the Creative Commons Attribution 4.0 License.
the Creative Commons Attribution 4.0 License.
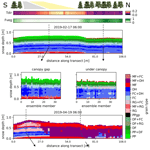
Exploring the potential of forest snow modeling at the tree and snowpack layer scale
Jari-Pekka Nousu
Vincent Vionnet
Tobias Jonas
Rafife Nheili
Matthieu Lafaysse
Boreal and sub-alpine forests host seasonal snow for multiple months per year; however, snow regimes in these environments are rapidly changing due to rising temperatures and forest disturbances. Accurate prediction of forest snow dynamics, relevant for ecohydrology, biogeochemistry, cryosphere, and climate sciences, requires process-based models. While snow schemes that track the microstructure of individual snow layers have been proposed for avalanche research, so far, tree-scale processes resolving canopy representations only exist in a few snow-hydrological models. A framework that enables layer- and microstructure-resolving forest snow simulations at the meter scale is lacking to date. To fill this research gap, this study introduces the forest snow modeling framework FSMCRO, which combines two detailed, state-of-the art model components: the canopy representation from the Flexible Snow Model (FSM2) and the snowpack representation of the Crocus ensemble model system (ESCROC). We apply FSMCRO to discontinuous forests at boreal and sub-alpine sites to showcase how tree-scale forest snow processes affect layer-scale snowpack properties. Simulations at contrasting locations reveal marked differences in stratigraphy throughout the winter. These arise due to different prevailing processes at under-canopy versus gap locations and due to variability in snow metamorphism dictated by a spatially variable snowpack energy balance. Ensemble simulations allow us to assess the robustness and uncertainties of simulated stratigraphy. Spatially explicit simulations unravel the dependencies of snowpack properties on canopy structure at a previously unfeasible level of detail. Our findings thus demonstrate how hyper-resolution forest snow simulations can complement observational approaches to improve our understanding of forest snow dynamics, highlighting the potential of such models as research tools in interdisciplinary studies.
- Article
(12114 KB) - Full-text XML
-
Supplement
(2002 KB) - BibTeX
- EndNote
Seasonal snow takes many roles in the Earth's systems. As part of the land surface, it acts as reflective and insulating material, substantially influencing the Earth's energy budget (e.g., Thackeray and Fletcher, 2016; Colman, 2013; Sturm et al., 1997). Snow further constitutes an important water storage, shaping the hydrograph of snow-dominated catchments (e.g., Barnhart et al., 2016; Bales et al., 2006; Viviroli and Weingartner, 2004). Snow is also a crucial ecosystem and habitat component, affecting animal movement, food accessibility, and soil thermodynamics and biogeochemistry (e.g., Boelman et al., 2019; Gilbert et al., 2017; Stark et al., 2020; Zhang et al., 2018). Lastly, snow avalanches are a common natural hazard in many mountain regions (Schweizer et al., 2003). As the seasonal snow of the Northern Hemisphere often occurs in forested areas (Rutter et al., 2009; Kim et al., 2017), the dynamics of the forest snow cover are relevant in any of these contexts, which is why process-based (or physics-based) models applied across disciplines need to accurately capture the processes that shape forest snow cover evolution. Today, both seasonal snow regimes and forest structure are subject to rapid change (e.g., Notarnicola, 2020; Mote et al., 2018; Goeking and Tarboton, 2020; Seidl et al., 2017). Process-based models are our best available tool for predicting the evolution of forested snow-covered environments under unprecedented conditions, enabling us to assess the impacts of ongoing change.
Plenty of process-based models have evolved in the context of different research disciplines, with widely varying complexity largely determined by the intended application (e.g., Etchevers et al., 2004; Essery et al., 2009; Krinner et al., 2018). For instance, snow cover presence or absence is the key variable of interest for land surface models that primarily target the accurate simulation of land–atmosphere energy exchanges, hydrological models require the snow water equivalent to be well quantified, and avalanche risk estimates rely on information on the microstructure of individual snowpack layers. Consequently, snowpack and canopy representations in hydrological, atmospheric, and land surface models feature a broad range of model structures, where the capability to explicitly represent more processes, and in a more detailed manner, is usually linked to higher model complexity.
Snowpack properties and internal processes can exhibit strong vertical variability arising from the history of individual snow layers. Resolving the physical properties of individual layers requires complex, multi-layer snow physics models capable of prognostically tracking the evolution of snow's microstructural and thermal states, including variables such as temperature, liquid water content, density, and snow microstructure descriptors. The development of such models has traditionally been driven by avalanche research (e.g., Morin et al., 2020; Bartelt and Lehning, 2002; Vionnet et al., 2012). More recently, these models have found further use in the remote sensing community due to the need for a priori knowledge on snow physical properties when interpreting electromagnetic signals (e.g., Picard et al., 2018; Kontu et al., 2017; Picard et al., 2022). In contrast, the use of detailed snowpack schemes to study the snowpack's influence on terrestrial processes is less common. Applications include permafrost (Barrere et al., 2017; Gouttevin et al., 2018) and shrub tundra thermal regimes (Domine et al., 2016) but only rarely include ecological research (Saccone et al., 2013; Domine et al., 2018; Ouellet et al., 2017) and forest areas (Rasmus et al., 2016). When applied to forests, these models are usually coupled to big-leaf canopy representations intended for coarse-resolution simulations (Gouttevin et al., 2018; Nousu et al., 2024), which hampers our understanding of the interactions between canopy and snowpack layering at small spatial scales.
Indeed, the canopy's impact on snowpack mass and energy fluxes is dictated by tree-scale processes and is thus highly variable in space, creating strong horizontal snowpack heterogeneity (Safa et al., 2021; Trujillo et al., 2007; Mazzotti et al., 2019). In recent years, approaches to explicitly resolve this variability have been brought forward by the snow-hydrological community to meet the need for accurate snowmelt estimates from forested watersheds for downstream water provision (Bales et al., 2006) and for an improved understanding of the role of canopy gaps in snow cover retention (Ellis et al., 2013; Broxton et al., 2020) and to inform forest management practices in support of sustainable water management (Krogh et al., 2020). Leveraging the opportunities offered by novel observational systems and increasingly detailed canopy structure datasets, these efforts have led to hyper-resolution models that can explicitly resolve canopy structure variability at the meter scale (Broxton et al., 2015; Mazzotti et al., 2020a, b). Current implementations and applications range from case studies at the scale of few square kilometers (km2) (Mazzotti et al., 2023) to entire catchments spanning hundreds of square kilometers (km2) (Lewis et al., 2023; Moeser et al., 2020) to operational modeling at the national scale (Mott et al., 2023). In these cases, however, the detailed canopy representations are coupled to intermediate-complexity snow schemes that represent a few layers only without a parameterization of microstructure.
A modeling system that combines the most complex representations of both the snow and canopy is lacking to date. At the same time, a few observational studies have provided evidence that tree-scale processes do impact layer-scale snowpack properties (Bouchard et al., 2022; Teich et al., 2019; Molotch et al., 2016). These observations document, for example, different microstructural properties under trees, in the unloading zone, and in canopy gaps. Increased accuracy in representing how such small-scale canopy processes impact snowpack features would enable new forest snow model applications. For instance, it remains unexplored how snowpack heterogeneity and forest structure interact to shape ecologically relevant snow properties and resultant exchange processes between soil, snow, and the atmosphere (Lembrechts et al., 2019; Bramer et al., 2018; Martz et al., 2016). Assessing the influence of forest management strategies on snowpack layering, as well as cascading impacts on ecology and biogeochemistry, would require such a complex model.
To fill this research gap, here, we combine tree-scale canopy structure and layer-scale physics-based snowpack representations into one modeling framework. Our system merges the capabilities of the canopy implementation of the Flexible Snow Model, FSM2 (Mazzotti et al., 2020a, b), and the Ensemble System Crocus snowpack model, ESCROC (Lafaysse et al., 2017), creating a framework that (1) extends current applications of layer- and microstructure-resolving snowpack modeling to heterogeneous forest environments, (2) enhances tree-scale process-resolving forest snow simulations through the capability to represent snow microstructure at increased vertical resolution, and (3) includes a notion of uncertainties associated with the snowpack representation in terms of an ensemble. As a proof of concept, we then apply the model to boreal and sub-alpine forests, providing first evidence on how the spatiotemporal dynamics of forest snow processes translate to snowpack properties. The specific goals of our study are thus (1) to introduce the modeling system; (2) to present a first scientific application, showcasing the model's potential as a research tool; and (3) to identify potential model improvements and motivate possible ways forward. Ultimately, we hope to inspire and provide guidance for new research and applications using novel hyper-resolution forest snow modeling tools.
This paper is structured around our objectives as follows: in the Methods section, we introduce the modeling framework and present the sites at which it was tested. In the Results section, we first assess the plausibility of our simulations and then present ways to analyze the wealth of information contained in the simulations, aimed at exploring canopy structure impacts on internal snowpack properties. Based on these results, we finally discuss the system, our findings, and potential future developments and applications.
2.1 Modeling framework
To enable simulations that resolve canopy–snow interactions at the scale of individual trees and internal snowpack processes at the scale of individual physical layers, the modeling framework used in this study combines elements of two state-of-the-art models: Crocus and FSM2. The choice of these two models was motivated by their individual capabilities and their current widespread use in both operational and research applications, and it leveraged the authors' involvement in their developments. The two models and their integration into a single framework are outlined in the following.
2.1.1 The Crocus snowpack model and ESCROC ensemble system
The Crocus snowpack model (Brun et al., 1989, 1992) is a physics-based snow model of high complexity originally developed in the context of avalanche hazard forecasting. It represents snowpack layering with a Lagrangian, dynamic discretization; i.e., one or several snow layers are created upon a snow precipitation event, but these can be merged once they attain sufficiently similar physical properties. The model represents the major internal snowpack processes, including heat diffusion, compaction, liquid water transport, and snow metamorphism. Each layer is characterized by the state variables of depth, density, liquid water content, temperature, age, and microstructure descriptors. Snow type can be diagnosed from these snow microstructure variables. Their evolution in time depends on temperature, temperature gradients, and liquid water content (Morin et al., 2013), which evolve depending on surface energy and mass balance terms computed by the model and parameterizations of the physical properties of fresh snow.
Crocus is integrated in the ISBA land surface model within the SURFEX system (Masson et al., 2013) and is therefore coupled to a soil scheme (Decharme et al., 2011). A detailed description of the Crocus snow scheme was provided by Vionnet et al. (2012). Since then, there have been several updates and enhancements to the model (Carmagnola et al., 2014; Tuzet et al., 2017). Of particular interest to this study, Lafaysse et al. (2017) extended Crocus by means of additional parameterizations for a variety of snow processes to yield a multi-physics ensemble modeling framework (ESCROC, Ensemble System Crocus). The ensemble spread provides an estimate of model uncertainty arising from uncertainty in the surface and internal snow process parameterizations. The ESCROC ensemble was evaluated in a large range of environments and climatic conditions (Lafaysse, 2023) and is thus expected to represent model uncertainty well, even when near-surface atmospheric conditions are modified to account for the effect of the canopy, as done in this study.
Crocus has seen widespread usage and continued development for both research purposes (Dumont et al., 2020; Di Mauro et al., 2019; Spandre et al., 2019) and operational use at Météo-France (Le Moigne et al., 2020; Vernay et al., 2022). In response to repeated interest from the snow modeling community with regard to incorporating elements of Crocus into other modeling systems, e.g., CryoGrid (Zweigel et al., 2021) and WRF-Hydro (Eidhammer et al., 2021), or incorporating the whole code, as in MAR (Gallée et al., 2001; Navari et al., 2021), a standalone version of Crocus was recently established (see “Code and data availability” section). It allows for Crocus to be more easily implemented within existing land surface models, which has, to date, been achieved in the Canadian land surface model SVS2 (Vionnet et al., 2022; Woolley et al., 2024).
Within SURFEX, Crocus can be coupled to an explicit canopy representation for forest simulations. MEB (multi-energy balance, Boone et al., 2017), the corresponding scheme, follows a big-leaf approach, where canopy structure is characterized by specifying vegetation class, leaf area index (LAI), and canopy height. Due to intricate dependencies with other components of SURFEX, including MEB was beyond the scope of the standalone Crocus version. For the same reason, adapting MEB to represent tree-scale processes is nontrivial. So far, the use of Crocus-MEB is limited to a few site-scale studies (Vincent et al., 2018; Nousu et al., 2024).
2.1.2 The Flexible Snow Model (FSM2) and hyper-resolution canopy representation
The Flexible Snow Model, FSM2 (Mazzotti et al., 2020a, b), is an intermediate-complexity snow model that has evolved from the Factorial Snow Model, FSM (Essery, 2015), and that has been adapted for high-resolution (meter-scale) simulations in forested areas. Originally, the FSM was developed to investigate the performance of snow schemes used in land surface models and aimed to provide a platform that would allow easy integration and testing of alternative snow properties and process parameterizations (Essery et al., 2013). The snowpack in FSM/FSM2 is thus represented with a few layers only (three by default), and the aim is not to capture physical layers. The FSM2 canopy structure is represented as one model layer, which is coupled to the snowpack (surface) via a canopy air space, and the representation of canopy–snow interactions is based on established parameterizations. However, radiation transfer through the local three-dimensional canopy structure may be explicitly resolved by an external radiation transfer model (such as HPEval, Jonas et al., 2020) associated with FSM hyper-resolution runs.
Mazzotti et al. (2020b) presented a version specifically intended for the simulation of forest snow cover at spatial resolutions of just a few meters (FSM2.0.3, hereafter referred to as FSM2 for simplicity). For a detailed description, we refer to Mazzotti et al. (2020a, b) and Mazzotti et al. (2023). The main difference between this hyper-resolution version and the default canopy representation is that it uses a diverse set of process-specific canopy structure descriptors, allowing different processes to be affected by different and potentially uncorrelated local canopy features (e.g., a forest gap can experience, at the same time, little interception but frequent shading). Canopy descriptors computed at each modeled location capture the location's structural diversity with horizontal, vertical, local, and stand-scale metrics. As stated above, transmission of direct shortwave radiation through the canopy, which is dictated by the presence of canopy elements in the path of the solar beam and is thus highly variable in time and space, is not parameterized. Instead, FSM2 accepts transmissivity time series as model input, which can be obtained from any external radiative transfer model. In doing so, FSM2 maintains a simple model structure while leveraging the accuracy of radiative transfer models that resolve canopy shortwave radiation transmission explicitly.
