasing SWE (Derksen et al., 2010; Dong et al., 2005). Errors in SWE retrievals generally increase with increasing forest density (Cho et al., 2020; Foster et al., 2005; Vander Jagt et al., 2013). Passive microwave radiation is also highly sensitive to small amounts of liquid water content in the snowpack (Kang et al., 2013; Walker and Goodison, 1993), hampering accurate SWE retrievals under wet snow conditions. Through data assimilation efforts such as the GlobSnow project (Pulliainen et al., 2020; Takala et al., 2011), improved SWE estimates have been generated by integrating passive microwave brightness temperature measurements and in situ observations; however, coverage over mountainous regions is still lacking in these products due to large uncertainties over these areas (Larue et al., 2017; Pulliainen et al., 2020). Therefore, global coverage of SWE information is still elusive despite the long legacy of passive microwave instruments.
Active microwave sensors, in particular using synthetic aperture radar (SAR) approaches, have great potential to measure SWE at higher spatial resolution and with improved capabilities in deeper snowpack and forest cover (Lievens et al., 2019; Rott et al., 2010; Tsang et al., 2022). SWE retrieval using X- and/or Ku-band radar is a viable approach as a global satellite mission concept because these measurements are sensitive to SWE through the volume-scattering properties of dry snow. In recent decades, this approach has been explored in the snow remote sensing community. The Cold Regions Hydrology High-Resolution Observatory (CoReH2O) mission concept, a dual-mode high-frequency (X-band (9.6 GHz) and Ku-band (17.2 GHz)) SAR, was proposed to the European Space Agency (ESA) in response to the 2005 Earth Explorer Core Mission call. This mission was selected by ESA for feasibility studies (Phase A) in 2009 but was not selected for further implementation (Rott et al., 2010). In addition, as part of the NASA Snow and Cold Land Processes (SCLP) mission (National Research Council, 2007; Yueh et al., 2009) and the Cold Land Processes Field Experiment (CLPX) activities (Cline et al., 2009; Elder et al., 2009; Tedesco et al., 2005), a high-frequency SAR and high-frequency (K- and Ka-band) passive microwave radiometer were explored. Recently, Environment and Climate Change Canada (ECCC) in partnership with the Canadian Space Agency (CSA) initiated a new Ku-band dual-frequency (13.5 and 17.2 GHz) SAR mission (Terrestrial Snow Mass Mission, TSMM) concept study (Derksen et al., 2019), and a X- and Ku-band concept is being considered for the upcoming NASA Earth System Explorers solicitation.
The series of radar mission development activities in recent decades, which include multi-year field and airborne campaigns, has played a major role in the considerable progress achieved towards the use of radar remote sensing techniques not only to estimate snow microstructure and SWE but also to identify retrieval uncertainties in diverse regions such as deep snow and forests (King et al., 2018; Nagler et al., 2008; Rott et al., 2010; Rutter et al., 2019; Zhu et al., 2018). Early results demonstrated accurate SWE retrieval capability up to approximately 300 mm using X- and Ku-band frequencies (Nagler et al., 2008; Rott et al., 2010), based on limited observations. More recently, studies have shown sensitivity using these frequencies at snow depths over 600 mm (Borah et al., 2022; Santi et al., 2022). As a future direction of the algorithm development at the X- and/or Ku-band, Tsang et al. (2022) mentioned that the co-polarization X-band backscatter signal could be used for estimating deeper SWE (>300 mm) along with a multilayer algorithm (King et al., 2018; Rutter et al., 2019). As a different frequency approach, Lievens et al. (2019) show the capability of C-band cross-polarization backscatters (5.4 GHz) from Sentinel-1 for measuring deep snow depths (e.g., more than 2 m in Fig. 7 of Lievens et al., 2019). For dry snow, the empirical change detection algorithm can retrieve snow depth up to 5 m deep at less than 1 km spatial resolution over mountain ranges (Lievens et al., 2022).
For forest effects, Nagler et al. (2008) found that the presence of dormant herbaceous vegetation has a small influence on the backscattering of the active microwave signals but does not affect sensitivity to SWE. However, the backscatter signal may be affected in coniferous forests based on simulation studies. In the case of low fractional cover (<25 %) in coniferous forests, simulations with a radiative transfer model found that the snow backscatter dominated the radar signal (Macelloni et al., 2001; Magagi et al., 2002). When the forest fraction increases, the sensitivity of the backscatter to SWE generally decreases. Tsang et al. (2022) demonstrated that at Ku-band frequency (17.2 GHz; wavelength: 1.74 cm), the wave can travel through the gaps in trees in straight lines. The Ku-band waves could pass through the gaps like lidar (light wave detection and ranging), which is considered able to penetrate forest canopies. This suggests that the SWE retrievals in areas with a tree cover fraction (TCF) of up to 30 % could be achievable with efforts to account for the three-dimensional structure of the canopy for a more detailed and accurate assessment of the impact of forest type and density on the SWE sensitivity (Rott et al., 2011; Montomoli et al., 2015). Considering that forested regions are a significant portion of the global snow-covered extent (Rutter et al., 2009; Kim et al., 2021), even slight advancements in retrieval algorithms for improved handling of forest effects will directly help extend valid coverage of the SWE measurements as a global snow mission. A formal assessment of the utility of hypothetical active microwave sensors for SWE estimation under different observing conditions (e.g., deep snow, dense forests, and the presence of liquid water) is, therefore, needed to establish the potential benefits of such future sensors and to set priorities related to algorithm developments.
An observing system simulation experiment (OSSE; Arnold and Dey, 1986; Masutani et al., 2010) is a modeling-based and data-assimilation-based approach that is often used to assess the utility of spaceborne observations from proposed designs of new satellite missions before the instruments are deployed. OSSEs enable the quantification of the utility of spaceborne observations and help in the design and configuration of future missions (Crow et al., 2005; De Lannoy et al., 2010; Garnaud et al., 2019; Kumar et al., 2014a; Kwon et al., 2021; Nearing et al., 2012). Specifically for SWE, De Lannoy et al. (2010) used an OSSE to explore techniques for downscaling coarse-scale SWE products to the underlying fine-scale model state variables within a data assimilation system. More recently, Kwon et al. (2021) conducted light detection and ranging (lidar) OSSE to quantify the accuracy requirement of spaceborne lidar snow depth retrieval, which provides its beneficial impact on SWE and hydrologic variables within a land surface model. They found that synthetic lidar observations provided utility in assimilation processes when the realistic snow depth retrieval's error standard deviation was lower than 60 cm. Like in the current study, Garnaud et al. (2019) used an OSSE to estimate the potential value of the Ku-band radar mission concept for the Environment and Climate Change Canada–Canadian Space Agency (ECCC-CSA) Terrestrial Snow Mass Mission (TSMM). They used an OSSE to provide information on the optimal mission configuration (i.e., resolution, revisit time, and snow mass retrieval uncertainty) using a test bed in southern Quebec, Canada. In the non-mountainous, forested domain, this study found that bias in a baseline SWE simulation was largely reduced by improving the revisit frequency (e.g., 93 % with 1 d revisit time), and systematic errors were also reduced by a higher revisit frequency as well as an increased resolution (1 km rather than 2 or 10 km spatial resolution).
The main objective of this study is to quantify the usefulness of X- and/or Ku-band volume-scattering SAR SWE retrievals at a 1 km spatial resolution and approximately 7 d repeat time for improving spatially distributed characterization of snow conditions through an OSSE setup over a mountainous region of western Colorado. Specifically, we focus on the SWE retrieval utility over deep snow and forest-covered regions. We introduce the study area and describe our OSSE design, including the main steps, in Sects. 2 and 3, respectively. The results of OSSE performances are reported in Sect. 4. Lastly, we discuss implications and limitations and provide concluding remarks in Sect. 5.
The western Colorado region is selected as the OSSE domain and provides a representative continental mountainous region (Fig. 1). The study area includes five seasonal snow classes: tundra (7.1 %), boreal forest (14.3 %), montane forest (44.9 %), prairie (28.9 %), and ephemeral (4.7 %). The seasonal snow classification is based on the 1 km new seasonal snow classification developed by Sturm and Liston (2021). The elevation over the domain ranges from 1400 to 4000 m (41 % of the domain area is between 1400–2500 m, 33 % between 2500–3000 m, and 26 % between 3000–4000 m), which is based on a 1 km elevation map derived from the United States Geological Survey's (USGS) Shuttle Radar Topography Mission (SRTM) “Native” elevation data (Farr et al., 2007). The tree cover fraction (TCF; %) ranges from 0 % to 80 % (49 % of the domain area with 10 % or lower, 13 % with 10 %–20 %, 19 % with 20 %–40 %, 14 % with 40 %–60 %, and 4.3 % with 60 % and higher TCFs). Individual maps of each snow class with different TCF ranges are also included in the Supplement (Fig. S1). The upscaled 1 km TCF map is derived from the 30 m resolution global tree cover data developed by the University of Maryland (Hansen et al., 2013) using a bilinear resampling approach. This domain also includes previous field campaign experiment locations such as those of the NASA–NOAA Cold Land Processes Field Experiment (CLPX; 2001–2003) and NASA SnowEx field campaign (2017, 2020, and 2021).
