the Creative Commons Attribution 4.0 License.
the Creative Commons Attribution 4.0 License.
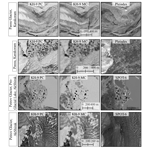
Brief communication: Glacier mapping and change estimation using very high-resolution declassified Hexagon KH-9 panoramic stereo imagery (1971–1984)
Sajid Ghuffar
Owen King
Grégoire Guillet
Ewelina Rupnik
The panoramic cameras (PCs) on board Hexagon KH-9 (KH-9PC) satellite missions from 1971–1984 captured very high-resolution stereo imagery with up to 60 cm spatial resolution. This study explores the potential of this imagery for glacier mapping and change estimation. We assess KH-9PC imagery using data from the KH-9 mapping camera (KH-9MC), KH-4PC, and SPOT and Pléiades satellite imagery. The high resolution of KH-9PC leads to higher-quality DEMs, which better resolve the accumulation region of the glaciers in comparison to the KH-9MC. On stable terrain, KH-9PC DEMs achieve an elevation accuracy of <4 m with respect to SPOT and Pléiades DEMs. While the estimated geodetic mass balances using PC and MC data are similar after outlier filtering, the elevation change data show superior spatial coverage and considerably less noise when using KH-9PC data.
- Article
(4298 KB) -
Supplement
(23827 KB) - BibTeX
- EndNote
Recent studies confirmed that glaciers have been losing mass globally at an accelerated rate over the last 2 decades, and available data indicate global glacier recession at least since the 1960s (Zemp et al., 2019; Hugonnet et al., 2021). The provision of reliable estimates of these long-term changes at regional scales still remains a challenge due to data scarcity. For some regions aerial photos provide the basis for long-term information (Geyman et al., 2022; Korsgaard et al., 2016), but for most regions no historical images exist or are not available. Declassified data from the US satellite reconnaissance program, especially the KH-9 mapping camera (KH-9MC) and the KH-4 panoramic cameras (KH-4PC), have emerged as a key data source for mapping the state of the glaciers from the 1960s to 1980s (Bhattacharya et al., 2021; Dehecq et al., 2020; Zhou et al., 2017; Pieczonka and Bolch, 2015; Bolch et al., 2011). However, poor contrast and texture in this imagery lead to large data gaps in the corresponding elevation datasets, hindering an accurate investigation of glacier changes. The recent availability of declassified very high-resolution (VHR) Hexagon KH-9 panoramic stereo imagery offers further opportunities for improved glacier mapping for the 1970s and 1980s due to its very high spatial resolution and distinct ground coverage.
The US satellite reconnaissance program designed for strategic surveillance during the Cold War had its first successful launch and film recovery in 1960 involving a Corona KH-1 series mission. Parallel to the development of the Corona program with cameras having the highest spatial resolution of 1.8 m, the Gambit-1 KH-7 (1963–1967) and Gambit-3 KH-8 (1966–1984) programs involved the development of a VHR (60–90 cm) camera system to acquire detailed information about specific targets. The Hexagon program, consisting of 20 missions from 1971–1986, aimed to provide Corona-type coverage, together with the high resolution of the Gambit program (NRO, 2011). The main camera system in Hexagon KH-9 missions consisted of two PCs with a 20∘ stereo convergence angle and the highest spatial resolution of around 60 cm (NRO, 1968), while the later missions (1205–1216) also included a frame MC with a nadir-looking configuration capable of stereo and tri-stereo overlaps and a spatial resolution of 6–9 m (Burnett, 2012).
The Corona KH-4PC imagery (declassified in 1995) and Hexagon KH-9MC imagery (declassified in 2002) have played a pivotal role in the estimation of long-term glacier changes (Bhattacharya et al., 2021; Maurer et al., 2019; Bolch et al., 2011). The processing of declassified photographic films requires special considerations due to the presence of film distortions and limited information of camera parameters. The modeling of panoramic imaging geometry further complicates the processing of PC imagery. While the majority of the earlier work on KH-4PC and KH-9MC has been limited in terms of the number of images used or area covered, automated pipelines for both KH-9MC and KH-4PC have recently been proposed, which enables large-scale mapping (Ghuffar et al., 2022; Dehecq et al., 2020; Maurer and Rupper, 2015).
The VHR KH-9PC data, which were declassified more recently (in 2011), consist of more than 670 000 scenes covering the majority of the Earth's land area with multiple acquisitions over most glacierized regions of the world (Fig. S1 in Supplement). While the first 1700 rolls of the KH-9PC imagery were released through the U.S. Geological Survey's (USGS) EarthExplorer in 2015, the scanning of the whole KH-9PC archive and its availability through the USGS is still underway. By the end of February 2023, USGS had scanned about 70 % of the browse images, with the completion aimed at early 2024 (USGS, personal communication, 23 February 2023). The potential for high-resolution DEM generation and mapping using KH-9PC has remained largely unexplored until now (Zhou et al., 2021; Fowler, 2016). The aim of this study is to evaluate the potential of this imagery in the context of glacier mapping and change estimation. We aim to quantify the accuracy of glacier DEMs generated from KH-9PC imagery and assess changes in glacier mass balance and its associated uncertainty.