This FSM2 version with enhanced canopy representation has been shown to replicate spatiotemporal forest snow distribution patterns well at boreal and sub-alpine sites (Mazzotti et al., 2023, 2020b), which makes it an adequate choice for this study. The model has so far been used for research purposes (Mazzotti et al., 2023) and as the starting point for the development of intermediate-resolution modeling strategies (Mazzotti et al., 2021b) that are implemented today in the modeling framework of Switzerland's operational snow-hydrological service (Mott et al., 2023).
Because FSM2 was developed as a snow research model and was not designed to be coupled with atmospheric models over all kinds of land surfaces, its code is much lighter than that of a typical land surface model (such as SURFEX). Both the canopy and the soil representations comprise only the state variables that are relevant to their interactions with the snowpack. The simplicity of the code, however, makes installation and usage relatively straightforward. New model developers can familiarize themselves with the entire code rather quickly, and model enhancements are generally easier to implement than in a typical land surface model. This is certainly a reason why FSM/FSM2 has become a popular model tool in snow (hydrology) research (e.g., Magnusson et al., 2019; Smyth et al., 2022; Alonso-González et al., 2022; Rutter et al., 2023).
2.1.3 FSMCRO: integration of the FSM2 canopy and ESCROC snow representations
Technically, the integration of the two models was achieved by incorporating the Crocus snowpack scheme into the FSM2 code base as an alternative snow scheme, which is in line with the purpose of the standalone Crocus version. The integration was facilitated by the fact that both models are coded in Fortran and that driving data required by Crocus largely correspond to that used by FSM2 (i.e., including snow and rainfall rates, incoming shortwave and longwave radiation fluxes, air temperature and pressure, relative humidity, and wind speed). As a further advantage, this implementation preserved the simple structure of the FSM2 code. The current implementation also enables version tracking of both model components separately, which will facilitate continued development in the future and avoid long-term code divergence between the different implementations of Crocus in SURFEX, FSM2, and SVS2.
In most land surface models that represent canopy and snowpack as separate model layers, numerical coupling entails the sequential computation of, first, the canopy energy balance, including the energy fluxes to the snowpack, and then heat diffusion through the snowpack, with the previously computed energy fluxes as the boundary condition (e.g., Boone et al., 2017; Lawrence et al., 2019). Snow surface temperature affects (and is potentially updated during) both steps, which can lead to numerical inconsistencies and instabilities if solving of the two steps occurs separately. While both FSM2 and MEB-Crocus have this structure, their approach to avoid numerical instabilities differs (see Essery, 2015, for FSM, as well as Appendix I in Boone et al., 2017, for MEB-Crocus). Furthermore, it must be noted that the consideration of snow surface temperature in the coupling between snow surface fluxes and the internal snowpack energy budget (Fourteau et al., 2024) does not follow the same approach between FSM/FSM2 (skin temperature) and Crocus (first-layer temperature). Initial tests using both the skin and the first-layer approaches revealed that direct coupling of the FSM2 canopy implementation with Crocus was prone to instabilities, particularly in the case of very thin snow surface layers.
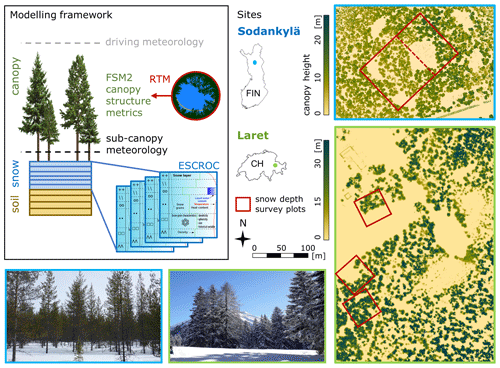
Figure 1Overview of the FSMCRO modeling framework and its components, as well as of the Sodankylä (blue) and Laret (green) sites, including their location in Finland and Switzerland, the canopy height model, the location of the snow depth survey plots, and site pictures. Parts of this figure are adapted from Mazzotti et al. (2021a).
To circumvent the issue and to enable the use of Crocus without further modification, we opted for a “zero-layer” approach, in which FSM2 provides sub-canopy forcings but remains uncoupled to Crocus. Rather than using the FSM2 sub-routine that solves the canopy energy balance and computes energy fluxes at the snow surface, we implemented meteorological transfer functions by means of which the above-canopy meteorological data are modified to represent sub-canopy meteorological states, as illustrated in the schematic in Fig. 1. These sub-canopy meteorological states are then fed to Crocus, which is thus applied in the same way as for an open site.
Modifications applied to the above-canopy meteorological data are based on the forest snow process implementations in FSM2, the main goal being an accurate representation of the spatiotemporal variability of sub-canopy micrometeorological conditions as dictated by canopy structure across spatial scales of just a few meters. This also involves the treatment of canopy snow interception and its subsequent depletion as modifications to the precipitation input. Although this approach sacrifices some features of an explicit and coupled canopy, it maintains the main conceptual assets of FSM2 in terms of the inclusion of detailed, process-specific canopy structure metrics and time-varying transmissivity for direct shortwave radiation. Consequently, it accounts for the different spatiotemporal patterns of the different meteorological variables which are at the core of the FSM2 canopy implementation. The equations used are reported in Appendix A1, and Table A1 therein summarizes where and how the treatment of these canopy processes differs from the original implementation of FSM2. The advantages and limitations of this implementation are discussed in Sect. 4.1.
2.2 Model application: sites, datasets, and simulations
For a first application of FSMCRO, we leverage datasets from the two forest sites described in Mazzotti et al. (2020b) and (2021a), which include a boreal and a sub-alpine site, and used in the context of FSM2 development. The following sections describe the sites and the data sources and provide an overview of the simulations. The site locations and canopy structures are shown in Fig. 1. The use of published datasets (Mazzotti et al., 2020c, 2021b) allowed us to focus on the novelties of FSMCRO and ensured comparability with results obtained with earlier FSM2 simulations.
2.2.1 Sodankylä, Finland
As an example of a sub-arctic boreal forest site, we applied the model to forest locations within the boundaries of the Finnish Meteorological Institute (FMI) Arctic Research Centre at Sodankylä, located at 67°22′ N, 26°38′ E and 179 m a.s.l. During a field campaign in April 2019, spatially distributed snow depth observations at ∼2 m spacing along forest transects were conducted on a 120×80 m area in a discontinuous Scots pine forest. Mazzotti et al. (2020b) used the snow depth observations to validate snow distribution patterns simulated by FSM2. Hemispherical images co-registered with snow depth observations were used to obtain sky-view fraction time series of canopy transmissivity for direct shortwave radiation at each surveyed location by applying the radiative transfer model HPEval (Jonas et al., 2020). All other necessary canopy structure metrics were derived from a canopy height model at 1 m resolution, which was based on terrestrial laser-scanning data acquired during the same campaign. All meteorological forcings necessary to drive FSM2 were measured by an automatic weather station on site and were assembled by Mazzotti et al. (2020b).
2.2.2 Laret, Switzerland
Our sub-alpine forest site is located at Laret, (46°50′ N, 9°52′ E and 1520 m a.s.l.) at 4 km distance from the WSL Institute for Snow and Avalanche Research SLF in Davos. We used snow depth observations acquired during peak accumulation and throughout the snowmelt phase of the water year 2019 from three 50 m × 50 m plots, along north- and south-exposed edges (i.e., shaded and sun-exposed) and within the closed canopy of a Norway spruce forest, with a setup equivalent to that of the Sodankylä data. Likewise, canopy structure metrics and time series of transmissivity for direct shortwave radiation were derived from a canopy height model at 1 m resolution (based on a helicopter-borne lidar acquisition in 2010) and from co-registered hemispherical images subsequently analyzed with HPEval. Meteorological forcing data were available from an on-site automatic weather station operated by SLF; further detail is provided by Mazzotti et al. (2020b).
To complement the point locations of the manual measurements, we further consider the full extent of the 250 m × 400 m area of discontinuous forest at Laret shown in Fig. 1 (canopy height model), which is located within the study domain of Mazzotti et al. (2021a). In their study, they derived canopy structure input for FSM2 at 2 m spacing to enable fully distributed simulations at 2 m resolution. This included calculation of synthetic hemispherical images for the subsequent creation of datasets of time-varying transmissivity for direct shortwave radiation and sky-view fraction based on the methodology presented by Webster et al. (2020). These datasets were leveraged in this study to achieve fully distributed FSMCRO simulations.
2.2.3 Overview of FSMCRO simulations
Multiple sets of FSMCRO simulations were considered in this study:
-
First, we considered deterministic point simulations performed at the snow survey sites from Mazzotti et al. (2020b), designed to capture a broad range of forest structures, including sites in both Sodankylä and Laret. These simulations served to assess whether the snow distribution captured by FSMCRO was consistent with manual snow depth observations and were used for a general assessment of differences in simulated snow stratigraphy at locations with varying canopy structure.
-
Next, we considered ensemble simulations at a subset of the snow survey sites, including points along a ∼100 m transect across a forest gap within the Sodankylä site. These runs were intended to assess the robustness of simulated differences between locations with contrasting canopy structures compared to the uncertainties of the snow model parameterizations.
-
We considered spatially explicit, deterministic simulations over a 250 m × 400 m area in Laret at 2 m spatial resolution, aimed at showcasing the fully resolved spatiotemporal variability of snowpack properties and their link to canopy structure.
-
Finally, we considered ensemble simulations at a point featuring canopy properties that represent a spatial average of the spatially explicit model domain in Laret, aimed at contrasting the fully resolved variability captured by the spatially explicit simulation with the ensemble spread at the point with average forest properties.
The deterministic simulation applies the Crocus options currently used operationally at Météo-France. The ensemble used here comprises 35 members and is based on the E2 ensemble introduced in Lafaysse et al. (2017). Merely, the snow metamorphism options based on Flanner and Zender (2006) are not yet available in the standalone version of Crocus and were replaced by an improved metamorphism parameterization recently developed at Météo-France (“B21”, unpublished but used, e.g., in Dick et al., 2023). The Crocus options used for these runs are listed in Appendix A2 in Table A2.
All simulations were run from 1 October 2018 to 31 May 2019, corresponding to the period covered by the meteorological forcing assembled by Mazzotti et al. (2020b), with a temporal resolution of 1 h. The model was initialized with homogeneous soil temperature conditions (FSM2 default: 285 K) and no snow.
Simulations obtained with FSMCRO cover five dimensions: (1) different snowpack properties, (2) their vertical distribution within the snow profile, (3) their horizontal variability across modeled locations, (4) their evolution in time, and (5) their uncertainty as captured by the spread of the ensemble. The following sections present some ideas on how this wealth of information can be exploited, depending on what dimensions are of primary interest to the analysis. Following some plausibility considerations, we assess the different snow states and the seasonal evolution of their vertical profiles at contrasting locations in a discontinuous forest. We then contrast this variability to associated model uncertainties and showcase how FSMCRO can be applied to estimate sub-grid variability of snow properties. While by no means exhaustive, these results serve to highlight the potential usage of these simulations and to inspire future work on and with the modeling system.
3.1 Plausibility considerations
Figure 2 shows snow depth distributions observed at three contrasting forest plots in Laret during a spring survey on 17 April 2019, including a plot within-stand (upper row), a shaded north-exposed forest edge (middle row), and a sunny south-exposed forest edge (lower row). These plots cover a broad range of canopy densities and insolation regimes, examples of which are show by the hemispherical images displayed next to each plot's snow depth data. Consequently, within-plot snow depth variability is large, and snow distribution patterns differ markedly between plots. Observations (right column) are compared to simulations obtained with both FSMCRO (left) and FSM2 (middle), following the analysis presented in Mazzotti et al. (2020b); see Fig. 9 therein. Overall, the snow depth patterns resulting from the FSMCRO simulations are consistent with the observations and are comparable to the results obtained with FSM2, although FSMCRO appears to simulate slightly larger snow depths than FSM2 (mean bias: 0.29 and 0.21 m, respectively). Additional validations including error metrics and comparisons for a mid-winter survey at the same sites, as well as for a spring survey at Sodankylä, are provided in Sect. S1 in the Supplement. While a more detailed model validation is beyond the scope of this study, an adequate reproduction of observed snow depth patterns is a prerequisite for a meaningful subsequent analysis of snowpack vertical properties. In this regard, Fig. 2 attests to the satisfactory performance of FSMCRO at Laret, which is underpinned by consistent coefficients of variation (FSMCRO: 0.12; FSM2: 0.17; observations: 0.19). Spatially distributed datasets that would allow us to validate FSMCRO simulations at the scale of individual snowpack layers do not exist to date at this site. Noteworthily, FMI's long-term snow-monitoring program has included weekly snow pits in the forest (Leppänen et al., 2016) since 2019; exemplary comparisons of these datasets to FSMCRO simulations are presented and discussed in the Supplement in Sect. S2 for interested readers.
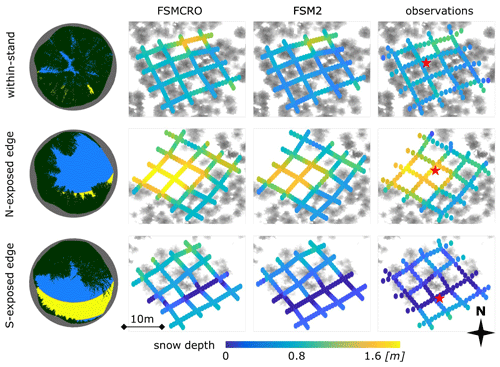
Figure 2Snow distribution observed on 17 April 2019 at three 50 m × 50 m forest plots in Laret and simulated with FSMCRO and FSM2; data are from Mazzotti et al. (2020b). Hemispherical images taken at the position of the red star are shown for each site, including canopy structure (gray and black), terrain (gray), sky (blue), and the solar tracks between 1 October and 17 April (yellow). These images exemplify the characteristics of the contrasting within-stand, north-exposed, and south-exposed canopy edge locations.
