the Creative Commons Attribution 4.0 License.
the Creative Commons Attribution 4.0 License.
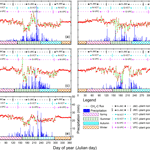
Consumption of atmospheric methane by the Qinghai–Tibet Plateau alpine steppe ecosystem
Hanbo Yun
Qingbai Wu
Tong Yu
Zhou Lyu
Yuzhong Yang
Huijun Jin
Guojun Liu
Yang Qu
Licheng Liu
The methane (CH4) cycle on the Qinghai–Tibet Plateau (QTP), the world's largest high-elevation permafrost region, is sensitive to climate change and subsequent freezing and thawing dynamics. Yet, its magnitudes, patterns, and environmental controls are still poorly understood. Here, we report results from five continuous year-round CH4 observations from a typical alpine steppe ecosystem in the QTP permafrost region. Our results suggest that the QTP permafrost region was a CH4 sink of g CH4-C m−2 yr−1 over 2012–2016, a rate higher than that of many other permafrost areas, such as the Arctic tundra in northern Greenland, Alaska, and western Siberia. Soil temperature and soil water content were dominant factors controlling CH4 fluxes; however, their correlations changed with soil depths due to freezing and thawing dynamics. This region was a net CH4 sink in autumn, but a net source in spring, despite both seasons experiencing similar top soil thawing and freezing dynamics. The opposite CH4 source–sink function in spring versus in autumn was likely caused by the respective seasons' specialized freezing and thawing processes, which modified the vertical distribution of soil layers that are highly mixed in autumn, but not in spring. Furthermore, the traditional definition of four seasons failed to capture the pattern of the annual CH4 cycle. We developed a new seasonal division method based on soil temperature, bacterial activity, and permafrost active layer thickness, which significantly improved the modeling of the annual CH4 cycle. Collectively, our findings highlight the critical role of fine-scale climate freezing and thawing dynamics in driving permafrost CH4 dynamics, which needs to be better monitored and modeled in Earth system models.
- Article
(4359 KB) - Full-text XML
-
Supplement
(2318 KB) - BibTeX
- EndNote
Since 2007, the global atmospheric methane concentration [CH4] continues to rise, after remaining stable between the 1990s and 2006 (Rigby et al., 2008; IPCC, 2013; Patra and Kort, 2016). Understanding mechanisms for this recent increase requires improved knowledge of CH4 sources and sinks for regional and global CH4 budgets (Kirschke et al., 2013; Zona et al., 2016). However, estimates on global CH4 emissions and consumptions are still highly uncertain (Spahni et al., 2011; Kirschke et al., 2013). In particular, the bottom-up approach, which estimates CH4 budgets using ground observations and inventory, overestimated the global CH4 budget by 6–20 times, compared to the atmospherically constrained top-down approach (Zhu et al., 2004; Lau et al., 2015). This discrepancy is partly due to limited monitoring data and to our poor understanding of important factors regulating the production and consumption of CH4 (Whalen and Reeburgh, 1990; Dengel et al., 2013; Bohn et al., 2015).
The Qinghai–Tibet Plateau (QTP) is the world's largest high-elevation permafrost region of 1.23×106 km2 (Wang et al., 2000). The QTP is currently experiencing a rapid change in climate, which affects freezing and thawing processes. The change in the freezing and thawing dynamics profoundly impacts methanotrophy and methanogenesis, which consequently impacts net CH4 fluxes (Mastepanov et al., 2013; Lau et al., 2015). However, due to the scarcity of year-round monitoring data at high temporal resolution, we still know little about the size, seasonal pattern, and underlying controls of climate and permafrost freezing and thawing and the resulting effects on CH4 exchanges in the QTP permafrost region (Cao et al., 2008; Wei et al., 2015a; Song et al., 2015). This knowledge gap also hampers our capacity to predict and understand QTP permafrost CH4 cycles under current and projected future climates.
Here, we report results from a 5-year continuous in situ monitoring of CH4 dynamics with an eddy covariance (EC) technique at the Beilu'he research station, which is a representative site for QTP permafrost heartland. The site was covered by alpine steppe vegetation from 1 January 2012 to 31 December 2016. The primary aims of this investigation are to understand (1) the long-term annual and seasonal variation in the methane budget for a typical alpine permafrost site in the QTP and (2) the environmental factors controlling these CH4 variations and possible underlying mechanisms. In addition, while the consumption and production of ecosystem methane are known through microbial activities, conventional investigations on seasonal methane fluxes usually used climate- or vegetation-defined “seasons”. Therefore, a third research goal of this current study is to investigate if the classical vegetation productivity-based definition of growing season will be useful for defining the methane flux seasonality.
There are three advantages of our data acquisition system. First, the EC system recorded the data of CH4 fluxes, climate, and soil properties every half hour. As the QTP permafrost is characterized by a rapidly changing climate and rapidly changing soil freezing and thawing dynamics, even over a time period as short as 1 day, different aerobic or anaerobic soil environments that favor different types of CH4 bacteria may change (Rivkina et al., 2004; Lau et al., 2015). Thus, high-resolution in situ monitoring data enable us to quantify CH4 exchange patterns from diel to annual timescales and investigate their major environmental drivers. Second, our field investigation spanned 5 full calendar years, including both plant growing and nongrowing seasons. Observations of the plant nongrowing season, which accounts for two-thirds of a year, are very rare in current literature (Song et al., 2015). Third, the EC system we used overcame some technical problems caused by the often used static chambers, including limited representation of local site heterogeneity and additional heating of the soil surface (Chang et al., 2014; Wei et al., 2015b).