The OSSE performed in this study focuses on quantifying the beneficial impacts of hypothetical X- and/or Ku-band active microwave SWE observations with different levels of retrieval uncertainties at a 1 km spatial resolution. The OSSE setup includes three main elements: (1) the nature run (NR), (2) the open-loop (OL) simulation, and (3) data assimilation (DA) simulations with synthetic observations (Fig. 2). NR is the calibrated land surface model (LSM) simulation, which is considered the “truth” in the OSSE framework (Sect. 3.2). OL is an uncalibrated LSM simulation as the default configuration (Sect. 3.3). NR and OL are designed at the same spatial resolution (1 km). The DA scenarios are simulation results that assimilate hypothetical synthetic observations with different error constraints with OL. Detailed information about synthetic observations and DA is provided in Sect. 3.4 and 3.5, respectively. To develop realistic synthetic observations, we apply a subsampling method to obtain a realistic active satellite viewing area from hypothetical satellite-based radar using the Trade-space Analysis Tool for designing Constellations (TAT-C) simulator (Le Moigne et al., 2017). The study period used in the analysis is the winter season from 1 October 2016 to 31 May 2017, which experienced moderate snow conditions and provided sufficient differences between NR and OL SWE and thus made the OSSE setup effective for quantifying improvement. A model time step of 15 min was used, and daily averaged model outputs were saved for analysis. For all experiments, relevant physical parameterization options of Noah with multi-parameterization (Noah-MP) version 4.0.1 were used as listed in Table 1. Then we developed 24 DA experiments from synthetic observations with assumptions of uncertainty related to deep snow and forest coverage. Detailed descriptions of how to apply those limitations to DA experiments are given below.
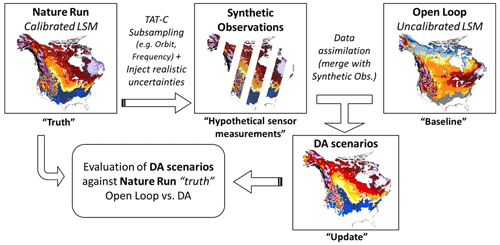
Figure 2Schematic diagram of the synthetic observing system simulation experiment (OSSE) setup of this study. The nature run (NR) indicates synthetic-truth simulation, and open loop (OL) and data assimilation (DA) are model simulations without and with assimilation of the synthetic snow water equivalent (SWE) retrievals, respectively, derived from NR.
Table 1Relevant physical parameterization schemes of Noah-MP (version 4.0.1) used in the observing system simulation experiments (OSSEs).
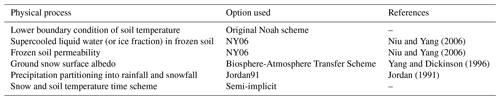
3.1 NASA Land Information System and Noah-MP land surface model
The OSSE simulations were conducted using the NASA Land Information System (LIS; Kumar et al., 2006; Peters-Lidard et al., 2007), which is a software framework for high-performance land surface modeling and data assimilation experiments. Within LIS, we employed Noah-MP LSM version 4.0.1 (Niu et al., 2011; Yang et al., 2011). Noah-MP was developed based on the original Noah LSM (Ek et al., 2003) with augmented representations of biophysical and hydrological processes. Noah-MP includes a multilayer snowpack representation (up to three layers) to simulate the physical processes of varying snow density over time, allowing patchy snow cover to evolve as a function of snow depth and vegetation type. The model simulates snowpack liquid water retention, refreezing of meltwater, and frost/sublimation, all of which are important for the accurate characterization of snow conditions. The model also accounts for snow age, grain size growth, and the effect of impurities on snow evolution. Previous studies found that Noah-MP has superior performance compared to the original Noah LSM and other LSMs for simulating SWE (Cho et al., 2022b; Kim et al., 2021; Minder et al., 2016).
3.2 Nature run (synthetic truth)
We used the calibrated Noah-MP simulation at 0.01∘ spatial resolution (∼ 1 km) described in Wrzesien et al. (2022) for NR in this experiment. The meteorological forcing data for the simulation comprised the North American Land Data Assimilation System phase 2 (NLDAS-2; Xia et al., 2012). In that study, the optimization and uncertainty subsystem (Kumar et al., 2012) within LIS was used to calibrate Noah-MP SWE against estimates from the observation-based University of Arizona gridded snow dataset (UA; Zeng et al., 2018). For the optimization, Wrzesien et al. (2022) used a genetic algorithm to calibrate 23 model parameters related to snow parameterizations that are hard-coded into the default Noah-MP configuration, and an additional snowfall scaling term was included to address precipitation biases in the meteorological forcing data (Enzminger et al., 2019; He et al., 2019; Henn et al., 2018; Raleigh et al., 2015; Schmucki et al., 2014). The calibration approach generated spatially varying parameters, as compared to the spatially uniform values in the default Noah-MP. When evaluated against both UA and SNOw Data Assimilation System (SNODAS) estimates, the calibrated simulation decreased the domain-averaged temporal root mean square error (RMSE) and bias for SWE and snow depth, relative to the default Noah-MP configuration, for the same western Colorado domain as that used here in the OSSE. Further, the snowfall scaling term was shown to be important for increasing the magnitude of snow accumulation, especially in higher-elevation grid cells.
3.3 Open-loop simulation
The model run without assimilation, called the open-loop (OL) simulation, is conducted with meteorological boundary conditions from Modern-Era Retrospective analysis for Research and Applications (MERRA-2, version 2) forcing data produced by NASA's Global Modeling and Assimilation Office (Gelaro et al., 2017). MERRA-2 forcing data, which have a native spatial resolution of 0.5∘ latitude by 0.625∘ longitude (roughly 50 km), are downscaled to a 1 km grid of the model setup within LIS. Note that the OL configuration has two primary differences relative to the NR setup: (1) the boundary conditions are different (MERRA-2 for OL vs. NLDAS-2 for NR) and (2) OL uses the default configuration of Noah-MP, whereas NR uses the calibrated, spatially distributed parameters developed by Wrzesien et al. (2022).
3.4 Synthetic observations with TAT-C subsampling
We develop synthetic SWE observations by including factors that represent uncertainties related to snow estimation over deep snow and when vegetation is present. For deep snow, four different hypothetical limits of retrieval algorithm are considered: 200, 400, and 600 mm and no limit of SWE. The influence of forest cover is examined by considering six scenarios that limit SWE detection at different levels of the forest fraction (0 %, 10 %, 20 %, 40 %, 60 %, and 80 %) based on the 30 m University of Maryland global tree cover fraction (TCF) data (Hansen et al., 2013). The 24 scenarios of active microwave synthetic SWE observations are used in the OSSE. For example, a DA run with a 20 % TCF limit means that grids with a forest fraction >20 % are masked out from DA, assuming that the hypothetical sensor cannot measure SWE in those grids. Because active microwave sensors cannot detect SWE if the snowpack contains liquid water (Matzler, 1987; Rott et al., 2010), synthetic observations are only assimilated when the snowpack does not include liquid water content (LWC). That is, when LWC values from the OL run are positive (>0) in certain grids and periods, corresponding synthetic observations are not assimilated with the OL run. Unbiased random errors with zero mean and 30 mm of standard deviation expected as an error level of the SWE retrievals from previous findings (Rott et al., 2010; Garnaud et al., 2019) are applied to the synthetic observations. To support the impact of the standard deviation on SWE evaluation, different DA scenarios with different ranges of standard deviations (10, 30, 50, and 100 mm) are compared in the Supplement (Figs. S2 and S3).
To simulate the viewing extent of hypothetical X- and/or Ku-band sensors, we use TAT-C (Le Moigne et al., 2017), which is a NASA-developed software system specifically designed for future distributed spacecraft missions (DSMs). TAT-C allows for the exploration of a range of feasible design options (e.g., single vs. constellation, polar-orbiting vs. geostationary, low-frequency vs. high-frequency overpasses) to quantify measurable gains as a function of mission configuration. In this study, the orbital configuration (e.g., Keplerian elements) of a volume-scattering SAR mission is used in the orbit and coverage module to simulate the nadir position track. Then, the realistic spatial coverage and temporal frequency are simulated by extending the ground track to a given swath width (i.e., 250 km) in the cross-track direction. In this study, the viewing extent simulation is expressed as a daily binary map (so-called “cookie cutter”) marking the surface as viewed (or not) at a 1 km spatial resolution. Examples of TAT-C masked swath maps are provided in Fig. S4.