3.2 Seasonal evolution of snowpack profiles at gap vs. under-canopy locations
An overview of snow properties accessible through FSMCRO simulations is given in Fig. 3, contrasting closed-canopy and gap locations (upper vs. lower rows) in Sodankylä (blue frame) and Laret (green), respectively. The evolution of snow profiles is shown for the specific surface area (SSA), grain type, temperature, and density of snow. This is the standard way of visualizing layer-scale properties and mainly serves as a qualitative comparison of different locations for the entire winter; nevertheless, some impacts of the presence of canopy on both accumulation and ablation processes are visible at both sites. The most prominent difference is the snow depth variability created by differences in interception during accumulation and differences in melt rates, resulting in similar melt-out dates at under-canopy and gap locations despite more snow accumulating in the canopy gaps. A closer look at the snow stratigraphy reveals specific snowpack features that are present in under-canopy locations but not in gaps and vice versa. At Sodankylä, for instance, the formation of surface melt forms and/or crusts (red) happens ca. 10 d earlier in under-canopy locations than in the canopy gap (around 15 vs. 25 February), but the snowpack in the canopy gap features a much thicker bottom layer consisting of melt forms throughout winter. At Laret, such examples include a layer of depth hoar and faceted crystals (light blue) formed close to the surface in January that persists longer in the canopy gap than under the canopy. Later in the season (mid-February), a surface melt crust (clearly visible yellow layer in the density profile) develops in the canopy gap but not under the closed canopy. Beyond the within-site comparison, it should further be noted that FSMCRO simulates substantially different stratigraphy in the two climates, as expected (Sturm and Liston, 2021).
3.3 Horizontal variability of snowpack stratigraphy along a forest discontinuity at different points in time
Results presented in Sect. 3.2 (Fig. 3) provide evidence that, at any given point in time, the spatial variability in the canopy may translate to variability in layer-scale snowpack properties. To explore this more systematically, here, we take a closer look at the spatial variability in snow stratigraphy along a transect through discontinuous forest, focusing our analysis on the examples of grain type and SSA. While still considering the vertical dimension, we now also fully resolve the horizontal dimension, which allows us to capture the complex influence of canopy edges as well. Replicating the concept of a long snow trench, Fig. 4 presents temporal snapshots of snowpack stratigraphy, as characterized by grain type, along a ∼100 m transect in Sodankylä over the course of the season. The transect crosses a large forest gap and thus encompasses (from left to right) a shady north-exposed canopy edge, the canopy gap, a sunny south-exposed canopy edge, and the closed canopy (see dashed line in the canopy height model in Fig. 1). Canopy structure is visualized at the top of the figure, both quantitatively by local canopy cover fraction (Fveg) and conceptually (note the relative positions of the sun to the trees). The five selected time steps cover a period of 3 months (mid-February to mid-May), approximately centered around peak winter accumulation (end of March), and thus include scenes during both accumulation and ablation periods. They were chosen to capture typical situations within the snow season: (1) melt crust formation in the forest following a snowfall event (17 February), (2) subsequent burial of the crust by a snowfall event and its survival within the snowpack (12 March), (3) the onset of snowpack ripening following peak snow water equivalent (SWE) (3 April), (4) the vertical progression of the melting front and associated disappearance of dry-snow grain types (19 April), and (5) the onset of melt-out on the transect (10 May). The same temporal snapshots, but showing SSA as an example of a quantitative snowpack property, are provided in Fig. 5.
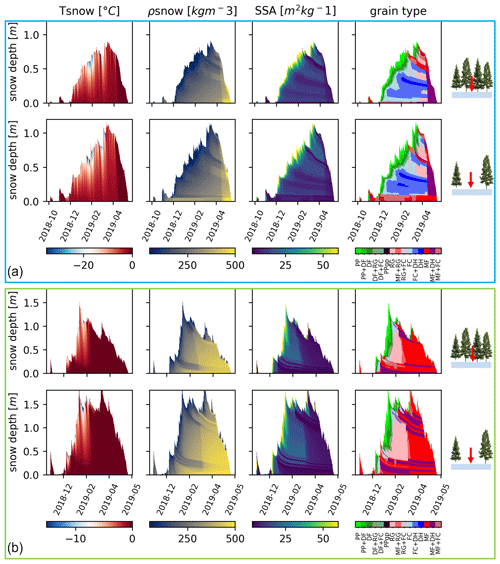
Figure 3Simulated snow profiles at contrasting within-forest locations at Sodankylä (blue frame) and Laret (green frame) including points in under-canopy locations (a) and in forest gaps (b); see icons right of the plots. Profiles are shown for snow temperature (first column), snow density (second), snow specific surface area (third), and snow grain type (fourth). Grain type abbreviations correspond to the international classification for seasonal snow on the ground (Fierz et al., 2009) and are reported in Appendix A3.
Several noteworthy features are revealed by these plots. Differences between the closed canopy and canopy gaps mentioned in Sect. 3.2 are confirmed and especially pronounced during accumulation: the surface melt crust visible on 17 February is consistently present at under-canopy locations but absent in the canopy gap and likely results from drip unloading of intercepted snow at relatively warm air temperatures. Snow depth variability corresponding to the distribution of Fveg develops during accumulation due to interception-related processes and remains the prominent pattern until around peak winter accumulation. However, later in the season, different variability patterns along the transect evolve due to the variability in pathways of snow metamorphism, which arises from spatial differences in the snow energy balance. This becomes especially visible in spring when the snowpack starts to ripen (3 to 19 April). Melt forms appear much earlier at the south-exposed edge of the gap than at the north-exposed edge. We also find that ripening is completed at the sun-exposed edge first, while other locations still feature dry-snow grain types (19 April). Overall, these results highlight that gradients in snow properties caused by a heterogeneous canopy are highly dynamic in time. They further suggest that vertical snowpack heterogeneity generally outweighs horizontal variability during accumulation but that the opposite may become the case during ablation, when vertical stratigraphy at individual locations becomes more homogeneous while horizontal differences between locations become more pronounced. Spatiotemporal patterns of SSA in Fig. 5 underpin this tendency and show that the variability suggested by the discrete snow grain type classification is associated with a remarkable variability of this continuous snow microstructure descriptor. For the sake of completeness, the same figures for snow temperatures, density, liquid water content, and ram resistance (as a proxy for snow hardness; see Fierz et al., 2009) are included in the Supplement in Sect. S3. They reveal that the spatiotemporal variability along the transect is considerable for all these snow state variables and diagnostics.
Ensemble simulations provide a means to assess whether snowpack properties simulated at locations with specific canopy features are robust. Consequently, considering results from the full ensemble at locations that feature contrasting canopy structures reveals whether snowpack variability induced by a heterogeneous canopy is greater than snow model uncertainty. Examples of such comparisons are shown in Fig. 6 and include two of the time steps shown in Figs. 4 and 5 (accumulation vs. ablation). On 17 February, profiles simulated with the full ensemble are shown for a characteristic location within the canopy gap and below the closed canopy. All ensemble members exhibit a surface melt crust and only a thin bottom layer, with melt forms at the location in the closed canopy. A surface crust is consistently lacking at the canopy gap location across the ensemble, whereas a thick bottom layer with melt forms is present in all members. In contrast, snow depth variability between ensemble members at each location is of the same order of magnitude as snow depth differences between the two locations. This implies that modeled structural differences are more robust than simulated snow depth differences because all ensemble members agree on the structural differences, while the difference in snow depth between the two locations is within the uncertainty represented by the ensemble at each location.
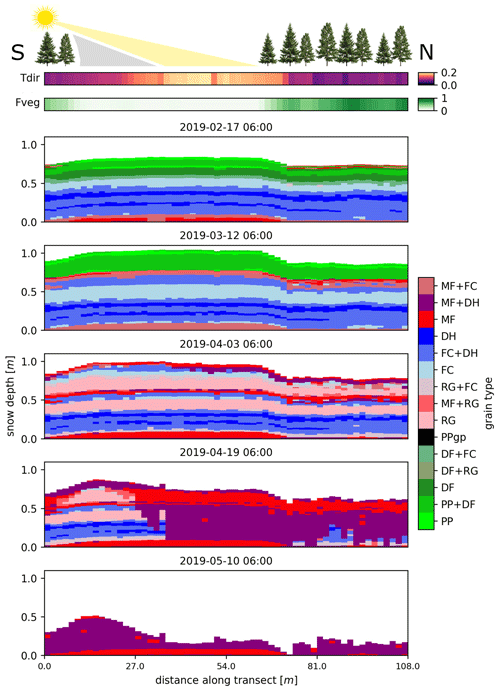
Figure 4Snow stratigraphy along a discontinuous forest transect at Sodankylä, visualized in terms of snow grain type for five different dates covering the 3-month period between mid-February and mid-May. Canopy structure along the transect is visualized in terms of local canopy cover fraction, Fveg, and direct-beam transmissivity averaged over the period shown (Tdir).
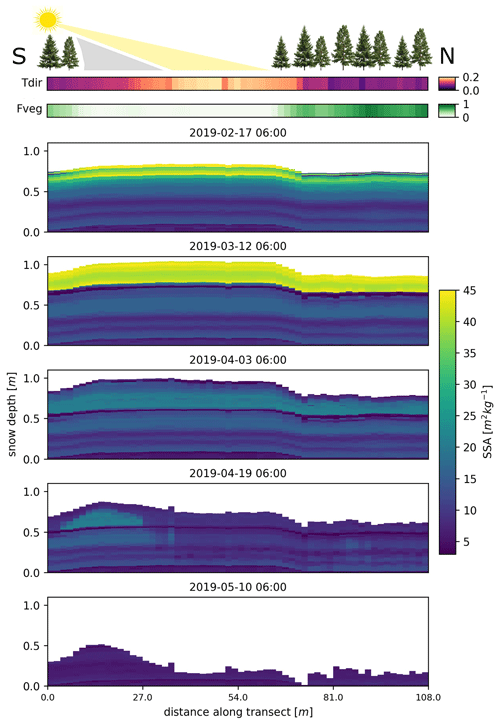
Figure 5Snow specific surface area at the layer scale along a discontinuous forest transect at Sodankylä for five different dates covering the 3-month period between mid-February and mid-May. Canopy structure along the transect is visualized in terms of Fveg and Tdir, as in Fig. 4.
For 19 April, results from the full ensemble are shown for two example locations at the north- and south-exposed canopy edges. Differences between stratigraphies are much more marked than for the 17 February example. Most ensemble members simulate a fully ripened snowpack at the south-exposed edge, but none do so at the north-exposed one, where dry-snow grain types prevail in the bulk of the snowpack across the ensemble at this location. Snow depth variability between ensemble members is pronounced at both locations, yet snow depths at the north-exposed edge are systematically larger than at the south-exposed edge. Some simulated snowpack properties appear to be less robust than others: at the north-exposed edge, the presence of melt forms at the surface is consistently simulated by all ensemble members, while depth hoar in the lower part of the snowpack appears in most but not all ensemble members. While this implies that this snowpack feature is associated with greater uncertainty, discrepancies between the snowpack stratigraphy predicted by different ensemble members are much smaller than differences between stratigraphy simulated at the two contrasting locations (by any ensemble member). This finding provides strong evidence of the substantial impact of canopy structural heterogeneity on modeled snow stratigraphy, suggesting that the resulting variability exceeds model uncertainty by far.
3.4 Spatial patterns and fractional partitioning of snow stratigraphy from fully distributed simulations
While Sect. 3.2 and 3.3 have a strong focus on vertical snow profiles, here, we show how fully distributed simulations can be used to assess the spatial patterns of specific snowpack properties and their relationship to canopy structure variability. As an example, Fig. 7 shows maps of snow grain type for the surface layer simulated based on the 400 m × 250 m domain in Laret at two points in time. The contribution of each grain type to the partitioning of the full domain is visualized with pie charts corresponding to each time step. These charts reveal a highly heterogeneous snow surface at both dates, which reflects a strongly variable surface energy balance and subsequent melt and metamorphism. On 13 February, precipitation particles persist over three-quarters of the domain, but melt forms prevail in the remaining quarter; 4 d later, melt forms prevail over a large part of the domain, but dendritic forms and faceted crystals persist at a few locations. Comparing these maps to the canopy height map in Fig. 1 and to average transmissivities for shortwave radiation (Sect. S4, Fig. S10) evidences the links between snow grain type distribution patterns and canopy structure. For instance, the early appearance of melt forms along south-exposed forest edges and the persistence of dry-snow grain types along north-exposed forest edges imply that shading of the snow surface exerts a major control on metamorphism.
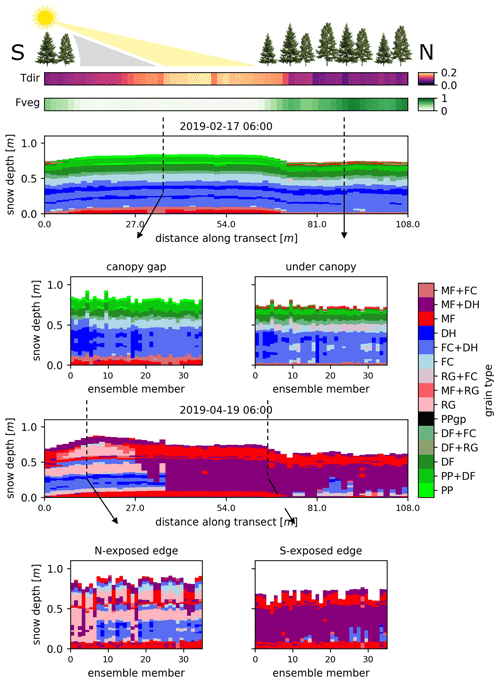
Figure 6Ensemble simulations of snow stratigraphy in terms of grain type at two contrasting locations within the forest transect from Fig. 4 for the first and third time steps shown therein. The upper scene contrasts points located in the canopy gap and in the closed canopy during accumulation, while the lower scene compares north- and south-exposed canopy edges during ablation.
