the Creative Commons Attribution 4.0 License.
the Creative Commons Attribution 4.0 License.
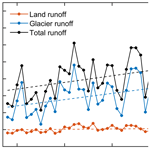
A long-term dataset of climatic mass balance, snow conditions, and runoff in Svalbard (1957–2018)
Veijo Pohjola
Rickard Pettersson
Sergey Marchenko
Jack Kohler
Bartłomiej Luks
Jon Ove Hagen
Thomas V. Schuler
Thorben Dunse
Brice Noël
Carleen Reijmer
The climate in Svalbard is undergoing amplified change compared to the global mean. This has major implications for runoff from glaciers and seasonal snow on land. We use a coupled energy balance–subsurface model, forced with downscaled regional climate model fields, and apply it to both glacier-covered and land areas in Svalbard. This generates a long-term (1957–2018) distributed dataset of climatic mass balance (CMB) for the glaciers, snow conditions, and runoff with a 1 km×1 km spatial and 3-hourly temporal resolution. Observational data including stake measurements, automatic weather station data, and subsurface data across Svalbard are used for model calibration and validation. We find a weakly positive mean net CMB (+0.09 m w.e. a−1) over the simulation period, which only fractionally compensates for mass loss through calving. Pronounced warming and a small precipitation increase lead to a spatial-mean negative net CMB trend (−0.06 m w.e. a−1 decade−1), and an increase in the equilibrium line altitude (ELA) by 17 m decade−1, with the largest changes in southern and central Svalbard. The retreating ELA in turn causes firn air volume to decrease by 4 % decade−1, which in combination with winter warming induces a substantial reduction of refreezing in both glacier-covered and land areas (average −4 % decade−1). A combination of increased melt and reduced refreezing causes glacier runoff (average 34.3 Gt a−1) to double over the simulation period, while discharge from land (average 10.6 Gt a−1) remains nearly unchanged. As a result, the relative contribution of land runoff to total runoff drops from 30 % to 20 % during 1957–2018. Seasonal snow on land and in glacier ablation zones is found to arrive later in autumn (+1.4 d decade−1), while no significant changes occurred on the date of snow disappearance in spring–summer. Altogether, the output of the simulation provides an extensive dataset that may be of use in a wide range of applications ranging from runoff modelling to ecosystem studies.
- Article
(12828 KB) - Full-text XML
- BibTeX
- EndNote
The Arctic climate is changing at a faster rate than the global mean (IPCC, 2014; AMAP, 2017) as a result of climate feedbacks triggered by changing sea-ice cover (Serreze and Barry, 2011; Bintanja and Van Der Linden, 2013). The climate in Svalbard, located at the southwestern boundary of wintertime sea ice and at the northeastern end of the North Atlantic Drift, is primarily controlled by sea-ice cover trends (Divine and Dick, 2006; Day et al., 2012) and trends in prevailing wind direction (Hanssen-Bauer and Førland, 1998; Lang et al., 2015). The homogenized observational air temperature time series from Longyearbyen (1898–2012) reveals a linear trend of 2.6 ∘C per century, with 3–4 times stronger warming in winter–spring than in summer (Nordli et al., 2014). Long-term precipitation records in Svalbard are uncertain due to the local character of measurements and instrumental errors (Førland and Hanssen-Bauer, 2000; Førland et al., 2011), but they show an overall increase that is coherent with large-scale Arctic-wide assessments (e.g. Zhang et al., 2013). Ongoing climate trends strongly affect the state of both glaciers and seasonal snow in Svalbard (e.g. van Pelt et al., 2016a; Østby et al., 2017).
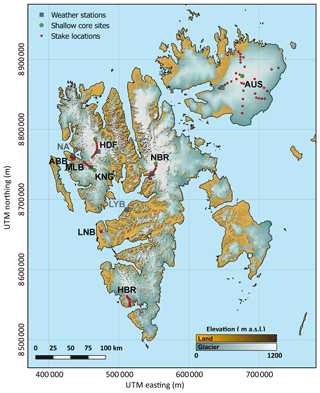
Figure 1Topographic map of Svalbard with different elevation colour maps to distinguish between glacier-covered and land areas. Sites of in situ data collection, including stakes, weather stations, and shallow ice cores, are indicated (ABB: Austre Brøggerbreen; AUS: Austfonna; HBR: Hansbreen; HDF: Holtedahlfonna; KNG: Kongsvegen; MLB: Midtre Lovénbreen; LNB: Linnébreen; NBR: Nordenskiöldbreen; LYB: Longyearbyen; NA: Ny-Ålesund). UTM coordinates in this and later figures are in zone 33 X. The digital elevation model and mask used to produce the map are described in Sect. 2.1, and an overview of the observational data is given in Table 1.
In response to warming, glaciers in Svalbard with a current estimated volume of ∼6200 km3 (1.5 cm sea level equivalent; Fürst et al., 2018), and area of 33 775 km2 (∼57 % of the total area of Svalbard; Fig. 1), have in recent decades shrunk by ∼80 km2 a−1 (Nuth et al., 2013), primarily due to low-elevation thinning and associated retreat (e.g. Moholdt et al., 2010; Nuth et al., 2012). Total glacier mass balance is the sum of frontal ablation, basal ablation, and the climatic mass balance (CMB), representing the mass change due to atmosphere–surface–snowpack interactions (Cogley et al., 2011). CMB measurements in Svalbard started on Austre Brøggerbreen (since 1967), followed by Midtre Lovénbreen (since 1968), both in northwestern Svalbard. Since the 1980s, CMB monitoring has also extended to southern, central, and northeastern Svalbard (Fig. 1, Table 1). Although a negative trend in CMB is apparent for most observed glaciers, the scarcity of the data in space and time does not allow for a detailed estimation of long-term CMB trends for different regions in Svalbard. To overcome this, CMB models, commonly forced with regional climate model or reanalysis fields, have previously been applied to individual glacier basins (e.g. Rye et al., 2012; van Pelt et al., 2012; Möller et al., 2013; van Pelt and Kohler, 2015) as well as for all glaciers in Svalbard (e.g. Lang et al., 2015; Aas et al., 2016; Østby et al., 2017; Möller et al., 2016; Möller and Kohler, 2018). The use of different CMB models, climate forcings, model calibration, and spatial resolution has resulted in a relatively large spread of multi-decadal Svalbard-wide mean CMB and trends in CMB in available literature. For example, Lang et al. (2015) report a negligible CMB trend for 1979–2013, while Østby et al. (2017) report a strong CMB decline over the same period and the longer period 1957–2014. As a result, despite confirmed significant warming in Svalbard since the 1960's (Nordli et al., 2014), its impact on glacier CMB remains poorly constrained.
Recent climate warming not only has a major impact on glaciers, but also exerts a strong influence on the state of seasonal snow in the glacier-free parts of Svalbard. Previous work has shown that despite a modest increase in Arctic precipitation in recent decades (Zhang et al., 2013; Bintanja and Selten, 2014), the duration of the snow-free season is increasing and the area with a permanent snow cover is declining (van Pelt et al., 2016a). It has also been shown that thick ice layers may form in snowpacks during winters with heavy rainfall events, thereby limiting reindeer access to food supplies and leading to population declines (Kohler and Aanes, 2009; Hansen et al., 2014). Formation of ice at the base of seasonal snowpacks has been projected to increase in a future climate (Hansen et al., 2011), as the fraction of precipitation falling as rain is rising (Bintanja and Andry, 2017). In situ snow observations by means of probing, snow pits, ground-penetrating radar and remote sensing, have been extensively used to assess local-scale patterns and evolution of seasonal snow in Svalbard (e.g. Hagen et al., 2003; Winther et al., 2003; Grabiec et al., 2011; van Pelt et al., 2014), but provide only limited insight into snowpack dynamics at large spatial and temporal scales.
In this study, we use a coupled surface energy balance–multilayer subsurface model (van Pelt et al., 2012, 2016b) and apply it to all of Svalbard to generate a model dataset with a 3-hourly temporal and 1 km×1 km spatial resolution for the period 1957–2018. In contrast to previous large-scale coupled modelling of glaciers in Svalbard (Lang et al., 2015; Aas et al., 2016; Østby et al., 2017), we apply our model to both glacierized and glacier-free terrain. Furthermore, we implement improved model physics, and adopt new techniques for climate downscaling and calibration (Sect. 3). Two different model setups are chosen to enable simulation of deep subsurface conditions for the glacier-covered part and detailed seasonal snowpack evolution on permafrost for the land part. In situ data of stake mass balance, automatic weather stations, and snow conditions (Sect. 2) are used for model calibration and validation (Sect. 3). In Sect. 4 we present and discuss spatial patterns and trends of CMB, snow and firn conditions on glaciers, and seasonal snow conditions on land, which allows for a detailed and unprecedented quantification of seasonal snow and glacier contributions to total discharge from the Svalbard archipelago. The output dataset provides crucial input data for further cryospheric analyses, and may serve as input for studies of marine and terrestrial ecosystems.
In this section we describe the data used as model input (Sect. 2.1), for model calibration (Sect. 2.2) and for validation of model results (Sect. 2.3). An overview of all observational data used is given in Table 1.
Table 1Overview of in situ observational data used in this study. The number of stake locations per glacier are indicated in brackets in the second column. Location abbreviations are shown and described in Fig. 1. Variable names are introduced in the text. Other abbreviations: s: summer, w: winter, C: calibration, V: validation, NPI: Norwegian Polar Institute.
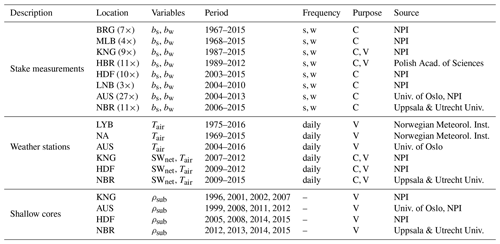
2.1 Input data
A digital elevation model (DEM) with a 20 m spatial resolution, provided by the Norwegian Polar Institute (S0 Terrengmodel Svalbard), has been averaged onto a 1 km resolution grid for the model experiments. Resulting elevations range from sea level to 1552 m a.s.l. (the actual highest point on Svalbard is 1717 m). Glacier outlines were extracted from the GLIMS database (Global Land Ice Measurements from Space; König et al., 2014) and used to split the terrain into land and glacier-covered areas (Fig. 1) and to estimate equilibrium line altitudes for individual glacier basins. Glacier outlines correspond to the period 2001–2010, while the data behind the DEM were collected during 1990–2010. We assume fixed elevations and glacier mask over the simulation period to produce surface mass balance and related quantities for a reference surface (Elsberg et al., 2001).