2.1 Site description
The research site, Beilu'he permafrost research station (34∘09′006 N, 92∘02′080 E), is located in the alpine steppe continuous permafrost area of the northern QTP, about 320 km southwest of Golmud, Qinghai Province (Fig. 1). At an elevation of 4765 m, the air is thin with only 0.6 standard atmospheric pressure. According to in situ observations, the site receives solar radiation of about 213.10 W m−2. The nongrowing season is long and cold, with 225 days per year having an annual air temperature of −18∘ on average from 2012 to 2016. The site's growing season is short and cool, with 140 days per year from 2012 to 2016 and a mean annual air temperature of 4.6 ∘C. According to the site drilling exploration, the permafrost depth can extend to 50–70 m below ground, and the thickness of the active layer (ALT) is about 2.2–4.8 m (Wu et al., 2010a). The soil is composed of Quaternary fine sand or silt (Table 1), overlying Triassic mudstone or weathered marl. Dominant plant species include Carex moorcroftii Falc. ex Boott, Kobresia tibetica Maxim, Androsace tanggulashanensis, and Rhodiola tibetica. Vegetation coverage is approximately 33.5 % and the average plant height is 15 cm.
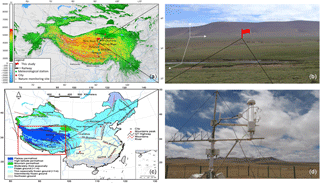
Figure 1Geographic location of the study site. (a) Map of China's permafrost distribution, and the red box marks the approximate location of the Qinghai–Tibet Plateau. (b) The study site location and meteorological stations along the Qinghai–Tibet railway. (c) Photo showing the study site's topography and physiognomy. The small red flag in (c) is the eddy covariance tower location. (d) Close-up shot of the LI-7700 for methane measurement. Map boundary and location are approximate. Geographic features and the names do not imply any official endorsement or recognition.
2.2 Eddy covariance observations
We have continuously monitored CH4, carbon dioxide (CO2), water (H2O), and heat flux using a standard EC system tower 3 m above the ground. CH4 flux was measured with an open-path CH4 analyzer system (Fig. 1d; LI-7700, Li-cor Inc., Lincoln, NE, USA). The precision is 5 ppb, with RMS noise at 10 Hz and 2000 ppb. The instrument was placed on site on 8 August 2011 and then connected to a three-dimensional sonic anemometer (heat and water flux; CSAT3, Campbell Scientific, and Logan, UT, USA; the precision is 0.1 ∘C with an accuracy within 1 % of the reading for half-hour measurements) and an open-path infrared gas analyzer (CO2 flux; LI-7500A, Li-cor Inc., Lincoln, NE, USA; the precision is 0.01 with an accuracy within 1 % of the reading for half-hour measurements; zero drift per degree Celsius is typically ±0.1 ppm) on 1 January 2012, when the system worked steadily. Monitoring data were recorded and stored at 10 Hz using a data logger (LI-7550, Li-cor Inc., Lincoln, NE, USA).
The operation, calibrations, and maintenance of the EC system followed standard procedures. To reduce the LI-7500A surface heating–cooling influence on CO2 and H2O molar densities in tough environments, each year “summer style”, in which the surface temperature setting was 5 ∘C, was used in Li-7500A from 1 May to 30 September. “Winter style”, in which the surface temperature setting was −5 ∘C, was used from 1 October to 30 April the next year in Li-7500A. Calibrations of CO2, water vapor, and dew point generator measurements for LI-7500A analyzers were performed regularly by the China Land–Atmosphere Coordinated Observation System (CLAROS). Up-and-down mirrors of LI-COR 7700 were cleaned regularly every 30 days to make sure the signal strength was stronger than 80. All of these instruments were powered by solar panel and battery.
2.3 Micrometeorological and soil measurements
A wide range of meteorological variables were measured by a standard automatic meteorological tower 3 m above the ground and 5 m north of the EC tower. Net radiation (Rn) and albedo were measured with a four-component radiometer (Rn; CNR-1, Kipp and Zonen, the Netherlands). Air temperature (Tair), air relative humidity, and atmospheric pressure were measured with a temperature and humidity sensor (HMP45C, Vaisala Inc., Helsinki, Finland) in the meteorological tower. A rain gauge (TE525MM, Texas Electronics Inc., Dallas, TX, USA) was used to measure precipitation. Wind speed and direction were observed using a propeller anemometer placed on the top of the meteorological tower.
We also measured soil heat fluxes, soil temperature, and soil relative water content (SWC). In August 2010, we installed sensors for soil environment and surface energy exchange monitoring 10 m apart from the EC tower. Two self-calibrating soil heat flux (SHF) sensors (HFP01) were placed 5 and 15 cm below the ground. A group pF meter sensor (Geoprecision, Germany) was embedded in the soil under the meteorological tower to measure soil temperature (Tsoil) at 0, 5, 10, 15, 20, 30, 40, 50, 70, 80, 100, 150, 160, and 200 cm of depth. The pF meter sensors also measured SWC at 10, 20, 40, 80, and 160 cm of depth.
