the Creative Commons Attribution 4.0 License.
the Creative Commons Attribution 4.0 License.
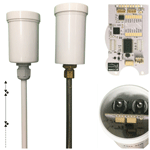
A distributed temperature profiling system for vertically and laterally dense acquisition of soil and snow temperature
Stijn Wielandt
John Lamb
Patrick McClure
Ian Shirley
Sebastian Uhlemann
Chen Wang
Sylvain Fiolleau
Carlotta Brunetti
Franklin H. Akins
John Fitzpatrick
Samuel Pullman
Robert Busey
Craig Ulrich
John Peterson
Susan S. Hubbard
Measuring soil and snow temperature with high vertical and lateral resolution is critical for advancing the predictive understanding of thermal and hydro-biogeochemical processes that govern the behavior of environmental systems. Vertically resolved soil temperature measurements enable the estimation of soil thermal regimes, frozen-/thawed-layer thickness, thermal parameters, and heat and/or water fluxes. Similarly, they can be used to capture the snow depth and the snowpack thermal parameters and fluxes. However, these measurements are challenging to acquire using conventional approaches due to their total cost, their limited vertical resolution, and their large installation footprint. This study presents the development and validation of a novel distributed temperature profiling (DTP) system that addresses these challenges. The system leverages digital temperature sensors to provide unprecedented, finely resolved depth profiles of temperature measurements with flexibility in system geometry and vertical resolution. The integrated miniaturized logger enables automated data acquisition, management, and wireless transfer. A novel calibration approach adapted to the DTP system confirms the factory-assured sensor accuracy of ±0.1 ∘C and enables improving it to ±0.015 ∘C. Numerical experiments indicate that, under normal environmental conditions, an additional error of 0.01 % in amplitude and 70 s time delay in amplitude for a diurnal period can be expected, owing to the DTP housing. We demonstrate the DTP systems capability at two field sites, one focused on understanding how snow dynamics influence mountainous water resources and the other focused on understanding how soil properties influence carbon cycling. Results indicate that the DTP system reliably captures the dynamics in snow depth and soil freezing and thawing depth, enabling advances in understanding the intensity and timing in surface processes and their impact on subsurface thermohydrological regimes. Overall, the DTP system fulfills the needs for data accuracy, minimal power consumption, and low total cost, enabling advances in the multiscale understanding of various cryospheric and hydro-biogeochemical processes.
Temperature is a key property for understanding and quantifying a multitude of processes occurring in and across the deep subsurface, soil, snow, vegetation, and atmosphere compartments of our Earth (e.g., Dingman, 2014; García et al., 2018). In addition to being a manifestation of thermal energy modulated by the heterogeneity of a given medium's thermal parameters, temperature influences a myriad of above- and belowground processes, including aboveground biological dynamics, energy–water exchanges, subsurface heat and water fluxes, soil and root biogeochemical processes, and cryospheric processes (e.g., Chang et al., 2021; Davidson and Janssens, 2006; Jorgenson et al., 2010; Natali et al., 2019). The predictive understanding of the above-mentioned processes across a large range of gradients in topography, air mass exposure, geology, soil type, and vegetation cover requires reliable measurement of the spatial and temporal distribution of snow and/or soil temperature (e.g., Lundquist et al., 2019; Strachan et al., 2016).
The acquisition of time series of soil temperature data has been crucial for improving the understanding of a range of ecosystem properties and processes. For example, temperature time series have been used to explore the control that climate and subsurface properties have over permafrost dynamics (Brewer, 1958; Jorgenson et al., 2010), biogeochemical fluxes (Reichstein and Beer, 2008), plant function and root growth (Iversen et al., 2015), species and community distribution (Myers-Smith et al., 2011), and heat and water fluxes (Cable et al., 2014). Further, many studies have relied on temperature data to determine the water vertical flow velocity (Bredehoeft and Papaopulos, 1965; Briggs et al., 2014; Constantz, 2008; Hatch et al., 2006; Irvine et al., 2020; Racz et al., 2012) or to quantify the soil thermal parameters and, in some cases, the fraction of soil constituents including organic matter content (Beardsmore et al., 2020; Nicolsky et al., 2009; Tabbagh et al., 2017; Tran et al., 2017; Zhu et al., 2019). Similarly, other studies have used vertically resolved temperature measurements in snow to infer snow depth (e.g., Reusser and Zehe, 2011) and snow thermal diffusivity (e.g., Oldroyd et al., 2013) and improve the predictive understanding of snowpack dynamics in general (e.g., Reusser and Zehe, 2011). In addition, the value of capturing the spatial variability in soil temperature has been recognized through organizing networks of temperature measurements (e.g., Biskaborn et al., 2015; Cable et al., 2016), as well as sequentially moving instruments to tens to thousands of locations across the landscape (Cartwright, 1968). Sequential acquisition of soil temperature down to a depth where thermal anomalies are larger than the effect of diurnal fluctuation has been done in volcanic and hydrothermal areas to delineate thermal anomalies and in some cases calculate ground fluxes (e.g., Hurwitz et al., 2012; Lubenow et al., 2016; Saba et al., 2007), as well as in discontinuous permafrost environments to identify near-surface permafrost (e.g., Léger et al., 2019).
Sensing soil or snow temperature at multiple depths and locations requires sensing devices designed to jointly optimize the measurement accuracy, the autonomous data collection with high temporal frequency at a low power consumption, the ability to withstand rough environmental stresses and limit the disturbance of the sensed environment, and the system footprint and total data cost (including material, deployment, and management) for duplicability. Though several tools have been developed to address one or several of the above-mentioned requirements, their characteristics limits their applicability beyond vertically resolved temperature measurements at a limited number of spatially distributed locations or at numerous locations but with poor vertical resolution. Examples of currently available tools include (1) point-scale arrays of self-logging temperature sensors aligned inside a pipe (Constantz et al., 2002; Naranjo and Turcotte, 2015; Rau et al., 2010); (2) point-scale arrays of thermocouple, thermistor, or digital sensors wired into a single electronic data-logging device (Cable et al., 2016; Constantz et al., 2002; Léger et al., 2019); and (3) fiber-optic distributed temperature sensing that measures temperature at various locations and depths (Briggs et al., 2012; Vogt et al., 2010). While the cost of traditional temperature point sensors can be considered low (in the range of USD 1 to 150), the total cost using the point-scale sensor methods – including the data logger, packaging, installation, localization, and management – increase quickly and limit extensive installations. Various efforts have concentrated on improving the packaging of sensors to ease data collection (Fanelli and Lautz, 2008; Gordon et al., 2013; Rau et al., 2010; Tonina et al., 2014), still without fundamentally overcoming other limitations. Recent developments, including custom vertically resolved probes linked to commercial (Aguilar et al., 2018; Andújar Márquez et al., 2016; Naranjo and Turcotte, 2015) or in-house loggers (Beardsmore et al., 2020; Léger et al., 2019), as well as some commercially available systems, are still limited in their vertical resolution, flexibility, and cost effectiveness for wide deployment. While fiber-optic-based methods have been widely applied for temperature measurement in deep wells, infrastructures, and streambeds (Briggs et al., 2012), their deployment for shallow and vertically resolved depth profiling of temperature is still challenging (Lundquist and Lott, 2008). Finally, it can be noted that the absence of systems to efficiently map soil thermal regimes at hundreds of locations has been recognized by several studies that have either relied on conventional thermocouple probes (≤ 25 cm) (e.g., Leon et al., 2014; Lubenow et al., 2016; Price et al., 2017) or developed their own acquisition devices that are costly to duplicate (Hurwitz et al., 2012; Léger et al., 2019).
Mapping or monitoring depth-resolved profiles of soil or snow temperature, as well as the scientific insights anticipated from data having much higher spatiotemporal resolution than currently possible, requires advances in flexible, affordable, and community-available temperature profiling systems, with custom hardware, software, and packaging, enabling optimized power consumption, accuracy, resolution, data transfer, and data management. In fact, while the “Vs”' (velocity, volume, variety, value, and veracity) scores (Demchenko et al., 2013) of temperature measurements in a “big-data” era are presumably very high in comparison to other measurements, there is room for significant improvements. This potential is mainly a result of recent advances in semiconductor technology, allowing for miniaturized digital temperature sensors with an unprecedented cost, accuracy, resolution, stability, and power consumption. Increasing the temperature Vs for mapping and monitoring soil or snow temperature in the Earth sciences promises to improve our ability to capture ecosystem dynamics across a large range of gradients in landscape properties. “Vs' improvement” would in turn improve data- or model-based prediction of heat and water fluxes at multiple scales, reduce uncertainty in the prediction of biogeochemical processes influenced by thermal and hydrological regimes, and move the community toward near-real-time predictions of hydro-biogeochemical processes using data streamed from the field. While recent technological advances in low-cost and low-power digital sensors facilitate the development of inexpensive and customizable platforms, including sensors and loggers, microcontrollers, and communication modules, efforts are still needed to integrate low-cost sensors and loggers for increasing spatial coverage and facilitating new insights into environmental process dynamics.
The objective of this study is to design and develop a distributed temperature profiling (DTP) system that will be suitable for characterizing and/or monitoring vertically resolved profiles of snow and soil temperature at an unparalleled number of locations. In particular, this development is aimed at building a path toward advancing snow or soil temperature measurements at multiple locations for various purposes, including (1) quantifying snow depth and snowpack dynamics, (2) inferring soil thermal metrics (e.g., thawed-layer thickness), (3) estimating soil thermal parameters and/or heat/water fluxes using data and physically based models, (4) developing proxies to facilitate the transfer of knowledge from intensive but sparsely distributed sites to sites where only a subset of variables are measured, and (5) integrating ground-based data with remote sensing products for the improved mapping of hydro-biogeochemical properties. To potentially fulfill the above goals, we hypothesize that measuring soil and snow temperature with unprecedented vertical and lateral resolution and relatively high accuracy (<0.05 ∘C) can become feasible with the development of a novel DTP system. Although an earlier prototype of a DTP system (Léger et al., 2019) offered a new paradigm in sequentially acquiring vertically resolved soil temperature measurements across the landscape, its limited accuracy of 0.15 ∘C, the time required to assemble the system, and the high power consumption and footprint of the connected Raspberry Pi-based logger limited its wide applicability for mapping soil temperature and impeded its use for autonomous monitoring of soil or snow temperature.