3.5 Data assimilation
For this OSSE work, the one-dimensional ensemble Kalman filter method (Reichle et al., 2002) is used to assimilate synthetic SWE observations into Noah-MP. The ensemble Kalman filter method allows us to flexibly characterize the model errors and to effectively handle non-linear dynamics and temporal discontinuities of observations (Kumar et al., 2015; Kwon et al., 2021; Lahmers et al., 2022; Cho et al., 2022a). The ensemble Kalman filter method includes forecast and update steps. In the forecast step, an ensemble of SWE and snow depth is propagated by Noah-MP until synthetic SWE observations become available. Each ensemble member is generated by perturbing model initial conditions, boundary conditions from a meteorological forcing, and Noah-MP model prognostic variables based on the assumption of a Gaussian distribution. The perturbation parameters used in this study are based on earlier DA works (Table S1; Kumar et al., 2014b; Kwon et al., 2021). Noah-MP OL was initialized by spinning up a simulation from 1 October 2012 to 31 September 2015. After that, a 20-member ensemble run was additionally spun up from 1 October 2015 to 31 September 2016 to establish the initial conditions of the ensemble. The OL and DA scenarios were simulated from 1 October 2016 to 31 May 2017.
3.6 Performance evaluation matrices
For evaluation, the root mean square error, RMSE, between the DA (or OL) SWE and NR SWE over a period is quantified as follows:
SWEDA and SWENR refer to DA (or OL) SWE and the NR SWE, and t is a date. The DA RMSE improvement as compared to baseline (OL) RMSE is calculated as follows:
4.1 Evaluation of OSSE at a domain-averaged scale
4.1.1 The impact of deep-SWE limits
To assess the impact of SWE retrievals on regional snowpack characterization, the DA performance is quantified using domain-averaged SWE; see Fig. 3. This figure shows domain-averaged SWE time series from NR (synthetic truth), OL (baseline), and multiple DA scenarios with different deep-snow limits from shallow (200 mm), moderate (400 mm), and deep (600 mm) SWE and no limit. The analysis also shows SWE from model integrations stratified over different elevation ranges. Note that here we assume no limitations due to forest coverage. For the entire domain, the peak values of the domain-averaged SWE time series of NR and OL are around 220 and 160 mm in early March, respectively. The OL simulation underestimates SWE by 27 % as compared to NR. The underestimations are partially reduced with DA scenarios, except for the DA integration with a 200 mm limit. The DA run with a shallow SWE limit (up to 200 mm; blue line) has little impact on the domain-averaged SWE and even contributes to a degradation near the peak SWE period (February and March). However, the DA scenario with a moderate SWE limit (up to 400 mm; cyan line) shows improvements relative to the OL SWE. This indicates that the retrieval algorithm with an SWE range up to 400 mm would add value to domain-averaged SWE time series in such a mountainous region. The improvement was observed even during the ablation period. As the deep-snow limits further increase (up to 600 mm and no limit), domain-averaged SWE estimates are also improved (see the pink and green lines). The capability to characterize deep snow has a larger impact on areas with higher elevations as those regions typically have deeper snowpacks. For mid-elevation and high-elevation ranges, the DA SWEs with 600 mm and no limits show improvements, whereas few improvements are obtained in low-elevation ranges. This indicates that a large portion of the SWE improvements for the entire domain is contributed by the high-elevation regions. When comparing the DA time series for mid-elevation and high-elevation regions, smaller differences from NR (black line) during the melting period are observed in the high-elevation regions, likely because melt starts later in these areas. The gaps (biases) in the SWE time series between the DA scenario with no limit and NR may be due to the limited ability to detect wet snow and the revisit frequency. Since the random errors added to NR are centered on zero, the random errors may not contribute to the biases found in the domain-averaged approach.
4.1.2 The impact of the sensor's detection capability over the forest fraction
To quantify the SWE characterization based on the sensor's capabilities over forest cover, domain-averaged SWE time series from DA scenarios with simulated observations capturing SWE in areas with bare ground (i.e., ability to detect SWE in bare-ground areas only where TCF rounds off to 0 %) or TCF limits of up to 10 %, 20 %, 40 %, 60 %, and 80 % (i.e., ability to detect SWE even in densely forested areas up to 80 % TCF) are shown in Fig. 4. The domain-averaged NR SWE (which is the synthetic truth) has a larger SWE than the DA and OL SWE throughout the whole period, and the differences are larger in the melting period than in the accumulation period. During the accumulation period, there are similar improvements in SWE (up to 25 %) among the DA scenarios with different TCF limits as compared to OL, except for TCF of 0 % which was similar to OL. The SWE difference between the DA scenarios slightly increases after the large melting event in March. This tendency continues until early May, when there are melting events. For areas with low and middle elevations, there are small SWE differences among most DA scenarios with different TCFs ranging from 10 % to 80 %. In areas with high elevations, there appear to be larger SWE differences between the DA scenarios in April and May than at lower elevations. The SWE improvements gradually increase with the increasing detection capability of TCFs. In other words, while the SWE retrieval capability in denser forests has a lower impact on the domain-averaged SWE performance in the low and middle elevations of this domain where there are fewer forested areas, it has larger impacts over high elevations. For areas with high elevations, the DA SWEs with 10 %–40 % TCFs show improvements, while no improvements are obtained in lower-elevation ranges, indicating that a large portion of the SWE improvement for the entire domain is from high-elevation regions.
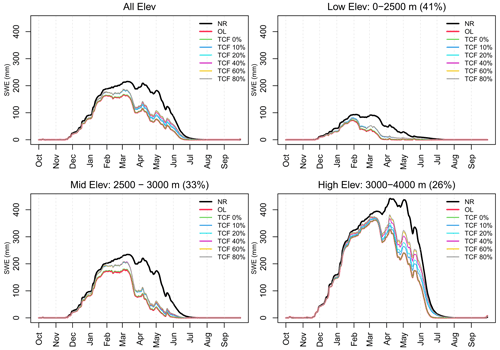
Figure 4Domain-averaged SWE comparison between NR, OL, and DA experiments with different levels of detection capability in areas with bare ground and tree cover fraction (TCF) limits of up to 10 %, 20 %, 40 %, 60 %, and 80 %. The areal proportions of the TCFs for three elevation ranges are provided in Table S2.
4.1.3 Different performances between accumulation and melting periods
Figure 5 provides a comprehensive comparison of RMSEs and the percent improvement calculated by the time series of domain-averaged SWE between all DA scenarios and the OL simulation relative to NR. DA performances are different between accumulation and melting periods, where, generally, the RMSEs between DA (or OL) and NR during the accumulation period are smaller than those during the melting period. While the RMSEs range from 16 mm (no limit for deep SWE and an ability to detect SWE at up to 80 % TCF) to 28 mm (200 mm SWE limit and 80 % TCF) for the accumulation period, the RMSEs' range for the melting period is between 33 and 47 mm. Note that the baseline OL simulation itself had a large difference between the two periods (accumulation 24 mm and melting 44 mm). The percentages of RMSE improvements calculated using Eq. (2) show relative improvements in DA scenarios from OL for a given period. As shown in Fig. 3, the DA scenarios with a shallow SWE limit (up to 200 mm) show little impact or degradation for domain-averaged SWE estimations as compared to OL for both periods. This implies that even though a hypothetical mission can provide a finer-spatial-resolution SWE product (1 km) than existing passive microwave missions (e.g., 25 km), we could not achieve the better SWE estimates unless the hypothetical mission has better retrieval algorithms than that of passive microwave missions (e.g., 200 mm deep-SWE limit and 20 % TCF). The DA scenarios with 400 and 600 mm and with no limits clearly show improvements for both periods, and the level of the improvements varies by TCF. For the accumulation period, the RMSEs were reduced by around 15 % (and 23 %) with capabilities for up to the 400 mm (600 mm) limit and 10 % or larger TCFs. For the melting period, the percent improvement is relatively small, ranging from 1 % (400 mm limit and TCF 10 %) to 16 % (600 mm limit and TCF 80 %). For the DA scenarios without deep-snow limits, the improvements range from 26 % to 33 % and from 12 % to 26 % for the accumulation and melting periods, respectively. This indicates that the ability of the active SWE retrievals to handle deep snow could help in achieving better estimations of SWE.
4.2 Spatial evaluation of SWE performance
In this section, we evaluate the DA performance based on the spatially distributed RMSE values. Figure 6 shows an example of spatial maps showing the annual-mean SWE distributions from NR (Fig. 6a), OL (Fig. 6b), and a DA (Fig. 6e) scenario, with no deep-SWE limit but TCF 40 %, along with a map of the number of valid days used to calculate RMSE. The annual-mean NR SWE map is noticeably different from that of OL. The annual-mean DA SWE map shows similar spatial patterns to OL and NR but different magnitudes regionally. The two RMSE maps also show similar spatial patterns but of regionally different magnitudes. For this DA run, there are clear differences between the two maps over areas with TCF <40 % such as a north-central region and some southern parts of the study area (e.g., Rio Grande National Forest). Figure 7 provides a spatial comparison of RMSE between DA scenarios with the four deep-SWE limits and NR. With increasingly deep-SWE limits, the DA's RMSEs decrease over mountainous regions where NR SWE is typically high. While there are some degradations over areas where SWEs are typically low (e.g., red color in Fig. 7b), the RMSE difference maps between DA and OL demonstrate that RMSEs can be improved by more than 400 mm (areas with blue color), highlighting the importance of SWE retrievals' capability for deep snow in those mountainous environments.