The KH-9PC system, developed by Pelkin-Elmer, consisted of stereoscopic cameras with a 20∘ convergence angle. The cameras scanned in opposite directions with a maximum scan angle of in the across-track direction. In contrast to Corona KH-4PCs, these cameras had the capability to acquire images in variable scan width and scan center modes. KH-9PC operated with 30, 60, 90 and 120∘ variable scan angles with scan centers of 0, ±15, ±30 and ±45 (Fig. 1). The KH-9PC system had a focal length of 152.4 cm (60 in.) with a folded Wright optical system. The orbital altitude of the KH-9 missions was typically in the range of 160–250 km. This resulted in a best ground resolution of around 60 cm towards the nadir direction. The ground resolution varied within the scan and reached around 3 m towards the 60∘ scan angle (for a 170 km satellite altitude, Fig. 1). The ground coverage for the 120∘ scan was approx. 12 000 km2. However, the distortions at high scan angles were considerable, in addition to the lower ground resolution. Consequently, the later KH-9 missions were restricted to a maximum 45∘ scan angle (NRO, 2011).
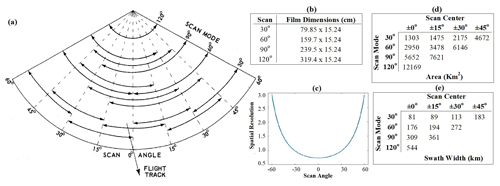
Figure 1(a) The KH-9PC-acquired images at four different scan angles, i.e., 30, 60, 90 and 120∘, along with different scan centers, i.e., ±0, ±15 and . (b) Film dimensions for each scan angle range. (c) Spatial resolution vs. scan angle with scan center at 0∘. (d, e) The area and swath width of the ground footprint for each combination of scan angle and scan center (modified from NRO, 1968).
To evaluate KH-9PC data for glacier mapping and change assessment with respect to contemporary high-resolution satellite imagery, we chose two study sites: the Ak-Shirak area with Petrov Glacier in central Tien Shan and Passu Glacier in central Karakoram (Figs. S2 and S3). The selection of these study sites was based on the availability of KH-9MC DEMs and orthoimages from earlier studies (Pieczonka and Bolch, 2015; Bolch et al., 2017; Goerlich et al., 2017), as well as the availability of contemporary high-resolution satellite stereo imagery over these areas. We compare KH-9PC DEMs with the KH-9MC DEMs from the same satellite overpass using the high-resolution Pléiades and SPOT-6 DEMs (Table S1 in the Supplement). We further include KH-4PC data over Ak-Shirak for comparison. The KH-9PC data consist of two aft images and one fore image for the Ak-Shirak area and vice versa for the Passu area. The KH-9PC images of Ak-Shirak had a scan angle range of −45 to 45∘, while the Passu KH-9PC images had a scan angle range of −60 to 30∘ (Table S1).
To assess the potential of KH-9PC data for large-scale or regional-scale mapping of glacier changes, we utilize the entire swath width of the successive KH-9PC stereo pairs (fore: F049–F052; aft: A050–A052), which contains the image subset used for the Passu Glacier area. We use the 30 m ALOS World DEM AW3D30 (Tadono et al., 2014) for the evaluation of the KH-9PC DEM of the successive stereo pairs and derive estimates of surface elevation change (dH) from the two DEMs to examine the potential for estimating glacier mass balance at a regional extent.
4.1 Processing of KH-9PC imagery
The photographic film of each KH-9PC scene is scanned into several parts by the USGS due to its large size. The film length of KH-9PC scenes depends on the total scan angle, while the width of the film (imaged area) is fixed at 15.24 cm. For a 120∘ scan the film length is 319.4 cm and is scanned into 14 parts with 7 µm resolution (approx. 456 000×22 000 pixels). We stitch individual scans using tie points extracted in the overlapping region of the successive scan parts to generate an image of the entire scan. The scanned film consists of the imaged area, as well as reference data such as the timing marks, scan angle marks and tilting information. We clip the imaged area and align the length and width of the film along the horizontal and vertical axes of the image, while no film-bending estimation and subsequent compensation nor radiometric correction are applied to the image.