To generate meteorological forcing fields of air temperature, precipitation, cloud cover, relative humidity, and air pressure, we use 3-hourly output from the High Resolution Limited Area Model (HIRLAM) regional climate model (NORA10 dataset; Norwegian Meteorological Institute; Reistad et al., 2011), covering the period 1957–2018. HIRLAM is forced by European Centre for Medium Range Weather Forecasts (ECMWF) reanalyses (ERA40 until 2002, ECMWF operation analysis since 2002; Uppala et al., 2005; Reistad et al., 2011). HIRLAM fields with an original 10 km resolution were downscaled to the 1 km model grid resolution using parameter-specific downscaling techniques (van Pelt et al., 2016a). All meteorological variables were first linearly interpolated onto the 1 km grid, before additionally applying elevation corrections for temperature (time-dependent lapse rate), precipitation (fixed linear fractional increase with elevation), and air pressure (time-dependent exponential decay with elevation). Elevation functions for temperature and air pressure were constructed per 3 h time step through respectively linear and exponential regression of the regional climate model values and their corresponding elevations; this procedure was repeated for blocks of 4×4 grid cells and regression coefficients were averaged for the whole grid to obtain a single lapse rate for temperature and exponential decay coefficient for air pressure per time step. Average temperature and precipitation as well as corresponding long-term linear trends are shown in Fig. 2. Throughout the paper temporal trends were calculated by means of linear regression of annual time series; non-significant trends at a 95 % confidence interval were set to zero and appear as grey in the associated figures. Throughout the paper, significant means that a zero slope is not included in the 2σ-confidence bounds of a trend. The long-term mean temperature distribution (Fig. 2a) reveals the highest temperatures at low-elevation sites in the southwest and the lowest temperatures at high elevations on the Lomonosovfonna ice cap in central Svalbard. Temperature trends are significantly positive for the whole of Svalbard, with the most pronounced trends in the northeast (Fig. 2b). The long-term mean precipitation distribution shows a clear elevation dependence (Fig. 2c), while long-term trends are generally found to be non-significant, except in the north, where there is a significant positive trend (Fig. 2d).
2.2 Calibration data
For model calibration, we use records of summer and winter balance (bs, bw) from stake measurements and net shortwave radiation (SWnet) observed at three automatic weather stations (Table 1).
Stake heights for a set of glaciers around Svalbard are recorded once or twice per year and, in combination with snow density and snow depth data, are converted into summer balance and winter balance estimates. Here, we use data from 82 stake locations in Svalbard, covering eight different glaciers and ice caps (Fig. 1). The Norwegian Polar Institute has collected stake data on a set of glaciers in western Svalbard, including Austre Brøggerbreen (ABB), Midtre Lovénbreen (MLB), Kongsvegen (KNG), Holtedahlfonna (HDF), and Linnébreen (LNB); the oldest record (ABB) dates back to 1967 (e.g. Hagen et al., 1999; Kohler et al., 2007). Stake data on Hansbreen (HBR) have been collected by the Institute of Geophysics, Polish Academy of Sciences since 1989 (Grabiec et al., 2012). The University of Oslo and Norwegian Polar Institute have made stake measurements on Austfonna since 2004 (e.g. Moholdt et al., 2010; Aas et al., 2016). Stake measurements on Nordenskiöldbreen were initiated in 2006 by Uppsala and Utrecht universities (e.g. van Pelt et al., 2012, 2018). Derived net glacier-wide mass balances of ABB, KNG, HDF, and HBR are included in the World Glacier Monitoring Service database (WGMS; https://wgms.ch/, last access: 6 November 2018).
For ABB, MLB, and LNB, the dense observation network caused several stake sites to fall within one 1 km×1 km model grid cell, in which case we only selected the stake location closest to model grid nodes for further comparison with the model results. As a result, we include only four (out of 10) stakes on MLB, seven (out of 11) on BRG, and three (out of eight) stakes on LNB. The winter balance data for the same set of glaciers were previously described and used in van Pelt et al. (2016a). Summer balance is estimated using information of spring (April) and end-of-summer (September) surface height, while spring snow depth is used to distinguish between snow and ice melt. In absence of direct end-of-summer surface height measurements, the depth of the summer surface was inferred from subsequent spring stake height and snow depth data. In the accumulation zone refreezing above the summer surface is accounted for by setting an assumed end-of-summer remaining snow density (van Pelt et al., 2018). For calculating summer and winter balance from the model output, we use fixed dates of 15 April and 1 September, corresponding to average dates for spring stake data collection and end-of-summer minimum surface height, respectively.
In situ data of SWnet (Table 1), i.e. incoming minus reflected solar radiation, are extracted from radiation measurements at automatic weather stations in central Svalbard (NBR; van Pelt et al., 2012) and western Svalbard (KNG and HDF; Karner et al., 2013; van Pelt and Kohler, 2015; Pramanik et al., 2018).
2.3 Validation data
In addition to the in situ data used for model calibration, we further use observed density profiles from shallow cores and air temperature time series observed at (automatic) weather stations for validation of model results.
Shallow cores were drilled during multiple years at four locations in the accumulation zones on KNG (722 m a.s.l.), HDF (1122 m a.s.l.), NBR (1187 m a.s.l.), and AUS (758 m a.s.l.) to obtain density profiles with maximum depths ranging from 7 to 15 m below the surface (Fig. 1; Table 1). For each of the four sites we selected four firn density profiles, collected during different years on NBR (2012, 2013, 2014, and 2015), KNG (1996, 2001, 2002, and 2007), HDF (2005, 2008, 2014, and 2015), and AUS (1999, 2008, 2011, and 2012). Bulk densities are calculated over the full depth of observations and compared to simulated values over the same depth intervals.
We use a combination of air temperature records from the automatic weather stations on the glaciers AUS (Schuler et al., 2014), NBR (van Pelt et al., 2012), and KNG and HDF (e.g. Karner et al., 2013), as well as from two land-based meteorological stations in Longyearbyen and Ny-Ålesund (data provided by the Norwegian Meteorological Institute through the eKlima data portal) for comparison with downscaled temperatures (Fig. 1; Table 1).
3.1 Coupled modelling
A coupled modelling system is used to simulate surface and near-surface mass and energy exchange (van Pelt et al., 2012), which has been used previously to simulate glacier mass balance, (seasonal) snow development, and/or runoff in western Svalbard (e.g. van Pelt and Kohler, 2015; Vallot et al., 2017, 2018; How et al., 2017; Winsvold et al., 2018; Deschamps-Berger et al., 2019; Köhler et al., 2019), central Svalbard (e.g. Vega et al., 2016; Marchenko et al., 2017b; van Pelt et al., 2018), and on an idealized Svalbard glacier (van Pelt et al., 2016b). In this study, the model is applied for the first time to the whole of Svalbard. At the surface, an energy balance model determines radiative (short- and longwave) and turbulent (latent and sensible) heat fluxes, and accounts for conductive heat exchange with the underlying medium, in order to calculate surface temperature and melt. Solving the surface energy balance requires input of near-surface meteorological conditions, including air temperature, cloudiness, relative humidity, air pressure, and precipitation (van Pelt et al., 2012). No wind information is needed since sensible and latent heat exchange depend solely on near-surface temperature and specific humidity gradients, following katabatic turbulent exchange relations by Oerlemans and Grisogono (2002). A multilayer subsurface model simulates temperature, density, and water content, while accounting for snow compaction, water transport, refreezing, heat conduction, irreducible water storage, and runoff. To model seasonal snow in glacier-free terrain, the subsurface model has been extended with a soil routine (Westermann et al., 2011) to simulate permafrost thawing and freezing and heat exchange within the soil and between the soil and overlying snowpack (if present), as described in Pramanik et al. (2018). Potential local impacts of (sparse) vegetation or surface roughness on the surface energy balance in land areas are neglected.
New in the model code used in this study, with respect to the most recent model application in Pramanik et al. (2018), is the incorporation of a new percolation scheme (Marchenko et al., 2017b), as well as the implementation of an updated albedo scheme. A deep water percolation scheme, inspired by subsurface temperature measurements on the Lomonosovfonna ice cap (Marchenko et al., 2017b), has recently been implemented to mimic the effects of preferential flow pathways in snow/firn. Additionally, we have extended the original snow-age- and snow-depth-dependent albedo scheme (Oerlemans and Knap, 1998). The original fixed characteristic timescale for exponential decay of snow albedo due to ageing has been replaced with a temperature-dependent timescale (t*). As in Bougamont et al. (2005), snow albedo decays fastest when the surface is melting ( d), and for dry snow t* linearly increases from 30 to 100 d between 0 and −10 ∘C. The updated albedo scheme avoids overestimation of the albedo of melting surfaces in the early melt season. Other albedo parameters, including the albedo of ice (0.39), albedo of firn (0.52), and the characteristic snow depth for albedo decay of thin snow covers (7 mm w.e.), were taken as in van Pelt and Kohler (2015). To avoid potential systematic biases resulting from the new albedo scheme, we have included the fresh snow albedo (αfs) and minimum snowfall threshold used to reset the snow albedo to the fresh snow albedo (Pth) in the calibration process, as described in Sect. 3.2.
The climatic mass balance refers to the sum of the surface mass balance and internal mass balance (Cogley et al., 2011) and thereby accounts for internal accumulation, i.e. refreezing and liquid water storage below the previous summer surface. Here it is calculated as the sum of mass fluxes at the surface, including precipitation (+) and moisture exchange (), and mass loss through runoff (−) at the transition of snow/firn to ice (i.e. at the surface in absence of snow). No horizontal exchange of liquid water is accounted for; i.e. runoff is assumed to occur locally.
The simulation covers the period from 1 September 1957 to 31 August 2018 with a 3-hourly temporal resolution on a distributed 1 km resolution grid. We initialize the simulation by performing a 25-year spin-up using input data for the period 1957–1982, to generate initialized subsurface conditions. The subsurface model uses a Lagrangian grid to avoid numerical diffusion; surface mass fluxes due to precipitation, melt, and moisture exchange induce thickness changes in the uppermost model layer with a thickness between 0 and 0.1 m. For both glacier-covered and land grid cells, a vertical grid consisting of 50 vertical layers is used. On glaciers, layer thickness doubles at the 15th, 25th, and 35th layers through layer merging/splitting to yield vertical layer thicknesses from <0.1 to 0.8 m down to a depth of up to 20 m below the surface. In land areas, a fixed (initial) layer thickness of 0.1 m is used, extending to a depth of up to 5 m below the surface. Snow layer thickness gradually decreases over time due to snow compaction, which results in a lower total depth for grid cells with deep snow/firn columns. A central differencing scheme is used to simulate heat conduction, in which adaptive time-stepping assures stability; a zero heat flux is assumed at the lower boundary (van Pelt and Kohler, 2015).
3.2 Calibration
Extensive calibration of energy balance model parameters in applications on Svalbard has previously been described in van Pelt et al. (2012) and van Pelt and Kohler (2015). Here, we use the parameter setup as described in van Pelt and Kohler (2015), and only recalibrate constants to which melt rates have previously been found to be most sensitive, including the background turbulent exchange coefficient (Cb), the snow-to-rain transition temperature (Tsr), the fresh snow albedo (αfs), and the snowfall threshold at which the albedo is reset to the fresh snow albedo (Pth). Additionally, since the simulated climatic mass balance is highly sensitive to the downscaling of precipitation from the regional climate model grid onto the 1 km×1 km model grid, we also calibrate the precipitation downscaling function.