All of the above environmental parameters were synchronously monitored with EC, and the data were recorded every 30 min by CR3000 (data logger, Campbell Scientific Ltd., Salt Lake City, UT, USA). The air temperature sensors, the humidity sensors, and the pF meter sensors were calibrated in the State Key Laboratory of Frozen Soil Engineering at the Chinese Academy of Sciences in order to ensure the measurement accuracy was within ±0.05 ∘C and ±5 %.
We also sampled soil profiles for soil physical and chemical measurements with one pit 10 m from the EC tower in August 2010. Five profile samples were taken from the pit at depths of 0–20, 20–50, 50–120, 120–160, and 160–200 cm. Sampling at each depth was repeated five times and the samples of the same depths were then well mixed. After that, the mixed soil sample of each depth was stored in aluminum boxes and carefully sealed to prevent gas exchanges with air. The clod method was used to investigate the field wet bulk density (weight of soil per unit volume; Cate and Nelson, 1971). The soil moisture content was calculated gravimetrically with the ratio of the mass of water present to the oven-dried (60 ∘C for 24 h) weight of the soil sample. The soil organic carbon (SOC) content of the air-dried soil samples was analyzed using the wet combustion method, Walkley–Black modified acid dichromate digestion, FeSO4 titration, and an automatic titrator. Total nitrogen (TN) and pH were measured using standard soil test procedures from the Chinese Ecosystem Research Network.
To understand the potential effect of soil thawing and freezing dynamics on CH4 fluxes, we also reconstructed and verified semi-monthly data of soil ALT. Following Muller's original definition, ALT is the maximum thaw depth in the late autumn using a linear interpolation of Tsoil profiles between two neighboring points above and below the 0 ∘C isotherm (Muller, 1947). We used records of the soil thawing thickness measured with a self-made geological probe to verify the ALT data semi-monthly. More information about the measurement procedure was previously described by Wu and Zhang (2010a).
2.4 Microbial activity
To understand how soil microbial activity may have impacted the CH4 fluxes, we sampled 100 g of soils for soil microbial activity measurements. These soils were obtained using a soil sample drill device (∅=0.03 m), with depths of 0–25 cm taken every 5 days within 100 m of the EC tower. The sampled soil was fully mixed and divided into two equal parts. Each part was then stored in sterilized aluminum boxes and then placed in liquid nitrogen before sending to the lab for microbe RNA extraction. We then used a real-time polymerase chain reaction (PCR) method to genetically test methanotrophic–archaeal methanogens, and the procedure was repeated three times for each sample. By setting the maximum methanotrophic–archaeal methanogen gene expression cyclic number as 1, we calculated the variety coefficient of methanotrophic and archaeal methanogen gene expressions (ΔI and ΔII, respectively; %) with Eq. (1):
Δi is for the ith methanotrophic–archaeal methanogen gene expression; xi is the methanotrophic–archaeal methanogen gene expression cyclic number of the ith time; xMax is the maximum methanotrophic–archaeal methanogen gene expression cyclic number of the soil group from 2012 to 2016.
2.5 EC data processing and data filtering
Data collected from 1 January 2012 to 31 December 2016 were used in this study. Before processing, we removed data that were recorded at the time of precipitation events or with a LI-7700 signal strength under 85. We first processed the raw data in EddyPro (version 6.2.0, Li-cor, Lincoln, NE, USA). We adopted standardized procedures recommended in Lee et al. (2006) to process half-hourly flux raw measurements to ensure their quality.
- 1.
Data were processed through statistical analysis in EddyPro including spike removal (accepted spikes <5 % and replaced spikes with linear interpolation), amplitude resolution (range of variation: 7.0σ; number of bins: 100; accepted empty bins: 70 %), dropouts (percentile defining extreme bins: 10; accepted central dropouts: 10 %; accepted extreme dropouts: 6 %), absolute limits (, , ; , , ), skewness and kurtosis (, ; , ), discontinuities (hard-flag threshold: U=4.0, W=2.0, Tsoil=4.0, CO2 =40, CH4 =40, and H2O =3.26; soft-flag threshold: U=2.7, W=1.3, Tsoil=2.7, CO2 =27, CH4 =30, and H2O =2.2), angle of attack (minimum angle of attack ; maximum angle attack =30; accepted number of outliers =10 %), and steadiness of horizontal wind (accepted wind relative instationarity =0.5) (Vickers and Mahrt, 1997; Mauder et al., 2013).
- 2.
The data were then corrected using atmosphere physical calculations expressed by axis rotations of tilt correction (double rotation), time lags compensation (covariance maximization), and compensating density fluctuations of Webb–Pearman–Leuning (Webb et al., 1980). When CO2 and H2O molar densities are measured with the Li-cor 7500/Li-cor 7500A in cold environments (low temperatures below −10 ∘C), a correction should be applied to account for the additional instrument-related sensible heat flux due to instrument surface heating–cooling. Thus, we implemented the correction according to Burba et al. (2008), which involves calculating a corrected sensible heat flux (H′) by adding estimated sensible heat fluxes from key instrument surface elements, including the bottom window (Hbot), top window (Htop), and spar (Hspar), to the ambient sensible heat flux (H):
- 3.