In this study, we designed and field-tested a DTP system that enables (1) the customized deployment of probes with flexibility in assembling systems of different length, housing, vertical resolution, and accuracy, depending on the subsurface phenomena being sensed; (2) durability, specifically the ability to withstand rough environmental stresses; and (3) the dense acquisition of measurements by minimizing the total cost (including the costs of material, construction, deployment, and data management) and device footprint. An additional important step in this study for limiting the device cost and footprint is the design of a miniaturized, low-power logger with wireless connectivity for downloading data and setting up acquisition parameters, allowing for possible future integration within a LoRa (long-range) wireless sensor network (Wielandt and Dafflon, 2020). In the following, we first describe the design and components of the newly developed DTP system, providing sufficient detail for others to build a DTP system. Then we present a new, lab-based calibration approach to assess and, if desired, improve the DTP sensor accuracy. In addition, we assess the specifics of the developed system using numerical modeling, and we demonstrate its applications in two field cases: to measure snow and soil temperature and to infer snow depth and soil thawed- and frozen-layer thickness. Finally, we discuss the system's advantages and limitations.
2.1 DTP system hardware and connectivity
We designed a system composed of digital temperature sensors mounted on an array of cascaded printed circuit boards (PCBs) connected to a custom-designed low-power logger. The sequentially addressable digital temperature sensors (TMP117AIDRVR) are low cost, low power, and high accuracy, with a resolution of 0.0078125 ∘C and a factory-assured, National Institute of Standards and Technology-traceable (NIST) accuracy of ± 0.1 ∘C across a temperature range of −20 to 50 ∘C (http://www.ti.com/lit/ds/symlink/tmp117.pdf, last access: 25 February 2022). All sensors on the probe are connected to the data logger's two-wire interface (TWI, also known as I2C), and each sensor is accompanied by a discrete D flip-flop. The arrangement of these flip-flops constitutes a shift register that propagates an address bit along the probe, sequentially enabling each sensor. This approach enables a readout of an arbitrary number of sensors with just six signals (3.3 V supply, ground, TWI clock, TWI data, address, and address clock). The board-to-board connections between probe sections rely on custom-designed press-fit PCB connectors to ensure lasting structural stability and electrical contact under mechanical and thermal stress in the field. Once assembled, each PCB section is 20 cm long and contains two or four temperature sensors to enable 10 or 5 cm spacing, respectively. The upper PCB section is connected to a press-fit wire-to-board adaptor to link the entire probe assembly to the logger. The electrical design of the boards minimizes capacitive loading and crosstalk of the communication signals. In combination with a TCA9803 TWI bus buffer, this allows for sensor arrays over 2 m long, without affecting signal integrity. The entire temperature probe is powered down in between measurements, resulting in a 0.0 µA idle current and a reduced impact of electrical failures along the probe. A measurement of 16 sensors along the probe takes 100 ms and requires up to 220 µA per TMP117AIDRVR.
The logger is a custom-designed embedded system built around a low-power wireless system on a chip (NRF52832 ARM Cortex M4) that enables Bluetooth Low Energy (BLE) connectivity. On-board provisions include a TCA9803 TWI buffer, a load switch, a TMP117AIDRVR temperature sensor, 32 MB of low-power NOR flash memory for storing measurement data, a temperature-compensated real-time clock (RTC, i.e., PCF2129AT) for accurate timekeeping and generating watchdog and measurement interrupts, multiple connectors for existing and future sensor expansion, and an RFM95W LoRa modem for future integration in LoRa wireless sensor networks (Wielandt and Dafflon, 2020). The system operates in the 1.8–3.6 V range, allowing for operation with two AA batteries without requiring further power supply circuitry. The microcontroller and its peripherals are mostly asleep, drawing a system idle current of 7.085 µA. Taking regular BLE advertising and a 15 min sensor-measurement interval for 16 sensors into account, the total system's current consumption averages at 22 µA. Using Energizer L91 batteries (https://data.energizer.com/pdfs/l91.pdf, last access: 25 February 2022) with a 3500 mA h capacity, a total battery lifetime of 18 years can be reached in theory. With each measurement taking up 6 B + 2 B per sensor, the above described probe with 16 sensors would have sufficient memory for 3 years of measurements.
Logger parameters (measurement interval, time, etc.) and on-board stored data are managed using BLE connectivity and a custom companion app for Android devices. The app provides a list of nearby probe identifiers ranked by their Bluetooth signal strength, which usually correlates to the distance from the Android device. The app allows a user to erase logger memory, reset the system, synchronize the on-board clock, set a logging interval, transfer data, and assign GPS coordinates through the phone's GPS. Current data transfer speed is ∼60 kb s−1, which means that 2 weeks of data are downloaded every second (assuming a 15 min sampling interval and 16 connected sensors). The transferred data are converted into a .csv format.
2.2 DTP system assembly and deployment
The probe is built by cascading sensor boards to the desired length and inserting the sensor assembly into a tube, which is then further filled with a sealing urethane mixture and connected to a logger and its enclosure. Different types of tubes and connections to the logger can be used, based on the application. The default tube is a in. (∼10 mm) outer diameter (OD) and in. (∼6 mm) inner diameter (ID) white-colored cellulose acetate butyrate (CAB) plastic tube that is flexible, UV resistant, high albedo, and structurally stable in cold and warm temperatures. Alternatives include a in. (∼10 mm) OD and in. (∼6 mm) ID 304 stainless-steel tube or a (∼12 mm) OD and in. (∼6 mm) ID CAB tube. A cable gland is tightened and – if necessary – glued on the top of each tube before inserting the sensor assembly. Then, the tube is filled from the bottom with a urethane blend using a syringe to reduce the chance of air bubbles. The urethane blend (20-2360 from Epoxies Inc., https://www.epoxies.com/_resources/common/bulletins/20-2360R.pdf, last access:25 February 2022) is a thermosetting mixture designed for electrical potting applications over a temperature range of −40 to 125 ∘C and has a measured thermal conductivity of 0.191 W m−1 K−1. Its coefficient of thermal expansion () and high tensile strength (400 psi; pounds per square inch; 2.758×106 Pa) limit the risk for the probe to warp or snap under a large range of thermal or physical conditions. A in. (∼10 mm) OD metal spike is added at the bottom of the tube to act as a stopper while the urethane mixture sets, ease ground entry during deployment, and enable some electrical grounding with the use of a grounding wire attached to the bottom of the sensor assembly. A 4 oz (∼120 mL) polypropylene (PP) jar can be mounted to the top of the probe and serves as a UV-resistant and dust- and splash-proof enclosure for the data logger (Fig. 1). Depending on the application, sealant can be applied on the jar seams to achieve long-term waterproofing and submergibility.
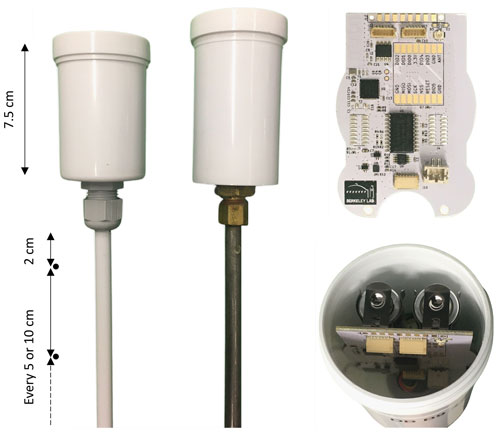
Figure 1General overview of the DTP system. The DTP system can be assembled in various lengths with temperature sensors every 5 or 10 cm along the probe and packaged in a plastic or stainless-steel tube, depending on deployment goals and environmental conditions. The data logger controls the temperature sensors on the probe, sequentially reading and storing the temperature data. An Android app is used to communicate with the logger and download data wirelessly.
The cost of materials for the default DTP system, including the logger, can be as low as USD 95 for a 1.2 m long probe with 16 temperature sensors, assuming a batch size of ∼300 probes. The cost is distributed between the logger components and manufacturing (USD 19), the batteries (USD 2), the CAB tube (USD 4), the logger enclosure (USD 1), the urethane mixture (USD 2), the cable glands (USD 2), and the sensor boards (USD 65) which include the cost of USD 2.50 per TMP117 sensor. A 304 stainless-steel probe implies an additional USD 20, distributed between the stainless-steel tube (USD 10) and the brass tube fitting (USD 10). The above-mentioned cost of the logger and sensor boards is only obtained under optimal factory yields and strongly depends on choices and fluctuations in component and PCB manufacturing prices. In sub-optimal conditions, additional costs can easily add up to ∼ USD 120 per probe. In addition, these price estimates do not consider the cost of the mechanical assembly of the various sensor boards and logger into their final housing. Under ideal conditions (i.e., the assembling of a large batch with adequate equipment), a person can assemble a probe in less than 15 min.
The field deployment of the DTP system can be performed in various ways, depending on the probe housing and application. For plastic probes, a custom-length drill bit with the same diameter of the probe is used to drill a guide hole in which the probe is then inserted. The probe can be inserted completely into the ground, or part of it can be left above ground (Fig. 1). Aboveground installation of the DTP system for snow temperature measurement is done by attaching the probe to a PVC or wood stake using low-temperature-resistant zip ties with a 1 cm plastic tube spacer attached at mid-distance between consecutive temperature sensors.
2.3 DTP sensor accuracy assessment and calibration
A procedure was developed to evaluate the accuracy of a sensor marketed with a factory-assured NIST-traceable accuracy of ±0.1 ∘C and possibly to improve its accuracy with an additional calibration procedure. The most common method for calibrating a temperature sensor consists of a single point calibration where a sensor is submerged in an ice bath, made by saturating 2–3 mm particles of shaved or crushed ice in distilled water and allowing the mixture to equilibrate (Mangum, 1995). If carefully prepared, the latent heat of fusion, which is needed for the phase change, stabilizes the bath within a few ten-thousandths (∼0.01) of 0 ∘C (Thomas, 1938). Cable et al. (2016) used this calibration approach to increase the accuracy of thermistors from 0.1 to approximately 0.02 ∘C for subsurface temperature measurements. While temperature-controlled water baths at temperatures above 0 ∘C (Aguilar et al., 2018; Naranjo and Turcotte, 2015) can be used for calibration using a reference thermometer, reaching an accuracy of 0.01 ∘C is challenging.