To process the KH-9PC imagery, we follow a workflow similar to the Corona Stereo Pipeline (CoSP) using the same set of software and libraries as presented in Ghuffar et al. (2022). CoSP uses a modified form of collinearity equations to model the imaging geometry of the panoramic cameras with a scanning mechanism. This panoramic camera model includes additional parameters to model the motion of the camera during the panoramic image scan, as well as the image motion compensation mechanism. Following the workflow in CoSP, we match feature points between KH-9PC imagery and Landsat 7 ETM+ panchromatic images using the deep-learning model SuperGlue (Sarlin et al., 2020). These feature points along with the corresponding elevation values derived from AW3D30 constitute the ground control points (GCPs), which are used in the estimation of the camera parameters. The initial approximation of the camera parameters is done using the image corner locations given in the metadata of KH-9PC imagery available from the USGS. These approximate camera parameters are then optimized in a bundle adjustment using GCPs and tie points of the stereo pair.
To map the corresponding image points of the stereo pair to the same image row, we use the generic epipolar resampling algorithm presented in Deseilligny and Rupnik (2020). Then, we use the semi-global matching algorithm (Hirschmuller, 2007) for dense matching of the resampled stereo image pair. These dense stereo correspondences are then triangulated to generate a 3D point cloud using the estimated camera parameters. To compensate for the misalignment between the reference DEM and the KH-9PC point cloud, a tile-based coregistration with the reference DEM is performed using a least-squares surface matching algorithm employing a 3D affine transformation (Pfeifer et al., 2014). The coregistered KH-9PC point cloud is then interpolated (using average elevation at each grid cell) to a raster DEM at the resolution of the reference DEM, i.e., 5 m for Passu, 10 m for Ak-Shirak and 30 m for comparison with AW3D30.
4.2 KH-9MC, KH-4PC, SPOT-6 and Pléiades DEMs
A Pléiades DEM (5 m, 2021) of Passu Glacier was generated using MicMac (Pierrot-Deseilligny et al., 2014). We used DEMs of KH-9MC (25 m for Ak-Shirak and 30 m for Passu Glacier), KH-4PC (25 m, 1964) and SPOT-6 (10 m, 2017) generated in earlier studies for DEM evaluation. The KH-9MC images of Ak-Shirak and Passu Glacier were processed in Leica Photogrammetry Suite (Pieczonka and Bolch, 2015) and ERDAS IMAGINE Photogrammetry (Bolch et al., 2017) respectively. The KH-4PC images were processed in Remote Sensing Software Package Graz (Goerlich et al., 2017), while the SPOT-6 DEM of Ak-Shirak was generated using PCI Geomatica (Bhattacharya et al., 2021). The fine coregistration of these DEMs has been performed using the method of Nuth and Kääb (2011), and the biases due to tilt in the DEMs have been removed using polynomial trend surfaces.
4.3 DEM differencing and elevation change post-processing
The coregistered DEMs were differenced from their reference DEMs (i.e., SPOT-6 DEM for Ak-Shirak and Pléiades DEM for Passu). To enable the robust estimation of geodetic glacier mass balance over the Passu and Petrov glaciers, we firstly remove erroneous elevation change (dH) estimates most common in glacier accumulation zones (Fig. 2). We discard dH values outside ±200 m under the assumption that glacier thinning or thickening outside of this range is unlikely. We then further filter the remaining dH data using a threshold of the standard deviation (SD) of dH within 50 m elevation bands of glacier surfaces through the full elevation range of each glacier. We fill the resulting gaps in the dH grids using the mean value of dH from the same 50 m elevation band (McNabb et al., 2019). We convert glacier-wide dH to volume change estimates considering the pixel size of the dH grids and then to mass change using a conversion factor of 850 kg m−3 (Huss, 2013).
The uncertainty associated with geodetic mass balance estimates over Petrov and Passu glaciers was calculated following the approach of Fischer et al. (2015) (following Rolstad et al., 2009), which considers the variance of dH data over stable off-glacier areas as being representative of the uncertainty of dH estimates on-glacier. The calculated correlation length was 605 m in the case of the KH-9PC dH data and 873 m in the case of the KH-9MC data over Passu Glacier. The correlation length was 1488 m in the case of the KH-9PC-derived dH data and 1220 m for the KH-9MC-derived dH data over Petrov Glacier. We include the uncertainty of the density conversion factor proposed by Huss (2013) when converting ice volume to mass changes, and we also follow the approach of Malz et al. (2018) to consider the impact of a changing glacier area on overall glacier mass balance estimates.