As suggested by Mazzotti et al. (2021a) and Broxton et al. (2021), hyper-resolution forest snow simulations can be used to assess the explicit sub-grid variability that cannot be captured with spatially aggregated, coarser-resolution simulations. Here, we contrast the distribution of surface grain type between the deterministic, spatially explicit FSMCRO simulation and the ensemble run obtained for one point that features canopy properties representing an average over the area. This allows us to assess whether unresolved variability in coarse-resolution simulations induced by forest structure is considerable compared to the uncertainty in snowpack simulations captured by the ensemble. Figure 8 shows the evolution of snow surface grain type partitioning over the domain from Fig. 7 during February 2019, with data sampled at 6-hourly intervals. The time series evidences that canopy-mediated processes induce strong spatial heterogeneity during some periods but not in others. Strong spatial variability, evidenced by the presence of many different grain types during a specific time step, notably occurs in phases following snowfall events when metamorphism occurs at variable rates across the domain. During these periods, the spatially averaged ensemble simulations feature a much more marked transition from precipitation particles to melt forms, while the deterministic, fully distributed simulation reveals a phase during which melt forms and dry-snow types co-exist at the surface (e.g., between 12 and 22 February). Not surprisingly, the ensemble does not capture variable metamorphism rates that are tightly linked to specific canopy structures. Overall, the hyper-resolution simulation reveals more diverse snow surface conditions and smoother transitions between dry- and wet-snow regimes.
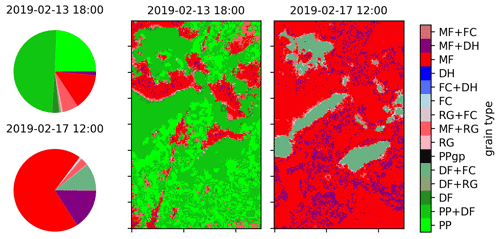
Figure 7Pie charts and maps visualizing the fractional partitioning of snow surface properties in terms of snow grain type over the 400 m × 250 m domain in Laret during two time steps in February 2019.
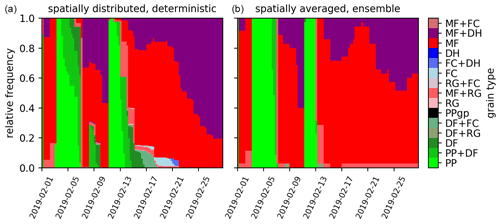
Figure 8Temporal evolution of grain type partitioning over the 400 m × 250 m domain shown in Fig. 7 during February 2019 at a temporal resolution of 6 h, as derived from the deterministic, spatially distributed simulation at 2 m resolution (a) and from the ensemble members of the point simulation representing spatially aggregated canopy properties (b). The period includes two snowfall events (around 3 and 11 February), characterized by the appearance of precipitation particles (PPs) across the domain.
4.1 FSMCRO – a new hyper-resolution forest snow modeling tool
For the first time, this study presented snow stratigraphy simulations obtained with a microstructure-resolving snow physics model that sees the explicit impact of forest canopy structure on sub-canopy micrometeorological conditions at the meter scale. To our knowledge, this type of forest snow simulation at a comparable spatial resolution had previously only been attempted by Perrot et al. (2014). In their study, however, the impact of fine-scale canopy structure on meteorological variables other than snowfall (via interception and unloading) was disregarded, neglecting the accurate representation of sub-canopy irradiance patterns. The establishment of the FSMCRO model framework, which overcomes this limitation and made our simulations possible, therefore constitutes a major contribution. Its development was a logical next step following recent progress in hyper-resolution forest snow modeling; however, an attempt to fully couple Crocus and the FSM2 canopy shed light on numerical pitfalls that are likely not to be uncommon but that are poorly documented in literature. For instance, Cristea et al. (2022) showed that choices of snow layer number and thickness can considerably impact snow simulations, which does suggest numerical instabilities; such potential model artifacts are difficult to identify and can be problematic, especially for model application studies.
The goal of the relatively simple approach chosen for FSMCRO was to develop a modeling system that avoids numerical issues and facilitates its integration into other model frameworks in the interest of transferability and easy usage in future applications. In this context, using meteorological transfer functions instead of a fully coupled canopy implementation entails some key advantages. Firstly, it allowed us to circumvent numerical issues by fully treating thermal diffusion in the snow routine rather than requiring a sequential calculation of canopy and snow thermal states. As a result, the model allows for the same type of snow surface boundary conditions at open and forested locations, which ensures that all options contained in the ensemble modeling framework ESCROC are usable. This is not (yet) the case with a MEB-like, coupled canopy implementation: for instance, a lacking implementation of the stability correction by Martin and Lejeune (1998) in MEB means the corresponding turbulent exchange parameterization option is not applicable to forest simulations with MEB-Crocus, as noted by Nousu et al. (2024). Moreover, although most transfer functions used here are relatively simple, each variable can be modified separately where more sophisticated, locally calibrated parameterizations are available, such as the sub-canopy longwave radiation model by Webster et al. (2017a) or alternative interception and unloading functions as considered in Lumbrazo et al. (2022). Lastly, the concept is not specific to Crocus and should be easily transferable to other complex snow models that have, so far, mainly been applied at open sites, e.g., SNOWPACK (Bartelt and Lehning, 2002). It should be noted that meteorological transfer functions have been used to apply snow models without canopy implementations to forested sites before (Gelfan et al., 2004; Perrot et al., 2014; Bonner et al., 2022b).
Foregoing a full canopy–snow coupling means that feedbacks between snow surface and canopy states cannot be accounted for, yet this simplification is acceptable. In fact, the canopy implementation in SNOWPACK (Gouttevin et al., 2015) also chose to neglect these interactions: snow surface temperature is therein assumed to be a constant variable when solving the canopy energy balance. Moreover, as shown, e.g., by Webster et al. (2017b), air temperature is a reasonable proxy for canopy temperature in many situations, except close to tree trunks that are exposed to direct sunlight over longer periods, while variability in longwave enhancement is mainly dictated by canopy density (Rutter et al., 2023). For these reasons, the representation of canopy temperatures in FSMCRO can be expected to capture canopy-induced spatial variability sufficiently well. The only major drawback of meteorological transfer functions is that they do not yield canopy states and are therefore not suitable for model frameworks that require these to be tracked (i.e., integrated land surface and atmospheric model systems). Yet, for most applications, the FSMCRO code base provides a convenient framework to test coupled canopy representations in the future. Such efforts should opt for a model structure that enables (or at least approximates) tight coupling of the canopy and snow model components by solving both the canopy and the snowpack energy budget in the same equation system. Corresponding numerical approaches have been proposed, e.g., by Boone et al. (2017) and Clark et al. (2015).
4.2 New insights into the impact of canopy structure on snow stratigraphy
The proposed FSMCRO system allowed for snow simulations that capture how canopy structure variability translates to variability in snow stratigraphy and associated microstructural properties. Spatially distributed ensemble simulations contain an enormous amount of information that is not accessible through simpler models or observations, including the horizontal, vertical, and temporal variability of multiple snowpack properties and their associated uncertainties. Results presented in Sect. 3 showcase some ideas on how to analyze these multidimensional data and yielded first insights into the impact of fine-scale canopy characteristics on layer-scale snow properties.
Our simulations indicate that canopy structure can have a considerable impact on snow stratigraphy. We identified two main canopy-mediated mechanisms that create variability in our simulations, with different spatiotemporal patterns. First, drip unloading of intercepted snow at above-freezing temperatures that occurs at under-canopy locations but is absent in gaps created layers that are only found under the closed canopy, typically consisting of melt forms. Second, variations in energy input into the snow surface, which are strongly linked to insolation patterns, entail spatial variability in metamorphism, yielding heterogeneous patterns of dry grain types and melt forms. This effect requires sufficiently high solar radiation input to be relevant and is therefore especially notable after peak winter accumulation. The first mechanism is in line with findings from Bonner et al. (2022a), who observed a clear signature of canopy-unloading events in snow density profiles under the canopy using SUMMA model point simulations, and from Bouchard et al. (2024), who found snow stratigraphy simulated by SNOWPACK to be sensitive to the properties of unloading snow. The second mechanism has, to our knowledge, not been captured by any simulation prior to this study.
An interesting consequence of these two mechanisms is that different patterns of spatial variability arise at different times in the snow season. Early in the winter, horizontal variability is rather small, and contrasts mainly occur between closed-canopy and canopy gap environments. As shortwave irradiance increases, insolation-driven variability in snow metamorphism and snowpack ripening can cause stratigraphy to be highly heterogeneous over short distances. Canopy edge environments with opposite orientations hereby contribute to enhancing this spatial heterogeneity further. Near the snow surface, spatial variability is strongly governed by snowfall events: while every snowfall creates homogeneous snow surface conditions for a short period, the subsequent metamorphism create strongly heterogeneous patterns that persist until surface melt is attained everywhere (or until a new snowfall event occurs).
We further demonstrated the use of ensemble simulations and hyper-resolution simulations in tandem to assess whether stratigraphy differences induced by canopy structure are robust relative to model uncertainties. Examples shown in Sect. 3.3 include distinct features at specific locations and differences between locations that are captured by a majority of the ensemble members. In such cases, we could confirm that simulated snowpack variability induced by canopy structure is indeed larger than estimated snow model uncertainty. A consequence of this finding is that the spread of ESCROC simulations cannot be considered to be a proxy of sub-grid variability for coarse-resolution simulations over heterogeneous forest landscapes, as shown in Sect. 3.4. This has important implications for future applications of ESCROC in forests. For instance, the fact that snowpack properties not captured by ESCROC are associated with specific canopy structure features would entail systematic, canopy-dependent model errors. A better understanding of where and when such errors occur would increase the utility of microstructure-resolving forest snow cover simulations, e.g., in the context of snow remote sensing applications.
Conclusions drawn from our simulations are, of course, dependent on the capabilities of the model system and are unavoidably affected by its limitations, such as missing processes. Nevertheless, although available observations did not allow a more detailed evaluation of our simulations, our model-based findings are generally consistent with the few observational studies that have addressed snow microstructural properties, stratigraphy, and its spatial variability within the forest. Comparing snow pits in canopy gaps and in the closed canopy, Bouchard et al. (2022) identified layers specific to closed-canopy locations that were absent in forest gaps, highlighting the impact of snow unloading from the canopy. Optical grain size measurements in trenches along tree boles presented by Molotch et al. (2016) revealed distinct differences in snow grain size at small vs. large distances from tree trunks and faster metamorphism on the south- vs. north-exposed sides of trees. Based on SnowMicroPen measurements along transects, Teich et al. (2019) found greater spatial heterogeneity in snow stratigraphy in within-stand transects than in open terrain. And while we are not aware of snow stratigraphy observations that contrast canopy edges of different orientations, earlier melt-out along sun-exposed forest edges than along shaded ones, as observed in numerous studies (e.g., Mazzotti et al., 2020b; Safa et al., 2021), attests to the fact that metamorphism rates must have diverged at an earlier stage of the snowpack evolution. Results from our simulations are in line with all of these experimental findings.
4.3 Paving the way for future forest snow model applications
The amount of information accessible through FSMCRO simulations creates plenty of opportunities for novel model applications. Besides avalanche research, several disciplines could benefit from model predictions of snow microstructures in forests. Snowpack properties such as surface density and the presence of ice layers are key features impacting wildlife ecology and affecting animal movement, prey–predator interactions, winter habitats, and foraging success (Sullender et al., 2023; Cosgrove et al., 2021). Gas transfer through the snowpack is also impacted by microstructural properties, with implications for wintertime greenhouse gas exchange in boreal environments (Pirk et al., 2016; Graham and Risk, 2018). Finally, knowledge of snow microstructures and/or of layer-scale properties is key for the interpretation of certain remote sensing retrievals, e.g., microwave remote sensing and ground-penetrating radar (Webb et al., 2018; Picard et al., 2018). In all these contexts, application of a model like FSMCRO holds great potential to advance research and facilitate studies in forested environments.
Ultimately, such detailed simulations also increase our understanding of the modeled system. The scale gap between the true spatial variability of snow–vegetation interactions and typical resolutions of hydrological and land surface models has long been a major challenge for modeling forest snow due to the difficulty of linking point-scale observations to simulations (Clark et al., 2011; Fassnacht, 2021). The framework proposed here constitutes an additional step in overcoming this gap. Linking specific snowpack features to specific locations in heterogeneous canopy structures based on hyper-resolution simulations can help in identifying process interactions that have never been studied and could, for instance, motivate strategies for the representation of sub-grid variability in coarse-resolution models. More analysis is needed to understand which canopy and meteorological conditions favor spatial heterogeneity in snow microstructures and for what time extents these heterogeneities persist. Our results suggest the unloading of canopy snow and insolation patterns in discontinuous forests as major drivers of spatial variability in snow microstructures, a hypothesis which should be explored and ideally backed up with validation data in future studies.
Alternatively, FSMCRO simulations could be used as a benchmark for further development of ESCROC in view of applications to forests at coarser resolutions. Our results suggest, for instance, that canopy heterogeneity, e.g., in terms of LAI perturbations or canopy parameter sets specific to characteristic forest locations, could be implemented as an additional option in the ESCROC ensemble to increase its spread when applied to forest locations. This approach would enable ensemble simulations that better capture the unresolved spatial variability which arises from heterogeneous canopy structures. Moreover, such ensemble simulations could also provide an estimate of model uncertainty when applied to forest sites where canopy descriptors are poorly constrained. Generally, the recent strong push towards replacing computational bottlenecks by emulators trained on detailed, physics-based, high-resolution models in geoscientific and cryospheric research (Jouvet, 2023; Uchôa da Silva et al., 2022) underlines the current need for further development and improvement of such detailed, physics-based modeling approaches.
4.4 Identifying priorities for follow-up research
Because the purpose of this study was to provide a proof of concept, we used FSM2 and ESCROC out of the box rather than putting effort into fine-tuning our simulations to match available validation data. Yet, our results already revealed some potential for further model enhancement, as well as a current need for additional validation data and improved approaches to analyze the multidimensional simulation results which would support such model development.