While the standard ice-bath approach is adapted for calibration of individual sensors or a string of sensors, initial tests performed in this study did not provide satisfactory results when scaling up this approach to submerge an entire 1.2 m long DTP system. Initial tests were conducted by filling a 1.5 m long 25 cm diameter pipe with a mixture of cool distilled water and cold crushed ice. The DTP system was centered in the pipe with a Fluke reference thermometer (Fluke 1524) collocated next to one of the DTP sensors for additional comparison. Results have shown that building a fine mixed water–ice bath at that scale was time-consuming, not always successful because of the difficulty of having a well homogenized mixture in such a large volume, and thus not adequate for calibrating hundreds of DTP systems.
In this study, a novel 0 ∘C point calibration approach was developed to calibrate tens of probes in one single run while achieving accuracy similar to the ice-bath method. Our approach includes cycling through water–ice phase changes in a 1.5 in. diameter tube filled with cold distilled water and with the DTP probe suspended at its center and logging every minute. Multiple probes and tubes are placed into an incubator (Thermo Scientific Precision Incubator) at −5 ∘C over a period of 12–24 h to ensure frozen conditions and then moved into an incubator at 3 ∘C until melting is complete. The average offset of the measured temperature from 0 ∘C occurring at the melting point due to the latent heat of fusion (heat-induced zero curtain) is extracted from the data for each individual sensor.
2.4 Assessment of controls on heat transfers
Numerical experiments were performed to evaluate the impact of various DTP characteristics and environmental factors on DTP measurement accuracy, beyond the sole sensor accuracy. In particular, we investigated how the temperature measurements are potentially impacted by the probe tubing material and diameter, different ground and probe surface heating, the air gap between the probe and soil, sensor positioning error, and variable soil thermal diffusivity.
A finite volume numerical model on an axis-symmetric cylindrical grid was developed to simulate heat transfer through conduction, in and between the probe and the soil. The thermal conductivity and heat capacity were explicitly represented in the model. Temperature in each cell was updated in time by summing the contributing heat flow across each cell boundary, and stability was controlled by heuristically reducing the time step to between 10−4 and 10−1 s. The model spanned across 50 cm and 50 cells vertically and 5 cm and 100 cells radially and was parameterized with the thermal conductivity and heat capacity of the probe and soil. The initial conditions and the moving Dirichlet boundary conditions at the top and bottom were calculated using the analytical solution for diurnal heat transfer in the half plane (Turcotte and Schubert, 2002). Boundary conditions at the outside edges were similarly obtained with the analytical solution using the ghost point method. The internal boundary condition at zero radius was treated as a zero-flux Neumann boundary condition for symmetry (Langtangen and Linge, 2017, p. 251). Validation of the numerical model was carried out by applying a naive T=0 Dirichlet boundary condition at the outside and bottom of a homogeneous domain and a sinusoidal forcing function at the upper surface. The simulated temperatures closely matched the analytical solution. For the numerical experiments, the simulated temperatures inside the probe using the finite volume model were compared to the analytical solution. The differences were evaluated through the percentage mismatch and time delay in amplitude.
2.5 Autonomous estimation of soil and snow properties
Vertically resolved measurements of soil and snow temperature can be used to infer various properties, including soil thermal parameters, snow depth, zero-curtain duration, first bare-ground date, frozen- and thawed-layer thickness, and many empirical indices. In this study, we evaluate the value of the DTP system to autonomously estimate snow depth, soil frozen, and thawed-layer thickness, as well as the possible probe upward displacement relative to soil surface that can occur in frost-susceptible soil using acquired temperature measurements.
2.5.1 Snow depth
The snow depth can be estimated from a vertically resolved temperature probe placed above the ground surface by identifying where the maximum reduction of the diurnal temperature variation occurs along the vertically resolved profile (Oldroyd et al., 2013; Reusser and Zehe, 2011). This maximum reduction occurring at the air–snow interface is caused by the insulating effect of the snow. In this study, we use a numerical approach relatively similar to the one presented in Reusser and Zehe (2011). Reusser and Zehe (2011) demonstrated their approach by placing nine HOBO pendant temperature data loggers on a square metal rod with a spacing of 15 cm covering a range from 0 to 120 cm above ground. Deploying this instrumentation at five locations, they found that the resulting time series of snow height was in good agreement with their reference measurements done using ultrasonic sensors. The mean absolute error between both types of measurements was 6 cm, which corresponds to the expected minimum error for their setup, where the temperature sensor spacing was 15 cm.
Our algorithm to retrieve snow depth from the DTP system consists of (1) calculating the gradient in temperature between each pair of consecutive sensors along the probe at each sampling time (15 min per default), (2) disregarding pairs where both members indicate one or more temperature measurements >2 ∘C during a 24 h window centered around the sampling time or where the temperature range is larger in the lowest sensor of the pair, (3) selecting the pair with the maximum range in gradient over the 24 h window and assigning the snow depth estimate to the lowest sensor in the pair, and (4) selecting only the solution where the obtained snow depth corresponded to the mode value in the preceding or following 6 h. The second and fourth steps are intended to avoid the possible occurrence of isolated suspicious estimates when the temperature diurnal variation is very small. The developed approach is relatively similar to the one presented by Reusser and Zehe (2011), with the major difference being that they relied on the maximum change in standard deviation over depth instead of the maximum range in gradient.
2.5.2 Frozen- and thawed-layer thickness and probe heave
Frozen- and thawed-layer thickness can be inferred from vertically resolved temperature measurements by extracting the 0 ∘C isotherm in the temperature time series during the freezing and thawing period, respectively. The accuracy of the estimated frozen- or thawed-layer thickness depends on the vertical resolution of the DTP probe, the true freezing point of the material, the accuracy of the temperature measurement, and the positioning of the DTP probe relative to the soil surface. The possible movement of the probe relative to the ground surface over time, which can result from soil mechanical processes or animal disturbance, is obviously the source of uncertainty that is the most difficult to assess. For example, a common concern in the Arctic is that the sensor, stake, or probe can rise upward relative to the soil surface elevation, due to frost jacking or soil frost heave or thaw settlement processes (Iwahana et al., 2021; Johnson and Hansen, 1974; Matsuoka, 1994). This potential upward displacement of the object or material in freeze/thaw cycles depends on various environmental factors, is difficult to predict, and cannot be fully dismissed unless the instrumentation is anchored in bedrock or in permafrost. Though not investigated here, modifying the probe frictional surface could possibly minimize probe heave or frost jacking.
In this study, we evaluate the detection of possible probe displacement relative to the soil surface. To this end, we consider the time delay between diurnal fluctuation in temperature observed by the top sensor located above the ground surface and the other sensors initially located in the ground. The algorithm involves (1) filtering the dataset with a 1 h moving window centered on each measurement, (2) selecting days when the aboveground sensor temperature shows a daily diurnal range in temperature larger than 4 ∘C and a maximum temperature higher than 0.1 ∘C, (3) selecting sensors which when compared to the aboveground sensor show less than a 2 ∘C difference in their diurnal range in temperature and a time delay in minimum daily temperature of 15 min or less, and (4) defining an upward movement when the above difference and shift is observed for 2 consecutive days. Note that only considering the days when the top sensor above the ground surface shows a maximum temperature above 0.1 ∘C is intended to dismiss days when the top sensor is under the snow surface, which complicates the detection of upward movements. Overall, this detection method provides an initial approach for assessing probe displacement without visual inspection, as well as flagging or correcting temperature measurements and inferred metrics.
3.1 Sensor accuracy assessment and additional calibration
The developed sensor accuracy assessment approach was validated by repeating the approach several times with one DTP system and then applying the approach on 846 sensors from 70 probes (Fig. 2). The zero curtain induced by the water phase change is consistently observed around 0 ∘C, with offsets that are always smaller than the ±0.1 ∘C factory-assured accuracy. Repeating the calibration cycle three times with the same probes shows that the offset of each sensor across the calibration cycles varies over a range of 0.015 ∘C. The offsets of 846 sensors indicate a relatively Gaussian distribution of offsets with a mean of +0.02433 ∘C, a standard deviation of 0.02095 ∘C, and a 95th percentile interval between −0.022 and 0.062 ∘C.
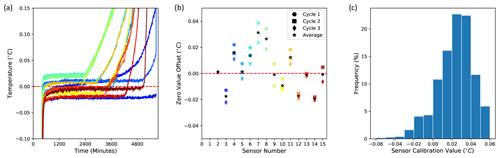
Figure 2Sensor accuracy assessment: (a) 0 ∘C curtain occurring during ice-to-water phase transition and observed by each sensor along the DTP probe, with offset related to sensor factory accuracy; (b) offset values observed by running the assessment approach three times with the same probe, indicating that the additional calibration improves the accuracy of the sensor to ±0.015 ∘C (i.e., based on variations in three cycles); and (c) distribution of the sensor factory offsets obtained from 846 sensors from 70 probes, with a mean of 0.02433 and a standard deviation of 0.02095 ∘C.
The results of the sensor accuracy assessment indicate that the sensor accuracy can be improved using the developed approach from a ±0.1 ∘C factory-assured accuracy to about ±0.015 ∘C. Note that the maximum offset measured on 846 sensors was +0.07 ∘C, which indicates the already high accuracy of the factory calibration for the tested sensors. The only observed caveat for the calibration approach is that the top sensor along the probe does not always show the clear zero curtain needed for precise calibration because it is not consistently covered with ice. This issue results from the need to leave sufficient air space, with some safety margin, between the water surface and the bottom of the logger enclosure, in order to account for ice extension, which is needed to avoid ice pushing on the logger enclosure directly and breaking the probe.
3.2 Numerical experiments
The effect of different probe characteristics and environmental factors on the measured temperature accuracy is quantified using numerical experiments. Accuracy is evaluated through the relative difference and time delay in diurnal amplitude between soil temperatures simulated with and without the numerical representation of the probe characteristics (Table 1 and Fig. 3). The maximum percentage error between the hypothetically measured and the true temperature at all times is also considered, through their absolute difference divided by the maximum amplitude at the same depth. Note that sensor accuracy is not considered in these numerical experiments and thus needs to be added to calculate the maximum total error.