In the first calibration step, multi-year records of SWnet observations from KNG, HDF, and NBR (Table 1) were used to collectively calibrate αfs and Pth. Since we aim to calibrate only fresh snow albedo and minimum snowfall to reset to the fresh snow albedo, we have selected SWnet measurements for the period April to June, when melt effects on albedo are limited, but solar insolation is high. A two-parameter exploration revealed a lowest-average root-mean-square error (RMSE) between modelled and observed daily SWnet of 14.9 W m−2 for the three glaciers when choosing αfs=0.83 and Pth=0.1 mm w.e. h−1. RMSE values ranged from a minimum 14.1 W m−2 on NBR to maximum 15.6 W m−2 on HDF, suggesting consistent performance on the three glaciers.
In the second calibration step, stake winter balance data from eight glaciers in Svalbard (Fig. 1; Table 1) were used to calibrate coefficients in the function used to project precipitation from the coarser regional climate model grid onto the finer model grid. The function describes the distribution of precipitation accounting for local topography not captured by the regional climate model, and is formulated as an elevation-dependent relation following van Pelt et al. (2016a):
where Pr is corrected precipitation, z is elevation, Pr0 and z0 are spatially interpolated precipitation and elevation from the regional climate model grid onto the 1 km grid, and K1 and K2 are calibration coefficients. While K1 is used to correct for potential biases in the regional climate model precipitation, K2 represents the local precipitation–elevation gradient, which, since it is a fractional (or relative) coefficient, generates steeper absolute precipitation–elevation gradients in regions with higher overall precipitation amounts. Values from the 1 km×1 km DEM (z) will contain more detail than the z0 values interpolated from the coarser regional climate model grid; any positive deviation of the surface height () will lead to a positive correction of the local precipitation, while a negative height deviation () will lead to a negative precipitation correction. With this approach, we account for the effect of local topography on precipitation, thereby capturing the impact of orographic lifting at scales smaller than the resolution of the regional climate model. In addition to compensation for biases in modelled precipitation (by calibrating K1), potential surface height discrepancies at spatial scales of 10 km and greater that may arise from the use of a different DEM in the regional climate model are also automatically compensated for. Using a total of 1438 stake winter balance measurements between 1967 and 2015, we performed a two-parameter search to find optimum values for K1 (1.11) and K2 (0.0022 m−1) for which a minimum RMSE of 0.23 m w.e. was found between modelled and observed winter balance (Fig. 3, Table 2). These values imply that we apply an 11 % bias correction to the regional climate model precipitation and a local precipitation lapse rate of 22 % per 100 m to correct for the orographic effect due to local topography. Precipitation is set to not increase further above 900 m a.s.l., in line with an observed negligible elevation gradient of bw above this elevation on Lomonosovfonna, central Svalbard (van Pelt et al., 2014), and Holtedahlfonna, western Svalbard (van Pelt and Kohler, 2015). Elevations on the 1 km×1 km grid do not exceed 900 m a.s.l. in southern and northeastern Svalbard.
Table 2Comparison of simulated and observed bw, bs, and bn after calibration. Biases and RMSE values for all data are given in bold.
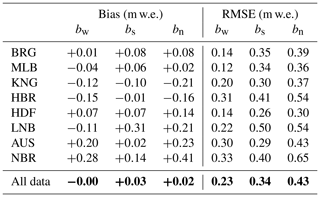
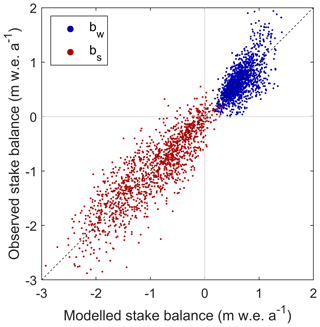
Figure 3Simulated vs. observed summer and winter balance for all available stake data from eight glaciers (Table 1).
The final calibration step uses the stake summer balance data to optimize two parameters (Cb and Tsr) that have a strong impact on summer melt and the summer balance, while the impact on winter balance is small. A two-parameter exploration revealed a minimum RMSE of 0.34 m w.e. between modelled and observed summer balance for a total of 1341 observations between 1967 and 2015 (Fig. 3) when choosing values for Cb=0.0025 and Tsr=0.6 ∘C.
Altogether, comparing modelled and observed net mass balance reveals an RMSE of 0.43 m w.e for all data (Table 2). For comparison, Østby et al. (2017) previously reported an RMSE of 0.59 m w.e. using a similar set of stake data for calibration. Contributing errors to the net mass balance RMSE include uncertainty in stake readings and bulk density estimation, model physics, climate forcing, and uncertainty in comparing observed point values with simulated spatially averaged values – the latter is particularly significant for locations where wind has a major impact on the snow distribution (e.g. van Pelt et al., 2014). After calibration, remaining biases (modelled minus observed; calculated as the mean absolute difference) of the winter, summer, and net balance are −0.00, +0.03, and +0.02 m w.e., respectively, for all data, which implies low systematic errors for long-term area-averaged climatic mass balance. Comparing net mass balance for individual glaciers reveals biases ranging from −0.21 m w.e. (KNG) to +0.41 m w.e. (NBR), while RMSE is found to range from 0.30 m w.e. on HDF to 0.65 m w.e. on NBR (Table 2). Overall, we find the largest errors for NBR in central Svalbard, which is primarily caused by a substantial overestimation of bw, which in turn also induces an overestimation of bs (underestimation of summer melt) due to a snow–albedo feedback. It is known that snow accumulation on NBR is highly influenced by wind-driven snow redistribution and erosion (van Pelt et al., 2014). This may explain the overestimation of snow accumulation in our modelling of NBR since effects of wind on snow accumulation are not accounted for in the downscaling of regional climate model precipitation. On the other hand, underestimation of bw is apparent for KNG and HBR (Table 2), which results from underestimated orographic precipitation at high elevations on these glaciers. Nevertheless, high-elevation biases of bw do not arise on the two glaciers extending above 1000 m a.s.l. (HDF and NBR), which indicates that the bw offsets on KNG and HBR are not a systematic feature for high-elevation sites in general. The relative lack of stake observations at heights above 1000 m a.s.l. implies increased uncertainty of modelled precipitation estimates at these elevations (Möller et al., 2016).
3.3 Validation
To assess how well the model is able to simulate time evolution of glacier-wide CMB, we compare simulated glacier-average winter CMB (Bw), summer CMB (Bs), and net CMB (Bn) for HBR and KNG against observation-based estimates from the WGMS database (Fig. 4). The long-term WGMS records in Svalbard from BRG and MLB are excluded due to a lack of model grid cells falling within the glacier outlines (nine for BRG and five for MLB); model grids of HBR and KNG include 66 and 110 grid cells, respectively. Simulated annual net CMB values show good agreement with the WGMS values for both KNG (R=0.86; P<0.001; RMSE =0.18 m w.e. a−1) and HBR (R=0.67; P<0.001; RMSE =0.27 m w.e. a−1). Furthermore, long-term simulated and observed net CMB trends are consistent for both KNG (modelled m w.e. a−1 decade−1; observed m w.e. a−1 decade−1) and HBR (modelled 0.02±0.20 m w.e. a−1 decade−1; observed m w.e. a−1 decade−1).
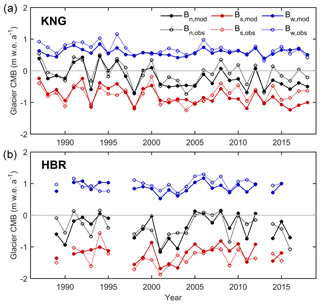
Figure 4Comparison of simulated glacier-wide summer, winter and net mass balance against WGMS records for KNG (a) and HBR (b).
Air temperature and precipitation are the main meteorological drivers of spatial patterns and trends in CMB and its components. As discussed in Sect. 3.1, the downscaling of precipitation has been optimized using in situ winter balance data from multiple sites in Svalbard. Here, we validate the temperature forcing by comparing downscaled daily 2 m temperature with in situ temperature records (recorded at 1–4 m heights) from six sites in Svalbard (Table 1; Sect. 2.3). Results are summarized in Table 3. We find very high correlations (R=0.95–0.97; P<0.001), RMSE ranging between 2.0 ∘C (KNG) and 4.6 ∘C (HDF), and biases ranging from −2.3 ∘C (AUS) to +0.7 ∘C (KNG). In general, we find good agreement between downscaled and observed temperatures for both glacier and non-glacier terrain in different regions in Svalbard. The largest bias and RMSE are found at AUS in northeast Svalbard, which can be ascribed to a substantial underestimation of air temperature during the cold season (September–May) of −3.2 ∘C, whereas the summer (June–August) air temperature bias is small (+0.4 ∘C).
Table 3Comparison of downscaled and observed air temperatures at glacier- and land-based weather stations.
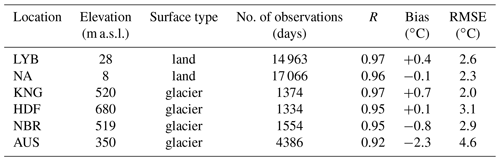
Finally, in situ observations from shallow cores (Sect. 2.3) are used to validate bulk density (ρsub) simulated at AUS, HDF, NBR, and KNG during 4 years down to depths of 7–15 m (Table 1). For three sites, we find negative model biases for ρsub of −25 kg m−3 (NBR), −30 kg m−3 (AUS), and −38 kg m−3 (HDF). On KNG, a positive bias of +48 kg m−3 is found. Table 2 shows that KNG is the only site of the four experiencing a negative bw bias. Based on this, we argue that an underestimation of accumulation explains the overestimation of ρsub at KNG, and vice versa at NBR, AUS, and HDF. An inverse relation between ρsub and accumulation follows from (1) the parametrization used for gravitational settling (Ligtenberg et al., 2011) and (2) an increased significance of refreezing on the vertical density distribution where accumulation rates are low (subsurface layers remain closer to the surface for a longer time and will hence experience refreezing of stored water in the cold season during more years).
In this section, we present and discuss spatial patterns and trends of simulated CMB, equilibrium line altitude (ELA), subsurface conditions, refreezing, and runoff over the period 1957–2018.
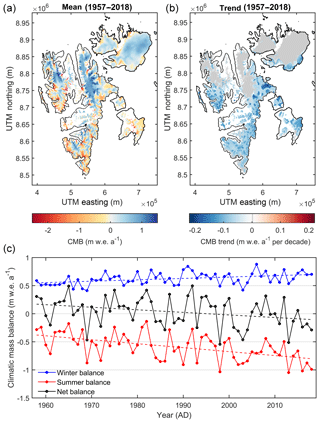
Figure 5Long-term mean spatial CMB distribution (a) and trends (b). In (c) time series of area-averaged annual mean summer, winter, and net CMB (solid lines) and linear trends (dashed lines) are shown. In (c) years are defined based on a mass balance year between 1 September (preceding year) and 31 August.
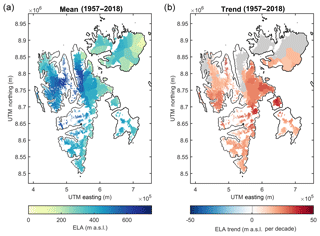
Figure 6Long-term mean spatial ELA distribution (a) and trends (b). Data are averaged per glacier basin, based on the glacier outline database in König et al. (2014).