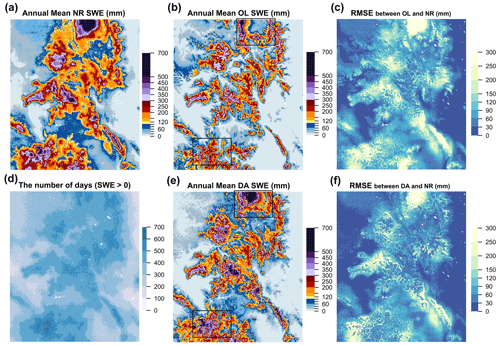
Figure 6The annual-mean SWE maps of the nature run (NR; a, d) and the open-loop (OL) simulation (b), as well as of a data assimilation (DA) run with no deep-SWE limit but a TCF limit up to 40 % (e), as an example, and the RMSE maps of OL (c) and DA (f) against NR.
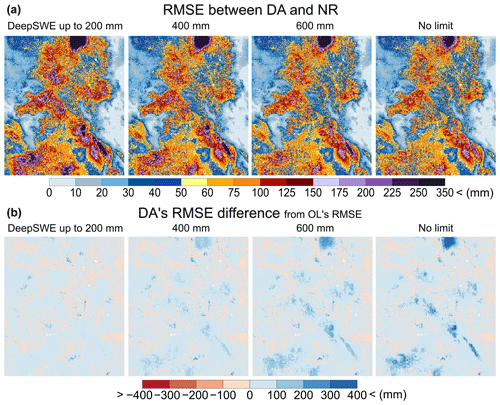
Figure 7(a) The RMSE maps between DA experiments with different deep-snow limits (200, 400, and 600 mm and no limit) against the nature run (NR) and (b) the four DA experiments' RMSE difference maps from OL's RMSE. Note that the four DA scenarios are with no tree cover fraction (TCF) limit (white color indicates areas with no DA occurrence such as lakes).
To quantify the improvements of 24 DA experiments relative to the OL run, RMSE comparisons between DA experiments and NR from all grid cells over the study domain are provided in Fig. 8. The RMSE boxplot of OL (bottom) has a range from 34 mm (lower quartile; Q1) to 112 mm (upper quartile; Q3) with a median of 67 mm (Q2). Each DA run shows different ranges of the RMSEs as compared to OL. For example, the DA run with a 200 mm SWE limit and a TCF limit up to 20 % has slightly lower RMSEs (median: 63 mm) ranging from 28 mm (Q1) to 108 mm (Q3). For a DA run with a better capability to detect deep SWE up to 600 mm and denser TCF up to 40 %, the median RMSE decreases by 67 to 50 mm. If the hypothetical sensors with an ideal retrieval algorithm have a better capability to detect all deep SWE values with TCF up to 80 %, the DA run has RMSEs ranging from 24 to 76 mm (median RMSE: 46 mm), a reduction by about 21 mm compared to OL's RMSEs.
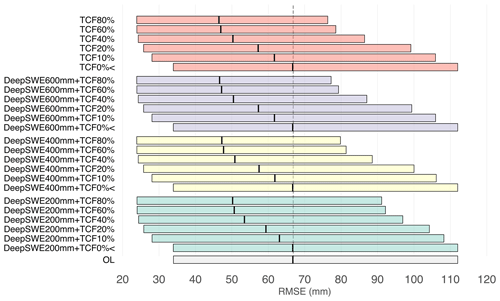
Figure 8Boxplots of RMSE (mm) from all grid cells between the 24 DA experiments having different combinations of deep-snow and tree cover fraction (TCF) detection limits and the nature run (NR) relative to the open-loop (OL) simulation. The vertical black lines in each boxplot indicate the median value.
To present the error improvements from each DA experiment effectively, spatial-mean RMSEs and improvements (%) of RMSEs for the 24 DA experiments relative to OL RMSE are provided in Fig. 9. The RMSEs of DA experiments with TCF 10 % are improved by 7 % (RMSE: 80 mm) to 10 % (RMSE: 73 mm) depending on the degree of deep-snow limits. The DA experiments with TCF 80 % can reduce errors by up to 25 % (RMSE: 54 mm) if there is no limit with deep SWE. The DA scenarios with TCF 40 % are capable of achieving up to 20 % improvements in RMSE, suggesting that it would be worth improving the retrieval algorithm to detect SWE in regions with forest fractions up to 40 %. To achieve around 20 % of the RMSE improvements, the SWE retrievals may have to work with either 600 mm of deep SWE with TCF 40 % or 400 mm of deep SWE with TCF 60 %.
4.3 OSSE performances by seasonal snow classes
The spatial-mean percentages of the RMSE improvement by seasonal snow classification developed by Sturm and Liston (2021) are presented in Fig. 10. The domain consists of four seasonal snow classes (except ephemeral): tundra (7.1 %), boreal forest (14.3 %), montane forest (44.9 %), and prairie (28.9 %). Maps of each seasonal snow class with different TCF ranges over the domain are provided in Fig. S1. Figure 10 reveals that the error improvements differ by snow classification, and thus different priorities for the algorithm development may be required by seasonal snow characteristics. For example, in the tundra class, there are large differences in performance between TCF 0 % and TCF 10 %, but minimal changes are found beyond TCF 10 %, due to the lack of trees in tundra environments. The ability to measure deep SWE is also important in this class because there are larger improvements with increasing deep-snow thresholds, whereas there are relatively small improvements with different TCF levels. In boreal and montane forest classes, there are large differences in performance between TCF 20 % and TCF 40 %, suggesting that the capability of the SWE retrieval algorithm even up to TCF 40 % can provide considerable improvement in SWE estimates in both forest environments. In the prairie class, the largest differences in performance are between the deep-snow limits of 200 and 400 mm, but minimal changes are found beyond 400 mm. This is because the prairie class typically has a shallow snowpack. Thus, a matured retrieval algorithm with active microwave sensors detecting SWE up to 400 mm may be enough to obtain accurate SWE measurements over the prairie snow class. Overall, priorities to improve the capabilities of the retrieval algorithm for deep snow or forest areas could differ by snow class based on the mission's goal.
Active microwave (radar) sensors have great potential to measure SWE because of their sensitivity to the volume scattering of dry snow, with enhanced capabilities in deep snow and forest effects at higher resolutions (Lievens et al., 2019; Tsang et al., 2022) relative to existing missions (e.g., passive microwave sensors). The OSSE results from this study suggest that the radar snow mission may be able to reduce the RMSE by 20 % in the mountainous regions if the retrieval algorithm works in snowpack environments with up to 600 mm of deep SWE with 40 % TCF. This means that algorithm developments should focus on enhancing the retrieval skill in both deep snowpack and moderate forest fractions. This could be achievable based on previous and ongoing efforts to demonstrate a sensitivity of X- and/or Ku-band signals to deep SWE in a forested environment. Recent studies found potential of X- and/or Ku-band backscatters to estimate SWE by testing them in various snow environments. Borah et al. (2022) showed a sensitivity of co-polarization backscatters from airborne X- and Ku-band data to deep SWE more than 650 mm when using a bi-continuous dense media radiative transfer (DMRT) model. Santi et al. (2022) found that the X-band backscattering coefficient from the COSMO-SkyMed satellite can estimate deep SWE up to 800 mm using a retrieval approach and machine learning methods. In preparation for ESA's CoReH2O mission, Montomoli et al. (2015) demonstrated, over a boreal forest site in northern Finland, that the X- and Ku-band SWE retrieval can provide SWE in a forested region with prior knowledge of TCF and tree height if TCF is smaller than about 30 %. It is possible that the SWE retrieval could be useful in areas with denser TCFs if detailed canopy structure, canopy height, and forest types are available and adequate corrections can be applied (Tsang et al., 2022).