Quality assurance (QA) and quality control (QC) were ensured through spectral analysis and correction analysis in EddyPro. Spectra and co-spectra calculations used power-of-two samples to speed up the fast Fourier transform (FFT) algorithm. Here we checked the “Filter (co-)spectra according to Vickers and Mahrt (1997) test results” box in EddyPro, which would then disregard EC flux time series that would likely create artifacts in spectral and co-spectral shapes. We also used the Foken and Wichura (1996) and Mauder et al. (2013) micrometeorological quality tests embedded in EddyPro to filter low-quality EC time series data. Low-frequency-range spectral correction was performed considering high-pass filtering effects. High-frequency-range spectral correction was carried out considering low-pass filtering effects (Moncrieff et al., 2004).
- 4.
We chose values of “0”, “1”, and “2” to flag the processed flux data into three quality classes in EddyPro. The combined flag attains the value 0 for the best-quality fluxes, 1 for fluxes suitable for general analysis, such as annual budgets, and 2 for fluxes that should be discarded from the dataset of results. For our dataset, approximately 67 % of the data fell into Class 0, 12 % in Class 1, and 21 % in Class 2.
- 5.
Our analysis indicated that, under average meteorological conditions, 80 % of the flux (footprint) came from an area within 175 m of the EC tower.
In addition, we also adopted the method in Burba et al. (2008) to adjust the half-hour flux data to avoid apparent measurement errors. In doing this, we rejected half-hour flux data that fell into one of the following situations: (1) incomplete half-hour measurements, (2) measurements under rain impacts, (3) nighttime measurements under stable atmospheric conditions (friction velocity m s−1), and (4) abnormal values detected by a three-dimensional ultrasonic anemometer. This screening resulted in the rejection of about 20.7 % of all the flux data.
After the above data QC, there was a 28.7 % data gap for CH4 fluxes over the entire period. These data gaps were then filled according to the method described in the literature (Falge et al., 2001; Papale et al., 2006). We used a linear interpolation to fill the gaps if they were less than 2 h, a method described in Falge et al. (2001) to fill gaps greater than 2 h but less than 1 day, and an artificial neural network approach as described in Papale et al. (2006) and Dengel et al. (2013) to fill gaps greater than 1 day.
The quality of the dataset was evaluated using the equation of energy closure:
where the EBR is surface energy balance ratio, H is heat flux, λE is latent heat, Rn is net radiation, G is SHF, and S is heat storage of the vegetation canopy. As vegetation coverage at this research site is sparse, S is ignored. From 2012 to 2016, the average EBR value at the Beilu'he EC site was about 0.675, falling within the range of 0.34 to 1.69 in an analysis of energy balance closure for global FLUXNET sites (Wilson et al., 2002).
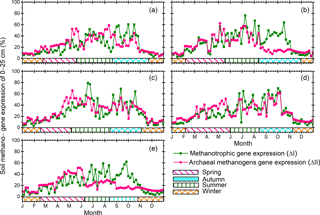
Figure 2Annual patterns of soil methanogen gene expression of 0–25 cm soil depth for the years (a) 2012, (b) 2013, (c) 2014, (d) 2015, and (e) 2016.
We analyzed two different major sources of CH4 flux gap-filling uncertainty. The first kind of uncertainty came from the U* threshold estimate. Following Burba et al. (2008), we excluded the probably false low CH4 flux at low U*. However, it was difficult to determine the value for the U* threshold. For instance, when choosing a lower U* threshold, the associated lower flux would contribute to the gap filling and the annual gross (Loescher, et al., 2006). Here we used the variance from 5 % to 95 % of the bootstrapped values to provide an estimate on the uncertainties caused by different U* thresholds. The second uncertainty source was due to insufficient power supply. In this research, all instrument power was supplied by solar panels. Extended periods of rainy, cloudy, and snowy weather would cause the instrument to stop working due to an insufficient power supply. When we used the gap-filling method mentioned above, it would cause the CH4 flux to deviate from the true value. To our knowledge, the CH4 flux data were largely uncertain under rainy conditions.
2.6 New classification system of the four seasons based on microbial activity classification
We redefined the four seasons of spring, summer, autumn, and winter based on the microbial activity parameters of the new seasons (Fig. 2), ALT variability coefficients (ALT variability coefficient = (ALT ALTi)/ALTMax, where ALTMax is the maximum of ALT per year), and Tsoil. Below, we describe the start date of each season (the end date of a season is the day immediately before the start of the next season).
Spring starts at the first day of two consecutive observation periods fulfilling both (1) (ΔII +ΔI)/2≥15 % and (2) the ALT variability coefficient ≥0.05.
Summer starts on the first day of two consecutive observation periods when (1) (ΔII +ΔI)/2≥45 %, (2) the ALT variability coefficient is ≥0.35, and (3) 5 successive days have a Tsoil at 40 cm of soil depth ≥0 ∘C.
Autumn starts on the first day of two consecutive observation periods when (1) (ΔII +ΔI)/2≥55 %, (2) the ALT variability coefficient is ≥0.60, and (3) 5 successive days have a Tsoil of 10 cm<5 ∘C.
Winter starts on the first day of two consecutive observation periods that have (1) (ΔII +ΔI)/2<15 % and (2) the ALT variability coefficient <0.05.