4.1 Climatic mass balance & ELA
Averaged over the entire simulation period, we find a spatial mean glacier net CMB (hereafter just CMB) of +0.09 m w.e. a−1, which is comparable to Østby et al. (2017) (+0.08 m w.e. a−1 over the period 1957–2014) and more positive than a recent estimate by Möller and Kohler (2018) (−0.03 m w.e. a−1 over the period 1957–2010). The spatial CMB distribution in Fig. 5a reveals most negative CMB values (down to −2.5 m w.e. a−1) at low elevations in southern and western Svalbard, and most positive CMB values (up to 1.3 m w.e. a−1) at high-elevation sites on the Lomonosovfonna ice cap (central Svalbard). Assuming a frontal ablation rate equivalent to −0.18 m w.e. a−1 (Blaszczyk et al., 2009), and negligible basal melting, we estimate a total mass balance of −0.09 m w.e. a−1. In the latter calculation it is implicitly assumed that frontal ablation rates from Blaszczyk et al. (2009) for the early 2000s apply during the whole simulation period. We find significantly negative CMB trends in southern and central Svalbard, while trends are not significant in the north (Fig. 5b). On average, a significantly negative CMB trend of m w.e. a−1 decade−1 is found (Fig. 5c). For comparison, a more negative trend of −0.14 m w.e. a−1 decade−1 was reported by Østby et al. (2017) over the period 1957–2014, although it was argued that the trend may have been overestimated based on a comparison of long-term CMB at a single stake site on MLB. Conversely, Lang et al. (2015) found a weaker negative CMB trend (−0.03 m w.e. a−1 decade−1) for 1979–2013, which is however not significantly different from our trend of m w.e. decade−1 over the same period. Significant trends of opposite sign are found for the winter balance ( m w.e. a−1 decade−1) and summer balance ( m w.e. a−1 decade−1), suggesting that a winter accumulation increase compensates for some of the increased summer ablation. Inter-annual variability of net CMB correlates strongly with both summer (June–August) air temperature (R=0.78; P<0.001) and annual (September–August) precipitation (R=0.60; P<0.001), while no significant correlation exists between annual temperature and net CMB (R=0.10; P>0.1).
The average ELA of the entire glacierized area in Svalbard is 367 m a.s.l. for 1957–2018. The ELA distribution resembles an earlier observation-based map by Hagen et al. (2003) with the highest ELA (>700 m a.s.l.) in relatively dry regions in northern Spitsbergen and the lowest ELA (<200 m a.s.l.) induced by cold conditions in northeastern Svalbard (Fig. 6a). Significant positive ELA trends are apparent for all of Svalbard, except for the most northern parts (Fig. 6b), where increased precipitation (Fig. 2d) offsets an ELA increase due to a melt increase. Based on annual ELA time series, we find a significant mean positive ELA trend of 17±12 m a.s.l. decade−1, which is slightly less than a previously reported trend of 25 m a.s.l. decade−1 over 1961–2012 in van Pelt et al. (2016a). As a result of upward ELA migration, the accumulation area ratio (AAR) has decreased at an absolute rate of −4 % per decade−1; the average AAR for 1957–2018 equals 65 % with annual values ranging from 17 % (1997–1998) to 91 % (1964–1965). As previously discussed in van Pelt and Kohler (2015), surface melt is amplified due to substantial lowering of the albedo in the new ablation areas exposed by the retreating ELA. The average albedo over the simulation period is 0.76 for all glaciers in Svalbard, with a significant negative trend of decade−1 (locally down to −0.024 decade−1), inducing an average 2 % decade−1 increase in absorbed solar radiation.
4.2 Glacier subsurface conditions
As a collective measure of density and depth of snow and firn in glacierized areas, we quantify the total pore space down to a depth of 14 m below the surface (P14), expressed in m3 m−2, and shown in Fig. 7a, b. Large accumulation zones with P14 exceeding 5 m3 m−2 are found at high elevations on the three major ice caps in northern Svalbard (Holtedahlfonna, Lomonosovfonna, and Austfonna); smaller accumulation zones with generally lower P14 prevail in southern Svalbard (Fig. 7a). Trends in P14 (Fig. 7b) are most negative (down to −0.6 m3 m−2 decade−1) in elevation bands close to the long-term mean ELA, as upward migration of the firn line causes a major decline in firn depth. As a result, the most negative P14 trends are found in central Svalbard, where ELA trends are most positive (Fig. 6b). For 1957–2018, average P14 for the glacierized area, i.e. including both ablation and accumulation zones, equals 2.3 m3 m−2; the average trend is significantly negative ( m3 m−2 decade−1) and equivalent to a 4 % decrease in P14 per decade.
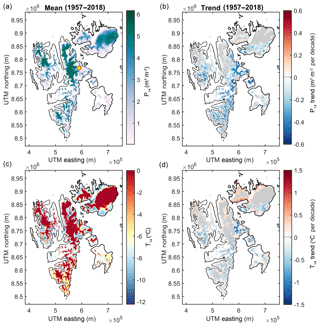
Figure 7Long-term mean P14 distribution (a) and trends (b). Long-term mean T14 distribution (c) and trends (d). The corresponding location of the subsurface profiles in Fig. 8 is marked with a red circle in (a).
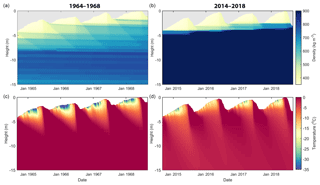
Figure 8Subsurface density (a, b) and temperature (c, d) evolution during the periods 1964–1968 (a, c) and 2014–2018 (b, d). The corresponding geographic location of the site is indicated in Fig. 7a.
The distribution and trends of deep temperature (T14), defined here as the temperature at 14 m below the surface, are shown in Fig. 7c, d for the glacierized area of Svalbard. The T14 distribution reveals a marked transition around the ELA from cold (non-temperate) conditions in the ablation zones to temperate conditions in accumulation areas for all glaciers in Svalbard. This thermodynamic structure is common for Svalbard glaciers (Björnsson et al., 1996; Pettersson, 2004), and has previously been linked to the high significance of (deep) percolation and refreezing in accumulation zones (e.g. van Pelt et al., 2012, 2016b). Temperate T14 conditions also precondition the potential formation of perennial firn aquifers, which have been detected using ground-penetrating radar on Holtedahlfonna in western Svalbard (Christianson et al., 2015), and recently also on Lomonosovfonna in central Svalbard (Rickard Pettersson, unpublished data). The widespread occurrence of temperate deep firn suggests the likelihood of perennial firn aquifers in other accumulation zones on Svalbard. On Austfonna, a radar survey in 2014 showed a strong reflector over large distances across the summit area, which potentially results from deep slush water storage (Thorben Dunse, unpublished data). In addition to temperature, other factors affecting firn aquifer development include surface topography (steering water flow in the aquifer), and the potential for englacial drainage through cracks, crevasses, and moulins. Our results suggest that even the highest (coldest) accumulation zones in Svalbard have average temperate deep firn conditions. This is in line with recent measurements (2012–2015) on Lomonosovfonna at 1200 m a.s.l. (Marchenko et al., 2017b), but does not agree with earlier findings of sub-temperate conditions at ice core drill sites on Lomonosovfonna in 1997 (Van de Wal et al., 2002) and Holtedahlfonna in 2005 (Beaudon et al., 2013). However, we infer that both these drill sites were likely drilled in locations with isolated cold deep temperature conditions within otherwise temperate accumulation zones, as confirmed by the widespread presence of perennial firn aquifers. Cold deep temperature conditions may occur locally at wind-exposed sites, e.g. on an ice divide or ridge, as accumulation rates are typically lower due to wind erosion, which has a cooling effect on deep firn (Kuipers Munneke et al., 2014). Additionally, we infer that the convex topography of ice divides promotes efficient drainage and reduces the significance of latent heat release by refreezing. For both drill sites, reported accumulation rates estimated from the ice cores of 0.41 m w.e. a−1 (Lomonosovfonna, 1950–1997, Pohjola et al., 2002) and 0.50 m w.e. a−1 (Holtedahlfonna, 1963–2005, Van der Wel et al., 2011) are indeed substantially lower than observed at the nearest stakes on Holtedahlfonna (0.98 m w.e. a−1 for 2003–2015) and Lomonosovfonna (0.85 m w.e. a−1 for 2006–2015). Long-term trends of T14 (Fig. 7d) reveal a warming trend in ablation zones and a cooling trend in former accumulation zones that recently became ablation zone due to upward migration of the firn line; the average trend is weakly negative ( ∘C decade−1).
An example of firn density and temperature evolution during two periods (1964–1968 and 2014–2018) at a site close to the long-term mean ELA in central Svalbard is shown in Fig. 8 (location indicated in Fig. 7). During 1964–1968, deep temperature is consistently at the melting point (Fig. 8c) and no thick ice layers are present in the upper 10–15 m of firn (Fig. 8a). During 2014–2018, the same site is still in the lower accumulation zone, but now firn density is markedly increased, with impermeable ice below a depth of 1–3 m below the surface (Fig. 8b). It is noteworthy that similar firn developments have recently been observed in the lower accumulation zone in western Greenland (Cox et al., 2015; Machguth et al., 2016), the Canadian Arctic (Bezeau et al., 2013), and the Larsen C ice shelf, Antarctica (Hubbard et al., 2016; Bevan et al., 2017) and have been argued to potentially affect horizontal drainage. As firn densifies, percolating water more readily runs off, and the potential for deep water storage and subsequent refreezing is reduced. In response to reduced refreezing, as well as faster heat conduction, deep firn/ice temperatures during 2014−2018 are no longer temperate at the site (Fig. 8d).
4.3 Refreezing
The distribution of refreezing for both glacier-covered and land areas reveals that the highest refreezing rates (up to 0.41 m w.e. a−1) are in the accumulation zones (Fig. 9a), where percolating water can be stored deep in snow/firn and refreeze over the course of the winter season (van Pelt et al., 2016b). The lowest refreezing rates (<0.05 m w.e. a−1) are at low elevations, i.e. in coastal regions and valleys, where thin seasonal snowpacks develop over winter, thereby limiting the potential for refreezing. For 1957–2018, we find average refreezing rates of 0.24 and 0.14 m w.e. a−1 for the glacier-covered and land areas, respectively. For comparison, Østby et al. (2017) found comparable refreezing rates of 0.22 m w.e. a−1 for all glaciers in Svalbard during 1957–2014. Long-term refreezing trends (Fig. 9b) reveal significantly decreasing refreezing rates (down to −0.03 m w.e. decade−1) primarily at elevations around the ELA in response to firn line retreat. No significant trends of refreezing are found in high accumulation zones, which implies the likely growth of perennial firn aquifers during the simulation period since input from surface melt and rainfall shows a clear positive trend ( m w.e. decade−1). On average, we find comparable negative trends for the glacier-covered areas ( m w.e. a−1 decade−1) and land areas ( m w.e. a−1 decade−1), implying a much faster relative decrease in refreezing on land (−6.0 % decade−1) than on glaciers (−2.9 % decade−1).
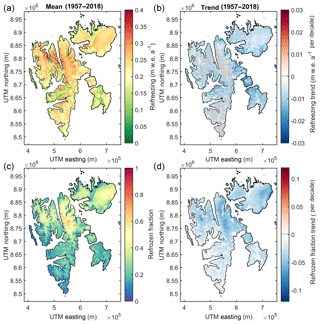
Figure 9Long-term mean spatial refreezing distribution (a) and trends (b). Long-term mean spatial refrozen fraction distribution (c) and trends (d).