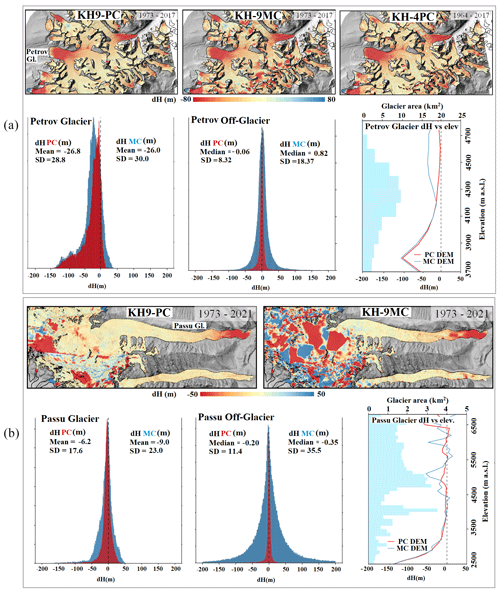
Figure 2(a) dH of KH-9PC (1973), KH-9MC (1973) and KH-4PC (1964) DEMs with the SPOT-6 (2017) DEM over Ak-Shirak. (b) dH of the KH-9PC (1973) and KH-9MC (1973) DEMs with Pléiades (2021) DEM over Passu Glacier. Bottom graphs: the corresponding dH histograms for glacier and off-glacier pixels and change in dH with respect to the elevation. These dH grids and the corresponding histograms show elevation differences without the filtering and gap filling, which is performed before mass balance computation. The filtered versions of the dH grids are available in Fig. S5. The linear feature visible in the dH image over the accumulation zone of Passu Glacier is due to the boundary of successive images having only a small overlap.
The differencing of KH-9PC DEMs with the SPOT-6 and Pléiades DEMs shows a normalized median absolute deviation (NMAD) and 68 % quantile (confidence interval) of less than 4 m over stable terrain (Table 1), while the KH-9MC DEMs show an NMAD of 12.66 m for Ak-Shirak and 27.28 m for the Passu area. Although the KH-9PC DEMs show significantly better accuracy, it should be emphasized that the quality of the KH-9MC and KH-4PC DEMs (Table 1) is also dependent on the DEM generation workflows adopted in the respective studies. The accuracy of the KH-9MC DEMs reported in earlier studies show significant variation due to processing differences (Dehecq et al., 2020; Bolch et al., 2017; Zhou et al., 2017). The best accuracy reported for KH-9MC data is around 5 m (68 %) with respect to the Shuttle Radar Topography Mission (SRTM) DEM using the KH-9MC imagery over the European Alps (Dehecq et al., 2020).
Table 1Mass balance (MB) of Petrov and Passu glaciers using KH-9PC and KH-9MC data and dH statistics of stable terrain between the KH-9 and the contemporary DEMs. The dH statistics over stable terrain from Dehecq et al. (2020)* and Zhou et al. (2017) are given for a comparison. SD is the standard deviation.
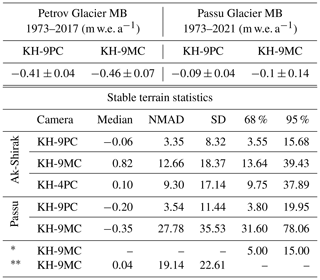
Over Petrov Glacier (Ak-Shirak), the mean thinning estimates after outlier filtering were similar for dH data derived using the KH-9PC (−26.8 m) and KH-9MC (−26.0 m), and the data were similarly dispersed (SD 28.8 m for PC, 30.0 m for MC) (Fig. 2). Over Passu Glacier, the KH-9PC (−6.2 m) and KH-9MC (−9.0 m) again produced similar dH estimates between 1973–2021, whilst the SDs of KH-9PC dH data (17.6 m) were slightly lower than those of the KH-9MC dH data (23.0 m). Unfiltered dH data (Fig. 2) clearly show the extent of glacier accumulation zones affected by low surface contrast in DEM generation, and resulting blunders caused anomalous, high-magnitude (−50 m) dH estimates (Fig. 2) over both study sites. The area affected by such errors was much smaller in the KH-9PC DEM than the KH-9MC DEM. Over Passu Glacier 82.0 % of KH-9PC dH data were retained after filtering, compared to 56.6 % in the case of the KH-9MC dH grid. Over Petrov Glacier, KH-9PC dH data covered 80.2 % of the glacier area following filtering, whereas 68.2 % of KH-9MC dH data were retained.
The mass balance of Petrov Glacier (after outlier filtering and gap filling) was estimated to be m w.e. a−1 for 1973–2017 when using KH-9PC data, while, using KH-9MC data, we estimate the mass balance of the glacier to be m w.e. a−1. The mass balance of Passu Glacier was estimated to be m w.e. a−1 for 1973–2021 based on KH-9PC data, while, using KH-9MC data, the estimated mass balance was m w.e. a−1.