To test the robustness of our new seasonal division method in our methane cycle analysis, we compared empirical CH4 flux estimates using different season definitions (Table 2). In addition to our new method that was based on top soil microbe activity, Tsoil of 0–40 cm, and permafrost active layer variability (hereafter referred to as SMT), we also used three conventional methods, based on (i) vegetation cover and temperature change (VCT), (ii) Julian months (JMC), and (iii) vegetation phenology change (VPC). The VCT method splits a year into a plant growing season and a nongrowing season; the JMC method assumes May to October as a plant growing season, and November to the following April as a nongrowing season; and the VPC method defines a plant growing season as the period between the time when all dominant grass species (Carex moorcroftii Falc. ex Boott, Kobresia tibetica Maxim, Androsace tanggulashanensis, Rhodiola tibetica) germinate and when they all senesce.
Table 2Measurements of four seasons from 2012 to 2016.
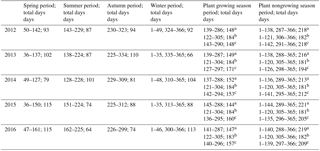
a Based on vegetation cover and temperature change (VCT) (Lund et al., 2010; Tang et al., 2013; Song et al., 2015). b Based on Julian months (JMC) (Wei et al., 2015a). c Based on vegetation phenology change (VPC). Spring, summer, autumn, and winter are based on parameters of microbial activities, ALT variety coefficient, and Tsoil (SMT).
2.7 Statistical analyses
To understand the connections between CH4 fluxes and associated environmental factors, we performed a series of statistical analyses, including correlation, principal component analysis (PCA), and linear regression analyses, in IBM SPSS (IBM SPSS Statistics 24; IBM, Armonk, NY, USA). Specifically, we used bivariate correlation to examine pairwise relationships between environmental factors and CH4 fluxes. We also used PCA and linear regressions to explore the sensitivity of CH4 fluxes to simultaneous environmental fluctuations in wind speed, Tair, air relative humidity, Rn, vapor pressure deficit (VPD), albedo, SHF, SWC, and Tsoil. Before performing PCA and linear regressions, the entire dataset was examined for outliers (Cook's distance, <0.002), homogeneity of variance (Levene's test, p<0.05), normality (Kolmogorov–Smirnov test; smooth line for histogram of Studentized residuals), collinearity (variance inflation factor, ), potential interactions (t test, p<0.05), and independence of observations (t test, p<0.05).
We performed structural equation modeling (SEM) to evaluate the effects of environmental variables on CH4 fluxes for different seasons. SEM is a widely used multivariate statistical tool that incorporates factor analysis, path analysis, and maximum likelihood analysis. This method uses a priori knowledge of the relationships among focus variables to verify the validity of hypotheses. Here we performed SEM analyses with AMOS 21.0 (Amos Development Corporation, Chicago, IL, USA). All data are presented as mean values with standard deviations.
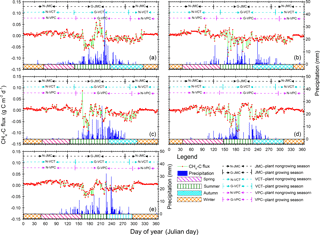
Figure 3Annual patterns of diel methane (CH4) flux and precipitation variations from 2012 to 2016. Positive values indicate CH4 release and negative values indicate CH4 uptake by ecosystems. Red dots and light green lines are CH4-C flux variation, and the deep blue histograms show diel precipitation accumulation. Pink, olive, cyan, and orange blocks mean spring, summer, autumn, and winter seasons, respectively, according to our new method of SMT (see Sect. 2). Black, cyan, and pink dotted lines with bars separated the plant growing from nongrowing seasons and stand for seasons with the JMC, VCT, and VPC methods, respectively. Details about the JMC, VCT, and VPC methods can be found in Sect. 3.2.
3.1 Meteorological conditions
We first reported the statistics of the meteorological conditions at the Beilu'he permafrost weather station between 2012 and 2016. Mean annual Tair was −4.5∘ (Fig. S1 in the Supplement), with minimum and maximum mean diel temperatures of −21.6∘ (12 January 2012) and 13.8∘ (28 July 2015), respectively. Average net radiation was 82.8 W m−2, with the maximum in August (136.2 W m−2; Fig. S2). The average VPD was about 0.3, with maximum and minimum values of 0.98, and 0.02, respectively (Fig. S3). Mean annual precipitation was 335.4 mm (Fig. 3), which was primarily based on rain and snowfall (only occupied 7 %). Maximum and minimum precipitation was recorded in 2013 (488.3 mm) and 2015 (310.0 mm), respectively. The majority of precipitation, approximately 92 %, hereby occurred in the summer. During the winter, precipitation was rare, with mean values around 6.7 mm. Spring was another important rainfall period in addition to summer, with mean precipitation being about 37.5 mm, or 8∼17 % of the total.
The Beilu'he site is windy during most of the year (Fig. S4). Its annual average speed was 4.4 m s−1 from 2012 to 2016, while the principal direction of the strongest winds was from the southwest. Late autumn, winter, and early spring drought brought increased risks of dust-blowing days, with an average of 122 days within a year. Its summer average wind speed was about 3.30 m s−1, predominantly driven by the southwest wind.
The SWC and Tsoil variability from 2012 to 2016 at the field site are summarized in Figs. S5 and S6, respectively. Mean SWC of depths of 10, 20, 40, 80, and 160 cm were 14 %, 9 %, 8 %, 14 %, and 19 %. Tsoil of depths <100 cm corresponded with the Tair changes, but showed stronger differences at depths >100 cm. The Tsoil at 200 cm of depth showed a remarkable difference from that of other layers. The reason could be the occurrence of peat in this layer, and that, during winter, the peat layer was not completely frozen. Figure S7 shows SHF half-hour and diel-scale variability of 5 and 15 cm in depth. The annual mean value of SHF at 5 and 15 cm of depth is 7.6 and 6.8 W m−2, respectively.