Results from Sect. 3.1 suggest that FSMCRO slightly overestimates snow depth on average and slightly underestimates its variability compared to FSM2. A possible reason is that parameterizations of snowpack properties in Crocus do not take forest into account. In FSM2, in contrast, roughness length and albedo parameterizations were modified as a function of canopy density to mimic the effect of litterfall on snow surface albedo and the impact of a heterogeneous surface on roughness length (Mazzotti et al., 2020b). While adaptations to the Crocus model were beyond the scope of this study, exploring whether its parameterizations can be adapted to better represent forest conditions should be addressed in future studies. Beyond adjustments to the default albedo parameterizations, Crocus' recent developments to incorporate impurities (Tuzet et al., 2017) provide an interesting avenue that could be extended to litterfall. Moreover, very recent work by Bouchard et al. (2024) has evidenced microstructure-resolving models like Crocus to be more sensitive to the phase and properties of unloading snow than intermediate-complexity snow models. More realistic representations of canopy snow states, as suggested in their work, would likely benefit FSMCRO as well. Further, Crocus is also more sensitive to initial soil conditions (Lafaysse et al., 2017); here, we focused on the snow season and provided homogeneous initial conditions to ensure compatibility with Mazzotti et al. (2020a, b), but differential shading might also imply differential initial conditions at the soil interface. Interdisciplinary efforts involving datasets acquired by a growing community of forest microclimate researchers (Lembrechts et al., 2020) would, apart from extended model spin-up, allow us to refine initial soil conditions for future FSMCRO applications at hyper-resolutions.
Any further model development effort would obviously benefit from more evaluation data. Even where snow stratigraphy data are available (e.g., Bonner et al., 2022b), comparison with model results is not straightforward due to unavoidable discrepancies in total snow depth and the often-limited vertical resolution of traditional snow pit measurements (e.g., Leppänen et al., 2016), requiring the application of layer-matching algorithms (Hagenmuller and Pilloix, 2016) that still face unsolved methodological challenges (Viallon-Galinier et al., 2020). For validating FSMCRO simulations, sampling multiple locations within the forest would be indispensable, and our findings could inform suitable sampling strategies to cover contrasting snow stratigraphies. Moreover, drone-based platforms could be explored as alternative data sources for validating simulated snow surface properties and their spatial variability, (e.g., through thermal imaging; Lundquist et al., 2018). Yet, besides validation data, new approaches to quantitatively analyze the multidimensional information accessible through the simulations also need to be developed. Existing layer-matching algorithms target the comparison of observations and simulations at the same locations, but fewer approaches exist to quantitatively compare profiles from different locations (Herla et al., 2024).
For the first time, this study merged the capabilities of a tree-scale forest snow model and a detailed snow physics model to enable layer- and microstructure-resolving forest snow simulations at 2 m spatial resolution, including uncertainty estimates with an ensemble. Application to sub-alpine and boreal forests, intended primarily as a proof of concept, evidenced a strong influence of tree-scale canopy properties on the evolution of snow stratigraphy, with marked spatiotemporal patterns arising (1) as a result of interception and subsequent drip unloading of canopy snow, which occurs in closed canopies but not in canopy gaps, and (2) due to insolation that drives spatial differences in the snow energy balance and subsequently also in metamorphism and melt – for instance, between canopy edges of opposite orientations. Our results underline the potential of FSMCRO simulations as a tool for scientific applications. The multidimensional information provided by these simulations, covering different state variables, their vertical and horizontal variability, their temporal evolution, and their uncertainty assessment, is unprecedented and cannot be obtained with observational methods or simpler models. It thus constitutes a valuable complement to existing tools and can contribute to advancing our understanding of forest snowpack dynamics for the benefit of several research disciplines. Besides inspiring new applications, we hope that this work will also motivate further data acquisition towards continued model validation and enhancement.
A1 Adjustments to above-canopy meteorological forcings to represent below-canopy conditions
FSMCRO requires meteorological inputs of snowfall and rainfall rates, direct and diffuse shortwave and longwave radiation, air temperature and relative humidity, surface pressure, and wind velocity. When driving a forest snow model with station data, meteorological forcing is usually from either above the canopy or a nearby open site. In the latter case, it is commonly assumed that measurements from the open site correspond to above-canopy conditions. The transfer functions applied with FSMCRO then aim to adjust above-canopy meteorology to below-canopy conditions.
For FSMCRO, the idea was to keep the adjustments as simple and close to FSM2 as possible while, at the same time, accounting for the specific impact of different characteristics of the canopy on different meteorological variables by means of process-specific canopy structure metrics as implemented in FSM2. Consequently, the below equations heavily rely on Mazzotti et al. (2020b, c) and the Appendices therein. Table A1 provides a summary of the similarities and differences in terms of process representation between FSM2 and ESCROC.
The below-canopy reference level was chosen to be 2 m, which means that the ESCROC snow schemes “see” the meteorological forcing as though it were measured at a given height above ground. In the following, the subscript “a” denotes above-canopy (or atmospheric) meteorological conditions, while the subscript “c” refers to the adjusted below-canopy variables.
Like in FSM2, above-canopy direct and diffuse shortwave radiation components SWa,b and SWa,d are scaled by the respective transmissivities (τb and τd) and are summed to obtain total sub-canopy incoming shortwave radiation:
where τb is provided as the time-varying input into the model, while τd is static and corresponds to the hemispherical sky-view fraction.
Atmospheric longwave radiation is enhanced by thermal radiation emitted by the canopy, where the weighting of atmospheric and canopy components is based on sky-view fraction (i.e., τd), as in FSM2. However, canopy temperature is assumed to equal air temperature (Ta, provided as forcing), unlike in FSM2 (where canopy temperature is a state variable).
Below-canopy wind velocity is obtained using the wind profiles implemented in FSM2, where the wind profile Usc at any location with a given stand-scale canopy cover fraction fvs (canopy structure input of FSM2, computed equivalently to canopy top height h) is a weighted average of the open-site logarithmic profile (Uopn) and the wind profile, corresponding to the dense canopy (Udc):
A composite log-exp wind profile is assumed in the dense canopy, where decay is logarithmic above the canopy, exponential from the canopy top h to a reference level zsub below the canopy (in FSM2, zsub=2 m), and logarithmic between zsub and the ground.
In the above, h denotes stand-scale canopy height (i.e., computed over a 50 m radius around a point – canopy structure input of FSM2), d=0.67h is the zero-plane displacement, z0v=0.1h is the vegetation roughness length, η=2.5 is a wind decay factor, and z0g is the ground roughness length. Wind speed at the below-canopy reference height is thus obtained as Usc(z) with z=2 m.
Snowfall and rainfall rates are modified to account for interception of snow in the canopy and its subsequent unloading and sublimation. Interception and unloading parameterizations, based on Hedstrom and Pomeroy (1998), are identical to those implemented in FSM2, including local canopy cover fraction fvl and leaf area index as canopy descriptors to yield realistic spatial variability. Over each time step δt, the increase in intercepted snow mass δSv is
where Smax=4.4LAI is the maximum canopy snow-holding capacity. Snow unloads from the canopy at a constant rate,
with different values of the time constant τu for cold and melting snow. Unloading snow is added to snowfall if the air temperature is below freezing, and it is added as rainfall otherwise.
Interception of rainfall is not accounted for, nor is snow redistribution by wind, which is, in any case, only minor at our sites (Mazzotti et al., 2020b). All other meteorological variables (air temperature, surface pressure, and relative humidity) are left unchanged from open-site conditions. The conversion of relative to specific humidity, which is needed by Crocus, is implemented in the model.
A2 Crocus options used in the deterministic and ensemble simulations
Table A2 lists the combinations of Crocus options used for the deterministic run (operational configuration, Vernay et al., 2022), as well as for the ensemble members applied in this study. Abbreviations used to denote the different Crocus options follow Lafaysse et al. (2017).
A3 Snow grain type classification
Abbreviations for the snow grain types used in Figs. 4 and 6–8 are defined in Fig. A1 and displayed alongside the corresponding categorical color codes, following Fierz et al. (2009). Colors in the first column correspond to categories consisting of each specific snow grain type only. Colors in the second column correspond to “mixed” categories composed of multiple grain types and include all mixed categories in which the corresponding grain type occurs (e.g., the purple color present in the rows of MF and DH indicates the category MF + DH). Only categories that contain melt forms are considered to be “wet-snow” types.
SURFEX is an open-source project (http://www.umr-cnrm.fr/surfex, SURFEX, 2024) but requires registration; instructions are available at https://opensource.umr-cnrm.fr/projects/snowtools_git/wiki/Procedure_for_new_users (last access: 27 September 2024). The standalone Crocus version used in this work is available in Git (access requires registration) (https://opensource.umr-cnrm.fr/projects/snowtools_git/wiki/Procedure_for_new_users, tag: s2m_top_202305; Lafaysse et al., 2024). The FSMCRO code is available on GitHub (https://github.com/GiuliaMazzotti/FSMCRO, last access: 2 October 2024) and Zenodo (https://doi.org/10.5281/zenodo.13881006; Mazzotti, 2024). Observational datasets are all published at https://doi.org/10.16904/envidat.162 (Mazzotti et al., 2020c) and https://doi.org/10.16904/envidat.220 (Mazzotti et al., 2021b).
The supplement related to this article is available online at: https://doi.org/10.5194/tc-18-4607-2024-supplement.
This study was designed by GM, ML, and TJ. RN, ML, and VV developed the standalone Crocus version. GM implemented the FSMCRO system, conducted the simulations, and analyzed the results, with support from JPN, ML, VV, and TJ. GM wrote the paper, with contributions and feedback from all the co-authors.
The contact author has declared that none of the authors has any competing interests.
Publisher's note: Copernicus Publications remains neutral with regard to jurisdictional claims made in the text, published maps, institutional affiliations, or any other geographical representation in this paper. While Copernicus Publications makes every effort to include appropriate place names, the final responsibility lies with the authors.
Giulia Mazzotti was funded by a Postdoc.Mobility grant from the Swiss National Science Foundation (project no. TP500PN_202741). Jari-Pekka Nousu was funded by the Research Council of Finland (ArcI Profi 4) and the EU Horizon Europe Framework Programme for Research and Innovation (GreenFeedBack project; grant no. 101056921). Fieldwork in Sodankylä was supported by InterACT (grants IME4Rad and UpForSnow). We thank Léo Viallon-Galinier and Mathieu Fructus for their substantial work on the snowtools visualization software and their support with it; Anna Kontu and Leena Leppänen for sharing FMI datasets and their insights regarding these; Richard Essery, Kévin Fourteau, Julien Brondex, Aaron Boone, and Isabelle Gouttevin for the insightful discussions on model coupling; Bertrand Cluzet for the help with the ensemble simulations; Marie Dumont and Jessica Lundquist their scientific input and support of this project; and Antoine Corcket and Antoine Courteaud for advancing Crocus applications in the forest during their internships at the CEN. Canopy structure and meteorological datasets used in this study would not exist without the valuable work of Clare Webster and Johanna Malle. Finally, we thank two anonymous reviewers for their constructive comments, which helped to improve this article.
This research has been supported by the Schweizerischer Nationalfonds zur Förderung der Wissenschaftlichen Forschung (grant no. TP500PN_202741).
This paper was edited by Alexandre Langlois and reviewed by two anonymous referees.
Alonso-González, E., Aalstad, K., Baba, M. W., Revuelto, J., López-Moreno, J. I., Fiddes, J., Essery, R., and Gascoin, S.: The Multiple Snow Data Assimilation System (MuSA v1.0), Geosci. Model Dev., 15, 9127–9155, https://doi.org/10.5194/gmd-15-9127-2022, 2022.
Bales, R. C., Molotch, N. P., Painter, T. H., Dettinger, M. D., Rice, R., and Dozier, J.: Mountain hydrology of the western United States, Water Resour. Res., 42, W08432, https://doi.org/10.1029/2005WR004387, 2006.
Barnhart, T. B., Molotch, N. P., Livneh, B., Harpold, A. A., Knowles, J. F., and Schneider, D.: Snowmelt rate dictates streamflow: Snowmelt Rate Dictates Streamflow, Geophys. Res. Lett., 43, 8006–8016, https://doi.org/10.1002/2016GL069690, 2016.
Barrere, M., Domine, F., Decharme, B., Morin, S., Vionnet, V., and Lafaysse, M.: Evaluating the performance of coupled snow–soil models in SURFEXv8 to simulate the permafrost thermal regime at a high Arctic site, Geosci. Model Dev., 10, 3461–3479, https://doi.org/10.5194/gmd-10-3461-2017, 2017.
Bartelt, P. and Lehning, M.: A physical SNOWPACK model for the Swiss avalanche warning Part I: numerical model, Cold Reg. Sci. Technol., 23, 123–145, https://doi.org/10.1016/S0165-232X(02)00074-5, 2002.
Boelman, N. T., Liston, G. E., Gurarie, E., Meddens, A. J. H., Mahoney, P. J., Kirchner, P. B., Bohrer, G., Brinkman, T. J., Cosgrove, C. L., Eitel, J. U. H., Hebblewhite, M., Kimball, J. S., LaPoint, S., Nolin, A. W., Pedersen, S. H., Prugh, L. R., Reinking, A. K., and Vierling, L. A.: Integrating snow science and wildlife ecology in Arctic-boreal North America, Environ. Res. Lett., 14, 010401, https://doi.org/10.1088/1748-9326/aaeec1, 2019.
Bonner, H. M., Raleigh, M. S., and Small, E. E.: Isolating forest process effects on modelled snowpack density and snow water equivalent, Hydrol. Process., 36, e14475, https://doi.org/10.1002/hyp.14475, 2022a.
Bonner, H. M., Smyth, E., Raleigh, M. S., and Small, E. E.: A Meteorology and Snow Data Set from Adjacent Forested and Meadow Sites at Crested Butte, CO, USA, Water Resour. Res., 58, e2022WR033006, https://doi.org/10.1029/2022WR033006, 2022b.
Boone, A., Samuelsson, P., Gollvik, S., Napoly, A., Jarlan, L., Brun, E., and Decharme, B.: The interactions between soil–biosphere–atmosphere land surface model with a multi-energy balance (ISBA-MEB) option in SURFEXv8 – Part 1: Model description, Geosci. Model Dev., 10, 843–872, https://doi.org/10.5194/gmd-10-843-2017, 2017.
Bouchard, B., Nadeau, D. F., and Domine, F.: Comparison of snowpack structure in gaps and under the canopy in a humid boreal forest, Hydrol. Process., 36, e14681, https://doi.org/10.1002/hyp.14681, 2022.
Bouchard, B., Nadeau, D. F., Domine, F., Wever, N., Michel, A., Lehning, M., and Isabelle, P.-E.: Impact of intercepted and sub-canopy snow microstructure on snowpack response to rain-on-snow events under a boreal canopy, The Cryosphere, 18, 2783–2807, https://doi.org/10.5194/tc-18-2783-2024, 2024.