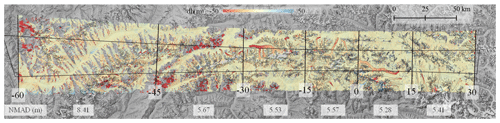
Figure 3Elevation differences using the entire KH-9 panoramic swath width of successive KH-9PC stereo images (D3C1206-200215F049-F52 and D3C1206-200215A050-A052) and the AW3D30 covering parts of the Karakoram and Hindu Kush mountains. The near-vertical lines show approximate 15∘ intervals of the panoramic scan. The NMADs of the elevation differences over stable terrain are shown at the bottom for each 15∘ scan area. The footprint of scene D3C1206-200215A051 is overlaid for reference. The part of the DEM beyond 30 and scan angles has been clipped. Occlusions due to high relief and slant viewing angle cause data gaps between −60 to scan angles, while some data gaps and outliers are due to clouds (see Fig. S4).
5.1 Towards large-scale mapping with KH-9PC
The differencing of the AW3D30 DEM with DEMs derived from successive KH-9PC stereo pairs over the wider Karakoram region surrounding Passu Glacier (using the entire image, i.e., −60 to 30∘ scan angle) shows that the systematic errors are relatively low up to 45∘ scan angle (Fig. 3). The NMAD of elevation differences over stable terrain is around 5.5 m from −45 to 30∘ scan angle, which shows that successive stereo DEMs are well coregistered and consistent with each other. However, the overlap between the successive frames is relatively small, i.e., around 3 % towards the nadir, which may lead to data gaps at the boundary of successive DEMs especially if the boundary falls over a textureless image area. Most moderate to large glaciers require mosaicking of multiple successive KH-9PC DEMs, and fine coregistration and filtering of any boundary artifacts are important to avoid introducing bias in the DEMs.
At higher scan angles (i.e., ) the occlusions (from mountains due to slant viewing angle) increase significantly, and higher perspective distortions lead to systematic bias in the DEM differences due to uncompensated systematic errors (Fig. 3). Even with an improved coregistration and bias and trend correction approach, reliable DEM and orthoimage generation for higher scan angles will be quite challenging especially for mountainous regions. Therefore, the scan angle range of the KH-9PC acquisitions should always be considered when investigating the ground coverage of KH-9PC images.
The accuracy of optical- and stereo-derived elevation data depends in particular on the image resolution, and our results show that KH-9PC data can achieve better accuracy in comparison to KH-9MC and KH-4PC, reducing uncertainties associated with the estimation of glacier surface elevation change. Our results demonstrate the ability of the KH-9PC to better resolve surface conditions in glacier accumulation zones, therefore providing a greater coverage of dH data to be used in the study of glacier accumulation and ablation processes (Figs. 2 and S6). Geodetic studies incorporating KH-9MC data (e.g., Zhou et al., 2017; King et al., 2019; Maurer et al., 2019) report considerable data gaps (up to 40 %), primarily over the higher reaches of glaciers, and therefore provide little information on their accumulation regime over multi-decadal time periods. In combination with contemporary sensors capable of capturing accumulation zone conditions (e.g., Pléiades), KH-9PC data can be used to more completely capture glacier accumulation and ablation processes over longer time periods than is currently possible. In our two case studies, this improved coverage resulted in relatively minor differences in geodetic mass budgets (Table 1), likely because of the effectiveness of outlier filtering and subsequent gap filling techniques in minimizing the impact of erroneous dH estimates on glacier volume change (and therefore mass balance) calculations (e.g., McNabb et al., 2019). Still, the improved coverage afforded by KH-9PC data over the higher reaches of mountain glaciers could be significant when examining processes such as quiescent-phase ice mass build-up over surge-type glaciers or concentrated ice mass accumulation prior to glacier instabilities (Kääb et al., 2018).
The VHR KH-9PC imagery complements the data from KH-9MC and KH-4PC in terms of area coverage and resolution and offers potential for improved glacier mapping capabilities. Although the radiometric resolution of scanned KH-9PC imagery is less compared to Pléiades and SPOT-6 (8 bits vs. 12 bits), the spatial details are quite similar to contemporary VHR satellite imagery (Fig. S7), and therefore KH-9PC has the capability of characterizing small-scale glacier surface features such as supraglacial ponds and ice cliffs. This improves our ability to study the role of these ablative features over longer timescales than is currently possible. The different ground footprint of KH-9PC (Fig. S2) compared to KH-9MC, as well as acquisitions from higher number of KH-9 missions, also enhances the chances of identifying and mapping glacier surge events from the 1970s to 1980s (see Fig. S8, which shows the Hispar Glacier (Karakoram) tributary surge in multi-temporal KH-9PC imagery). The crevasse pattern indicative of surge activity is also recognizable in VHR KH-9PC imagery. In addition, the KH-9PC imagery enhances the potential for the mapping of glacial lake outburst flood events (from the 1970s to 1980s) in high spatial detail (Fig. S8).