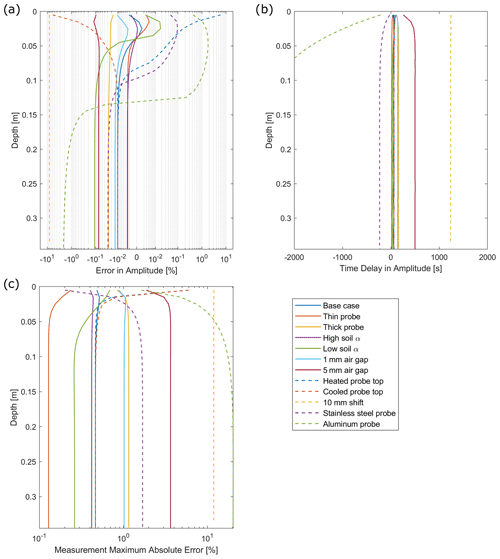
Figure 3Influence of various probe characteristics and environmental factors on the accuracy of the DTP soil temperature measurements at various depth: (a) relative error in amplitude, (b) time delay in amplitude, and (c) measurement maximum percentage error relative to true amplitude at each depth.
Table 1Evaluation of the impact of various factors on the measured temperature accuracy. The parameters for the base-case (Bc) scenario are changed one at a time to simulate various cases (Fig. 3). The soil conductivity K was taken as a linear function of soil diffusivity α. The probe is filled with a urethane blend mixture (α=0.11 mm2 s−1, κ=0.204 W m−1 K−1). Along with the base case of a 10 mm plastic probe, different error-causing variations were simulated, including probe diameter variation by ±50 %; a range of soil α; gaps of air between the probe and the soil; differential heating of the probe surface by ±50 % of diurnal variation; a shift in the probe of 1 cm downward; and different probe casing, including stainless steel and aluminum.
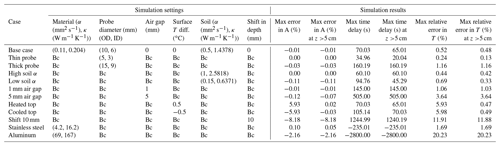
The simulated response of a DTP system in its standard plastic housing (10 mm (∼ in.) OD plastic tubing filled with urethane blend) indicates a maximum difference of −0.11 % between the hypothetically measured and the true amplitude and a time delay ranging between 60 and 95 s for a soil diffusivity between 0.15 and 1 mm2 s−1 and assuming an absence of an air gap between the probe and the soil. The maximum difference in amplitude is as small as 0.01 % with a soil diffusivity of 0.5 mm2 s−1. The temperature maximum error at any time and for the full range of soil diffusivity is ∼0.7 %.
Increasing the diameter of the plastic probe to 15 mm OD, or considering a hypothetical 1 mm air gap, produces a time delay of up to 160 s in a soil with a diffusivity of 0.5 mm2 s−1. The amplitude and measurement error can be as high as −0.03 % and ∼1.2 %, respectively. While the error is almost double the standard case, it is acceptable for many applications. Still, results show that further increasing the probe diameter or the air gap increases inaccuracies significantly. For example, the presence of an extreme hypothetical 5 mm air gap produces a time delay of up to 505 s (Fig. 3).
Different surface heating between the soil and aboveground probe surface, though difficult to assess because of the complexity of the surface energy exchanges, primarily influences the surficial soil (top 5 cm) temperature measurements. A temperature difference between the probe and soil surface equal to half the diurnal variation can create an amplitude difference of ∼6 % at 1 cm depth, decreasing to less than 0.03 % at a depth deeper than 5 cm. Such different heating or cooling responses between the soil and probe surfaces can result from different surface emissivity, insolation, near-surface wind, and water phase changes in the soil. Deployment of probes at locations where environmental factors may strengthen this source of error could benefit from burying the probe and the logger separately and using thin diameter plastic probes.
The use of stainless-steel housing may cause a slightly reduced accuracy in soil temperature measurement compared to the standard plastic 10 mm ( in.) OD tubing. Stainless steel, which facilitates vertical heat transfer along the probe, results in a maximum negative time delay of 235 s and a max error of 0.1 % between the measured and true amplitude. The stainless-steel standard probe setting increases the potential for an overestimation of the in situ amplitude in the top 15 cm and then an underestimation similar to the plastic standard case. Though stainless-steel tubing limits the accuracy in the top part of the soil, overall it can provide a tighter contact with soil because the stainless-steel probe can be driven in a thin guide hole or in some cases directly into the soil. Stainless-steel tubing with no air gap has the potential to provide relatively comparable performance to a plastic probe with an air gap larger than 2 mm. Finally, it can be noted that the use of aluminum instead of stainless steel is inadequate because it strongly decreases the measurement accuracy (Table 1).
The effect of most characteristics and factors mentioned above is minor compared to the error resulting from possible inaccuracies in positioning the sensor at a specific depth, which can occur with all measurement methods. Here, a hypothetical 1 cm downward shift of the probe can lead to an amplitude and measurement error of ∼8 % and ∼12 %, respectively, and a time delay of 1244 s. These errors are 2 times larger than the effect of an air gap of 5 mm between the soil and the probe.
For the case where the DTP system is installed temporarily for capturing a single time or snapshot of the soil temperature for mapping purpose, the amount of time needed to approach temperature equilibrium between soil and sensors depends on environmental factors and desired measurement accuracy (Fig. 4 and Table 2). The DTP system in its standard plastic housing (base case; 10 mm ( in.) OD plastic tubing filled with urethane blend) and the stainless-steel probe require 824 and 1040 s, respectively, to reach 1 % of the initial difference of temperature between the probe and the soil. Results indicate that a 1 mm air gap produces a significant delay in the early time of the equilibration process, although it reaches 1 % of the initial difference after a comparable amount of time, i.e., 1070 s. In the presence of low soil diffusivity, the equilibration time increases to 1748 s, implying that leaving a probe in place for about 30 min is appropriate for many applications. Finally, results indicate again the importance of ensuring a good coupling between the probe and the soil, as seen by the effect of a hypothetical 5 mm gap between the probe and soil, which more than doubles the equilibration time needed for reaching similar accuracy.
Table 2Time (in seconds) needed for the DTP sensor to approach a soil temperature of 0.1, 0.05, and 0.01 times their initial differences, depending on various probe and environmental parameters. The parameters for the base-case (Bc) scenario are changed one at a time to simulate various cases (Fig. 4). Table 1 provides the values of the various parameters for each scenario.

3.3 Simultaneous monitoring of snow depth and snowmelt-infiltration characteristics in a mountainous watershed
Quantifying snow and water distribution in snow-dominated mountainous watersheds is critical for managing downstream water resources and societal services (Viviroli et al., 2007), especially at a time when their functioning is increasingly altered by climate change (Barnett et al., 2005). Climate change and interannual variability in precipitation intensity and surface temperature strongly impact snowpack dynamics and snowmelt timing, streamflow, groundwater recharge, and surface energy balance. A particular challenge to the predictive understanding of watershed dynamics and response to perturbations is monitoring snowpack properties, the timing and magnitude of snowmelt events, and the repartitioning of water into surface and subsurface flow. Such monitoring must be conducted at multiple scales across complex terrains as needed to accurately capture the impact of a large range of gradients in topography, air mass exposure, and vegetation cover on these dynamics (e.g., Lundquist et al., 2019; Strachan et al., 2016). The DTP system has the potential to significantly improve the sampling of these properties and their variability along these gradients.
Two collocated DTP systems in a standard plastic housing, one above and one below the ground surface at a mountainous headwater site in the East River watershed of the upper Colorado River basin (Hubbard et al., 2018; Tran et al., 2019; Wainwright et al., 2022), are used to illustrate the DTP data information content on the timing and amplitude of thermal and hydrological processes in the snow and soil columns (Fig. 5). The snow DTP system provided temperature with 0.1 m resolution between 0.05 and 0.85 m above the ground surface and 0.05 m resolution between 0.85 and 1.15 m. The DTP system installed in the soil, next to the snow DTP system, provided measurements with 0.05 m resolution between 0 and 0.3 m depth and 0.1 m resolution between 0.3 and 0.7 m depth. Sensor accuracy was 0.06 ∘C, as probes were deployed before the development of the described calibration method. The snow-thickness algorithm is applied to the DTP system placed above the ground surface, and the estimated snow depth dynamic is compared to the snow depth pattern observed using a sonic sensor at the Butte SNOTEL site (snow telemetry; https://wcc.sc.egov.usda.gov/nwcc/site?sitenum=380, last access: 25 February 2022) located 2 km away and 350 m higher in elevation. Though similar snow depth trends are expected at both sites, their absolute values cannot be compared due to their difference in elevation and location. Soil moisture measurements at 0.1 and 0.5 m depth about 1 m away from the DTP probes are used to further evaluate the information contained in the DTP data.
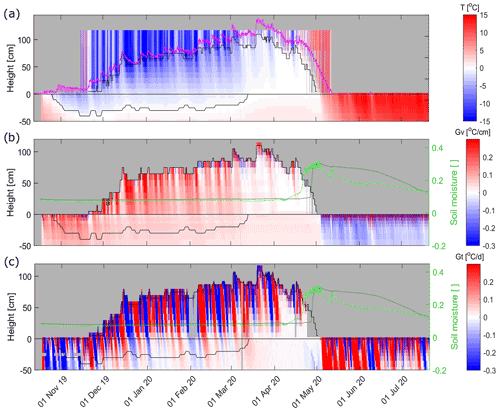
Figure 5DTP systems deployed for snow and soil temperature measurements at a site in the East River (Colorado) watershed. The DTP-inferred snow depth (aboveground black line) and soil frozen-layer thickness (belowground black line) are overlaid on the DTP (a) temperature (T), (b) its vertical gradient (Gv) at each sampling time, and (c) the temporal change in temperature (Gt) after averaging the time series with a moving 24 h time window. The pink line shows the snow depth from the Butte SNOTEL station located 2 km away and at a 350 m higher elevation. The light and dark green lines indicate the soil moisture (m3 m−3) at 10 and 50 cm depth, respectively. Color scales have been cropped to the displayed minimum and maximum values in order to improve visualization. Please note that the date format in this figure is day month year.