Finally, Fig. S8 shows the site's average soil freezing and thawing dynamics observed from January 2012 to December 2016. The average ALT is 4.4 m from 2012 to 2016. At 40 cm of depth the duration of the active layer ranged from 174 to 188 days, with an average variation of up to 14 days.
3.2 Annual, seasonal, and diel variabilities in methane fluxes
Our results indicated that the Beilu'he site was a CH4 sink, with an annual mean strength of g CH4-C m−2 (95 % confidence interval; negative values mean CH4 sinks; positive values mean CH4 sources). The strength of the CH4 sink varies across different years from g CH4-C m−2 yr−1 in 2015 to g CH4-C m−2 yr−1 in 2014 (Fig. 3). The amount of gene expression by methanogens and methanotrophs at 0–25 cm soils in March and November, for instance, was about 16.8 % and 35.6 %, respectively, suggesting strong microbial activities even during the cold and dry plant nongrowing season (Fig. 2).
We also clearly observed CH4 seasonal variations (Fig. S9) in both the number of CH4 exchanges and their diel cycles (Fig. 4). Across different seasons the footprint of the monitored CH4 flux changed following the change of the prevalent wind direction. In winter and spring, the major footprint was from east of the EC tower; while in summer and autumn, the major footprint was from west and north of the EC tower (Fig. S4).
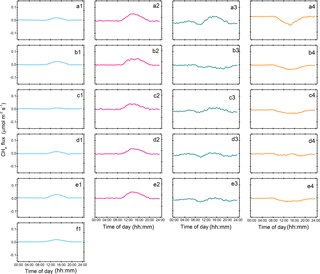
Figure 4Diel CH4 fluxes from 2012 to 2016 for different seasons. Blue, pink, green and orange, represent winter, spring, summer, and autumn, respectively: (a1–a4) 2012, (b1–b4) 2013, (c1–c4) 2014, (d1–d4) 2015, (e1–e4), and (f1) 2016.
In winter, the net CH4 flux at the Beilu'he site was an atmospheric source, with an average annual rate of 0.41±0.16 g CH4-C m−2 yr−1 or 4.35±0.33 mg CH4-C m−2 d−1 (Fig. S9a). It should also be noted that since the investigation started on 1 January 2012 and ended on 31 December 2016, the 2011–2012 and 2016–2017 winters were only about half of the regular length. The diel CH4 cycle of an average winter day was characterized by one single emission peak around 10:30–17:30 Beijing time (note all times hereafter are in Beijing time; Fig. 4a1–f1).
In spring, the Beilu'he site was a CH4 source of 0.90±0.37 g CH4-C m−2 yr−1 (Fig. S9b), accounting for 53 % of annual CH4 emissions, or 1.81±0.22 mg CH4-C m−2 d−1. For a typical spring day (Fig. 4a2–e2), diel CH4 emission usually started at around 10:00–10:30, when the thin ice layer on the soil surface started to thaw. It then reached the peak at 12:30–13:30. The emission peak started to weaken at around 15:30–16:00 and reached around zero or even turned into a small sink after 20:00.
Table 3Correlation coefficients between CH4 fluxes and environment factors on half-hour timescales.
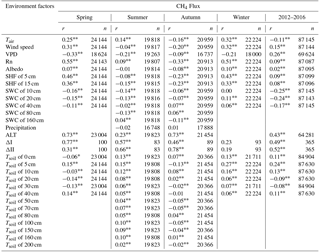
Note means p<0.01; * means p<0.05; r values are for the relationship between CH4 flux and environment factors. Tair means air temperature of 3 m above the ground surface. VPD is vapor pressure deficit, Rn is net radiation, and SWC is soil water content. ALT is active layer thickness, which fitted through the depth of soil 0∘ in Surfer 8.0, and the data are removed as meaningless in winter. Tsoil is the temperature of the soil. In spring and winter, precipitation data are too sparse for statistical analysis. ΔI is the soil 0–25 cm archaeal methanogen gene expression, and ΔII is the soil 0–25 cm methanotrophic gene expression. The coefficients (r) between CH4 flux and ΔI and ΔII are obtained using the synchronous CH4 fluxes averaged for 5 days.
In summer, the Beilu'he site was a CH4 sink of g CH4-C m−2 yr−1 (Fig. S9c), or mg CH4-C m−2 d−1. The diel cycle of CH4 fluxes in summer was characterized by two absorption peaks and one small emission peak (Fig. 4a3–e3). With Tair increasing after sunrise, the soil started to absorb atmospheric CH4 and this soil uptake process reached its first peak at around 09:30–10:30. After that, the continuously increasing Tair turned to suppress CH4 uptake and promote CH4 emissions, likely due to different temperature sensitivities of methanotrophic and methanogenic bacteria. At around 15:30–16:00, when Tair reached the maximum (Fig. S1b), CH4 emission also reached its peak. The following temperature decrease in the late afternoon again reversed the CH4 uptake–emission process, and by sunset we observed another CH4 sink peak. The rate of CH4 sink then decreased again through the night with further decreasing temperature.