The presence of film distortions due to long-term storage poses a limitation in processing historical imagery. The reseau marks in the KH-9MC film enables correction of the film distortion (Pieczonka and Bolch, 2015). However, such a reseau grid is not available in PC imagery due to the distinctive imaging mechanism. To the best of our knowledge, there exists no established methodology for the correction of film distortions in declassified panoramic imagery. In addition, the scanning artifacts have also been reported in the scanned imagery, which may further limit the accuracy of the derived data (Ghuffar et al., 2022; Dehecq et al., 2020).
This study shows that KH-9PC DEMs can achieve accuracy better than 4m over mountainous terrain, which is an improvement on the accuracy reported for KH-9MC and KH-4PC DEMs. The very high resolution and better image quality lead to more reliable elevation estimates towards the accumulation region of the glaciers. The estimated geodetic mass balance using KH-9PC data ( m w.e. a−1 for Passu Glacier and m w.e. a−1 for Petrov Glacier) and KH-9MC data ( m w.e. a−1 for Passu Glacier and m w.e. a−1 for Petrov Glacier) are quite similar, which shows the effectiveness of the outlier filtering approach. However, the uncertainty associated with mass balance estimates is significantly reduced when considering KH-9PC data due to fewer outliers in derived dH data and lower height differences over stable terrain. As cloud-free acquisitions in declassified imagery during late summer to early winter months over glacierized areas are rather limited, the VHR KH-9PC imagery with variable footprint offers multiple benefits in the context of glacier mapping and change estimation.
The DEM differencing grids and orthoimages are available from http://mountcryo.org/datasets (MountCryo, 2023). The KH-9PC (https://doi.org/10.5066/F7WD3Z10; USGS, 2018c), KH-9MC (https://doi.org/10.5066/F74X5684; USGS, 2018a), KH-4 (https://doi.org/10.5066/F78P5XZM; USGS, 2018b) and Landsat 7 ETM+ (https://doi.org/10.5066/P9TU80IG; USGS, 2020) images can be ordered and downloaded from https://earthexplorer.usgs.gov (last access: 14 March 2023; USGS, 2023) and the ALOS data (Tadono, 2014) from https://www.eorc.jaxa.jp/ALOS/en/dataset/aw3d30/aw3d30_e.htm (last access: 17 March 2023; ALOS, 2023).
The supplement related to this article is available online at: https://doi.org/10.5194/tc-17-1299-2023-supplement.
SG and TB designed the study. SG, supported by ER, processed the KH-9 panoramic camera images. OK and GG computed the mass balance and uncertainty estimates. SG, supported by TB, OK and GG, wrote the manuscript. All authors contributed to the final form of the article.
At least one of the (co-)authors is a member of the editorial board of The Cryosphere. The peer-review process was guided by an independent editor, and the authors also have no other competing interests to declare.
Publisher's note: Copernicus Publications remains neutral with regard to jurisdictional claims in published maps and institutional affiliations.
We thank the reviewers and the scientific editor for their constructive comments.
This study was supported by the Swiss National Science Foundation (grant no. 200021E_177652/1) and the Strategic Priority Research Program of the Chinese Academy of Sciences (grant no. XDA20100300).
This paper was edited by Bert Wouters and reviewed by Amaury Dehecq and one anonymous referee.
ALOS: https://www.eorc.jaxa.jp/ALOS/en/dataset/aw3d30/aw3d30_e.htm, last access: 17 March 2023. a
Bhattacharya, A., Bolch, T., Mukherjee, K., King, O., Menounos, B., Kapitsa, V., Neckel, N., Yang, W., and Yao, T.: High Mountain Asian glacier response to climate revealed by multi-temporal satellite observations since the 1960s, Nat. Commun., 12, 1–13, https://doi.org/10.1038/s41467-021-24180-y, 2021. a, b, c
Bolch, T., Pieczonka, T., and Benn, D. I.: Multi-decadal mass loss of glaciers in the Everest area (Nepal Himalaya) derived from stereo imagery, The Cryosphere, 5, 349–358, https://doi.org/10.5194/tc-5-349-2011, 2011. a, b