The snow depth estimated using the DTP system is consistent with the snow depth observed at the Butte SNOTEL site (Fig. 5). The DTP system captures the main changes in snow depth linked to snow precipitation, snowmelt, and/or snow compaction visible in the SNOTEL dataset. The earlier timing in snowmelt at the DTP site is explained by the fact that the SNOTEL station is at higher elevation than the DTP probe. Overall, the main differences between these methods is the lower temporal and spatial resolution of the DTP-inferred snow depth, which is caused by the use of a 24 h moving window to estimate snow depth, the occurrence of days involving snow precipitation events and/or very little diurnal fluctuations, and the 5–10 cm spacing between temperature sensors along the probe.
The DTP system placed below the ground surface shows that the soil freezing, which is estimated by extracting the 0 ∘C isotherm from the temperature data, starts in mid-October and reaches 0.4 m depth by mid-November. The first significant snowfall at the end of November increases the insulation of the ground, which leads to a slow decrease in the frozen-layer thickness from the bottom (Fig. 5a). The soil thawing accelerates in March after the snowpack becomes thicker and air temperature gets warmer. The thawing of soil occurs relatively quickly, likely because of the presence of a relatively dry soil, as indicated by the absence of a clear zero-curtain effect expected in the presence of a large amount of ice and subsequently latent heat absorbed during phase transition. After mid-March, the entirely thawed soil, still covered with snow, remains at an almost constant temperature for about 3 weeks, with less than 0.01 ∘C change per day (Fig. 5c).
The major snowmelt event occurring at the end of March is captured by the aboveground DTP system via the strong decrease in snow depth as well as by the temperature of 0 ∘C throughout the snowpack. Indeed, once the entire snowpack reaches 0 ∘C, the additional thermal energy entering the snowpack initiates the phase change and water infiltration throughout the snowpack (Fig. 5a) (Dingman, 2014; Reusser and Zehe, 2011). The snowmelt water reaching the ground is close to 0 ∘C, while the ground at this location shows a relatively constant temperature of 0.41, 0.65, and 1.38 ∘C at 10, 20, and 50 cm depth, respectively. The snowmelt infiltrating into the ground creates a slight decrease in soil temperature that is apparent in the soil temperature data (Fig. 5a) and more clearly identified by looking at the change in the 24 h average temperature difference (Fig. 5c). The temporal change in temperature with depth, which has a different shape than at earlier times during the winter – when heat conduction was dominating heat transfer, is related to the water infiltration. This change in soil temperature is consistent in timing with a soil moisture increase at 10 cm depth around mid-April and 10 d later at 50 cm depth. Overall, the high vertical resolution and accuracy of the DTP system and its deployment above and below ground enabled the observation of snowpack dynamics and its impact on the soil heat (and to some extent hydrological) fluxes at a resolution that is not achievable with traditional sensors.
3.4 Monitoring soil temperature, frozen-/thawed-layer thickness, and probe displacement in an Arctic permafrost system
Large uncertainty remains in how northern high-latitude environments will evolve under climate warming and in particular in how thaw and release of permafrost carbon will be offset by increased vegetation carbon uptake (Jorgenson et al., 2010; Parazoo et al., 2018). Arctic annual average air temperatures between 1971–2017 increased by 2.7 ∘C, at 2.4 times the rate of the Northern Hemisphere average (Box et al., 2019). This change in temperature is complemented with changes in other atmospheric properties, including humidity, cloud formation, rainfall, and snowfall precipitation. One particular challenge involves improving the predictive understanding of how permafrost regions transition to unfrozen ground and disentangling the various controls and their individual impact on the carbon cycle (Jorgenson et al., 2010). Overcoming this challenge requires improving our capability to estimate the soil freeze/thaw depth, the impact of spatially variable temporal shifts in insolation and insulation on the subsurface temperature, and the water/heat fluxes.
Here, a DTP system in a standard plastic housing located in a discontinuous permafrost environment along Teller Road (mile 27) near Nome, Alaska (Léger et al., 2019; Uhlemann et al., 2021), is used to further illustrate the value of the DTP system in monitoring temperature, frozen-layer thickness, and thawed-layer depth, as well as to discuss the potential issue of DTP system displacement relative to the ground surface (Fig. 6). The displayed DTP dataset comes from a probe deployed at a location where the permafrost table is deeper than the bottom of the probe located at 1.05 m depth. The deployed probe provided temperature with 0.05 m resolution from 0.05 m above the ground surface to 0.25 m depth and with 0.1 m resolution from 0.25 to 1.05 m depth. The bottom of the frozen layer in the fall and wintertime, as well as the bottom of the thawed layer in the spring to fall season, were estimated by selecting the deepest sensor with soil temperature below and above the 0 ∘C isotherm, respectively. The dataset discussion involves an evaluation of the snow depth and air temperature obtained at a nearby monitoring site (<1 km) using a sonic-based snow sensor and air temperature sensor, respectively (https://ngee-arctic.ornl.gov/data/pages/NGA243.html, last access: 25 February 2022).
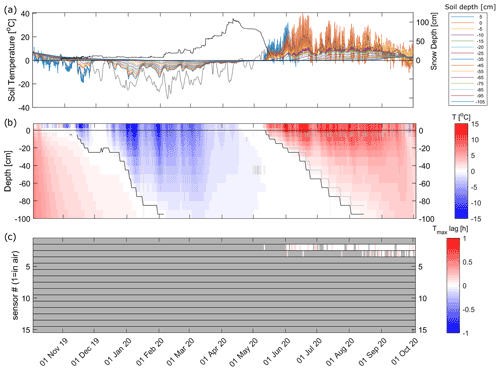
Figure 6(a) Soil temperature measurements from a DTP system in a discontinuous permafrost environment (Teller Road, mile 27, Nome, Alaska) with sensor from 5 cm above the surface to 105 cm depth, overlaid with the snow depth (black line) and daily average air temperature (grey line) measured at a weather station located about 1 km away from the DTP system; (b) same DTP dataset with black lines indicating the inferred bottom and top of the frozen layer; (c) time delay smaller than 0.25 h in daily minimum temperature between the aboveground top sensor and each sensor along the probe, indicating the presence of an additional sensor positioned above the ground surface due to an upward displacement of the DTP system relative to ground surface. A ∼2.5 cm displacement is flagged around 3 June and then 20 August, which together led to a ∼7.5 cm shift of the probe relative to the ground surface. This displacement is also qualitatively visible in (a) where the line representing aboveground temperature (initially at +5 cm) is successively overlapped by temperature data from the temperature sensors initially located at 0 and −5 cm depth. The grey line in (b) indicates the thawed-layer thickness estimate after correction applied for the 3 June displacement. Please note that the date format in this figure is day month year.
Soil freezing, which starts at the end of October before being slowed by a warm event coupled with snow precipitation in late November, reaches a depth deeper than the length of the DTP probe in early February (Fig. 6b). The small amount of snow (<30 cm) on the ground favors soil freezing until snow event intensity and air temperature increase in March and April. Consequently, the ground temperature increases and the temperature of the entire soil column reaches temperatures slightly below 0 ∘C at the end of April. The soil thawing process starts after the first bare-ground day, as indicated by the diurnal daily temperature variation becoming visible at the ground surface. The soil thawing occurs slowly, with a zero-curtain effect indicating the presence of wet conditions. The thawed-layer thickness increases from mid-May to mid-August, at which time the thawing occurs deeper than the DTP probe.
The detection of a persistent and negligible time delay in daily minimum temperature between the aboveground sensor and the underlying nearest sensor indicates the presence of a second sensor above the ground surface and thus an upward displacement of about 2.5 cm (±1.5 cm) of the DTP system relative to the ground surface around 3 June. Then, there is an additional displacement around 20 August, leading to a 7.5 cm (±1.5 cm) total displacement during the thawing season (Fig. 6c). The developed detection method provides reliable detection of probe movement relative to the ground, though it does not enable centimeter-scale resolution. Still, the approach allows us to flag the data for lower accuracy and possibly apply subsequent corrections to the reference depth of temperature data and inferred metrics.
Overall, the high vertical resolution and accuracy of the DTP system enables the monitoring of temperature – and related frozen-/thawed-layer thickness – in the Arctic environment at a resolution that allows us to disentangle the impact of various processes on soil warming and changes in hydro-biogeochemical processes. Even with the difficulties in monitoring extreme environments, the DTP system offers a way to account for various sources of measurement uncertainties and potentially develop the dense datasets needed to improve predictive understanding of Arctic feedback to climate change.
The developed DTP system fulfills numerous requirements for measuring soil or snow temperature with unprecedented lateral and vertical spatial resolution across the landscape. The development and assessment of the DTP system has shown that the use of digital sensors mounted on PCB sections is appropriate for (1) managing a large number of sensors; (2) enabling repeatable measurements and the assessment of measurement accuracy; (3) reaching low production and assembly costs needed for building hundreds of probes; and (4) providing flexibility in building probes with various sensor spacings, length, and packaging, depending on the intended applications. In addition, the development of a custom logger to communicate with the PCB-mounted temperature sensors offers (1) a compact and low-power solution crucial for limiting the installation complexity and footprint; (2) a low-cost solution compared to other logging options, which is needed for the deployment of a large number of probes; (3) efficient data transfer through BLE and other wireless connectivity solutions in the future; and (4) publicly documented hardware and software design that offers control over the entire data acquisition-to-management pipeline.
Our developed calibration approach also enables a reliable assessment of sensor accuracy and provides an additional calibration of the temperature sensors. Results indicate that the digital temperature sensors satisfy the factory-assured, NIST-traceable accuracy of ±0.1 ∘C. Moreover, all sensors tested in this study showed an accuracy better than ±0.07 ∘C. The novel calibration approach has also been successful in increasing the sensor accuracy to ±0.015 ∘C. This in-house calibration, along with the factory-assured accuracy, are (to the authors' knowledge) unprecedented for digital sensors deployed in environmental systems and are relatively close to the accuracy that can be reached with high-accuracy analog sensors and loggers. The main drawback in the calibration approach is the time needed to cycle probes (>2 d) and the uncommonness of incubators that fit probes longer than ∼1.3 m.