A radar-focused OSSE has been recently performed by Garnaud et al. (2019) to assess the utility of hypothetical snow observations in southern Quebec, Canada. As a part of the ECCC-CSA TSMM concept study, they conducted a Ku-band radar OSSE to quantify trade-offs between SWE performance and sensor configurations and the retrieval algorithm accuracy. There are several differences between the current study and Garnaud et al. (2019) in terms of domain characteristics, objectives, and conclusions. While Garnaud's work focused on lower-elevation (0–700 m elevation), forest-dominant regions with shallow and moderate snowpack (e.g., 80 mm of the peak SWE from the synthetic truth), this study focuses on a mountainous domain in western Colorado, with a wider range of high elevations (1000 to 4000 m) including various seasonal snow types. This domain includes both shallow snow at lower elevations (peak SWE: 95 mm) and deep snow at high elevations (peak SWE: 430 mm in Fig. 3), enabling us to quantify the utility of active microwave SWE stratified over deep-snow limits as well as snow classes. Both studies indicate that a SAR-based snow mission using a volume-scattering retrieval approach can significantly improve SWE estimates at various ranges of elevations, forest cover, and snow depths. The major findings from both studies complement one another. Garnaud et al. (2019) determined the impact of different spatial resolutions (i.e., 1, 2, 10 km), revisit frequencies (i.e., 1, 3, 5 d), and retrieval algorithm accuracies. In this study, with achievable sensor configurations (1 km spatial resolution, 7 d repeat time, and the realistic orbital configurations for a volume-scattering SAR mission developed using TAT-C), we demonstrated the impact of potential limitations (e.g., deep snow and forest fractions) on the SWE performance to help prioritize the algorithm developments. Also, we quantified the value of SWE assimilation over complex mountainous terrain through improved SWE retrievals of deep snow and snow in forested areas.
There are limitations to this study that may need to be considered in future research. First, the domain of this study (i.e., western Colorado) contains four seasonal snow classes and wide elevation ranges, enabling us to represent mountainous environments and quantify approximate performances in other regions that have similar snow regimes and land surface characteristics. However, we acknowledge that it is not enough to extrapolate our findings to global coverage of a future mission concept. Further OSSE investigations with multiple domains with different snow climates, vegetation characteristics, and terrain complexity (e.g., steep vs. flat terrain) will complement current efforts. Secondly, we applied a spatially constant error across the domain. While the error (30 mm standard deviation with zero mean) was based on the expected uncertainty from previous studies (e.g., Rott et al., 2010), spatially and temporally dynamic error characteristics of the radar (e.g., multiplicative errors according to the amount of SWE) in OSSEs could improve the performance assessment. At the same time, radar uncertainty in snowpack depends on the temporal evolution of snowpack and detailed spatial features of land properties (e.g., snow microstructure, tree structures, and canopy distribution within a grid). With ongoing efforts from current and upcoming field campaigns such as NASA SnowEx campaigns and airborne Cryosphere-Observing SAR (CryoSAR; led by Richard Kelly at the University of Waterloo), radar snow error characteristics will be better quantified in various environments, helping to develop more realistic OSSEs. Thirdly, even though the OSSE of this study considers realistic sensor configurations for a volume-scattering SAR mission using the TAT-C software, there are inherent geometrical limitations of SAR sensors (i.e., shadow/overlay) which complicate the retrieval of surface properties in mountain regions such as the region of interest in this study. To design the OSSE more accurately, the geometrical observing gaps related to incidence angles of the SAR sensors and surface elevations should be accurately estimated. This may increase the number of masked grid cells that would not have SWE retrievals from hypothetical satellite observations. Lastly, the improvement of the SWE uncertainties is inherently affected by the choice of land surface models, meteorological boundary conditions, and spatial and temporal domains. Future studies to quantify the impact of these contributing sources on the performance assessment will help maximize the suitability of the OSSE design.
In summary, we developed OSSEs that include characterization of expected error levels of SWE estimates and realistic orbital configurations of anticipated sensors within NASA LIS over a western Colorado domain. We found that active microwave X- and/or Ku-band frequencies can improve SWE estimation in a mountainous region over western Colorado. The active microwave sensors provided larger SWE improvements in tundra (43 %) and boreal forest (22 %) snow classes, and there are some improvements in the montane forest (17 %) due to the sensor capability for deep snowpack. Active microwave sensors, known to have limitations in relation to liquid water, can still reduce errors by up to 6 %–16 % of domain-averaged SWE even in the melting period, depending on TCFs, suggesting that active microwave SWE retrievals can add value for hydrological applications. Overall, this work provides general quantification of the utility of potential radar mission concepts for SWE in a mountainous domain, helping to prioritize algorithm developments and relevant upcoming field campaigns.
The LIS outputs and configuration files used in this study are available at http://www.hydroshare.org/resource/1ad0d4b62c4440e9bb9267a7470d7b81 (Cho et al., 2023). The Global Seasonal-Snow Classification data, Version 1, are available at the National Snow and Ice Data Center (NSIDC) (https://doi.org/10.5067/99FTCYYYLAQ0, Liston and Sturm, 2021). The USGS Shuttle Radar Topography Mission (SRTM) Native elevation data are available at the USGS Earth Resources Observation and Science (EROS) Center website (https://doi.org/10.5066/F7PR7TFT, USGS Earth Resources Observation and Science (EROS) Center, 2018). The 30 m resolution global tree cover data are available at https://glad.umd.edu/Potapov/TCC_2010/ (Hansen et al., 2013). The MERRA-2 forcing dataset is available at the NASA Goddard Global Modeling and Assimilation Office website (GMAO; https://gmao.gsfc.nasa.gov/reanalysis/MERRA-2/data_access/, Gelaro et al., 2017). NASA LIS source code is publicly available at https://github.com/NASA-LIS/LISF (Kumar et al., 2006).
The supplement related to this article is available online at: https://doi.org/10.5194/tc-17-3915-2023-supplement.
EC conceptualized the research, led the investigation, did the formal analysis, and wrote the initial draft. CMV and SVK conceptualized the research, took responsibility for the investigation, and supervised the project. RSK and MLW helped with the model simulations and investigation and provided technical and scientific inputs. All authors reviewed and edited the paper.
At least one of the (co-)authors is a member of the editorial board of The Cryosphere. The peer-review process was guided by an independent editor, and the authors also have no other competing interests to declare.
Publisher’s note: Copernicus Publications remains neutral with regard to jurisdictional claims in published maps and institutional affiliations.
Computing resources to run the NASA Land Information System (LIS) were supported by the NASA Center for Climate Simulation.
This research has been supported by the National Aeronautics and Space Administration (grant no. NNH16ZDA001N).
This paper was edited by Patricia de Rosnay and reviewed by Melody Sandells and one anonymous referee.
Arnold, C. and Dey, C.: Observing-systems simulation experiments: Past, present, and future, B. Am. Meteor. Soc., 67, 687–695, https://doi.org/10.1175/1520-0477(1986)067<0687:OSSEPP>2.0.CO;2., 1986.
Barnett, T. P., Adam, J. C., and Lettenmaier, D. P.: Potential impacts of a warming climate on water availability in snow-dominated regions, Nature, 438, 303–309, 2005.
Borah, F. K., Tsang, L., Kang, D. K., Kim, E., Siqueira, P., Barros, A., and Durand, M.: Data Analysis and SWE Retrieval of Airborne SAR Data AT X Band and KU Bands, in: 2022 IEEE International Geoscience and Remote Sensing Symposium, Kuala Lumpur, Malaysia, 17–22 July 2022, 4252–4255, https://doi.org/10.1109/IGARSS46834.2022.9884965, 2022.
Bormann, K. J., Brown, R. D., Derksen, C., and Painter, T. H.: Estimating snow-cover trends from space, Nat. Clim. Change, 8, 924–928, 2018.
Carroll, S. S., Carroll, T. R., and Poston, R. W.: Spatial modeling and prediction of snow-water equivalent using ground-based, air-borne, and satellite snow data, J. Geophys. Res., 104, 19623–19629, https://doi.org/10.1029/1999JD900093, 1999.
Cline, D., Yueh, S., Chapman, B., Stankov, B., Gasiewski, A., Masters, D., Elder, K. J., Kelly, R., Painter, T. H., Miller, S., Katzberg, S., and Mahrt, L.: NASA cold land processes experiment (CLPX 2002/03): Airborne remote sensing, J. Hydrometeorol., 10, 338–346, 2009.
Cho, E., Tuttle, S. E., and Jacobs, J. M.: Evaluating consistency of snow water equivalent retrievals from passive microwave sensors over the north central US: SSM/I vs. SSMIS and AMSR-E vs. AMSR2, Remote Sensing, 9, 465, https://doi.org/10.3390/rs9050465, 2017.
Cho, E., Jacobs, J. M., and Vuyovich, C.: The value of long-term (40 years) airborne gamma radiation SWE record for evaluating three observation-based gridded SWE datasets by seasonal snow and land cover classifications, Water Resour. Res., 56, e2019WR025813, https://doi.org/10.1029/2019WR025813, 2020.
Cho, E., Kwon, Y., Kumar, S. V., and Vuyovich, C. M.: Assimilation of airborne gamma observations provides utility for snow estimation in forested environments, Hydrol. Earth Syst. Sci. Discuss. [preprint], https://doi.org/10.5194/hess-2022-332, in review, 2022a.