The fraction of melt and rain that refreezes, i.e. the refrozen fraction, is on average 0.27 (Fig. 9c), implying a substantial reduction of runoff. It should however be acknowledged that indirect effects after refreezing, in particular heat release in the snowpack, will induce additional melt, which will reduce the net impact of refreezing on runoff (van Pelt et al., 2016b). The spatial distribution of the refrozen fraction (Fig. 9c) reveals minimum values in coastal land areas in the southwest, where melt and rainfall rates are high and winter cooling is limited; maximum values are found at high elevations in central Svalbard (Lomonosovfonna), where melt and rainfall are small and most percolating water is retained due to early melt season refreezing of percolating water and wintertime refreezing of stored irreducible water. The refrozen fraction trends (Fig. 9d) show a Svalbard-wide significant decrease (average decade−1) with most pronounced negative trends in cold (high-altitude) regions in central and northern Svalbard.
Overall, we find that no sites have a long-term mean refrozen fraction close to 1 (Fig. 9c), implying that deep cold firn has been absent throughout the simulation period. This implies that there is no potential for additional refreezing buffering higher melt rates in a warming climate, which is similar to what has been suggested for peripheral glaciers and ice caps of the Greenland ice sheet beyond a “tipping point” in 1997 (Noël et al., 2017). The consistently negative refreezing trend throughout the simulation period in this study suggests that the tipping point would have occurred already prior to the start of the simulation in 1957. Similar long-term negative refreezing trends were previously described by Noël et al. (2018) for ice caps in the southern Canadian Arctic. Future projections of refreezing in Svalbard show that while there will be less refreezing in the early melt season due to reduced winter cooling (reducing the cold content required for refreezing) and shrinking accumulation zones, at the same time wintertime refreezing during and after rainfall and melt events will increase (van Pelt et al., 2016b).
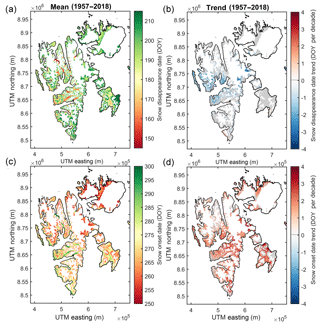
Figure 10Long-term mean spatial snow disappearance date distribution (a) and trends (b). Long-term mean spatial mean snow onset date distribution (c) and trends (d). Snow onset and disappearance dates are only calculated for sites where snow melts completely in summer during at least half of the years in the simulation.
4.4 Seasonal snow cover duration
Land areas and glacier ablation zones in Svalbard experience snow-free conditions during the summer season. The extent of the snow-free season is defined by the snow disappearance date, which we define to occur when snow amount first drops below a threshold (1 cm w.e.), and the snow onset date, which we define as the first date on which snow (>1 cm w.e.) accumulates and remains until next year. Long-term mean distributions of the snow disappearance and onset dates (Fig. 10a, c) show that the earliest snow disappearance (late May) and latest snow arrival (late October) are to be found in the relatively dry valleys of central Svalbard. Trends in the snow disappearance date are primarily controlled by winter accumulation (cumulative snowfall) and melting. We find negligible trends of the snow disappearance date for most of Svalbard, except for parts of central Svalbard, where snow disappears earlier over time (up to 4 d decade−1; Fig. 10b). There is however no significant average snow disappearance trend for all of Svalbard (0.0±0.9 d decade−1), suggesting that, on average, the slight increase in precipitation, generating thicker winter snowpacks, is compensated for by an earlier onset of melting. The snow onset date (September–October) is strongly influenced by air temperature affecting both precipitation type (snow/rain) and potential melt of freshly fallen snow. In response to the substantial autumn warming (Førland et al., 2011; van Pelt et al., 2016a), snow onset trends are significantly positive (up to +4 d decade−1) for most of Svalbard (Fig. 10d), leading to a significant mean positive snow onset date trend of d decade−1. The above discrepancy in trends for snow disappearance and onset dates was previously also found in van Pelt et al. (2016a), where estimates of +1.8 d decade−1 for the snow onset date and +0.7 d decade−1 for the snow disappearance date over the shorter period 1957–2012 were presented.
4.5 Runoff
The long-term mean runoff distribution (Fig. 11a) shows local discharge is apparent across all of Svalbard, with the highest rates (>3 m w.e. a−1) in the glacier ablation zones in southern Svalbard, and the lowest rates <0.3 m w.e. a−1 at the high elevations of the Lomonosovfonna ice cap in central Svalbard. Here, runoff refers to the amount of water originating from melt and rainfall at the surface and available at the base of the snow/firn pack or ice/soil surface after accounting for retention by refreezing and liquid water storage. Melt rates on land are limited to the amount of seasonal snow accumulating during the cold season, and therefore generate much lower runoff rates than nearby glacier sites at similar elevations (Fig. 11a). As a result, the area-averaged runoff from glaciers (0.81 m w.e. a−1) is higher than the runoff from land (0.63 m w.e. a−1), despite the lower mean elevation of the land cells compared to the glacier grid. Trends of runoff over the simulation period (Fig. 11b) are generally not significant for land, but are significantly positive for glaciers, with the largest increases (up to 0.2 m w.e. a−1 decade−1) in ablation zones recently exposed by the retreating ELA. Time series of runoff in gigatonnes per year (Fig. 11c) show average runoff of 10.6 and 34.3 Gt a−1 from land and glacier-covered areas, respectively, contributing to a total average annual runoff of 44.9 Gt a−1. Runoff from land is primarily controlled by precipitation, and as a result the long-term trend is not significant ( Gt a−1 decade−1). Conversely, runoff from glaciers is primarily controlled by summer melt, and is found to increase markedly over the simulation period ( Gt a−1 decade−1), in accordance with decreasing CMB. As a result, total runoff increases by Gt a−1 decade−1, which is equivalent to a 9 % decade−1 increase in runoff. The contrast in trends of runoff from glaciers and land implies a substantial decrease in the relative contribution of land runoff to total runoff from ∼30 % to ∼20 % between 1957 and 2018. Finally, the Svalbard averaged trend in runoff ( m w.e. decade−1) is substantially larger than the trend in the sum of melt and rainfall ( m w.e. decade−1), which is fully explained by a negative trend in refreezing ( m w.e. decade−1). That means that 11 % of the increase in runoff can be explained by reduced refreezing over the simulation period.
4.6 Uncertainties
As described in Sect. 3.2 and in previous studies using the same model in Svalbard (van Pelt et al., 2012; van Pelt and Kohler, 2015; Marchenko et al., 2017b; Pramanik et al., 2018), observational data have been extensively used for calibration, thereby reducing errors in downscaling climate input, solving the energy balance and simulating subsurface conditions. Nevertheless, uncertainty remains, and here we briefly summarize the main remaining sources of errors.
First, we assumed the elevation grid and glacier masks to be fixed throughout the simulation period (Sect. 2.1). As both elevations and masks are based on observational data collected after 1990, this may introduce CMB deviations relative to what would be observed on a time-evolving glacier surface, in particular during the first decades of the simulation. These deviations due to elevation offsets are most pronounced near glacier fronts where thinning rates between 1 and 2 m a−1 have been observed in the 2–4 decades preceding 2003–2007 (Nuth et al., 2010). With a mean balance gradient of 0.002 m w.e. a−1 m−1, this would generate a potential underestimation of CMB of 0.1–0.2 m w.e. a−1 during the first years of the simulation at sites near the glacier snout; at higher elevations errors will be markedly smaller. Typical deviations associated with the use of a fixed glacier mask, compared to a time-dependent glacier mask, have previously been quantified for Svalbard for a similar simulation period at around 0.02–0.04 m w.e. a−1 (Østby et al., 2017). We assume similar values would apply here. It is noteworthy that CMB errors induced by a fixed mask will be of opposite sign as errors induced through the use of a fixed DEM (underestimation of glacier extent in the early decades leads to a too positive CMB, while underestimation of elevations induces a too negative CMB), meaning that some of the above deviations are likely to cancel each other out. The largest deviations will apply to glaciers that surged during the simulation period. Note that the above deviations should not be regarded as errors, it only implies we present and analyse a different quantity (reference mass balance) than what would be observed on a transient glacier surface. Altogether, the use of a fixed mask and elevations has the advantage that all presented trends in climatic mass balance and related products can be attributed to changes in the climate forcing, and we can exclude any influences from dynamically induced geometric changes.
A second source of error comes from uncertainty in the climate input, more specifically the air temperature and precipitation forcings, to which climatic mass balance, seasonal snow development, and derived products are most sensitive. Validation of air temperature against glacier- and land-based measurements (Sect. 3.3) revealed good correlation and generally low biases. In turn, winter balance data were used to optimize the downscaling of precipitation, also returning good correlation and negligible biases (Sect. 3.2). Nevertheless, on average we find a substantially higher snowfall rate (0.89 m w.e. a−1) than previously reported rates of 0.61 m w.e. a−1 by Østby et al. (2017) for 1957–2014 and 0.44 m w.e. a−1 by Lang et al. (2015) for 1979–2013. Østby et al. (2017), however, found that winter accumulation was generally underestimated, primarily at higher elevations, based on a comparison with similar stake winter balance data as used in this study (Fig. 11 in Østby et al., 2017). Furthermore, Lang et al. (2015) only validated their precipitation estimates against meteorological station data in Svalbard, which are known to suffer severely from undercatch (Førland and Hanssen-Bauer, 2000). Nevertheless, we cannot rule out potential biases in our snowfall/precipitation estimates, in particular because all stakes used for calibration are located on glaciers and primarily along their centre lines, which may induce potential biases (e.g. Nuth et al., 2012; Deschamps-Berger et al., 2019). Additionally, the relatively coarse spatial resolution of the regional climate model forcing may cause spatial precipitation fields to miss some of the impacts of terrain on the precipitation distribution, even though this is to some extent compensated for by the precipitation downscaling, which accounts for local elevation. Finally, as also discussed in Sect. 3.2, the inconsistency between the point-wise nature of stake observations and gridded model output representing processes within 1 km2 cells induces uncertainty in the comparison of climatic mass balance components. This is likely to be most pronounced for the bw comparison in wind-affected areas across Svalbard since bw is known to vary over distances much smaller than the 1 km horizontal resolution used here, primarily due to wind-driven snow redistribution (e.g. Winther et al., 2003; Jaedicke and Gauer, 2005; Grabiec et al., 2011; van Pelt et al., 2014).