Bolch, T., Pieczonka, T., Mukherjee, K., and Shea, J.: Brief communication: Glaciers in the Hunza catchment (Karakoram) have been nearly in balance since the 1970s, The Cryosphere, 11, 531–539, https://doi.org/10.5194/tc-11-531-2017, 2017. a, b, c
Burnett, M. G.: Hexagon (KH-9) Mapping Camera Program and Evolution, Center for the Study of National Reconnaissance, https://www.nro.gov/Portals/65/documents/foia/declass/mapping1.pdf (last access: 17 March 2023), 2012. a
Dehecq, A., Gardner, A. S., Alexandrov, O., McMichael, S., Hugonnet, R., Shean, D., and Marty, M.: Automated processing of declassified KH-9 Hexagon satellite images for global elevation change analysis since the 1970s, Front. Earth Sci., 8, 566802, https://doi.org/10.3389/feart.2020.566802, 2020. a, b, c, d, e, f
Deseilligny, M. P. and Rupnik, E.: Epipolar rectification of a generic camera, IPOL Journal, in review, https://doi.org/10.5201/ipol, 2020. a
Fischer, M., Huss, M., and Hoelzle, M.: Surface elevation and mass changes of all Swiss glaciers 1980–2010, The Cryosphere, 9, 525–540, https://doi.org/10.5194/tc-9-525-2015, 2015. a
Fowler, M. J.: The archaeological potential of declassified HEXAGON KH-9 panoramic camera satellite photographs, AARG News, 53, 30–36, 2016. a
Geyman, E. C., van Pelt, J. J., W., Maloof, A. C., Aas, H. F., and Kohler, J.: Historical glacier change on Svalbard predicts doubling of mass loss by 2100, Nature, 601, 374–379, https://doi.org/10.1038/s41586-021-04314-4, 2022. a
Ghuffar, S., Bolch, T., Rupnik, E., and Bhattacharya, A.: A pipeline for automated processing of declassified Corona KH-4 (1962–1972) stereo imagery, IEEE T. Geosci. Remote, 60, 1–14, https://doi.org/10.1109/TGRS.2022.3200151, 2022. a, b, c
Goerlich, F., Bolch, T., Mukherjee, K., and Pieczonka, T.: Glacier mass loss during the 1960s and 1970s in the Ak-Shirak range (Kyrgyzstan) from multiple stereoscopic Corona and Hexagon imagery, Remote Sensing, 9, 275, https://doi.org/10.3390/rs9030275, 2017. a, b
Hirschmuller, H.: Stereo processing by semiglobal matching and mutual information, IEEE T. Pattern Anal., 30, 328–341, https://doi.org/10.1109/TPAMI.2007.1166, 2007. a
Hugonnet, R., McNabb, R., Berthier, E., Menounos, B., Nuth, C., Girod, L., Farinotti, D., Huss, M., Dussaillant, I., Brun, F., and Kääb, A.: Accelerated global glacier mass loss in the early twenty-first century, Nature, 592, 726–731, https://doi.org/10.1038/s41586-021-03436-z, 2021. a
Huss, M.: Density assumptions for converting geodetic glacier volume change to mass change, The Cryosphere, 7, 877–887, https://doi.org/10.5194/tc-7-877-2013, 2013. a, b
Kääb, A., Leinss, S., Gilbert, A., Bühler, Y., Gascoin, S., Evans, S. G., Bartelt, P., Berthier, E., Brun, F., Chao, W.-A., Farinotti, D., Gimbert, F., Guo, W., Huggel, C., Kargel, J. S., Leonard, G. J., Tian, L., Treichler, D., and Yao, T.: Massive collapse of two glaciers in western Tibet in 2016 after surge-like instability, Nat. Geosci., 11, 114–120, https://doi.org/10.1038/s41561-017-0039-7, 2018. a
King, O., Bhattacharya, A., Bhambri, R., and Bolch, T.: Glacial lakes exacerbate Himalayan glacier mass loss, Sci. Rep.-UK, 9, 1–9, https://doi.org/10.1038/s41598-019-53733-x, 2019. a
Korsgaard, N. J., Nuth, C., Khan, S. A., Kjeldsen, K. K., Bjørk, A. A., Schomacker, A., and Kjær, K. H.: Digital elevation model and orthophotographs of Greenland based on aerial photographs from 1978–1987, Sci. Data, 3, 1–15, https://doi.org/10.1038/sdata.2016.32, 2016. a
Malz, P., Meier, W., Casassa, G., Jaña, R., Skvarca, P., and Braun, M. H.: Elevation and mass changes of the Southern Patagonia Icefield derived from TanDEM-X and SRTM data, Remote Sensing, 10, 188, https://doi.org/10.3390/rs10020188, 2018. a
Maurer, J. and Rupper, S.: Tapping into the Hexagon spy imagery database: A new automated pipeline for geomorphic change detection, ISPRS J. Photogramm., 108, 113–127, https://doi.org/10.1016/j.isprsjprs.2015.06.008, 2015. a
Maurer, J. M., Schaefer, J., Rupper, S., and Corley, A.: Acceleration of ice loss across the Himalayas over the past 40 years, Sci. Adv., 5, eaav7266, https://doi.org/10.1126/sciadv.aav7266, 2019. a, b