Cho, E., Vuyovich, C. M., Kumar, S. V., Wrzesien, M. L., Kim, R. S., and Jacobs, J. M.: Precipitation biases and snow physics limitations drive the uncertainties in macroscale modeled snow water equivalent, Hydrol. Earth Syst. Sci., 26, 5721–5735, https://doi.org/10.5194/hess-26-5721-2022, 2022b.
Cho, E., Vuyovich, C. M., Kumar, S. V., Wrzesien, M. L., and Kim, R. S.: Data for ”Evaluating the utility of active microwave observations as a snow mission concept using observing system simulation experiments”, HydroShare, http://www.hydroshare.org/resource/1ad0d4b62c4440e9bb9267a7470d7b81 (last access: 7 September 2023), 2023.
Crow, W. T., Chan, S. T. K., Entekhabi, D., Houser, P. R., Hsu, A. Y., Jackson, T. J., Njoku, E. G., O'Neill, P. E., Shi, J., and Zhan, X.: An observing system simulation experiment for Hydros radiometer-only soil moisture productsm IEEE T. Geosci. Remote Sens., 43, 1289–1303, 2005.
De Lannoy, G. J. M., Reichle, R. H., Houser, P. R., Arsenault, K. R., Verhoest, N. E. C., and Pauwels, V. R. N.: Satellite-Scale Snow Water Equivalent Assimilation into a HighResolution Land Surface Model, J. Hydrometeorol., 11, 352–369, https://doi.org/10.1175/2009JHM1192.1, 2010.
Derksen, C., Walker, A., and Goodison, B.: Evaluation of passive microwave snow water equivalent retrievals across the boreal forest/tundra transition of western Canada, Remote Sens. Environ., 96, 315–327, https://doi.org/10.1016/j.rse.2005.02.014, 2005.
Derksen, C., Toose, P., Rees, A., Wang, L., English, M., Walker, A., and Sturm, M.: Development of a tundra-specific snow water equivalent retrieval algorithm for satellite passive microwave data, Remote Sens. Environ., 114, 1699–1709, https://doi.org/10.1016/j.rse.2010.02.019, 2010.
Derksen, C., Lemmetyinen, J., King, J., Belair, S., Garnaud, C., Lapointe, M., Crevier, Y., Burbidge, G., and Siqueira, P.: A DualFrequency Ku-Band Radar Mission Concept for Seasonal Snow, in: IGARSS 2019–2019 IEEE International Geoscience and Remote Sensing Symposium, IEEE, Yokohama, Japan, 28 July–2 August 2019, 5742–5744, https://doi.org/10.1109/IGARSS.2019.8898030, 2019.
Dong, J., Walker, J., and Houser, P.: Factors affecting remotely sensed snow water equivalent uncertainty, Remote Sens. Environ., 97, 68–82, https://doi.org/10.1016/j.rse.2005.04.010, 2005.
Dozier, J., Bair, E. H., and Davis, R. E.: Estimating the spatial distribution of snow water equivalent in the world's mountains, Wiley Interdisciplinary Reviews: Water, 3, 461–474, https://doi.org/10.1002/wat2.1140, 2016.
Ek, M. B., Mitchell, K., and Lin, Y.: Implementation of Noah land surface model advances in the National Centers for Environmental Prediction operational mesoscale Eta model, J. Geophys. Res., 108, 8851, https://doi.org/10.1029/2002JD003296, 2003.
Elder, K., Cline, D., Liston, G. E., and Armstrong, R.: NASA Cold Land Processes Experiment (CLPX 2002/03): field measurements of snowpack properties and soil moisture, J. Hydrometeorol., 10, 320–329, https://doi.org/10.1175/2008jhm877.1, 2009.
Farr, T. G., Rosen, P. A., Caro, E., Crippen, R., Duren, R., Hensley, S., Kobrick, M., Paller, M., Rodriguez, E., Roth, L., Seal, D., Shaffer, S., Shimada, J., Umland, J., Werner, M., Oskin, M., Burbank, D., and Alsdorf, D.: The Shuttle Radar Topography Mission, Rev. Geophys., 45, 1–33, https://doi.org/10.1029/2005rg000183, 2007.
Foster, J. L., Sun, C., Walker, J. P., Kelly, R., Chang, A., Dong, J., and Powell, H.: Quantifying the uncertainty in passive microwave snow water equivalent observations, Remote Sens. Environ., 94, 187–203, 2005.
Enzminger, T. L., Small, E. E., and Borsa, A. A.: Subsurface water dominates Sierra Nevada seasonal hydrologic storage, Geophys. Res. Lett., 46, 11993–12001, 2019.
Garnaud, C., Bélair, S., Carrera, M. L., Derksen, C., Bilodeau, B., Abrahamowicz, M., Gauthier, N., and Vionnet, V.: Quantifying Snow Mass Mission Concept Trade-Offs Using an Observing System Simulation Experiment, J. Hydrometeorol., 20, 155–173, https://doi.org/10.1175/JHM-D-17-0241.1, 2019.
Gelaro, R., McCarty, W., Suárez, M. J., Todling, R., Molod, A., Takacs, L., Randles, C. A., Darmenov, A., Bosilovich, M. G., Reichle, R., Wargan, K., Coy, L., Cullather, R., Draper, C., Akella, S., Buchard, V., Conaty, A., da Silva, A. M., Gu, W., Kim, G. K., Koster, R., Lucchesi, R., Merkova, D., Nielsen, J. E., Partyka, G., Pawson, S., Putman, W., Rienecker, M., Schubert, S. D., Sienkiewicz, M., and Zhao, B.: The modern-era retrospective analysis for research and applications, version 2 (MERRA2), J. Climate, 30, 5419–5454, https://doi.org/10.1175/JCLI-D-16-0758.1, 2017 (data available at: https://gmao.gsfc.nasa.gov/reanalysis/MERRA-2/data_access/, last access: 1 October 2022).
Hansen, M. C., Potapov, P. V., Moore, R., Hancher, M., Turubanova, S. A., Tyukavina, A., Thau, D., Stehman, S. V., Goetz, S. J., Loveland, T. R., Kommareddy, A., Egorov, A., Chini, L., Justice, C. O., and Townshend, J. R. G.: High-Resolution Global Maps of 21st-Century Forest Cover Change, Science, 342, 850–853, 2013 (data available at: https://glad.umd.edu/Potapov/TCC_2010/, last access: 1 October 2022).
He, C., Chen, F., Barlage, M., Liu, C., Newman, A., Tang, W., Ikeda, K., and Rasmussen, R.: Can Convection-Permitting Modeling Provide Decent Precipitation for Offline High-Resolution Snowpack Simulations Over Mountains?, J. Geophys. Res.-Atmos., 124, 12631–12654, https://doi.org/10.1029/2019JD030823, 2019.
Henn, B., Newman, A. J., Livneh, B., Daly, C., and Lundquist, J. D.: An assessment of differences in gridded precipitation datasets in complex terrain, J. Hydrol., 556, 1205–1219, https://doi.org/10.1016/j.jhydrol.2017.03.008, 2018.
Immerzeel, W. W., Lutz, A. F., Andrade, M., Bahl, A., Biemans, H., Bolch, T., Hyde, S., Brumby, S., Davies, B. J., Elmore, A. C., Emmer, A., Feng, M., Fernández, A., Haritashya, U., Kargel, J. S., Koppes, M., Kraaijenbrink, P. D. A., Kulkarni, A. V., Mayewski, P. A., Nepal, S., Pacheco, P., Painter, T. H., Pellicciotti, F., Rajaram, H., Rupper, S., Sinisalo, A., Shrestha, A. B., Viviroli, D., Wada, Y., Xiao, C., Yao, T., and Baillie, J. E. M.: Importance and vulnerability of the world's water towers, Nature, 577, 364–369, https://doi.org/10.1038/s41586-019-1822-y, 2019.
Jordan, R. E.: A one-dimensional temperature model for a snow cover: Technical documentation for SNTHERM, 89, https://erdc-library.erdc.dren.mil/jspui/bitstream/11681/11677/1/SR-91-16.pdf (last access: 1 October 2022), 1991.
Kang, D. H., Barros, A. P., and Dery, S. J.: Evaluating passive microwave radiometry for the dynamical transition from dry to wet snowpacks, IEEE T. Geosci. Remote Sens., 52, 3–15, 2013.
Kim, R. S., Kumar, S., Vuyovich, C., Houser, P., Lundquist, J., Mudryk, L., Durand, M., Barros, A., Kim, E. J., Forman, B. A., Gutmann, E. D., Wrzesien, M. L., Garnaud, C., Sandells, M., Marshall, H.-P., Cristea, N., Pflug, J. M., Johnston, J., Cao, Y., Mocko, D., and Wang, S.: Snow Ensemble Uncertainty Project (SEUP): quantification of snow water equivalent uncertainty across North America via ensemble land surface modeling, The Cryosphere, 15, 771–791, https://doi.org/10.5194/tc-15-771-2021, 2021.