Autumn was another season with a net CH4 sink, with the season having the highest observed value for the site as a CH4 sink in 2013 (Fig. S9d). The CH4 sink in autumn varied between g CH4-C m−2 (2015) and g CH4-C m−2 (2013), with an average diel rate of g CH4-C m−2 yr−1 or mg CH4-C m−2 d−1. The diel dynamics of autumn CH4 fluxes was like a letter “V”, with a single sink peak during 13:30–15:30 (Fig. 4a4–e4).
3.3 Response of methane fluxes to changes in environmental factors
Diel fluxes of CH4 were correlated either positively or negatively with many biotic and abiotic environmental factors (Table 3). Positive factors include metagenomics of both methanotrophic (r=0.52, p<0.01) and methanogens (r=0.49, p<0.01) at 0–25 cm soils, ALT (r=0.43, p<0.01), and wind speed (r=0.15, p<0.01). Important negative factors include VPD (, p<0.01), SWC at all depths (varied r values between −0.17 and −0.26, p<0.01), Tair (, p<0.01), and air pressure (, p<0.01). The correlation signal between CH4 fluxes and Tsoil changed with soil depths (varied r values between −0.09 and 0.24, p<0.01). Furthermore, path analysis results showed that Tsoil values at 5 and 10 cm were the most important factors, which together contributed about 25 % of the relative importance coefficient. Following these factors in importance were SWC at 80 cm (14 %) and 20 cm (12 %) and Tsoil at 20 cm (8 %).
Further analyses suggested that dominant control factors of CH4 fluxes also changed among different seasons. In spring, Rn was the most important factor, with a relative importance coefficient near 60 %, followed by SHF at 5 cm (9 %) and SWC at 20 cm (6 %). Table 4 shows the results of the PCA. In spring, PC1 explained 63 % of the CH4 variations, which was positively correlated with Tair, VPD, Rn, SHF of 15 cm, ALT, ΔI, SWC of 10–40 cm, Tsoil of 0 cm, Tsoil of 5–20 cm, and Tsoil of 30–50 cm and negatively correlated with wind speed. The PC2 explained about 23 % of CH4 flux variations. The first four principal components explained about 86 % of the CH4 variations.
In summer, CH4 fluxes were mostly related with Tsoil at 100 and 200 cm, with a relative importance coefficient of about 30.2 % and 26.5 %, respectively. Other important environmental determinants of CH4 fluxes were Tsoil at 70 cm (12.3 %) and Tsoil at 0–20 cm (11.4 %). The first four principal components explained about 88 % of the CH4 variations (Table 4). PC1 explained 70 % of the CH4 variations and was positively correlated with wind speed, Tair, VPD, SHF of 15 cm, ALT, ΔI, SWC of 50–160 cm, precipitation, Tsoil of 0 cm, Tsoil of 5–40 cm, Tsoil of 50–80 cm, and Tsoil of 100–200 cm, but negatively correlated with Rn and SWC of 10–40 cm.
In autumn, Rn and Tsoil at 5–20 cm had the highest relative importance coefficients for explaining the CH4 flux variation. The first four principal components explained about 86 % of the CH4 variations (Table 4). PC1 explained 69 % of the CH4 variations and was positively correlated with Tair, VPD, Rn, SHF of 15 cm, ALT, ΔI, SWC of 10–40 cm, SWC of 50–160 cm, Tsoil of 0 cm, Tsoil of 5–40 cm, Tsoil of 50–80 cm, and Tsoil of 100–200 cm, but negatively correlated with wind speed.
Table 4Principal component analysis (PCA) of the environmental factors.
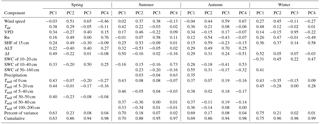
Note PC means principal component. Before PCA, SWC was divided into three parts, 10–20, 10–40, and 50–160 cm, according to a collinearity test in four seasons. Tsoil was divided into six parts of Tsoil of 0 cm, Tsoil of 5–20 cm, Tsoil of 5–40 cm, Tsoil of 30–50 cm, Tsoil of 50–80 cm, and Tsoil of 60–200 cm according to a collinearity test in different seasons.
During winter, Rn was again the most important factor (34 % relative importance coefficient), followed by Tsoil at 0–40 cm (27 % in total) and a SHF of 15 cm (17 % in total), in determining CH4 fluxes. The first four principal components explained about 96 % of the CH4 variations (Table 4). PC1 explained 75 % of the CH4 variations and was positively correlated with wind speed, Tair, VPD, Rn, SHF of 15 cm, ΔI, Tsoil of 0 cm, and Tsoil of 5–20 cm.
3.4 Empirical model comparison for different CH4 flux season classification system
Lastly, we also compared how different season definitions, including the methods of SMT, VCT, JMC, and VPC, may have impacted the predictability of CH4 fluxes. We established empirical maximum likelihood models between all environmental factors and diel CH4 fluxes over each season, and then we compared modeled CH4 fluxes and field observations under those methods of different seasonal definitions (Fig. 5). We found that the agreement between modeled and observed CH4 fluxes, using the new SMT method, reached R2=0.28, almost twice that of the VPC (R2=0.17) and VCT (R2=0.14) methods, and more than three times that of the JMC method (R2=0.08; Fig. 5). Hence, the comparison suggested that our new method could better model CH4 fluxes over a year. The use of the traditional plant growing season versus nongrowing season definitions may also underestimate or overestimate CH4 sinks or sources, especially when many studies assume CH4 is close to zero during the plant nongrowing season. Furthermore, the new SMT method accurately captures the impact of spring and autumn permafrost thawing–freezing cycles on CH4 fluxes and the different preferable environments for methanogens and methanotrophic bacteria during the summer season, while conventional methods do not.