A third source of error comes from modelling uncertainty, which includes uncertainties related to solving the energy balance, simulating subsurface conditions, and initializing the model. Descriptions of the heat fluxes comprising the surface energy balance have been optimized against observational data in glacier basin studies on Nordenskiöldbreen (van Pelt et al., 2012) and around Kongsfjorden (van Pelt and Kohler, 2015), as also discussed in Sect. 3.2. Energy balance parameters were taken as in the aforementioned studies, with the exception of the fresh snow albedo (αfs), the associated minimum snowfall threshold (Pth), and the background turbulent exchange coefficient (Cb), which were calibrated against observational data (Sect. 3.2). The new albedo scheme assumes that previously used values of t* for Greenland (Bougamont et al., 2005) are also applicable to Svalbard. Potential inaccuracies in parameters like t* will introduce uncertainty in modelled albedo values, as it introduces compensating errors in calibrated parameters; in the case of t*, compensating errors would arise in αfs and Pth. However, the calibration procedure assures that, despite compensating errors, net biases in most relevant model output, e.g. melt, are minimized. More careful calibration of albedo parameters, including t*, is planned for future work using a more extensive dataset of albedo measurements across Svalbard. As automatic weather station (AWS) data from only two regions in central and western Svalbard were used for energy balance model calibration, potential biases may arise for other areas in Svalbard. Regarding uncertainty in simulating subsurface conditions, it is worth noting that the recently implemented deep water percolation scheme (Sect. 3.1, Marchenko et al., 2017b) significantly reduces uncertainty in simulated firn temperatures compared to the earlier bucket scheme, which was found to underestimate rapid deep transport of water through piping. Furthermore, the comparison of simulated and observed bulk firn density shows good agreement (Sect. 3.3), and suggests that model-induced biases are small. We refrain from a detailed vertical comparison of simulated firn density profiles with observed firn core data since previous work has shown the extremely local character of firn stratigraphy in Svalbard (Marchenko et al., 2017a) due to local interactions between stratigraphy and vertical water percolation. As in previous glacier basin-scale applications, we have applied substantial spin-up (25 years) to generate subsurface conditions at the start of the simulation, using the climate forcing during 1957–1982. Obviously, this generates some uncertainty as the 1957–1982 may differ from the actual climate conditions in the decades prior to 1957. As discussed in van Pelt and Kohler (2015), the impacts of perturbing temperature and precipitation during initialization on simulated climatic mass balance are typically only significant in the first few years of the simulation; impacts on simulated firn air content were found to be present even after 20 years into the simulation, which is, however, likely to be less significant in this study given the relatively shallow depth of the vertical domain of <20 m.
We present a model dataset of climatic mass balance, snow conditions, and runoff for all of Svalbard for the period 1957–2018. Output with a 3-hourly temporal and 1 km×1 km spatial resolution is generated with a coupled surface energy balance–snow/firn/soil model. The model is forced with downscaled regional climate model fields and applied to both glacier-covered and land areas. In situ observational data from mass balance stakes, weather stations, and shallow cores are used for model calibration and/or validation of the results. Based on the model output we analyse spatial variability and trends of climatic mass balance, equilibrium line altitude, glacier subsurface conditions, refreezing, seasonal snow season length, and runoff.
We find an area-averaged positive CMB (+0.09 m w.e. a−1) and a significant negative long-term trend (−0.06 m w.e. a−1 decade−1) over the simulation period. The negative CMB trend has caused the ELA to increase (+17 m decade−1) and the AAR to decrease (−0.04 decade−1) markedly. These trends are significant for all of Svalbard, except for the most northern regions. Retreat of the ELA causes a significant reduction of mean firn air content (−0.09 m decade−1), with the most pronounced changes (down to −0.6 m decade−1) in ablation areas that were recently exposed by the retreating ELA. These new ablation zones also experience a strong decrease in temperature at 14 m depth (down to −1.5 ∘C decade−1), while the remainder of the ablation zones show a general warming trend. All high-altitude accumulation zones are found to exhibit temperate deep firn conditions, suggesting the potential for widespread presence of firn aquifers across Svalbard. We find average refreezing rates of 0.24 m w.e. a−1, showing pronounced negative trends for both glacier-covered areas (−0.007 m w.e. a−1 decade−1) and land areas (−0.008 m w.e. a−1 decade−1). Increased precipitation and melt cause the date of disappearance of seasonal snowpacks to remain stable throughout the simulation period, while increased autumn temperatures induce a significant increase in the date of seasonal snow onset (+1.4 d decade−1). The average total runoff for Svalbard (44.9 Gt a−1) is dominated by runoff from glaciers (34.3 Gt a−1) rather than runoff from land (10.6 Gt a−1). A strong positive runoff trend applies to glacier runoff (+3.7 Gt a−1 decade−1), while runoff from land remained nearly stable (+0.2 Gt a−1 decade−1), causing an increase in the relative contribution of glacier discharge to total runoff from 70 % to 80 % over the simulation period.
The digital elevation model can be accessed at https://doi.org/10.21334/npolar.2014.dce53a47 (Norwegian Polar Institute, 2014). The glacier and land masks were constructed from glacier outlines, which are available at https://doi.org/10.21334/npolar.2013.89f430f8 (König et al., 2013). The model outputs behind the presented figures of air temperature, precipitation, CMB, ELA, runoff, refreezing, T14, P14, snow onset, and disappearance dates are available in the following repository: https://doi.org/10.6084/m9.figshare.7836530.v1 (van Pelt et al., 2019). The full model dataset, of which only a selection is presented here, contains data with a 3-hourly temporal resolution and for an extended set of variables; a description of readily available data is accessible online (model output, http://www.wardvanpelt.com/model_output.txt, last access: 13 March 2019). Glacier-wide mass balances for KNG, HBR, HDF, MLB, and ABB are available in the database of the World Glacier Monitoring Service (WGMS; https://wgms.ch/, last access: 6 November 2018). Meteorological time series for Ny-Ålesund and Longyearbyen are accessible through the eKlima portal (Norwegian Meteorological Institute; http://eklima.met.no/, last access: 13 March 2019). The Kongsvegen AWS time series are also accessible at https://doi.org/10.21334/npolar.2017.5dc31930 (Kohler et al., 2017). Unrestricted access to the HIRLAM regional climate model data, point stake mass balance data, and the remaining AWS time series is provided upon request by contacting the institutes that collected/generated the data (see Sect. 2).
The research idea was conceptualized by WvP, who also performed the main analysis. VP, RP, SM, JK, BL, JOH, TD, CR, TVS, and WvP contributed to observational data collection and/or processing. WvP developed the model with support from SM. WvP wrote the paper with contributions from all co-authors.
The authors declare that they have no conflict of interest.
We thank the Norwegian Meteorological Institute for providing access to the HIRLAM regional climate model output. We are further grateful to all the people that assisted with stake mass balance, AWS, and shallow-core measurements on glaciers in Svalbard over the years.
This research has been supported by the Swedish Research Council (VR) (grant no. VR 3903), the Stiftelsen Ymer-80, the Research Council of Norway – Svalbard Integrated Arctic Earth Observing System (SIOS) (grant no. 2018_00010), the Finn Malmgren's stipend (Uppsala Univ.), the Research Council of Norway – TIGRIF project, the Polish-Norwegian Research Programme GLAERE, the TW-ICE (Norwegian Polar Institute), and Ministry of Science and Higher Education of Poland (grant no. 3841/E-41/S/2019).
This paper was edited by Tobias Sauter and reviewed by Marco Möller and one anonymous referee.
Aas, K. S., Dunse, T., Collier, E., Schuler, T. V., Berntsen, T. K., Kohler, J., and Luks, B.: The climatic mass balance of Svalbard glaciers: a 10-year simulation with a coupled atmosphere–glacier mass balance model, The Cryosphere, 10, 1089–1104, https://doi.org/10.5194/tc-10-1089-2016, 2016. a, b, c
AMAP: Snow, water, ice and permafrost in the Arctic (SWIPA), Arctic Monitoring and Assessment Programme (AMAP), Oslo, Norway, 2017. a
Beaudon, E., Moore, J. C., Martma, T., Pohjola, V. A., Van de Wal, R. S., Kohler, J., and Isaksson, E.: Lomonosovfonna and Holtedahlfonna ice cores reveal east–west disparities of the Spitsbergen environment since AD 1700, J. Glaciol., 59, 1069–1083, https://doi.org/10.3189/2013JoG12J203, 2013. a
Bevan, S. L., Luckman, A., Hubbard, B., Kulessa, B., Ashmore, D., Kuipers Munneke, P., O'Leary, M., Booth, A., Sevestre, H., and McGrath, D.: Centuries of intense surface melt on Larsen C Ice Shelf, The Cryosphere, 11, 2743–2753, https://doi.org/10.5194/tc-11-2743-2017, 2017. a
Bezeau, P., Sharp, M., Burgess, D., and Gascon, G.: Firn profile changes in response to extreme 21st-century melting at Devon Ice Cap, Nunavut, Canada, J. Glaciol., 59, 981–991, https://doi.org/10.3189/2013JoG12J208, 2013. a
Bintanja, R. and Andry, O.: Towards a rain-dominated Arctic, Nat. Clim. Change, 7, 263–267, https://doi.org/10.1038/nclimate3240, 2017. a