King, J., Derksen, C., Toose, P., Langlois, A., Larsen, C., Lemmetyinen, J., Marsh, P., Montpetit, B., Roy, A., Rutter, N., and Sturm, M.: The influence of snow microstructure on dual-frequency radar measurements in a tundra environment, Remote Sens. Environ., 215, 242–254, https://doi.org/10.1016/j.rse.2018.05.028, 2018.
Kumar, S. V., Peters-Lidard, C. D., Tian, Y., Houser, P. R., Geiger, J., Olden, S., Lighty, L., Eastman, J. L., Doty, B., and Dirmeyer, P.: Land information system: An interoperable framework for high resolution land surface modeling, Environ. Model. Softw., 21, 1402–1415, 2006 (code available at: https://github.com/NASA-LIS/LISF, last access: 1 July 2023).
Kumar, S. V., Reichle, R. H., Harrison, K. W., Peters-Lidard, C. D., Yatheendradas, S., and Santanello, J. A.: A comparison of methods for a priori bias correction in soil moisture data assimilation, Water Resour. Res., 48, W03515, https://doi.org/10.1029/2010WR010261, 2012.
Kumar, S. V., Harrison, K. W., Peters-Lidard, C. D., Santanello Jr., J. A., and Kirschbaum, D.: Assessing the impact of L-band observations on drought and flood risk estimation: A decision-theoretic approach in an OSSE environment, J. Hydrometeorol., 15, 2140–2156, 2014a.
Kumar, S. V., Peters-Lidard, C. D., Mocko, D., Reichle, R., Liu, Y., Arsenault, K. R., Xia, Y., Ek, M., Riggs, G., Livneh, B., and Cosh, M.: Assimilation of remotely sensed soil moisture and snow depth retrievals for drought estimation, J. Hydrometeorol., 15, 2446–2469, 2014b.
Kumar, S. V., Peters-Lidard, C. D., Arsenault, K. R., Getirana, A., Mocko, D., and Liu, Y.: Quantifying the added value of snow cover area observations in passive microwave snow depth assimilation, J. Hydrometeorol., 16, 1736–1741, https://doi.org/10.1175/JHM-D-15-0021.1, 2015.
Kwon, Y., Yoon, Y., Forman, B. A., Kumar, S. V., and Wang, L.: Quantifying the observational requirements of a space-borne LiDAR snow mission, J. Hydrol., 601, 126709, https://doi.org/10.1016/j.jhydrol.2021.126709, 2021.
Lahmers, T. M., Kumar, S. V., Rosen, D., Dugger, A., Gochis, D. J., Santanello, J. A., Gangodagamage, C., and Dunlap, R.: Assimilation of NASA's Airborne Snow Observatory Snow Measurements for Improved Hydrological Modeling: A Case Study Enabled by the Coupled LIS/WRF-Hydro System, Water Resour. Res., 58, e2021WR029867, https://doi.org/10.1029/2021WR029867, 2022.
Larue, F., Royer, A., De Sève, D., Langlois, A., Roy, A., and Brucker, L.: Validation of GlobSnow-2 snow water equivalent over Eastern Canada, Remote Sens. Environ., 194, 264–277, https://doi.org/10.1016/j.rse.2017.03.027, 2017.
Le Moigne, J., Dabney, P., de Weck, O., Foreman, V., Grogan, P., Holland, M., Hughes, S., and Nag, S.: Tradespace analysis tool for designing constellations (TAT-C), in: 2017 IEEE International Geoscience and Remote Sensing Symposium (IGARSS), 1181–1184, 2017.
Lettenmaier, D. P., Alsdorf, D., Dozier, J., Huffman, G. J., Pan, M., and Wood, E. F.: Inroads of remote sensing into hydrologic science during the WRR era, Water Resour. Res., 51, 7309–7342, https://doi.org/10.1002/2015WR017616, 2015.
Li, D., Wrzesien, M. L., Durand, M., Adam, J., and Lettenmaier, D. P.: How much runoff originates as snow in the western United States, and how will that change in the future?, Geophys. Res. Lett., 44, 6163–6172, 2017.
Lievens, H., Demuzere, M., Marshall, H. P., Reichle, R. H., Brucker, L., Brangers, I., de Rosnay, P., Dumont, M., Girotto, M., Immerzeel, W. W., Jonas, T., Kim, E. J., Koch, I., Marty, C., Saloranta, T., Schöber, J., and De Lannoy, G. J. M.: Snow depth variability in the Northern Hemisphere mountains observed from space, Nat. Commun., 10, 4629, https://doi.org/10.1038/s41467-019-12566-y, 2019.
Lievens, H., Brangers, I., Marshall, H.-P., Jonas, T., Olefs, M., and De Lannoy, G.: Sentinel-1 snow depth retrieval at sub-kilometer resolution over the European Alps, The Cryosphere, 16, 159–177, https://doi.org/10.5194/tc-16-159-2022, 2022.
Liston, G. E. and Sturm, M.: Global Seasonal-Snow Classification, Version 1, Boulder, Colorado USA, National Snow and Ice Data Center [data set], https://doi.org/10.5067/99FTCYYYLAQ0, 2021.
Macelloni, G., Paloscia, S., Pampaloni, P., and Tedesco, M.: Microwave emission from dry snow: A comparison of experimental and model results, IEEE T. Geosci. Remote Sens., 39, 2649–2656, 2001.
Magagi, R., Bernier, M., and Ung, C. H.: Quantitative analysis of RADARSAT SAR data over a sparse forest canopy, IEEE T. Geosci. Remote Sens., 40, 1301–1313, 2002.
Masutani, M., Woollen, J. S., Lord, S. J., Emmitt, G. D., Kleespies, T. J., Wood, S. A., Greco, S., Sun, H. B., Terry, J., Kapoor, V., Treadon, R., and Campana, K. A.: Observing system simulation experiments at the National Centers for Environmental Prediction, J. Geophys. Res.-Atmos., 115, D07101, https://doi.org/10.1029/2009JD012528, 2010.
Mätzler, C.: Applications of the interaction of microwaves with the natural snow cover, Remote Sens. Rev., 2, 259–387, https://doi.org/10.1080/02757258709532086, 1987.
Minder, J. R., Letcher, T. W., and Skiles, S. M.: An evaluation of high-resolution regional climate model simulations of snow cover and albedo over the Rocky Mountains, with implications for the simulated snow-albedo feedback, J. Geophys. Res.-Atmos., 121, 9069–9088, https://doi.org/10.1002/2016JD024995, 2016.
Molotch, N. P. and Bales, R. C.: Scaling snow observations from the point to the grid element: Implications for observation network design, Water Resour. Res., 41, W11421, https://doi.org/10.1029/2005WR004229, 2005.
Montomoli, F., Macelloni, G., Brogioni, M., Lemmetyinen, J., Cohen, J., and Rott, H.: Observations and simulation of multifrequency SAR data over a snow-covered boreal forest, IEEE J. Sel. Top. Appl., 9, 1216–1228, https://doi.org/10.1109/JSTARS.2015.2417999, 2015.
Nagler, T., Rott, Heidinger, M., Malcher, P., Macelloni, G., Pettinato, S., Santi, E., Essery, R., Pulliainen, J., Takal, M., Malnes, E., Storvold, R., Johnson, H., Haas, C., and Duguay, C.: Retrieval of physical snow properties from SAR observations at Ku- and X-band frequencies, Final Report, ESTEC contract, 20756(56), 07, 2008.
National Research Council: Earth Science and Applications from Space: National Imperatives for the Next Decade and Beyond, Washington, DC: The National Academies Press, https://doi.org/10.17226/11820, 2007.
Nearing, G. S., Crow, W. T., Thorp, K. R., Moran, M. S., Reichle, R. H., and Gupta, H. V.: Assimilating remote sensing observations of leaf area index and soil moisture for wheat yield estimates: An observing system simulation experiment, Water Resour. Res., 48, W05525, https://doi.org/10.1029/2011wr011420, 2012.
Niu, G. Y. and Yang, Z. L.: Effects of frozen soil on snowmelt runoff and soil water storage at a continental scale, J. Hydrometeorol., 7, 937–952, https://doi.org/10.1175/JHM538.1, 2006.
Niu, G. Y., Yang, Z. L., Mitchell, K. E., Chen, F., Ek, M. B., Barlage, M., Kumar, A., Manning, K., Niyogi, D., Rosero, E., Tewari, M., and Xia, Y.: The community Noah land surface model with multiparameterization options (Noah-MP): 1. Model description and evaluation with local-scale measurements, J. Geophys. Res.-Atmos., 116, D12109, https://doi.org/10.1029/2010JD015139, 2011.
Peters-Lidard, C. D., Houser, P. R., Tian, Y., Kumar, S. V., Geiger, J., Olden, S., Lighty, L., Doty, B., Dirmeyer, P., Adams, J., and Mitchell, K.: High-performance Earth system modeling with NASA/GSFC's Land Information System, Innovations in Systems and Software Engineering, 3, 157–165, 2007.