McNabb, R., Nuth, C., Kääb, A., and Girod, L.: Sensitivity of glacier volume change estimation to DEM void interpolation, The Cryosphere, 13, 895–910, https://doi.org/10.5194/tc-13-895-2019, 2019. a, b
MountCryo: Data, http://mountcryo.org/datasets, last access: 17 March 2023. a
NRO: Prelimnary KH-9 Camera Manual, Tech. rep., In National Reconnaissance Office Data Book, https://www.nro.gov/Portals/65/documents/foia/declass/ForAll/101917/F-2017-00094b.pdf (last access: 6 June 2022), 1968. a, b
NRO: Critical to US Security, The Gambit and Hexagon satellite reconnaissance systems, Tech. rep., In National Reconnaissance Office Data Book, https://www.nro.gov/Portals/65/documents/history/csnr/gambhex/Docs/Critical to US Security.pdf (last access: 6 June 2022), 2011. a, b
Nuth, C. and Kääb, A.: Co-registration and bias corrections of satellite elevation data sets for quantifying glacier thickness change, The Cryosphere, 5, 271–290, https://doi.org/10.5194/tc-5-271-2011, 2011. a
Pfeifer, N., Mandlburger, G., Otepka, J., and Karel, W.: OPALS–A framework for Airborne Laser Scanning data analysis, Computers, Environment and Urban Systems, 45, 125–136, https://doi.org/10.1016/j.compenvurbsys.2013.11.002, 2014. a
Pieczonka, T. and Bolch, T.: Region-wide glacier mass budgets and area changes for the Central Tien Shan between 1975 and 1999 using Hexagon KH-9 imagery, Global Planet. Change, 128, 1–13, https://doi.org/10.1016/j.gloplacha.2014.11.014, 2015. a, b, c, d
Pierrot-Deseilligny, M., Jouin, D., Belvaux, J., Maillet, G., Girod, L., Rupnik, E., Muller, J., Daakir, M., Choqueux, G., and Deveau, M.: Micmac, apero, pastis and other beverages in a nutshell, Institut Géographique National, 2014. a
Rolstad, C., Haug, T., and Denby, B.: Spatially integrated geodetic glacier mass balance and its uncertainty based on geostatistical analysis: application to the western Svartisen ice cap, Norway, J. Glaciol., 55, 666–680, https://doi.org/10.3189/002214309789470950, 2009. a
Sarlin, P.-E., DeTone, D., Malisiewicz, T., and Rabinovich, A.: Superglue: Learning feature matching with graph neural networks, in: Proceedings of the IEEE/CVF Conference on Computer Vision and Pattern Recognition, 13–19 June 2020, Los Alamitos, CA, USA, IEEE Computer Society, 4938–4947, https://doi.org/10.1109/CVPR42600.2020.00499, 2020. a
Tadono, T., Ishida, H., Oda, F., Naito, S., Minakawa, K., and Iwamoto, H.: Precise global DEM generation by ALOS PRISM, ISPRS Annals of the Photogrammetry, Remote Sensing and Spatial Information Sciences, II4, 71–76, https://doi.org/10.5194/isprsannals-II-4-71-2014, 2014. a
USGS: USGS EROS Archive – Declassified Data – Declassified Satellite Imagery – 2, USGS [data set], https://doi.org/10.5066/F74X5684, 2018a. a
USGS: USGS EROS Archive – Declassified Data – Declassified Satellite Imagery – 1, USGS [data set], https://doi.org/10.5066/F78P5XZM, 2018b. a
USGS: USGS EROS Archive – Declassified Data – Declassified Satellite Imagery – 3, USGS [data set], https://doi.org/10.5066/F7WD3Z10, 2018c. a
USGS: USGS EROS Archive – Landsat Archives – Landsat 7 Enhanced Thematic Mapper Plus Collection 2 Level-1 Data, USGS [data set], https://doi.org/10.5066/P9TU80IG, 2020. a
USGS: EarthExplorer, https://earthexplorer.usgs.gov, last access: 14 March 2023. a
Zemp, M., Huss, M., Thibert, E., Eckert, N., McNabb, R., Huber, J., Barandun, M., Machguth, H., Nussbaumer, S. U., Gärtner-Roer, I., Thomson, L., Paul, F., Maussion, F., Kutuzov, S., and Cogley, J. G.: Global glacier mass changes and their contributions to sea-level rise from 1961 to 2016, Nature, 568, 382–386, https://doi.org/10.1038/s41586-019-1071-0, 2019. a
Zhou, Y., Li, Z., and Li, J.: Slight glacier mass loss in the Karakoram region during the 1970s to 2000 revealed by KH-9 images and SRTM DEM, J. Glaciol., 63, 331–342, https://doi.org/10.1017/jog.2016.142, 2017. a, b, c, d
Zhou, Y., Chen, G., Qiao, X., and Lu, L.: Mining High-Resolution KH-9 Panoramic Imagery to Determine Earthquake Deformation: Methods and Applications, IEEE T. Geosci. Remote, 60, 4506012, https://doi.org/10.1109/TGRS.2021.3116441, 2021. a