Bramer, I., Anderson, B. J., Bennie, J., Bladon, A. J., De Frenne, P., Hemming, D., Hill, R. A., Kearney, M. R., Körner, C., Korstjens, A. H., Lenoir, J., Maclean, I. M. D., Marsh, C. D., Morecroft, M. D., Ohlemüller, R., Slater, H. D., Suggitt, A. J., Zellweger, F., and Gillingham, P. K.: Chapter Three - Advances in Monitoring and Modelling Climate at Ecologically Relevant Scales, in: Advances in Ecological Research, vol. 58, edited by: Bohan, D. A., Dumbrell, A. J., Woodward, G., and Jackson, M., Academic Press, 101–161, https://doi.org/10.1016/bs.aecr.2017.12.005, 2018.
Broxton, P. D., Harpold, A. A., Biederman, J. A., Troch, P. A., Molotch, N. P., and Brooks, P. D.: Quantifying the effects of vegetation structure on snow accumulation and ablation in mixed-conifer forests, Ecohydrol., 8, 1073–1094, https://doi.org/10.1002/eco.1565, 2015.
Broxton, P. D., Leeuwen, W. J. D., and Biederman, J. A.: Forest cover and topography regulate the thin, ephemeral snowpacks of the semiarid Southwest United States, Ecohydrology, 13, e2202, https://doi.org/10.1002/eco.2202, 2020.
Broxton, P. D., Moeser, C. D., and Harpold, A.: Accounting for Fine-Scale Forest Structure is Necessary to Model Snowpack Mass and Energy Budgets in Montane Forests, Water Resour. Res., 57, e2021WR029716, https://doi.org/10.1029/2021WR029716, 2021.
Brun, E., Martin, E., Simon, V., Gendre, C., and Coleou, C.: An Energy and Mass Model of Snow Cover Suitable for Operational Avalanche Forecasting, J. Glaciol., 35, 333–342, https://doi.org/10.3189/S0022143000009254, 1989.
Brun, E., David, P., Sudul, M., and Brunot, G.: A numerical model to simulate snow-cover stratigraphy for operational avalanche forecasting, J. Glaciol., 38, 13–22, 1992.
Carmagnola, C. M., Morin, S., Lafaysse, M., Domine, F., Lesaffre, B., Lejeune, Y., Picard, G., and Arnaud, L.: Implementation and evaluation of prognostic representations of the optical diameter of snow in the SURFEX/ISBA-Crocus detailed snowpack model, The Cryosphere, 8, 417–437, https://doi.org/10.5194/tc-8-417-2014, 2014.
Clark, M. P., Hendrikx, J., Slater, A. G., Kavetski, D., Anderson, B., Cullen, N. J., Kerr, T., Örn Hreinsson, E., and Woods, R. A.: Representing spatial variability of snow water equivalent in hydrologic and land-surface models: A review, Water Resour. Res., 47, W07539, https://doi.org/10.1029/2011WR010745, 2011.
Clark, M. P., Nijssen, B., Lundquist, J., Kavetski, D., Rupp, D., Woods, R., Gutmann, E., Wood, A., Gochis, D., Rasmussen, R., Tarboton, D., Mahat, V., Flerchinger, G., and Marks, D.: A unified approach for process-based hydrologic modeling: Part 2. Model implementation and example applications, Water Resour. Res., 51, 2498–2514, https://doi.org/10.1002/2015WR017200, 2015.
Colman, R. A.: Surface albedo feedbacks from climate variability and change, J. Geophys. Res.-Atmos., 118, 2827–2834, https://doi.org/10.1002/jgrd.50230, 2013.
Cosgrove, C. L., Wells, J., Nolin, A. W., Putera, J., and Prugh, L. R.: Seasonal influence of snow conditions on Dall's sheep productivity in Wrangell-St Elias National Park and Preserve, PLoS One, 16, e0244787, https://doi.org/10.1371/journal.pone.0244787, 2021.
Cristea, N. C., Bennett, A., Nijssen, B., and Lundquist, J. D.: When and Where Are Multiple Snow Layers Important for Simulations of Snow Accumulation and Melt?, Water Resour. Res., 58, e2020WR028993, https://doi.org/10.1029/2020WR028993, 2022.
Decharme, B., Boone, A., Delire, C., and Noilhan, J.: Local evaluation of the Interaction between Soil Biosphere Atmosphere soil multilayer diffusion scheme using four pedotransfer functions, J. Geophys. Res.-Atmos., 116, D20126, https://doi.org/10.1029/2011JD016002, 2011.
Dick, O., Viallon-Galinier, L., Tuzet, F., Hagenmuller, P., Fructus, M., Reuter, B., Lafaysse, M., and Dumont, M.: Can Saharan dust deposition impact snowpack stability in the French Alps?, The Cryosphere, 17, 1755–1773, https://doi.org/10.5194/tc-17-1755-2023, 2023.
Di Mauro, B., Garzonio, R., Rossini, M., Filippa, G., Pogliotti, P., Galvagno, M., Morra di Cella, U., Migliavacca, M., Baccolo, G., Clemenza, M., Delmonte, B., Maggi, V., Dumont, M., Tuzet, F., Lafaysse, M., Morin, S., Cremonese, E., and Colombo, R.: Saharan dust events in the European Alps: role in snowmelt and geochemical characterization, The Cryosphere, 13, 1147–1165, https://doi.org/10.5194/tc-13-1147-2019, 2019.
Domine, F., Barrere, M., and Morin, S.: The growth of shrubs on high Arctic tundra at Bylot Island: impact on snow physical properties and permafrost thermal regime, Biogeosciences, 13, 6471–6486, https://doi.org/10.5194/bg-13-6471-2016, 2016.
Domine, F., Gauthier, G., Vionnet, V., Fauteux, D., Dumont, M., and Barrere, M.: Snow physical properties may be a significant determinant of lemming population dynamics in the high Arctic, Arctic Sci., 4, 813–826, 2018.
Dumont, M., Tuzet, F., Gascoin, S., Picard, G., Kutuzov, S., Lafaysse, M., Cluzet, B., Nheili, R., and Painter, T. H.: Accelerated Snow Melt in the Russian Caucasus Mountains After the Saharan Dust Outbreak in March 2018, J. Geophys. Res.-Earth Surf., 125, e2020JF005641, https://doi.org/10.1029/2020JF005641, 2020.
Eidhammer, T., Booth, A., Decker, S., Li, L., Barlage, M., Gochis, D., Rasmussen, R., Melvold, K., Nesje, A., and Sobolowski, S.: Mass balance and hydrological modeling of the Hardangerjøkulen ice cap in south-central Norway, Hydrol. Earth Syst. Sci., 25, 4275–4297, https://doi.org/10.5194/hess-25-4275-2021, 2021.
Ellis, C. R., Pomeroy, J. W., and Link, T. E.: Modeling increases in snowmelt yield and desynchronization resulting from forest gap-thinning treatments in a northern mountain headwater basin, Water Resour. Res., 49, 936–949, https://doi.org/10.1002/wrcr.20089, 2013.
Essery, R.: A factorial snowpack model (FSM 1.0), Geosci. Model Dev., 8, 3867–3876, https://doi.org/10.5194/gmd-8-3867-2015, 2015.
Essery, R., Rutter, N., Pomeroy, J., Baxter, R., Stähli, M., Gustafsson, D., Barr, A., Bartlett, P., and Elder, K.: SNOWMIP2: An Evaluation of Forest Snow Process Simulations, B. Am. Meteorol. Soc., 90, 1120–1136, https://doi.org/10.1175/2009BAMS2629.1, 2009.
Essery, R., Morin, S., Lejeune, Y., and B Ménard, C.: A comparison of 1701 snow models using observations from an alpine site, Adv. Water Resour., 55, 131–148, https://doi.org/10.1016/j.advwatres.2012.07.013, 2013.
Etchevers, P., Martin, E., Brown, R., Fierz, C., Lejeune, Y., Bazile, E., Boone, A., Dai, Y.-J., Essery, R., Fernandez, A., Gusev, Y., Jordan, R., Koren, V., Kowalczyk, E., Nasonova, N. O., Pyles, R. D., Schlosser, A., Shmakin, A. B., Smirnova, T. G., Strasser, U., Verseghy, D., Yamazaki, T., and Yang, Z.-L.: Validation of the energy budget of an alpine snowpack simulated by several snow models (Snow MIP project), Ann. Glaciol., 38, 150–158, https://doi.org/10.3189/172756404781814825, 2004.
Fassnacht, S. R.: A Call for More Snow Sampling, Geosciences, 11, 435, https://doi.org/10.3390/geosciences11110435, 2021.
Fierz, C., Armstrong, R. L., Durand, Y., Etchevers, P., Greene, E., McClung, D. M., Nishimura, K., Satyawali, P. K., and Sokratov, S. A.: The International Classification for Seasonal Snow on the Ground, IHP-VII Technical Documents in Hydrology No. 83, IACS Contribution No. 1, UNESCO-IHP, Paris. UR L, https://unesdoc.unesco.org/ark:/48223/pf0000186462#:~:text=That is why in%201985 ICSI, last access: 29 September 2024), 2009.
Flanner, M. G. and Zender, C. S.: Linking snowpack microphysics and albedo evolution, J. Geophys. Res.-Atmos., 111, D12208, https://doi.org/10.1029/2005JD006834, 2006.
Fourteau, K., Brondex, J., Brun, F., and Dumont, M.: A novel numerical implementation for the surface energy budget of melting snowpacks and glaciers, Geosci. Model Dev., 17, 1903–1929, https://doi.org/10.5194/gmd-17-1903-2024, 2024.
Gallée, H., Guyomarc'h, G., and Brun, E.: Impact of snow drift on the Antarctic ice sheet surface mass balance : possible sensitivity to snow-surface properties, Bound.-Lay. Meteorol., 99, 1–19, 2001.
Gelfan, A. N., Pomeroy, J. W., and Kuchment, L. S.: Modeling Forest Cover Influences on Snow Accumulation, Sublimation, and Melt, J. Hydrometeorol., 5, 785–803, https://doi.org/10.1175/1525-7541(2004)005<0785:MFCIOS>2.0.CO;2, 2004.
Gilbert, S. L., Hundertmark, K. J., Person, D. K., Lindberg, M. S., and Boyce, M. S.: Behavioral plasticity in a variable environment: snow depth and habitat interactions drive deer movement in winter, J. Mammal., 98, 246–259, https://doi.org/10.1093/jmammal/gyw167, 2017.
Goeking, S. A. and Tarboton, D. G.: Forests and Water Yield: A Synthesis of Disturbance Effects on Streamflow and Snowpack in Western Coniferous Forests, J. Forestry, 118, 172–192, https://doi.org/10.1093/jofore/fvz069, 2020.
Gouttevin, I., Lehning, M., Jonas, T., Gustafsson, D., and Mölder, M.: A two-layer canopy model with thermal inertia for an improved snowpack energy balance below needleleaf forest (model SNOWPACK, version 3.2.1, revision 741), Geosci. Model Dev., 8, 2379–2398, https://doi.org/10.5194/gmd-8-2379-2015, 2015.
Gouttevin, I., Langer, M., Löwe, H., Boike, J., Proksch, M., and Schneebeli, M.: Observation and modelling of snow at a polygonal tundra permafrost site: spatial variability and thermal implications, The Cryosphere, 12, 3693–3717, https://doi.org/10.5194/tc-12-3693-2018, 2018.
Graham, L. and Risk, D.: Explaining CO2 fluctuations observed in snowpacks, Biogeosciences, 15, 847–859, https://doi.org/10.5194/bg-15-847-2018, 2018.
Hagenmuller, P. and Pilloix, T.: A New Method for Comparing and Matching Snow Profiles, Application for Profiles Measured by Penetrometers, Front. Earth Sci., 4, 52, https://doi.org/10.3389/feart.2016.00052, 2016.
Hedstrom, N. R. and Pomeroy, J. W.: Measurements and modelling of snow interception in the boreal forest, Hydrol. Process., 12, 1611–1625, https://doi.org/10.1002/(SICI)1099-1085(199808/09)12:10/11<1611::AID-HYP684>3.0.CO;2-4, 1998.
Herla, F., Haegeli, P., Horton, S., and Mair, P.: A large-scale validation of snowpack simulations in support of avalanche forecasting focusing on critical layers, Nat. Hazards Earth Syst. Sci., 24, 2727–2756, https://doi.org/10.5194/nhess-24-2727-2024, 2024.
Jonas, T., Webster, C., Mazzotti, G., and Malle, J.: HPEval: A canopy shortwave radiation transmission model using high-resolution hemispherical images, Agric. Forest Meteorol., 284, 107903, https://doi.org/10.1016/j.agrformet.2020.107903, 2020.
Jouvet, G.: Inversion of a Stokes glacier flow model emulated by deep learning, J. Glaciol., 69, 13–26, https://doi.org/10.1017/jog.2022.41, 2023.
Kim, E., Gatebe, C., Hall, D., Newlin, J., Misakonis, A., Elder, K., Marshall, H. P., Hiemstra, C., Brucker, L., De Marco, E., Crawford, C., Kang, D. H., and Entin, J.: NASA's snowex campaign: Observing seasonal snow in a forested environment, in: 2017 IEEE International Geoscience and Remote Sensing Symposium (IGARSS), 2017 IEEE International Geoscience and Remote Sensing Symposium (IGARSS), Fort Worth, TX, 1388–1390, https://doi.org/10.1109/IGARSS.2017.8127222, 2017.
Kontu, A., Lemmetyinen, J., Vehviläinen, J., Leppänen, L., and Pulliainen, J.: Coupling SNOWPACK-modeled grain size parameters with the HUT snow emission model, Remote Sens. Environ., 194, 33–47, https://doi.org/10.1016/j.rse.2016.12.021, 2017.