Bintanja, R. and Selten, F. M.: Future increases in Arctic precipitation linked to local evaporation and sea-ice retreat, Nature, 509, 479–482, https://doi.org/10.1038/nature13259, 2014. a
Bintanja, R. and Van Der Linden, E. C.: The changing seasonal climate in the Arctic, Scientific Reports, 3, 1556, https://doi.org/10.1038/srep01556, 2013. a
Björnsson, H., Gjessing, Y., Hamran, S.-E., Hagen, J. O., LiestøL, O., Pálsson, F., and Erlingsson, B.: The thermal regime of sub-polar glaciers mapped by multi-frequency radio-echo sounding, J. Glaciol., 42, 23–32, https://doi.org/10.3189/S0022143000030495, 1996. a
Blaszczyk, M., Jania, J. A., and Hagen, J. O.: Tidewater glaciers of Svalbard: Recent changes and estimates of calving fluxes, Pol. Polar Res., 30, 85–142, 2009. a, b
Bougamont, M., Bamber, J. L., and Greuell, W.: A surface mass balance model for the Greenland Ice Sheet, J. Geophys. Res., 110, F04018, https://doi.org/10.1029/2005JF000348, 2005. a
Christianson, K., Kohler, J., Alley, R. B., Nuth, C., and van Pelt, W. J. J.: Dynamic perennial firn aquifer on an Arctic glacier, Geophys. Res. Lett., 42, 1418–1426, https://doi.org/10.1002/2014GL062806, 2015. a
Cogley, J. G., Hock, R., Rasmussen, L. A., Arendt, A. A., Bauder, A., Braithwaite, R. J., Jansson, M., Kaser, G., Möller, M., Nicholson, L., and Zemp, M.: Glossary of Glacier Mass Balance and Related Terms, IHP-VII Technical Documents in Hydrology No. 86, IACS Contribution No. 2, UNESCO-IHP, Paris, 2011. a, b
Cox, C., Humphrey, N., and Harper, J.: Quantifying meltwater refreezing along a transect of sites on the Greenland ice sheet, The Cryosphere, 9, 691–701, https://doi.org/10.5194/tc-9-691-2015, 2015. a
Day, J. J., Bamber, J. L., Valdes, P. J., and Kohler, J.: The impact of a seasonally ice free Arctic Ocean on the temperature, precipitation and surface mass balance of Svalbard, The Cryosphere, 6, 35–50, https://doi.org/10.5194/tc-6-35-2012, 2012. a
Deschamps-Berger, C., Nuth, C., Van Pelt, W. J. J., Berthier, E., Kohler, J., and Altena, B.: Closing the mass budget of a tidewater glacier: the example of Kronebreen, Svalbard, J. Glaciol., 65, 136–148, https://doi.org/10.1017/jog.2018.98, 2019. a, b
Divine, D. V. and Dick, C.: Historical variability of sea ice edge position in the Nordic Seas, J. Geophys. Res., 111, C01001, https://doi.org/10.1029/2004JC002851, 2006. a
Elsberg, D. H., Harrison, W. D., Echelmeyer, K. A., and Krimmel, R. M.: Quantifying the effects of climate and surface change on glacier mass balance, J. Glaciol., 47, 649–658, https://doi.org/10.3189/172756501781831783, 2001. a
Førland, E. J. and Hanssen-Bauer, I.: Increased precipitation in the Norwegian Arctic: True or false?, Climatic Change, 46, 485–509, https://doi.org/10.1023/A:1005613304674, 2000. a, b
Førland, E. J., Benestad, R., Hanssen-Bauer, I., Haugen, J. E., and Skaugen, T. E.: Temperature and precipitation development at Svalbard 1900–2100, Adv. Meteorol., 2011, 893790, https://doi.org/10.1155/2011/893790, 2011. a, b
Fürst, J. J., Navarro, F., Gillet-Chaulet, F., Huss, M., Moholdt, G., Fettweis, X., Lang, C., Seehaus, T., Ai, S., Benham, T. J., Benn, D. I., Björnsson, H., Dowdeswell, J. A., Grabiec, M., Kohler, J., Lavrentiev, I., Lindbäck, K., Melvold, K., Pettersson, R., Rippin, D., Saintenoy, A., Sánchez-Gámez, P., Schuler, T. V., Sevestre, H., Vasilenko, E., and Braun, M. H.: The Ice-Free Topography of Svalbard, Geophys. Res. Lett., 45, 11760–11769, https://doi.org/10.1029/2018GL079734, 2018. a
Grabiec, M., Puczko, D., Budzik, T., and Gajek, G.: Snow distribution patterns on Svalbard glaciers derived from radio-echo soundings, Pol. Polar Res., 32, 393–421, https://doi.org/10.2478/v10183-011-0026-4, 2011. a, b
Grabiec, M., Jania, J., Puczko, D., Kolondra, L., and Budzik, T.: Surface and bed morphology of Hansbreen, a tidewater glacier in Spitsbergen, Pol. Polar Res., 33, 111–138, https://doi.org/10.2478/v10183-012-0010-7, 2012. a
Hagen, J., Melvold, K., Eiken, T., Isaksson, E., and Lefauconnier, B.: Mass balance methods on Kongsvegen, Svalbard, Geografiska Annaler, Series A: Physical Geography, 81, 593–601, https://doi.org/10.1111/1468-0459.00087, 1999. a
Hagen, J. O., Kohler, J., Melvold, K., and Winther, J. G.: Glaciers in Svalbard: Mass balance, runoff and freshwater flux, Polar Res., https://doi.org/10.3402/polar.v22i2.6452, 2003. a, b
Hansen, B. B., Aanes, R., Herfindal, I., Kohler, J., Sæther, B. E., and Oli, M. K.: Climate, icing, and wild arctic reindeer: Past relationships and future prospects, Ecology, 92, 1917–1923, https://doi.org/10.1890/11-0095.1, 2011. a
Hansen, B. B., Isaksen, K., Benestad, R. E., Kohler, J., Pedersen, Å., Loe, L. E., Coulson, S. J., Larsen, J. O., and Varpe, Ø.: Warmer and wetter winters: Characteristics and implications of an extreme weather event in the High Arctic, Environ. Res. Lett., 9, 114021, https://doi.org/10.1088/1748-9326/9/11/114021, 2014. a
Hanssen-Bauer, I. and Førland, E. J.: Long-term trends in precipitation and temperature in the Norwegian Arctic: Can they be explained by changes in atmospheric circulation patterns?, Clim. Res., 10, 143–153, https://doi.org/10.3354/cr010143, 1998. a
How, P., Benn, D. I., Hulton, N. R. J., Hubbard, B., Luckman, A., Sevestre, H., van Pelt, W. J. J., Lindbäck, K., Kohler, J., and Boot, W.: Rapidly changing subglacial hydrological pathways at a tidewater glacier revealed through simultaneous observations of water pressure, supraglacial lakes, meltwater plumes and surface velocities, The Cryosphere, 11, 2691–2710, https://doi.org/10.5194/tc-11-2691-2017, 2017. a
Hubbard, B., Luckman, A., Ashmore, D. W., Bevan, S., Kulessa, B., Kuipers Munneke, P., Philippe, M., Jansen, D., Booth, A., Sevestre, H., Tison, J.-L., O'Leary, M., and Rutt, I.: Massive subsurface ice formed by refreezing of ice-shelf melt ponds, Nat. Commun., 7, 11897, https://doi.org/10.1038/ncomms11897, 2016. a
IPCC: Summary for policymakers, in: Climate Change 2013 – The Physical Science Basis, edited by: Intergovernmental Panel on Climate Change, Cambridge University Press, Cambridge, https://doi.org/10.1017/CBO9781107415324.004, 2014. a
Jaedicke, C. and Gauer, P.: The influence of drifting snow on the location of glaciers on western Spitsbergen, Svalbard, Ann. Glaciol., 42, 237–242, https://doi.org/10.3189/172756405781812628, 2005. a
Karner, F., Obleitner, F., Krismer, T., Kohler, J., and Greuell, W.: A decade of energy and mass balance investigations on the glacier Kongsvegen, Svalbard, J. Geophys. Res.-Atmos., 118, 3986–4000, https://doi.org/10.1029/2012JD018342, 2013. a, b
Köhler, A., Maupin, V., Nuth, C., and van Pelt, W.: Characterization of seasonal glacial seismicity from a single-station on-ice record at Holtedahlfonna, Svalbard, Ann. Glaciol., 60, 23–36, https://doi.org/10.1017/aog.2019.15, 2019. a
Kohler, J. and Aanes, R.: Effect of winter snow and ground-icing on a Svalbard reindeer population: results of a simple snowpack model, Arct. Antarct. Alp. Res., 36, 333–341, https://doi.org/10.1657/1523-0430(2004)036[0333:EOWSAG]2.0.CO;2, 2009. a
Kohler, J., James, T. D., Murray, T., Nuth, C., Brandt, O., Barrand, N. E., Aas, H. F., and Luckman, A.: Acceleration in thinning rate on western Svalbard glaciers, Geophys. Res. Lett., 34, L18502, https://doi.org/10.1029/2007GL030681, 2007. a
Kohler, J., Hudson, S., and Obleitner, F.: Automatic weather station data from Kongsvegen, Ny-Ålesund, Data set, Norwegian Polar Institute, https://doi.org/10.21334/npolar.2017.5dc31930, 2017. a
König, M., Kohler, J., and Nuth, C.: Glacier Area Outlines – Svalbard, Data set, Norwegian Polar Institute, https://doi.org/10.21334/npolar.2013.89f430f8, 2013. a
König, M., Nuth, C., Kohler, J., Moholdt, G., and Pettersen, R.: A digital glacier database for Svalbard, in: Global Land Ice Measurements from Space, Springer Praxis Books, Berlin, Heidelberg, https://doi.org/10.1007/978-3-540-79818-7_10, 2014. a, b
Kuipers Munneke, P., Ligtenberg, S. R. M., van den Broeke, M. R., van Angelen, J. H., and Forster, R. R.: Explaining the presence of perennial liquid water bodies in the firn of the Greenland Ice Sheet, Geophys. Res. Lett., 41, 476–483, https://doi.org/10.1002/2013GL058389, 2014. a
Lang, C., Fettweis, X., and Erpicum, M.: Stable climate and surface mass balance in Svalbard over 1979–2013 despite the Arctic warming, The Cryosphere, 9, 83–101, https://doi.org/10.5194/tc-9-83-2015, 2015. a, b, c, d, e, f, g
Ligtenberg, S. R. M., Helsen, M. M., and van den Broeke, M. R.: An improved semi-empirical model for the densification of Antarctic firn, The Cryosphere, 5, 809–819, https://doi.org/10.5194/tc-5-809-2011, 2011. a
Machguth, H., MacFerrin, M., van As, D., Box, J. E., Charalampidis, C., Colgan, W., Fausto, R. S., Meijer, H. A. J., Mosley-Thompson, E., and van de Wal, R. S. W.: Greenland meltwater storage in firn limited by near-surface ice formation, Nat. Clim. Change, 6, 390–393, https://doi.org/10.1038/nclimate2899, 2016. a
Marchenko, S., Pohjola, V. A., Pettersson, R., Van Pelt, W. J. J., Vega, C. P., Machguth, H., Boggild, C. E., and Isaksson, E.: A plot-scale study of firn stratigraphy at Lomonosovfonna, Svalbard, using ice cores, borehole video and GPR surveys in 2012–14, J. Glaciol., 63, 67–78, https://doi.org/10.1017/jog.2016.118, 2017a. a
Marchenko, S., Van Pelt, W. J. J., Claremar, B., Pohjola, V., Pettersson, R., Machguth, H., and Reijmer, C.: Parameterizing deep water percolation improves subsurface temperature simulations by a multilayer firn model, Front. Earth Sci., 5, 16, https://doi.org/10.3389/feart.2017.00016, 2017b. a, b, c, d, e, f
Moholdt, G., Nuth, C., Hagen, J. O., and Kohler, J.: Recent elevation changes of Svalbard glaciers derived from ICESat laser altimetry, Remote Sens. Environ., 114, 2756–2767, https://doi.org/10.1016/j.rse.2010.06.008, 2010. a, b
Möller, M. and Kohler, J.: Differing climatic mass balance evolution across Svalbard glacier regions over 1900–2010, Front. Earth Sci., 6, 128, https://doi.org/10.3389/feart.2018.00128, 2018. a, b