Besides the sensor accuracy, numerical simulations of heat transfer in soil and along probes have enabled an evaluation of how probe characteristics and various environmental factors can further affect measurement accuracy. The assessment of measurement errors, though rarely done, informs both the potential and limitations of various methods in capturing small changes in temperature gradients. Capturing small changes in temperature is critical for estimating fluxes or thermal parameters using physically based models (e.g., Brunetti et al., 2021) or in evaluating processes linked to water phase changes. Results of the numerical study indicate that, in favorable environmental conditions and a soil diffusivity around 0.5 mm2 s−1, the use of a 6 mm ( in.) ID and 10 mm ( in.) OD diameter plastic probe provides measurements with up to 0.01 % and 70 s in amplitude error and time delay, respectively. Still, results have shown that the potential presence of an air gap between the soil and the probe and/or different surface heating between the probe and soil surface can decrease the measurement accuracy (Fig. 3). In addition, results have indicated that the use of stainless steel instead of plastic tubing, though implying a decrease in accuracy, can provide similar performance deeper than the top few centimeters if driving the probe in a thin guide hole or directly into the soil, precluding the presence of an air gap between the soil and probe. While plastic probes are preferable for monitoring soil and snow temperature, stainless-steel probes are suitable for sequentially acquiring soil temperature across the landscape, as they are sturdier.
Importantly, the above probe characteristics have shown impacts on temperature measurements smaller than those owing to uncertainty in sensor vertical positioning. An error of ±1 cm in positioning a sensor in the soil can lead to an 8.2 % and 1460 s amplitude error and time delay, respectively, for a typical soil with a thermal diffusivity of 0.5 mm2 s−1. Note that such positioning inaccuracy can occur as a result of either an error in installing a sensor at a precise distance from the ground surface and/or relative to another sensor. The first issue is relevant to all measurement techniques and linked to the difficulty in assessing what the ground surface is (particularly in heavily vegetated landscapes), as well as the potential upward movement of the sensors relative to the soil surface. The second issue – which is absent in the DTP system, where millimeter precision in sensor spacing is achieved – is conspicuously present in other types of measurement techniques, including fiber-optic-based methods or individual point-scale sensors deployed at different depths (e.g., Steele-Dunne et al., 2010). Overall, while the DTP equilibrium time and measurement accuracy is not as high as theoretically achievable with sensors in direct and tight contact with the soil, the numerical experiments enable a clear assessment of the advantages and limitations of various measurement strategies and devices.
The deployment of the plastic DTP system for monitoring snowpack thickness has confirmed the results from earlier studies (e.g., Reusser and Zehe, 2011). In particular, this study confirms that a vertically resolved temperature probe can be used for the daily estimation of snow depth with an accuracy close to the spacing of the temperature sensors. The algorithm presented in this study was preferred to another algorithm (Reusser and Zehe, 2011), as it prevented spurious estimates occurring sporadically during times with low diurnal variation in air temperature at the site. Still, an assessment of the advantages and limitations of various algorithms would require datasets from a much larger number of sites. Results of this study have also confirmed that capturing temperature throughout the snowpack provides crucial information on the snow cold content and on the onset of snowmelt events driving water infiltration into the soil or potential surface water runoff. Although it is beyond the scope of this particular study, the acquired snow temperature data can be potentially used further for estimating soil thermal parameters (e.g., Oldroyd et al., 2013) and validating the modeling of cryospheric processes. An additional advantage of the DTP snow probes is their low spatial footprint and suitability for deployment in steep hillslope and at-risk locations. This advantage comes with the caveat that the DTP-inferred snow depth has lower resolution and accuracy than temperature-corrected sonic-based sensors and that its overall value is limited where snowpack is generally thicker than a few meters, as the costs of the probe scale up with the number of temperature sensors. Though the DTP system is not intended to replace sonic-based sensor and intensive sites, it opens the door for dense networks of snow depth, temperature, and potentially SWE (snow water equivalent) estimates at the watershed scale, where predictability is still limited owing to the complexity and cost of capturing precipitation heterogeneity and widely variable hillslope-scale heterogeneity, as well as a wide range of energy dynamics. Capturing both the local and larger-scale snow characteristics is critical in developing statistics on the different coupling of landscape and environmental factors and enabling advances in the understanding of watershed aggregated snow and water dynamics.
Besides snow temperature, there is a broad range of applications of the DTP system for monitoring soil temperature, inferring metrics (such as thawed-layer thickness, frozen-layer thickness, zero curtain, and thermal parameters), informing on heat and water dynamics, and validating thermohydrological models. As a simple example, this study reported on the use of the DTP system to monitor the frozen-layer thickness and the thawing process in a mountainous and Arctic environment. Results show that the 5–10 cm spacing between temperature sensors along the DTP system is adequate to reliably track the freezing and thawing front, with a vertical resolution that has been rarely obtained (Cable et al., 2016; Léger et al., 2019). While this study shows promising results to deploy the DTP system at numerous locations, it also underlines the importance of automated algorithms to extract metrics, assess data quality, and in some cases improve the DTP system accuracy. In this regard, the DTP fine vertical resolution and the upward-movement detection approach developed in this study enable the satisfactory detection of possible upward displacement due to mechanical processes or interaction with animals. Note that frost jacking or the impact of soil frost and thaw settlement on temperature sensors displacement is related not only to the DTP system but also to buried individual sensors (Johnson and Hansen, 1974). Overall, the developed DTP system is extensible to a wide range of applications and modular enough to facilitate future developments.
Similarly, the system has the potential for popularizing single-time or sporadic mapping of soil temperature across the landscape for various purposes, including the delineation of near-surface permafrost (Léger et al., 2019), the identification of temperature hotspots or geothermal areas (Lubenow et al., 2016), or the delineation of thermal regimes – indicators of various soil hydro-biogeochemical regimes (Cartwright, 1968). Indeed, the DTP system developed in this study, in particular the stainless-steel version of it, is (to the authors' knowledge) the first system that provides the ability to efficiently install DTP systems for a short period of time (e.g., 30 min) and move them across the landscape at a pace that can enable surveys of soil temperature at hundreds to thousands of locations within a short time period. Such surveys have remained limited, presumably because of the lack of equipment with an adequate trade-off between the acquisition depth needed to minimize land surface boundary impacts to the extent needed to identify a thermal anomaly and adequate sensor accuracy, vertical resolution, and total cost (including material, acquisition, and data management).
This study aimed at developing a low-power and small-footprint DTP system providing vertically dense and high-accuracy temperature measurements at a total cost that would enable its deployment in a substantial number of locations, as needed, to improve the multiscale observation and understanding of environmental system functioning – in particular snowpack and soil thermal and hydrological dynamics. The developed DTP system and our assessment of it have demonstrated its potential for measuring soil temperature with unprecedented vertical resolution, high accuracy, and low cost while minimizing physical footprint and energy consumption. Also, this study shows that the developed system provides flexibility in using various types of housing (depending on project goals and environmental requirements) and offers simplicity in downloading and managing data. To our knowledge, it is the first time that soil and snow temperature data are gathered with such high spatial resolution to simultaneously capture changes in snow depth and frozen-/thawed-layer thickness. We anticipate that the datasets acquired with this system will be crucial in improving the estimation of thermal parameters and dynamics across watershed scales, which both benefit from high-resolution and high-accuracy data. These advances are particularly critical for improving our understanding of the timing and intensity of snowpack and soil thermohydrological dynamics in heterogeneous environments. We expect that the improved monitoring data and scientific insights developed from the data will greatly improve the predictive understanding of the heat and water fluxes in snow and soil, which is essential for improving water resources and carbon cycle assessment and management.
The DTP system development and accuracy assessment presented in this study is an important step toward deploying large numbers of sensors, as part of a strategy optimized with regard to environmental monitoring objectives, emphasizing accuracy, resolution, repeatability, and low equipment and measurement costs. The development of hardware and software, as well as their release into the public domain, is similarly important to ensure knowledge transfer and future developments. Here as a first step toward this objective, we presented the capabilities of a DTP system that uses TMP117 sensors and a custom logger design described in detail. The level of detail that has been provided about the system design assures the repeatability of experiments and the development and advancement of future DTP systems, using the same or improved components. The DTP system opens new possibilities for observing thermohydrological processes at numerous locations and provides the flexibility for adapting it to applications not discussed in this study, including in-stream deployment. Ongoing additional developments include a Python-based numerical framework and toolbox for the automated extraction of metrics and estimation of temperature-related processes, the addition of LoRa connectivity for the real-time transmission of data from hundreds of nodes over several kilometers to data hubs, and the incorporation of additional low-cost and low-power sensors to the system.
The data presented in this study are available from the NGEE Arctic (Next-Generation Ecosystem Experiments) data portal at https://doi.org/10.5440/1819363.
BD, SW, JL, IS, and FA designed the DTP acquisition strategy. BD, FA, SP, JF, and SW carried out the development of the DTP hardware and software. JL performed numerical simulations. BD and PM developed the calibration approach. BD, CB, and CW worked on algorithm development. BD, PM, JL, SF, SW, SU, IS, CU, and JP participated in building the DTP systems and/or data collection. RB provided meteorological datasets. BD prepared the manuscript with SW, JL, PM, CW, SU, and SSH. All authors contributed to the study and approved the final version of the paper.
The contact author has declared that neither they nor their co-authors have any competing interests.
Publisher’s note: Copernicus Publications remains neutral with regard to jurisdictional claims in published maps and institutional affiliations.
We acknowledge the assistance of Berkeley Lab's Geoscience Measurement Facility (GMF) for providing help in early prototyping of the DTP system. In addition, the authors would like to thank the editor, Adam Booth, as well as Michael Prior-Jones and two anonymous reviewers for their suggestions that helped improve this publication.
This material is based upon work supported primarily by Next-Generation Ecosystem Experiments (NGEE Arctic) and secondly by the Watershed Function Scientific Focus Area, both funded by the U.S. Department of Energy Office of Science Office of Biological and Environmental Research (award no. DE-AC02-05CH11231).
This paper was edited by Adam Booth and reviewed by Michael Prior-Jones and two anonymous referees.
Aguilar, O. F. S., Arredondo, J. A. A., Trujillo, B. A. E. and Fundora, A. J. B.: Determining the in situ apparent thermal diffusivity of a sandy soil, Rev. Bras. Ciênc. Solo, 42, e0180025, https://doi.org/10.1590/18069657rbcs20180025, 2018.