Pulliainen, J., Luojus, K., Derksen, C., Mudryk, L., Lemmetyinen, J., Salminen, M., Ikonen, J., Takala, M., Cohen, J., Smolander, T., and Norberg, J.: Patterns and trends of Northern Hemisphere snow mass from 1980 to 2018, Nature, 581, 294–298, 2020.
Raleigh, M. S., Lundquist, J. D., and Clark, M. P.: Exploring the impact of forcing error characteristics on physically based snow simulations within a global sensitivity analysis framework, Hydrol. Earth Syst. Sci., 19, 3153–3179, https://doi.org/10.5194/hess-19-3153-2015, 2015.
Reichle, R. H., McLaughlin, D. B., and Entekhabi, D.: Hydrologic data assimilation with the ensemble Kalman filter, Mon. Weather Rev., 130, 103–114, 2002.
Rott, H., Yueh, S. H., Cline, D. W., Duguay, C., Essery, R., Haas, C., Hélière, F., Kern, M., Macelloni, G., Malnes, E., Nagler, T., Pulliainen, J., Rebhan, H., and Thompson, A.: Cold regions hydrology high-resolution observatory for snow and cold land processes, Proc. IEEE, 98, 752–765, 2010.
Rutter, N., Essery, R., Pomeroy, J., Altimir, N., Andreadis, K., Baker, I., Barr, A., Bartlett, P., Boone, A., Deng, H., Douville, H., Dutra, E., Elder, K., Ellis, C., Feng, X., Gelfan, A., Goodbody, A., Gusev, Y., Gustafsson, D., Hellström, R., Hirabayashi, Y., Hirota, T., Jonas, T., Koren, V., Kuragina, A., Lettenmaier, D., Li, W.-P., Luce, C., Martin, E., Nasonova, O., Pumpanen, J., Pyles, R. D., Samuelsson, P., Sandells, M., Schädler, G., Shmakin, A., Smirnova, T. G., Stähli, M., Stöckli, R., Strasser, U., Su, H., Suzuki, K., Takata, K., Tanaka, K., Thompson, E., Vesala, T., Viterbo, P., Wiltshire, A., Xia, K., Xue, Y., and Yamazaki, T.: Evaluation of forest snow processes models (SnowMIP2), J. Geophys. Res.-Atmos., 114, D06111, https://doi.org/10.1029/2008JD011063, 2009.
Rutter, N., Sandells, M. J., Derksen, C., King, J., Toose, P., Wake, L., Watts, T., Essery, R., Roy, A., Royer, A., Marsh, P., Larsen, C., and Sturm, M.: Effect of snow microstructure variability on Ku-band radar snow water equivalent retrievals, The Cryosphere, 13, 3045–3059, https://doi.org/10.5194/tc-13-3045-2019, 2019.
Santi, E., De Gregorio, L., Pettinato, S., Cuozzo, G., Jacob, A., Notarnicola, C., Günther, D., Strasser, U., Cigna, F., Tapete, D., and Paloscia, S.: On the Use of COSMO-SkyMed X-Band SAR for Estimating Snow Water Equivalent in Alpine Areas: A Retrieval Approach Based on Machine Learning and Snow Models, IEEE T. Geosci. Remote, 60, 1–19, https://doi.org/10.1109/TGRS.2022.3191409, 2022.
Schmucki, E., Marty, C., Fierz, C., and Lehning, M.: Evaluation of modelled snow depth and snow water equivalent at three contrasting sites in Switzerland using SNOWPACK simulations driven by different meteorological data input, Cold Reg. Sci. Technol., 99, 27–37, https://doi.org/10.1016/j.coldregions.2013.12.004, 2014.
Sturm, M. and Liston, G. E.: Revisiting the Global Seasonal Snow Classification: An Updated Dataset for Earth System Applications, J. Hydrometeorol., 22, 2917–2938, https://doi.org/10.1175/jhm-d-21-0070.1, 2021.
Sturm, M., Goldstein, M. A., and Parr, C.: Water and life from snow: A trillion dollar science question, Water Resour. Res., 53, 3534–3544, 2017.
Takala, M., Luojus, K., Pulliainen, J., Derksen, C., Lemmetyinen, J., Kärnä, J. P., Koskinen, J., and Bojkov, B.: Estimating Northern Hemisphere snow water equivalent for climate research through assimilation of spaceborne radiometer data and groundbased measurements, Remote Sens. Environ., 115, 3517–3529, 2011.
Tsang, L., Durand, M., Derksen, C., Barros, A. P., Kang, D.-H., Lievens, H., Marshall, H.-P., Zhu, J., Johnson, J., King, J., Lemmetyinen, J., Sandells, M., Rutter, N., Siqueira, P., Nolin, A., Osmanoglu, B., Vuyovich, C., Kim, E., Taylor, D., Merkouriadi, I., Brucker, L., Navari, M., Dumont, M., Kelly, R., Kim, R. S., Liao, T.-H., Borah, F., and Xu, X.: Review article: Global monitoring of snow water equivalent using high-frequency radar remote sensing, The Cryosphere, 16, 3531–3573, https://doi.org/10.5194/tc-16-3531-2022, 2022.
Tedesco, M., Kim, E. J., Gasiewski, A., Klein, M., and Stankov, B.: Analysis of multiscale radiometric data collected during the Cold Land Processes Experiment-1 (CLPX-1), Geophys. Res. Lett., 32, https://doi.org/10.1029/2005GL023006, 2005.
USGS Earth Resources Observation and Science (EROS) Center: Shuttle Radar Topography Mission (SRTM) 1 Arc-Second Global, [data set], https://doi.org/10.5066/F7PR7TFT, 2018.
Vander Jagt, B. J., Durand, M. T., Margulis, S. A., Kim, E. J., and Molotch, N. P.: The effect of spatial variability on the sensitivity of passive microwave measurements to snow water equivalent, Remote Sens. Environ., 136, 163–179, 2013.
Vuyovich, C. M., Jacobs, J. M., and Daly, S. F.: Comparison of passive microwave and modeled estimates of total watershed SWE in the continental United States, Water Resour. Res., 50, 9088–9102, https://doi.org/10.1002/2013WR014734, 2014.
Walker, A. and Goodison, B.: Discrimination of a wet snow cover using passive microwave satellite data, Ann. Glaciol., 17, 307–311, 1993.
Wrzesien, M. L., Kumar, S., Vuyovich, C., Gutmann, E. D., Kim, R. S., Forman, B. A., Durand, M., Raleigh, M. S., Webb, R., and Houser, P.: Development of a “nature run” for observing system simulation experiments (OSSEs) for snow mission development, J. Hydrometeorol., 23, 351–375, 2022.
Xia, Y., Mitchell, K., Ek, M., Sheffield, J., Cosgrove, B., Wood, E., Luo, L., Alonge, C., Wei, H., Meng, J., Livneh, B., Lettenmaier, D., Koren, V., Duan, Q., Mo, K., Fan, Y., and Mocko, D.: Continental-scale water and energy flux analysis and validation for the North American Land Data Assimilation System project phase 2 (NLDAS-2): 1. Intercomparison and application of model products, J. Geophys. Res., 117, D03109, https://doi.org/10.1029/2011JD016048, 2012.
Yang, Z.-L. and Dickinson, R. E.: Description of the BiosphereAtmosphere Transfer Scheme (BATS) for the soil moisture workshop and evaluation of its performance, Global Planet. Change, 13, 117–134, 1996.
Yang, Z. L., Niu, G. Y., Mitchell, K. E., Chen, F., Ek, M. B., Barlage, M., Longuevergne, L., Manning, K., Niyogi, D., Tewari, M., and Xia, Y.: The community Noah land surface model with multiparameterization options (Noah-MP): 2. Evaluation over global river basins, J. Geophys. Res.-Atmos., 116, D12, https://doi.org/10.1029/2010JD015139, 2011.
Yueh, S. H., Dinardo, S. J., Akgiray, A., West, R., Cline, D. W., and Elder, K.: Airborne Ku-Band Polarimetric Radar Remote Sensing of Terrestrial Snow Cover, IEEE T. Geosci. Remote, 47, 3347–3364, https://doi.org/10.1109/tgrs.2009.2022945, 2009.
Zeng, X., Broxton, P., and Dawson, N.: Snowpack change from 1982 to 2016 over conterminous United States, Geophys. Res. Lett., 45, 12940–12947, https://doi.org/10.1029/2018GL079621, 2018.
Zhu, J., Tan, S., King, J., Derksen, C., Lemmetyinen, J., and Tsang, L.: Forward and Inverse Radar Modeling of Terrestrial Snow Using SnowSAR Data, IEEE T. Geosci. Remote, 56, 7122–7132, https://doi.org/10.1109/TGRS.2018.2848642, 2018.