Möller, M., Finkelnburg, R., Braun, M., Scherer, D., and Schneider, C.: Variability of the climatic mass balance of Vestfonna ice cap, northeastern Svalbard, 1979–2011, Ann. Glaciol., 54, 254–264, https://doi.org/10.3189/2013AoG63A407, 2013. a
Möller, M., Obleitner, F., Reijmer, C. H., Pohjola, V. A., Głowacki, P., and Kohler, J.: Adjustment of regional climate model output for modeling the climatic mass balance of all glaciers on Svalbard, J. Geophys. Res.-Atmos., 121, 5411–5429, https://doi.org/10.1002/2015JD024380, 2016. a, b
Noël, B., Van de Berg, W. J., Lhermitte, S., Wouters, B., Machguth, H., Howat, I., Citterio, M., Moholdt, G., Lenaerts, J. T. M., and van den Broeke, M. R.: A tipping point in refreezing accelerates mass loss of Greenland's glaciers and ice caps, Nat. Commun., 8, 14730, https://doi.org/10.1038/ncomms14730, 2017. a
Noël, B., Van de Berg, W. J., Lhermitte, S., Wouters, B., Schaffer, N., and Van den Broeke, M. R.: Six Decades of Glacial Mass Loss in the Canadian Arctic Archipelago, J. Geophys. Res.-Earth, 123, 1430–1449, https://doi.org/10.1029/2017JF004304, 2018. a
Nordli, Ø., Przybylak, R., Ogilvie, A. E., and Isaksen, K.: Long-term temperature trends and variability on Spitsbergen: The extended Svalbard airport temperature series, 1898–2012, Polar Res., 33, 21349, https://doi.org/10.3402/polar.v33.21349, 2014. a, b
Norwegian Polar Institute: Terrengmodell Svalbard (S0 Terrengmodell), Data set, Norwegian Polar Institute, https://doi.org/10.21334/npolar.2014.dce53a47, 2014. a
Nuth, C., Moholdt, G., Kohler, J., Hagen, J. O., and Kääb, A.: Svalbard glacier elevation changes and contribution to sea level rise, J. Geophys. Res., 115, F01008, https://doi.org/10.1029/2008JF001223, 2010. a
Nuth, C., Schuler, T. V., Kohler, J., Altena, B., and Hagen, J. O.: Estimating the long-term calving flux of Kronebreen, Svalbard from geodetic elevation changes and mass-balance modelling, J. Glaciol., 58, 119–133, https://doi.org/10.3189/2012JoG11J036, 2012. a, b
Nuth, C., Kohler, J., König, M., von Deschwanden, A., Hagen, J. O., Kääb, A., Moholdt, G., and Pettersson, R.: Decadal changes from a multi-temporal glacier inventory of Svalbard, The Cryosphere, 7, 1603–1621, https://doi.org/10.5194/tc-7-1603-2013, 2013. a
Oerlemans, J. and Grisogono, B.: Glacier winds and parameterisation of the related surface heat fluxes, Tellus A, 54, 440–452, https://doi.org/10.3402/tellusa.v54i5.12164, 2002. a
Oerlemans, J. and Knap, W. H.: A 1 year record of global radiation and albedo in the ablation zone of Morteratschgletscher, Switzerland, J. Glaciol., 44, 231–238, https://doi.org/10.3189/S0022143000002574, 1998. a
Østby, T. I., Schuler, T. V., Hagen, J. O., Hock, R., Kohler, J., and Reijmer, C. H.: Diagnosing the decline in climatic mass balance of glaciers in Svalbard over 1957–2014, The Cryosphere, 11, 191—215, https://doi.org/10.5194/tc-11-191-2017, 2017. a, b, c, d, e, f, g, h, i, j, k, l
Pettersson, R.: Dynamics of the cold surface layer of polythermal Storglaciären, Sweden, PhD thesis, Stockholm University, Stockholm, Sweden, 2004. a
Pohjola, V. A., Martma, T. A., Meijer, H. A. J., Moore, J. C., Isaksson, E., Vaikmäe, R., and van de Wal, R. S.: Reconstruction of three centuries of annual accumulation rates based on the record of stable isotopes of water from Lomonosovfonna, Svalbard, Ann. Glaciol., 35, 57–62, https://doi.org/10.3189/172756402781816753, 2002. a
Pramanik, A., Van Pelt, W. J. J., Kohler, J., and Schuler, T. V.: Simulating climatic mass balance, seasonal snow development and associated freshwater runoff in the Kongsfjord basin, Svalbard (1980–2016), J. Glaciol., 64, 943–956, https://doi.org/10.1017/jog.2018.80, 2018. a, b, c, d
Reistad, M., Breivik, Ø., Haakenstad, H., Aarnes, O. J., Furevik, B. R., and Bidlot, J. R.: A high-resolution hindcast of wind and waves for the North Sea, the Norwegian Sea, and the Barents Sea, J. Geophys. Res., 116, C05019, https://doi.org/10.1029/2010JC006402, 2011. a, b
Rye, C. J., Willis, I. C., Arnold, N. S., and Kohler, J.: On the need for automated multiobjective optimization and uncertainty estimation of glacier mass balance models, J. Geophys. Res., 117, F02005, https://doi.org/10.1029/2011JF002184, 2012. a
Schuler, T. V., Dunse, T., Østby, T. I., and Hagen, J. O.: Meteorological conditions on an Arctic ice cap-8 years of automatic weather station data from Austfonna, Svalbard, Int. J. Climatol., 34, 2047–2058, https://doi.org/10.1002/joc.3821, 2014. a
Serreze, M. C. and Barry, R. G.: Processes and impacts of Arctic amplification: A research synthesis, Global Planet. Change, 77, 85–96, https://doi.org/10.1016/j.gloplacha.2011.03.004, 2011. a
Uppala, S. M., Kållberg, P. W., Simmons, A. J., Andrae, U., Bechtold, V. D. C., Fiorino, M., Gibson, J. K., Haseler, J., Hernandez, A., Kelly, G. A., Li, X., Onogi, K., Saarinen, S., Sokka, N., Allan, R. P., Andersson, E., Arpe, K., Balmaseda, M. A., Beljaars, A. C. M., Berg, L. V. D., Bidlot, J., Bormann, N., Caires, S., Chevallier, F., Dethof, A., Dragosavac, M., Fisher, M., Fuentes, M., Hagemann, S., Hólm, E., Hoskins, B. J., Isaksen, L., Janssen, P. A. E. M., Jenne, R., Mcnally, A. P., Mahfouf, J.-F., Morcrette, J.-J., Rayner, N. A., Saunders, R. W., Simon, P., Sterl, A., Trenberth, K. E., Untch, A., Vasiljevic, D., Viterbo, P., and Woollen, J.: The ERA-40 re-analysis, Q. J. Roy. Meteor. Soc., 131, 2961–3012, https://doi.org/10.1256/qj.04.176, 2005. a
Vallot, D., Pettersson, R., Luckman, A., Benn, D. I., Zwinger, T., Van Pelt, W. J. J., Kohler, J., Schäfer, M., Claremar, B., and Hulton, N. R.: Basal dynamics of Kronebreen, a fast-flowing tidewater glacier in Svalbard: non-local spatio-temporal response to water input, J. Glaciol., 63, 1012–1024, https://doi.org/10.1017/jog.2017.69, 2017. a
Vallot, D., Åström, J., Zwinger, T., Pettersson, R., Everett, A., Benn, D. I., Luckman, A., van Pelt, W. J. J., Nick, F., and Kohler, J.: Effects of undercutting and sliding on calving: a global approach applied to Kronebreen, Svalbard, The Cryosphere, 12, 609–625, https://doi.org/10.5194/tc-12-609-2018, 2018. a
Van de Wal, R. S., Mulvaney, R., Isaksson, E., Moore, J. C., Pinglot, J. F., Pohjola, V. A., and Thomassen, M. P. A.: Reconstruction of the historical temperature trend from measurements in a medium-length borehole on the Lomonosovfonna plateau, Svalbard, Ann. Glaciol., 35, 371–378, https://doi.org/10.3189/172756402781816979, 2002. a
Van der Wel, L., Streurman, H., Isaksson, E., Helsen, M., Van de Wal, R., Martma, T., Pohjola, V., Moore, J., and Meijer, H.: Using high-resolution tritium profiles to quantify the effects of melt on two Spitsbergen ice cores, J. Glaciol., 57, 1087–1097, https://doi.org/10.3189/002214311798843368, 2011. a
van Pelt, W. J. J. and Kohler, J.: Modelling the long-term mass balance and firn evolution of glaciers around Kongsfjorden, Svalbard, J. Glaciol., 61, 731–744, https://doi.org/10.3189/2015JoG14J223, 2015. a, b, c, d, e, f, g, h, i, j, k, l
van Pelt, W. J. J., Oerlemans, J., Reijmer, C. H., Pohjola, V. A., Pettersson, R., and van Angelen, J. H.: Simulating melt, runoff and refreezing on Nordenskiöldbreen, Svalbard, using a coupled snow and energy balance model, The Cryosphere, 6, 641–659, https://doi.org/10.5194/tc-6-641-2012, 2012. a, b, c, d, e, f, g, h, i, j, k
van Pelt, W. J. J., Pettersson, R., Pohjola, V. A., Marchenko, S., Claremar, B., and Oerlemans, J.: Inverse estimation of snow accumulation along a radar transect on Nordenskiöldbreen, Svalbard, J. Geophys. Res.-Earth, 119, 816–835, https://doi.org/10.1002/2013JF003040, 2014. a, b, c, d, e
van Pelt, W. J. J., Kohler, J., Liston, G. E., Hagen, J. O., Luks, B., Reijmer, C. H., and Pohjola, V. A.: Multidecadal climate and seasonal snow conditions in Svalbard, J. Geophys. Res.-Earth, 121, 2100–2117, https://doi.org/10.1002/2016JF003999, 2016a. a, b, c, d, e, f, g, h
van Pelt, W. J. J., Pohjola, V. A., and Reijmer, C. H.: The changing impact of snow conditions and refreezing on the mass balance of an idealized Svalbard glacier, Front. Earth Sci., 4, 102, https://doi.org/10.3389/feart.2016.00102, 2016b. a, b, c, d, e, f
van Pelt, W. J. J., Pohjola, V. A., Pettersson, R., Ehwald, L. E., Reijmer, C. H., Boot, W., and Jakobs, C. L.: Dynamic response of a High Arctic glacier to melt and runoff variations, Geophys. Res. Lett., 45, 4917–4926, https://doi.org/10.1029/2018GL077252, 2018. a, b, c
van Pelt, W. J. J., Pohjola, V. A., Pettersson, R., Marchenko, S., Kohler, J., Luks, B., Hagen, J. O., Schuler, T. V., Dunse, T., Noël, B., and Reijmer, C. H.: A long-term dataset of climatic mass balance, snow conditions and runoff in Svalbard (1957–2018), https://doi.org/10.6084/m9.figshare.7836530.v1, 2019. a
Vega, C. P., Pohjola, V. A., Beaudon, E., Claremar, B., van Pelt, W. J. J., Pettersson, R., Isaksson, E., Martma, T., Schwikowski, M., and Bøggild, C. E.: A synthetic ice core approach to estimate ion relocation in an ice field site experiencing periodical melt: a case study on Lomonosovfonna, Svalbard, The Cryosphere, 10, 961–976, https://doi.org/10.5194/tc-10-961-2016, 2016. a
Westermann, S., Boike, J., Langer, M., Schuler, T. V., and Etzelmüller, B.: Modeling the impact of wintertime rain events on the thermal regime of permafrost, The Cryosphere, 5, 945–959, https://doi.org/10.5194/tc-5-945-2011, 2011. a
Winsvold, S. H., Kääb , A., Nuth, C., Andreassen, L. M., van Pelt, W. J. J., and Schellenberger, T.: Using SAR satellite data time series for regional glacier mapping, The Cryosphere, 12, 867–890, https://doi.org/10.5194/tc-12-867-2018, 2018. a
Winther, J.-G., Bruland, O., Sand, K., Gerland, S., Marechal, D., Ivanov, B., Głowacki, P., and König, M.: Snow research in Svalbard – An overview, Polar Res., 22, 125–144, https://doi.org/10.3402/polar.v22i2.6451, 2003. a, b
Zhang, X., He, J., Zhang, J., Polyakov, I., Gerdes, R., Inoue, J., and Wu, P.: Enhanced poleward moisture transport and amplified northern high-latitude wetting trend, Nat. Clim. Change, 3, 47–51, https://doi.org/10.1038/nclimate1631, 2013. a, b