Krinner, G., Derksen, C., Essery, R., Flanner, M., Hagemann, S., Clark, M., Hall, A., Rott, H., Brutel-Vuilmet, C., Kim, H., Ménard, C. B., Mudryk, L., Thackeray, C., Wang, L., Arduini, G., Balsamo, G., Bartlett, P., Boike, J., Boone, A., Chéruy, F., Colin, J., Cuntz, M., Dai, Y., Decharme, B., Derry, J., Ducharne, A., Dutra, E., Fang, X., Fierz, C., Ghattas, J., Gusev, Y., Haverd, V., Kontu, A., Lafaysse, M., Law, R., Lawrence, D., Li, W., Marke, T., Marks, D., Ménégoz, M., Nasonova, O., Nitta, T., Niwano, M., Pomeroy, J., Raleigh, M. S., Schaedler, G., Semenov, V., Smirnova, T. G., Stacke, T., Strasser, U., Svenson, S., Turkov, D., Wang, T., Wever, N., Yuan, H., Zhou, W., and Zhu, D.: ESM-SnowMIP: assessing snow models and quantifying snow-related climate feedbacks, Geosci. Model Dev., 11, 5027–5049, https://doi.org/10.5194/gmd-11-5027-2018, 2018.
Krogh, S. A., Broxton, P. D., Manley, P. N., and Harpold, A. A.: Using Process Based Snow Modeling and Lidar to Predict the Effects of Forest Thinning on the Northern Sierra Nevada Snowpack, Front. For. Glob. Change, 3, 21, https://doi.org/10.3389/ffgc.2020.00021, 2020.
Lafaysse, M.: Modélisation numérique de la neige: la fin du déterminisme? Météorologie, Université Toulouse III – Paul Sabatier, https://theses.hal.science/tel-04130109/ (last access: 29 September 2024), 2023.
Lafaysse, M., Cluzet, B., Dumont, M., Lejeune, Y., Vionnet, V., and Morin, S.: A multiphysical ensemble system of numerical snow modelling, The Cryosphere, 11, 1173–1198, https://doi.org/10.5194/tc-11-1173-2017, 2017.
Lafaysse, M., Fructus, M., Vernay, M., Radanovics, S., Dumont, M., and Viallon-Galinier, L.: Procedure for new users of Crocus model, CNRS [code], https://opensource.umr-cnrm.fr/projects/snowtools_git/wiki/Procedure_for_new_users, last access: 27 September 2024.
Lawrence, D. M., Fisher, R. A., Koven, C. D., Oleson, K. W, Swenson, S. C., Bonan, G., Collier, N., Ghimire, B., van Kampenhout, L., Kennedy, D., Kluzek, E., Lawrence, P. J., Li, F., Li, H., Lombardozzi, D., Riley, W. J., Sacks, W. J., Shi, M., Vertenstein, M., Wieder, W. R., Xu, C., Ali, A. A., Badger, A. M., Bisht, G., van den Broeke, M., Brunke, M. A, Burns, S. P., Buzan, J., Clark, M., Craig, A., Dahlin, K., Drewniak, B., Fisher, J. B., Flanner, M., Fox, A. M., Gentine, P., Hoffman, F., Keppel-Aleks, G., Knox, R., Kumar, S., Lenaerts, J., Leung, L. R., Lipscomb, W. H., Lu, Y., Pandey, A., Pelletier, J. D., Perket, J., Randerson, J. T., Ricciuto, D. M., Sanderson, B. M., Slater, A., Subin, Z. M., Tang, J., Thomas, R. Q., Val Martin, M., and Zeng, X.: The Community Land Model version 5: Description of new features, benchmarking, and impact of forcing uncertainty, J. Adv. Model. Earth Sy., 11,4245–4287, https://doi.org/10.1029/2018MS001583, 2019.
Lembrechts, J. J., Nijs, I., and Lenoir, J.: Incorporating microclimate into species distribution models, Ecography, 42, 1267–1279, https://doi.org/10.1111/ecog.03947, 2019.
Lembrechts, J. J., Aalto, J., Ashcroft, M. B., Frenne, P. D., Kopecký, M., Lenoir, J., Luoto, M., Maclean, I. M. D., Roupsard, O., Fuentes-Lillo, E., García, R. A., Pellissier, L., Pitteloud, C., Alatalo, J. M., Smith, S. W., Björk, R. G., Muffler, L., Backes, A. R., Cesarz, S., Gottschall, F., Okello, J., Urban, J., Plichta, R., Svátek, M., Phartyal, S. S., Wipf, S., Eisenhauer, N., Pu?ca?, M., Turtureanu, P. D., Varlagin, A., Dimarco, R. D., Jump, A. S., Randall, K., Dorrepaal, E., Larson, K., Walz, J., Vitale, L., Svoboda, M., Higgens, R. F., Halbritter, A. H., Curasi, S. R., Klupar, I., Koontz, A., Pearse, W. D., Simpson, E., Stemkovski, M., Graae, B. J., Sørensen, M. V., Høye, T. T., Calzado, M. R. F., Lorite, J., Carbognani, M., Tomaselli, M., Forte, T. G. W., Petraglia, A., Haesen, S., Somers, B., Meerbeek, K. V., Björkman, M. P., Hylander, K., Merinero, S., Gharun, M., Buchmann, N., Dolezal, J., Matula, R., Thomas, A. D., Bailey, J. J., Ghosn, D., Kazakis, G., Pablo, M. A. de, Kemppinen, J., Niittynen, P., Rew, L., Seipel, T., Larson, C., Speed, J. D. M., Ardö, J., Cannone, N., Guglielmin, M., Malfasi, F., Bader, M. Y., Canessa, R., Stanisci, A., Kreyling, J., Schmeddes, J., Teuber, L., Aschero, V., Čiliak, M., Máliš, F., Smedt, P. D., Govaert, S., Meeussen, C., Vangansbeke, P., Gigauri, K., Lamprecht, A., Pauli, H., Steinbauer, K., Winkler, M., Ueyama, M., Nuñez, M. A, Ursu, T. M., Haider, S., Wedegärtner, R. E. M., Smiljanic, M., Trouillier, M., Wilmking, M., Altman, J., Brůna, J., Hederová, L., Macek, M., Man, M., Wild, J., Vittoz, P., Pärtel, M., Barančok, P., Kanka, R., Kollár, J., Palaj, A., Barros, A., Mazzolari, A. C., Bauters, M., Boeckx, P., Alonso, J. L. B., Zong, S., Di Cecco, V., Sitková, Z., Tielbörger, K., van den Brink, L., Weigel, R., Homeier, J., Dahlberg, C. J., Medinets, S., Medinets, V., De Boeck, H. J., Portillo-Estrada, M., Verryckt, L. T., Milbau, A., Daskalova, G. N., Thomas, H. J. D., Myers-Smith, I. H., Blonder, B., Stephan, J. G., Descombes, P., Zellweger, F., Frei, E. R., Heinesch, B., Andrews, Ch., Dick, J., Siebicke, L., Rocha, A., Senior, R. A., Rixen, C., Jimenez, J. J., Boike, J., Pauchard, A., Scholten, T., Scheffers, B., Klinges, D., Basham, E. W., Zhang, J., Zhang, Z., Géron, C., Fazlioglu, F., Candan, O., Bravo, J. S., Hrbacek, F., Laska, K., Cremonese, E., Haase, P., Moyano, F. E., Rossi, Ch., and Nij, I.: SoilTemp: A global database of near-surface temperature, Glob. Change Biol., 26, 6616–6629, https://doi.org/10.1111/gcb.15123, 2020.
Le Moigne, P., Besson, F., Martin, E., Boé, J., Boone, A., Decharme, B., Etchevers, P., Faroux, S., Habets, F., Lafaysse, M., Leroux, D., and Rousset-Regimbeau, F.: The latest improvements with SURFEX v8.0 of the Safran–Isba–Modcou hydrometeorological model for France, Geosci. Model Dev., 13, 3925–3946, https://doi.org/10.5194/gmd-13-3925-2020, 2020.
Leppänen, L., Kontu, A., Hannula, H.-R., Sjöblom, H., and Pulliainen, J.: Sodankylä manual snow survey program, Geosci. Instrum. Method. Data Syst., 5, 163–179, https://doi.org/10.5194/gi-5-163-2016, 2016.
Lewis, G., Harpold, A., Krogh, S. A., Broxton, P., and Manley, P. N.: The prediction of uneven snowpack response to forestthinning informs forest restoration in the central Sierra Nevada, Ecohydrology, 17, e2580, https://doi.org/10.1002/eco.2580, 2023.
Lumbrazo, C., Bennett, A., Currier, W. R., Nijssen, B., and Lundquist, J.: Evaluating Multiple Canopy-Snow Unloading Parameterizations in SUMMA With Time-Lapse Photography Characterized by Citizen Scientists, Water Resour. Res., 58, e2021WR030852, https://doi.org/10.1029/2021WR030852, 2022.
Lundquist, J. D., Chickadel, C., Cristea, N., Currier, W. R., Henn, B., Keenan, E., and Dozier, J.: Separating snow and forest temperatures with thermal infrared remote sensing, Remote Sens. Environ., 209, 764–779, https://doi.org/10.1016/j.rse.2018.03.001, 2018.
Magnusson, J., Eisner, S., Huang, S., Lussana, C., Mazzotti, G., Essery, R., Saloranta, T., and Beldring, S.: Influence of Spatial Resolution on Snow Cover Dynamics for a Coastal and Mountainous Region at High Latitudes (Norway), Water Resour. Res., 55, 5612–5630, https://doi.org/10.1029/2019WR024925, 2019.
Martin, E. and Lejeune, Y.: Turbulent fluxes above the snow surface, Ann. Glaciol., 26, 179–183, https://doi.org/10.3189/1998aog26-1-179-183, 1998.
Martz, F., Vuosku, J., Ovaskainen, A., Stark, S., and Rautio, P.: The Snow Must Go On: Ground Ice Encasement, Snow Compaction and Absence of Snow Differently Cause Soil Hypoxia, CO2 Accumulation and Tree Seedling Damage in Boreal Forest, PLOS ONE, 11, e0156620, https://doi.org/10.1371/journal.pone.0156620, 2016.
Masson, V., Le Moigne, P., Martin, E., Faroux, S., Alias, A., Alkama, R., Belamari, S., Barbu, A., Boone, A., Bouyssel, F., Brousseau, P., Brun, E., Calvet, J.-C., Carrer, D., Decharme, B., Delire, C., Donier, S., Essaouini, K., Gibelin, A.-L., Giordani, H., Habets, F., Jidane, M., Kerdraon, G., Kourzeneva, E., Lafaysse, M., Lafont, S., Lebeaupin Brossier, C., Lemonsu, A., Mahfouf, J.-F., Marguinaud, P., Mokhtari, M., Morin, S., Pigeon, G., Salgado, R., Seity, Y., Taillefer, F., Tanguy, G., Tulet, P., Vincendon, B., Vionnet, V., and Voldoire, A.: The SURFEXv7.2 land and ocean surface platform for coupled or offline simulation of earth surface variables and fluxes, Geosci. Model Dev., 6, 929–960, https://doi.org/10.5194/gmd-6-929-2013, 2013.
Mazzotti, G.: FSMCRO v1.0.0 (v1.0.0), Zenodo [code], https://doi.org/10.5281/zenodo.13881006, 2024.
Mazzotti, G., Currier, W. R., Deems, J. S., Pflug, J. M., Lundquist, J. D., and Jonas, T.: Revisiting Snow Cover Variability and Canopy Structure Within Forest Stands: Insights From Airborne Lidar Data, Water Resour. Res., 55, 6198–6216, https://doi.org/10.1029/2019WR024898, 2019.
Mazzotti, G., Essery, R., Moeser, C. D., and Jonas, T.: Resolving Small-Scale Forest Snow Patterns Using an Energy Balance Snow Model With a One-Layer Canopy, Water Resour. Res., 56, e2019WR026129, https://doi.org/10.1029/2019WR026129, 2020a.
Mazzotti, G., Essery, R., Webster, C., Malle, J., and Jonas, T.: Process-Level Evaluation of a Hyper-Resolution Forest Snow Model Using Distributed Multisensor Observations, Water Resour. Res., 56, e2020WR027572, https://doi.org/10.1029/2020WR027572, 2020b.
Mazzotti, G., Malle, J., and Jonas, T.: Distributed sub-canopy datasets from mobile multi-sensor platforms (CH / FIN, 2018–2019) for hyper-resolution forest snow model evaluation, EnviDat [data set], https://doi.org/10.16904/envidat.162, 2020c.
Mazzotti, G., Webster, C., Essery, R., and Jonas, T.: Increasing the Physical Representation of Forest-Snow Processes in Coarse-Resolution Models: Lessons Learned From Upscaling Hyper-Resolution Simulations, Water Resour. Res., 57, e2020WR029064, https://doi.org/10.1029/2020WR029064, 2021a.
Mazzotti, G., Webster, C., and Jonas, T.: Forest canopy structure data for radiation and snow modelling (CH/FIN), EnviDat [data set], https://doi.org/10.16904/envidat.220, 2021b.
Mazzotti, G., Webster, C., Quéno, L., Cluzet, B., and Jonas, T.: Canopy structure, topography, and weather are equally important drivers of small-scale snow cover dynamics in sub-alpine forests, Hydrol. Earth Syst. Sci., 27, 2099–2121, https://doi.org/10.5194/hess-27-2099-2023, 2023.
Moeser, C. D., Broxton, P. D., Harpold, A., and Robertson, A.: Estimating the Effects of Forest Structure Changes From Wildfire on Snow Water Resources Under Varying Meteorological Conditions, Water Resour. Res., 56, e2020WR027071, https://doi.org/10.1029/2020WR027071, 2020.
Molotch, N. P., Barnard, D. M., Burns, S. P., and Painter, T. H.: Measuring spatiotemporal variation in snow optical grain size under a subalpine forest canopy using contact spectroscopy: SNOW OPTICAL GRAIN SIZE IN FORESTS FROM CONTACT SPECTROSCOPY, Water Resour. Res., 52, 7513–7522, https://doi.org/10.1002/2016WR018954, 2016.
Morin, S., Domine, F., Dufour, A., Lejeune, Y., Lesaffre, B., Willemet, J.-M., Carmagnola, C. M., and Jacobi, H.-W.: Measurements and modeling of the vertical profile of specific surface area of an alpine snowpack, Adv. Water Res., 55, 111–120, https://doi.org/10.1016/j.advwatres.2012.01.010, 2013.
Morin, S., Horton, S., Techel, F., Bavay, M., Coléou, C., Fierz, C., Gobiet, A., Hagenmuller, P., Lafaysse, M., Ližar, M., Mitterer, C., Monti, F., Müller, K., Olefs, M., Snook, J. S., van Herwijnen, A., and Vionnet, V.: Application of physical snowpack models in support of operational avalanche hazard forecasting: A status report on current implementations and prospects for the future, Cold Reg. Sci. Technol., 170, 102910, https://doi.org/10.1016/j.coldregions.2019.102910, 2020.