Andújar Márquez, J. M., Martínez Bohórquez, M. Á., and Gómez Melgar, S.: Ground thermal diffusivity calculation by direct soil temperature measurement, Application to very low enthalpy geothermal energy systems, Sensors, 16, 306, https://doi.org/10.3390/s16030306, 2016.
Barnett, T. P., Adam, J. C., and Lettenmaier, D. P.: Potential impacts of a warming climate on water availability in snow-dominated regions, Nature, 438, 303–309, 2005.
Beardsmore, G., Egan, S., and Sandiford, M.: A Fourier Spectral Method to Measure the Thermal Diffusivity of Soil, Geotech. Test. J., 43, https://doi.org/10.1520/GTJ20180300, 2020.
Biskaborn, B. K., Lanckman, J.-P., Lantuit, H., Elger, K., Streletskiy, D. A., Cable, W. L., and Romanovsky, V. E.: The new database of the Global Terrestrial Network for Permafrost (GTN-P), Earth Syst. Sci. Data, 7, 245–259, https://doi.org/10.5194/essd-7-245-2015, 2015.
Box, J. E., Colgan, W. T., Christensen, T. R., Schmidt, N. M., Lund, M., Parmentier, F.-J. W., Brown, R., Bhatt, U. S., Euskirchen, E. S., Romanovsky, V. E., Walsh, J. E., Overland, J. E., Wang, M., Corell, R. W., Meier, W. N., Wouters, B., Mernild, S., Mård, J., Pawlak, J., and Olsen, M. S.: Key indicators of Arctic climate change: 1971–2017, Environ. Res. Lett., 14, 045010, https://doi.org/10.1088/1748-9326/aafc1b, 2019.
Bredehoeft, J. D. and Papaopulos, I. S.: Rates of vertical groundwater movement estimated from the Earth's thermal profile, Water Resour. Res., 1, 325–328, 1965.
Brewer, M. C.: The thermal regime of an Arctic lake, EOS T. Am. Geophys. Un., 39, 278–284, 1958.
Briggs, M. A., Lautz, L. K., McKenzie, J. M., Gordon, R. P., and Hare, D. K.: Using high-resolution distributed temperature sensing to quantify spatial and temporal variability in vertical hyporheic flux, Water Resour. Res., 48, 0043–1397, https://doi.org/10.1029/2011WR011227, 2012.
Briggs, M. A., Lautz, L. K., Buckley, S. F., and Lane, J. W.: Practical limitations on the use of diurnal temperature signals to quantify groundwater upwelling, J. Hydrol., 519, 1739–1751, 2014.
Brunetti, C., Lamb, J., Wielandt, S., Uhlemann, S., Shirley, I., McClure, P., and Dafflon, B.: Estimation of depth-resolved profiles of soil thermal diffusivity from temperature time series and uncertainty quantification, Earth Surf. Dynam. Discuss. [preprint], https://doi.org/10.5194/esurf-2021-68, in review, 2021.
Cable, J. M., Ogle, K., Bolton, W. R., Bentley, L. P., Romanovsky, V., Iwata, H., Harazono, Y. and Welker, J.: Permafrost thaw affects boreal deciduous plant transpiration through increased soil water, deeper thaw, and warmer soils, Ecohydrology, 7, 982–997, 2014.
Cable, W. L., Romanovsky, V. E., and Jorgenson, M. T.: Scaling-up permafrost thermal measurements in western Alaska using an ecotype approach, The Cryosphere, 10, 2517–2532, https://doi.org/10.5194/tc-10-2517-2016, 2016.
Cartwright, K.: Temperature prospecting for shallow glacial and alluvial aquifers in Illinois, Circular no. 433, Illinois state geological survey, Department of Registration and Education, Illinois, USA, 1968.
Chang, K.-Y., Riley, W. J., Knox, S. H., Jackson, R. B., McNicol, G., Poulter, B., Aurela, M., Baldocchi, D., Bansal, S., Bohrer, G., Campbell, D. I., Cescatti, A., Chu, H., Delwiche, K. B., Desai, A. R., Euskirchen, E., Friborg, T., Goeckede, M., Helbig, M., Hemes, K. S., Hirano, T., Iwata, H., Kang, M., Keenan, T., Krauss, K. W., Lohila, A., Mammarella, I., Mitra, B., Miyata, A., Nilsson, M. B., Noormets, A., Oechel, W. C., Papale, D., Peichl, M., Reba, M. L., Rinne, J., Runkle, B. R. K., Ryu, Y., Sachs, T., Schäfer, K. V. R., Schmid, H. P., Shurpali, N., Sonnentag, O., Tang, A. C. I., Torn, M. S., Trotta, C., Tuittila, E.-S., Ueyama, M., Vargas, R., Vesala, T., Windham-Myers, L., Zhang, Z., and Zona, D.: Substantial hysteresis in emergent temperature sensitivity of global wetland CH4 emissions, Nat. Commun., 12, 2266, https://doi.org/10.1038/s41467-021-22452-1, 2021.
Constantz, J.: Heat as a tracer to determine streambed water exchanges, Water Resour. Res., 44, 0043–1397, https://doi.org/10.1029/2008WR006996, 2008.
Constantz, J., Stewart, A. E., Niswonger, R., and Sarma, L.: Analysis of temperature profiles for investigating stream losses beneath ephemeral channels, Water Resour. Res., 38, 52-1–52-13, https://doi.org/10.1029/2001WR001221, 2002.
Dafflon, B., Wielandt, S., Lamb, J., Shirley, I., and Uhlemann, S.: A Distributed Temperature Profiling System for Vertically and Laterally Dense Acquisition of Soil and Snow Temperature: Supporting Data, Next Generation Ecosystem Experiments Arctic Data Collection [data set], Oak Ridge National Laboratory, U.S. Department of Energy, Oak Ridge, Tennessee, USA, https://doi.org/10.5440/1819363, 2021.
Davidson, E. A. and Janssens, I. A.: Temperature sensitivity of soil carbon decomposition and feedbacks to climate change, Nature, 440, 165–173, 2006.
Demchenko, Y., Grosso, P., De Laat, C. and Membrey, P.: Addressing big data issues in scientific data infrastructure, International conference on collaboration technologies and systems (CTS), IEEE, 48–55, https://doi.org/10.1109/CTS.2013.6567203, 2013.
Dingman, S. L.: Physical hydrology, Waveland press, Long Grove, IL, ISBN 9781478611189, 2014.
Fanelli, R. M. and Lautz, L. K.: Patterns of water, heat, and solute flux through streambeds around small dams, Groundwater, 46, 671–687, 2008.
García, F. C., Bestion, E., Warfield, R., and Yvon-Durocher, G.: Changes in temperature alter the relationship between biodiversity and ecosystem functioning, P. Natl. Acad. Sci., 115, 10989–10994, 2018.
Gordon, R. P., Lautz, L. K., and Daniluk, T. L.: Spatial patterns of hyporheic exchange and biogeochemical cycling around cross-vane restoration structures: Implications for stream restoration design, Water Resour. Res., 49, 2040–2055, 2013.
Hatch, C. E., Fisher, A. T., Revenaugh, J. S., Constantz, J., and Ruehl, C.: Quantifying surface water-groundwater interactions using time series analysis of streambed thermal records: Method development, Water Resour. Res., 42, 0043–1397, https://doi.org/10.1029/2005WR004787, 2006.
Hubbard, S. S., Williams, K. H., Agarwal, D., Banfield, J., Beller, H., Bouskill, N., Brodie, E., Carroll, R., Dafflon, B., Dwivedi, D., Falco, N., Faybishenko, B., Maxwell, R., Nico, P., Steefel, C., Steltzer, H., Tokunaga, T., Tran, P. A., Wainwright, H., and Varadharajan, C.: The East River, Colorado, Watershed: A Mountainous Community Testbed for Improving Predictive Understanding of Multiscale Hydrological – Biogeochemical Dynamics, Vadose Zone J., 17, 1539–1663, https://doi.org/10.2136/vzj2018.03.0061, 2018.
Hurwitz, S., Harris, R. N., Werner, C. A., and Murphy, F.: Heat flow in vapor dominated areas of the Yellowstone Plateau Volcanic Field: Implications for the thermal budget of the Yellowstone Caldera, J. Geophys. Res.-Sol. Ea., 117, 0148–0227, https://doi.org/10.1029/2012JB009463, 2012.
Irvine, D. J., Kurylyk, B. L., and Briggs, M. A.: Quantitative guidance for efficient vertical flow measurements at the sediment – water interface using temperature – depth profiles, Hydrol. Proc., 34, 649–661, 2020.
Iversen, C. M., Sloan, V. L., Sullivan, P. F., Euskirchen, E. S., McGuire, A. D., Norby, R. J., Walker, A. P., Warren, J. M. and Wullschleger, S. D.: The unseen iceberg: plant roots in arctic tundra, New Phytol., 205, 34–58, 2015.
Iwahana, G., Busey, R. C., and Saito, K.: Seasonal and Interannual Ground-Surface Displacement in Intact and Disturbed Tundra along the Dalton Highway on the North Slope, Alaska, USA, 10, 22, https://doi.org/10.3390/land10010022, 2021.
Johnson, D. L. and Hansen, K. L.: The Effects of Frost-Heaving on Objects in Soils, Plains Anthropol., 19, 81–98, 1974.
Jorgenson, M. T., Romanovsky, V., Harden, J., Shur, Y., O'Donnell, J., Schuur, E. A. G., Kanevskiy, M., and Marchenko, S.: Resilience and vulnerability of permafrost to climate change, Can. J. Forest Res., 40, 1219–1236, 2010.
Langtangen, H. P. and Linge, S.: Finite difference computing with PDEs: a modern software approach, Springer Nature, 530 p., ISBN 9783319554556, 2017.
Léger, E., Dafflon, B., Robert, Y., Ulrich, C., Peterson, J. E., Biraud, S. C., Romanovsky, V. E., and Hubbard, S. S.: A distributed temperature profiling method for assessing spatial variability in ground temperatures in a discontinuous permafrost region of Alaska, The Cryosphere, 13, 2853–2867, https://doi.org/10.5194/tc-13-2853-2019, 2019.
Leon, E., Vargas, R., Bullock, S., Lopez, E., Panosso, A.R. and La Scala, N.: Hot spots, hot moments, and spatio-temporal controls on soil CO2 efflux in a water-limited ecosystem, Soil Biol. Biochem., 77, 12–21, https://doi.org/10.1016/j.soilbio.2014.05.029, 2014.
Lubenow, B. L., Fairley, J. P., Lindsey, C. R., and Larson, P. B.: Influences on shallow ground temperatures in high flux thermal systems, J. Volcanol. Geoth. Res., 323, 53–61, 2016.
Lundquist, J. and Lott, F.: Using inexpensive temperature sensors to monitor the duration and heterogeneity of snow-covered areas, Water Resour. Res., 44, 0043–1397, https://doi.org/10.1029/2008WR007035, 2008.
Lundquist, J., Hughes, M., Gutmann, E., and Kapnick, S.: Our skill in modeling mountain rain and snow is bypassing the skill of our observational networks, Bull. Am. Meteorol. Soc., 100, 2473–2490, 2019.
Mangum, B.: Reproducibility of the temperature of the ice point in routine measurements, US Department of Commerce, Technology Administration, National Institute of Standards and Technology, https://www.govinfo.gov/app/details/GOVPUB-C13-5ce1be74727a73a2d8c89172d042b3a4 (last access: 25 February 2022), 1995.
Matsuoka, N.: Continuous Recording of Frost Heave and Creep on a Japanese Alpine Slope, Arctic Alpine Res., 26, 245–254, 1994.
Myers-Smith, I. H., Forbes, B. C., Wilmking, M., Hallinger, M., Lantz, T., Blok, D., Tape, K. D., Macias-Fauria, M., Sass-Klaassen, U., Lévesque, E., Boudreau, S., Ropars, P., Hermanutz, L., Trant, A., Collier, L. S., Weijers, S., Rozema, J., Rayback, S. A., Schmidt, N. M., Schaepman-Strub, G., Wipf, S., Rixen, C., Ménard, C. B., Venn, S., Goetz, S., Andreu-Hayles, L., Elmendorf, S., Ravolainen, V., Welker, J., Grogan, P., Epstein, H. E., and Hik, D. S.: Shrub expansion in tundra ecosystems: dynamics, impacts and research priorities, Environ. Res. Lett., 6, 045509, https://doi.org/10.1088/1748-9326/6/4/045509, 2011.
Naranjo, R. C. and Turcotte, R.: A new temperature profiling probe for investigating groundwater-surface water interaction, Water Resour. Res., 51, 7790–7797, 2015.
Natali, S. M., Watts, J. D., Rogers, B. M., Potter, S., Ludwig, S. M., Selbmann, A.-K., Sullivan, P. F., Abbott, B. W., Arndt, K. A., Birch, L., Björkman, M. P., Bloom, A. A., Celis, G., Christensen, T. R., Christiansen, C. T., Commane, R., Cooper, E. J., Crill, P., Czimczik, C., Davydov, S., Du, J., Egan, J. E., Elberling, B., Euskirchen, E. S., Friborg, T., Genet, H., Göckede, M., Goodrich, J. P., Grogan, P., Helbig, M., Jafarov, E. E., Jastrow, J. D., Kalhori, A. A. M., Kim, Y., Kimball, J. S., Kutzbach, L., Lara, M. J., Larsen, K. S., Lee, B.-Y., Liu, Z., Loranty, M. M., Lund, M., Lupascu, M., Madani, N., Malhotra, A., Matamala, R., McFarland, J., McGuire, A. D., Michelsen, A., Minions, C., Oechel, W. C., Olefeldt, D., Parmentier, F.-J. W., Pirk, N., Poulter, B., Quinton, W., Rezanezhad, F., Risk, D., Sachs, T., Schaefer, K., Schmidt, N. M., Schuur, E. A. G., Semenchuk, P. R., Shaver, G., Sonnentag, O., Starr, G., Treat, C. C., Waldrop, M. P., Wang, Y., Welker, J., Wille, C., Xu, X., Zhang, Z., Zhuang, Q., and Zona, D.: Large loss of CO2 in winter observed across the northern permafrost region, Nat. Clim. Change, 9, 852–857, 2019.
Nicolsky, D., Romanovsky, V., and Panteleev, G.: Estimation of soil thermal properties using in-situ temperature measurements in the active layer and permafrost, Cold Reg. Sci. Technol., 55, 120–129, 2009.
Oldroyd, H. J., Higgins, C., Huwald, H., Selker, J. S., and Parlange, M.: Thermal diffusivity of seasonal snow determined from temperature profiles, Adv. Water Resour., 55, 121–130, 2013.
Parazoo, N. C., Koven, C. D., Lawrence, D. M., Romanovsky, V., and Miller, C. E.: Detecting the permafrost carbon feedback: talik formation and increased cold-season respiration as precursors to sink-to-source transitions, The Cryosphere, 12, 123–144, https://doi.org/10.5194/tc-12-123-2018, 2018.
Price, A. N., Lindsey, C. R., and Fairley, J. P.: Interpretation of Ground Temperature Anomalies in Hydrothermal Discharge Areas, Water Resour. Res., 53, 10173–10187, 2017.
Racz, A. J., Fisher, A. T., Schmidt, C. M., Lockwood, B. S. and Huertos, M. L.: Spatial and temporal infiltration dynamics during managed aquifer recharge, Ground Water, 50, 562–570, 2012.
Rau, G. C., Andersen, M. S., McCallum, A. M., and Acworth, R. I.: Analytical methods that use natural heat as a tracer to quantify surface water-groundwater exchange, evaluated using field temperature records, Hydrogeol. J., 18, 1093–1110, 2010.
Reichstein, M. and Beer, C.: Soil respiration across scales: The importance of a model-data integration framework for data interpretation, J. Plant Nutr. Soil Sc., 171, 344–354, 2008.
Reusser, D. E. and Zehe, E.: Low-cost monitoring of snow height and thermal properties with inexpensive temperature sensors, Hydrol. Proc., 25, 1841–1852, 2011.
Saba, M., Nishida, Y., Takakura, S., Matsushima, N., and Mogi, T.: Development of geothermal field following the 2000 eruption of Usu volcano as revealed by ground temperature, resistivity and self-potential variations, Ann. Geophys., 50, 1, https://doi.org/10.4401/ag-3088, 2007.
Steele-Dunne, S. C., Rutten, M. M., Krzeminska, D. M., Hausner, M., Tyler, S. W., Selker, J., Bogaard, T. A., and van de Giesen, N. C.: Feasibility of soil moisture estimation using passive distributed temperature sensing, Water Resour. Res., 46, 0043–1397, https://doi.org/10.1029/2009WR008272, 2010.
Strachan, S., Kelsey, E. P., Brown, R. F., Dascalu, S., Harris, F., Kent, G., Lyles, B., McCurdy, G., Slater, D., and Smith, K.: Filling the data gaps in mountain climate observatories through advanced technology, refined instrument siting, and a focus on gradients, Mt. Res. Dev., 36, 518–528, 2016.
Tabbagh, A., Cheviron, B., Henine, H., Guérin, R., and Bechkit, M.-A.: Numerical determination of vertical water flux based on soil temperature profiles, Adv. Water Resour., 105, 217–226, 2017.
Thomas, W.: Experiments on the Freezing of Building Materials, Research Technical Paper no. 17, H. M. Stationery Office, University of Wisconsin, Madison, p. 146, 1938.
Tonina, D., Luce, C., and Gariglio, F.: Quantifying streambed deposition and scour from stream and hyporheic water temperature time series, Water Resour. Res., 50, 287–292, 2014.
Tran, A. P., Dafflon, B., and Hubbard, S. S.: Coupled land surface–subsurface hydrogeophysical inverse modeling to estimate soil organic carbon content and explore associated hydrological and thermal dynamics in the Arctic tundra, The Cryosphere, 11, 2089–2109, https://doi.org/10.5194/tc-11-2089-2017, 2017.
Tran, A. P., Rungee, J., Faybishenko, B., Dafflon, B., and Hubbard, S. S.: Assessment of Spatiotemporal Variability of Evapotranspiration and Its Governing Factors in a Mountainous Watershed, Water, 11, 243, https://doi.org/10.3390/w11020243, 2019.
Turcotte, D. L. and Schubert, G.: Geodynamics, Cambridge university press, 456, ISBN 0521666244, 2002.
Uhlemann, S., Dafflon, B., Peterson, J., Ulrich, C., Shirley, I., Michail, S., and Hubbard, S. S.: Geophysical Monitoring Shows that Spatial Heterogeneity in Thermohydrological Dynamics Reshapes a Transitional Permafrost System, Geophys. Res. Lett., 48, e2020GL091149, https://doi.org/10.1029/2020GL091149, 2021.
Viviroli, D., Dürr, H. H., Messerli, B., Meybeck, M., and Weingartner, R.: Mountains of the world, water towers for humanity: Typology, mapping, and global significance, Water Resour. Res., 43, 0043–1397, https://doi.org/10.1029/2006WR005653, 2007.
Vogt, T., Schneider, P., Hahn-Woernle, L., and Cirpka, O. A.: Estimation of seepage rates in a losing stream by means of fiber-optic high-resolution vertical temperature profiling, J. Hydrol., 380, 154–164, 2010.
Wainwright, H. M., Uhlemann, S., Franklin, M., Falco, N., Bouskill, N. J., Newcomer, M. E., Dafflon, B., Siirila-Woodburn, E. R., Minsley, B. J., Williams, K. H., and Hubbard, S. S.: Watershed zonation through hillslope clustering for tractably quantifying above- and below-ground watershed heterogeneity and functions, Hydrol. Earth Syst. Sci., 26, 429–444, https://doi.org/10.5194/hess-26-429-2022, 2022.
Wielandt, S. and Dafflon, B.: A Local LoRa Based Network Protocol with Low Power Redundant Base Stations Enabling Remote Environmental Monitoring, 2020 54th Asilomar Conference on Signals, Systems, and Computers, 520–523, https://doi.org/10.1109/IEEECONF51394.2020.9443344, 2020.
Zhu, D., Ciais, P., Krinner, G., Maignan, F., Puig, A. J., and Hugelius, G.: Controls of soil organic matter on soil thermal dynamics in the northern high latitudes, Nat. Commun., 10, 1–9, 2019.