Mote, P. W., Li, S., Lettenmaier, D. P., Xiao, M., and Engel, R.: Dramatic declines in snowpack in the western US, npj Clim. Atmos. Sci., 1, 1–6, https://doi.org/10.1038/s41612-018-0012-1, 2018.
Mott, R., Winstral, A., Cluzet, B., Helbig, N., Magnusson, J., Mazzotti, G., Quéno, L., Schirmer, M., Webster, C., and Jonas, T.: Operational snow-hydrological modeling for Switzerland, Front. Earth Sci., 11, 1228158, https://doi.org/10.3389/feart.2023.1228158, 2023.
Navari, M., Margulis, S.A., Tedesco, M., Fettweis, X., and van de Wal, R. S. W.: Reanalysis Surface Mass Balance of the Greenland Ice Sheet Along K-Transect (2000–2014), Geophys. Res. Lett., 48, e2021GL094602, https://doi.org/10.1029/2021GL094602, 2021.
Notarnicola, C.: Hotspots of snow cover changes in global mountain regions over 2000–2018, Remote Sens. Environ., 243, 111781, https://doi.org/10.1016/j.rse.2020.111781, 2020.
Nousu, J.-P., Lafaysse, M., Mazzotti, G., Ala-aho, P., Marttila, H., Cluzet, B., Aurela, M., Lohila, A., Kolari, P., Boone, A., Fructus, M., and Launiainen, S.: Modeling snowpack dynamics and surface energy budget in boreal and subarctic peatlands and forests, The Cryosphere, 18, 231–263, https://doi.org/10.5194/tc-18-231-2024, 2024.
Ouellet, F., Langlois, A., Blukacz-Richards, E. A., Johnson, C. A., Royer, A., Neave, E., and Larter, N. C.: Spatialization of the SNOWPACK snow model for the Canadian Arctic to assess Peary caribou winter grazing conditions, Phys. Geogr., 38, 143–158, 2017.
Perrot, D., Molotch, N. P., Musselman, K. N., and Pugh, E. T.: Modelling the effects of the mountain pine beetle on snowmelt in a subalpine forest, Ecohydrology, 7, 226–241, https://doi.org/10.1002/eco.1329, 2014.
Picard, G., Sandells, M., and Löwe, H.: SMRT: an active–passive microwave radiative transfer model for snow with multiple microstructure and scattering formulations (v1.0), Geosci. Model Dev., 11, 2763–2788, https://doi.org/10.5194/gmd-11-2763-2018, 2018.
Picard, G., Löwe, H., Domine, F., Arnaud, L., Larue, F., Favier, V., Le Meur, E., Lefevbre, E., Savarino, J., and Royer, A.: The Microwave Snow Grain Size: A New Concept to Predict Satellite Observations Over Snow-Covered Regions, AGU Advances, 3, e2021AV000630, https://doi.org/10.1029/2021AV000630, 2022.
Pirk, N., Tamstorf, M. P., Lund, M., Mastepanov, M., Pedersen, S. H., Mylius, M. R., Parmentier, F.-J. W., Christiansen, H. H., and Christensen, T. R.: Snowpack fluxes of methane and carbon dioxide from high Arctic tundra, J. Geophys. Res.-Biogeo., 121, 2886–2900, https://doi.org/10.1002/2016JG003486, 2016.
Rasmus, S., Gustafsson, D., Lundell, R., and Saarinen, T.: Observations and snow model simulations of winter energy balance terms within and between different coniferous forests in southern boreal Finland, Hydrol. Res., 47, 201–216, https://doi.org/10.2166/nh.2015.177, 2016.
Rutter, N., Essery, R., Pomeroy, J., Altimir, N., Andreadis, K., Baker, I., Barr, A., Bartlett, P., Boone, A., Deng, H., Douville, H., Dutra, E., Elder, K., Ellis, C., Feng, X., Gelfan, A., Goodbody, A., Gusev, Y., Gustafsson, D., Hellström, R., Hirabayashi, Y., Hirota, T., Jonas, T., Koren, V., Kuragina, A., Lettenmaier, D., Li, W.-P., Luce, C., Martin, E., Nasonova, O., Pumpanen, J., Pyles, R. D., Samuelsson, P., Sandells, M., Schädler, G., Shmakin, A., Smirnova, T. G., Stähli, M., Stöckli, R., Strasser, U., Su, H., Suzuki, K., Takata, K., Tanaka, K., Thompson, E., Vesala, T., Viterbo, P., Wiltshire, A., Xia, K., Xue, Y., and Yamazaki, T.: Evaluation of forest snow processes models (SnowMIP2), J. Geophys. Res., 114, D06111, https://doi.org/10.1029/2008JD011063, 2009.
Rutter, N., Essery, R., Baxter, R., Hancock, S., Horton, M., Huntley, B., Reid, T., and Woodward, J.: Canopy Structure and Air Temperature Inversions Impact Simulation of Sub-Canopy Longwave Radiation in Snow-Covered Boreal Forests, J. Geophys. Res.-Atmos., 128, e2022JD037980, https://doi.org/10.1029/2022JD037980, 2023.
Saccone, P., Morin, S., Baptist, F., Bonneville, J.-M., Colace, M.-P., Domine, F., Faure, M., Geremia, R., Lochet, J., Poly, F., Lavorel, S., and Clément, J.-C.: The effects of snowpack properties and plant strategies on litter decomposition during winter in subalpine meadows, Plant Soil, 363, 215–229, https://doi.org/10.1007/s11104-012-1307-3, 2013.
Safa, H., Krogh, S. A., Greenberg, J., Kostadinov, T. S., and Harpold, A. A.: Unraveling the Controls on Snow Disappearance in Montane Conifer Forests Using Multi-Site Lidar, Water Resour. Res., 57, e2020WR027522, https://doi.org/10.1029/2020WR027522, 2021.
Schweizer, J., Bruce Jamieson, J., and Schneebeli, M.: Snow avalanche formation, Rev. Geophys., 41, 1016, https://doi.org/10.1029/2002RG000123, 2003.
Seidl, R., Thom, D., Kautz, M., Martin-Benito, D., Peltoniemi, M., Vacchiano, G., Wild, J., Ascoli, D., Petr, M., Honkaniemi, J., Lexer, M. J., Trotsiuk, V., Mairota, P., Svoboda, M., Fabrika, M., Nagel, T. A., and Reyer, C. P. O.: Forest disturbances under climate change, Nat. Clim. Change, 7, 395–402, https://doi.org/10.1038/nclimate3303, 2017.
Smyth, E. J., Raleigh, M. S., and Small, E. E.: The Challenges of Simulating SWE Beneath Forest Canopies are Reduced by Data Assimilation of Snow Depth, Water Resour. Res., 58, e2021WR030563, https://doi.org/10.1029/2021WR030563, 2022.
Spandre, P., François, H., Verfaillie, D., Lafaysse, M., Déqué, M., Eckert, N., George, E., and Morin, S.: Climate controls on snow reliability in French Alps ski resorts, Nature Scientific Reports, 9, 8043, https://doi.org/10.1038/s41598-019-44068-8, 2019.
Stark, S., Martz, F., Ovaskainen, A., Vuosku, J., Männistö, M. K., and Rautio, P.: Ice-on-snow and compacted and absent snowpack exert contrasting effects on soil carbon cycling in a northern boreal forest, Soil Biol. Biochem., 150, 107983, https://doi.org/10.1016/j.soilbio.2020.107983, 2020.
Sturm, M. and Liston, K.: Revisiting the Global Seasonal Snow Classification: An Updated Dataset for Earth System Applications, J. Hydrometeorol., 22, 2917–2938, https://doi.org/10.1175/JHM-D-21-0070.1, 2021.
Sturm, M., Holmgren, J., König, M., and Morris, K.: The thermal conductivity of seasonal snow, J. Glaciol., 43, 26–41, https://doi.org/10.3189/S0022143000002781, 1997.
Sullender, B. K., Cunningham, C. X., Lundquist, J. D., and Prugh, L. R.: Defining the danger zone: critical snow properties for predator–prey interactions, Oikos, 2023, e09925, https://doi.org/10.1111/oik.09925, 2023.
SURFEX: SURFEX Home Page, http://www.umr-cnrm.fr/surfex/ (last access: 1 October 2024), 2011.
Teich, M., Giunta, A. D., Hagenmuller, P., Bebi, P., Schneebeli, M., and Jenkins, M. J.: Effects of bark beetle attacks on forest snowpack and avalanche formation – Implications for protection forest management, Forest Ecol. Manage., 438, 186–203, https://doi.org/10.1016/j.foreco.2019.01.052, 2019.
Thackeray, C. W. and Fletcher, C. G.: Snow albedo feedback: Current knowledge, importance, outstanding issues and future directions, Prog. Phys. Geogr.: Earth and Environment, 40, 392–408, https://doi.org/10.1177/0309133315620999, 2016.
Trujillo, E., Ramírez, J. A., and Elder, K. J.: Topographic, meteorologic, and canopy controls on the scaling characteristics of the spatial distribution of snow depth fields, Water Resour. Res., 43, W07409, https://doi.org/10.1029/2006WR005317, 2007.
Tuzet, F., Dumont, M., Lafaysse, M., Picard, G., Arnaud, L., Voisin, D., Lejeune, Y., Charrois, L., Nabat, P., and Morin, S.: A multilayer physically based snowpack model simulating direct and indirect radiative impacts of light-absorbing impurities in snow, The Cryosphere, 11, 2633–2653, https://doi.org/10.5194/tc-11-2633-2017, 2017.
Uchôa da Silva, Y., França, G. B., Ruivo, H. M., and Fraga de Campos Velho, H.: Forecast of convective events via hybrid model: WRF and machine learning algorithms, Appl. Comput. Geosci., 16, 100099, https://doi.org/10.1016/j.acags.2022.100099, 2022.
Vernay, M., Lafaysse, M., Monteiro, D., Hagenmuller, P., Nheili, R., Samacoïts, R., Verfaillie, D., and Morin, S.: The S2M meteorological and snow cover reanalysis over the French mountainous areas: description and evaluation (1958–2021), Earth Syst. Sci. Data, 14, 1707–1733, https://doi.org/10.5194/essd-14-1707-2022, 2022.
Viallon-Galinier, L., Hagenmuller, P., and Lafaysse, M.: Forcing and evaluating detailed snow cover models with stratigraphy observations, Cold Reg. Sci. Technol., 180, 103163, https://doi.org/10.1016/j.coldregions.2020.103163, 2020.
Vincent, L., Lejeune, Y., Lafaysse, M., Boone, A., Le Gac, E., Coulaud, C., Freche, G., and Sicart, J. E.: Interception of snowfall by the trees is the main challenge for snowpack simulations under forests, Proceedings of the International Snow Science Workshop, Innsbruck, Austria, 2018, https://arc.lib.montana.edu/snow-science/objects/ISSW2018_O08.4.pdf#:~:text=Lafaysse et al. (2017). MEB is (last access: 1 October 2024), 6, 2018.
Vionnet, V., Brun, E., Morin, S., Boone, A., Faroux, S., Le Moigne, P., Martin, E., and Willemet, J.-M.: The detailed snowpack scheme Crocus and its implementation in SURFEX v7.2, Geosci. Model Dev., 5, 773–791, https://doi.org/10.5194/gmd-5-773-2012, 2012.
Vionnet, V., Verville, M., Fortin, V., Brugman, M., Abrahamowicz, M., Lemay, F., Thériault, J. M., Lafaysse, M., Milbrandt, J. A.: Snow Level From Post-Processing of Atmospheric Model Improves Snowfall Estimate and Snowpack Prediction in Mountains, Water Resour. Res., 2022, 58, https://doi.org/10.1029/2021WR031778, 2022.
Viviroli, D. and Weingartner, R.: The hydrological significance of mountains: from regional to global scale, Hydrol. Earth Syst. Sci., 8, 1017–1030, https://doi.org/10.5194/hess-8-1017-2004, 2004.
Webb, R. W., Jennings, K. S., Fend, M., and Molotch, N. P.: Combining Ground-Penetrating Radar with Terrestrial LiDAR Scanning to Estimate the Spatial Distribution of Liquid Water Content in Seasonal Snowpacks, Water Resour. Res., 54, 10339–10349, https://doi.org/10.1029/2018WR022680, 2018.
Webster, C., Rutter, N., and Jonas, T.: Improving representation of canopy temperatures for modeling subcanopy incoming longwave radiation to the snow surface, J. Geophys. Res.-Atmos., 122, 9154–9172, https://doi.org/10.1002/2017JD026581, 2017a.
Webster, C., Rutter, N., Zahner, F., and Jonas, T.: Modeling subcanopy incoming longwave radiation to seasonal snow using air and tree trunk temperatures, J. Geophys. Res.-Atmos., 121, 1220-1235, https://doi.org/10.1002/2015JD024099, 2017b.
Webster, C., Mazzotti, G., Essery, R., and Jonas, T.: Enhancing airborne LiDAR data for improved forest structure representation in shortwave transmission models, Remote Sens. Environ., 249, 112017, https://doi.org/10.1016/j.rse.2020.112017, 2020.
Woolley, G. J., Rutter, N., Wake, L., Vionnet, V., Derksen, C., Essery, R., Marsh, P., Tutton, R., Walker, B., Lafaysse, M., and Pritchard, D.: Multi-physics ensemble modelling of Arctic tundra snowpack properties, EGUsphere [preprint], https://doi.org/10.5194/egusphere-2024-1237, 2024.
Zhang, Y., Sherstiukov, A. B., Qian, B., Kokelj, S. V., and Lantz, T. C.: Impacts of snow on soil temperature observed across the circumpolar north, Environ. Res. Lett., 13, 044012, https://doi.org/10.1088/1748-9326/aab1e7, 2018.
Zweigel, R. B., Westermann, S., Nitzbon, J., Langer, M., Boike, J., Etzelmüller, B., and Schuler, T. V.: Simulating Snow Redistribution and its Effect on Ground Surface Temperature at a High-Arctic Site on Svalbard, J. Geophys. Res.-Earth Surf., 126, e2020JF005673, https://doi.org/10.1029/2020JF005673, 2021.