4.1 Annual and season mean and diel variability
Our results suggested that the alpine steppe ecosystem in Beilu'he was a CH4 sink of about g CH4-C m−2 yr−1 during the study period of 2012–2016. This sink strength is larger than that of previous reports from other sites of the QTP (Cao et al., 2008; Wei et al., 2012; Li et al., 2012; Song et al., 2015; Chang and Shi, 2015) and many other high-latitude Arctic tundra ecosystems, like northeast Greenland (Jørgensen et al., 2015), western Siberia (Liebner et al., 2011), and Alaska (Whalen et al., 1992; Zhuang et al., 2004; Whalen, 2005). Different soil hydrothermal conditions, which previous studies have shown will greatly influence CH4 cycles in permafrost regions (Spahni et al., 2011; Kirschke et al., 2013), may partly explain the site difference in CH4 dynamics. For example, compared to the wet and often snow-covered high-latitude Arctic tundra ecosystems, there is no or little snow cover during the cold season in the QTP alpine steppes (Table S1 in the Supplement). During winter, the Beilu'he meteorological data show that the snow cover lasting <33.7 h, with a SWC of 0–40 cm within a footprint <7.6 % from 2012 to 2016 (Table S1), is far below high-latitude Arctic tundra ecosystems. Jansson and Taş (2014) pointed out that relatively dry soils could facilitate the oxidation of CH4 since the increased number of gaps between soil particles in dry soils enhances the diffusion of oxygen (O2) and CH4 molecules and promotes aerobic respiration of soil microorganisms (Wang et al., 2014; Song et al., 2015). Meanwhile, unfrozen or capillary water found in cold season permafrost soils ensures sufficient soil moisture for microbial activities, even in relatively drier and cold soils (Panikov and Dedysh, 2000; Rivkina et al., 2004). In addition, many previous studies used static chambers in CH4 measurements and may not have included a plant nongrowing season (Wei et al., 2015a; Wang et al., 2014). Static chambers could underestimate CH4 uptake because of the additional chamber heating-induced CH4 emissions and frequent measurement gaps from overheating preventive shutdowns (Sturtevant et al., 2012).
We argued that seasonal freezing and thawing dynamics may be a key reason to explain the site's seasonal difference in CH4 dynamics. Freezing and thawing processes are typical characteristics of the QTP permafrost (Wang et al., 2008, 2000; Qin et al., 2016). Our work suggests that freezing and thawing dynamics have played a critical role in governing permafrost seasonal and diel CH4 cycling. For instance, while both spring and autumn are active seasons for the freeze–thaw dynamics of top soil layers and share many similarities, they have opposite CH4 processes and soils that emit CH4 during spring (Fig. S9b) but consume CH4 during autumn (Fig. S9d). We hypothesize that the difference in the freezing and thawing processes of the two seasons may have played a critical role in determining the direction of CH4 dynamics. In spring, the SWC of 10 cm, of 20–40 cm, of 80 cm, and of 160 cm depth is 12.4 %, 9.2 %, 11.4 %, and 13.6 %, respectively (Table S1). The active soil layer thaws from top to bottom (Jin et al., 2000; Cao et al., 2017), and the permafrost table is very shallow (about 10–45 cm) and often waterproof (Wu and Zhang, 2008; Song et al., 2015; Lin et al., 2015). The water thawed during the daytime would freeze again at night on the soil surface (Fig. S10a; Shi et al., 2006; Wu and Zhang, 2010b). The thin-ice layer could stop atmospheric gases of CH4 and O2 from getting into the soils (Gažovič et al., 2010). During autumn, the SWC is 15.3 % at 10 cm below ground, decreases to 9.4 % at 20–40 cm, and then increases to 13.6 % and 21.0 % at 80 cm and 160 cm, respectively (Table S1). However, soils are bidirectionally frozen from both the top (ground surface) and bottom (permafrost table), which is about 200–400 cm below ground (Fig. S8; Wu and Zhang, 2010a). On the one hand, the frozen soil of the ground surface (about 0–40 cm) prevents the outside liquid water from permeating. On the other hand, the freezing itself will reduce the liquid water content in the soil. Therefore, it creates finely closed anaerobic gaps that allow CH4 and O2 gases into deep soils (about 50–400 cm; Mastepanov et al., 2008, 2013; Zona et al., 2016). Meanwhile, the temperature of deep soils (about 50–400 cm) still remains at a relatively high level (Fig. S10b), and methanotrophic bacteria will still be active at this high Tsoil (Fig. 2). This could be one important mechanism for autumn soil CH4 consumption. In addition, in principal it is also possible that the observed seasonal variation in CH4 flux may actually arise from the spatial variation in the footprint covered by the EC site (within 175 m), given that prevalent wind direction changes seasonally (Fig. S4). Nonetheless, we found that the same vegetation species and soil exist in different directions from the tower within the footprint (Fig. S11). This spatial vegetation and soil homogeneity rules out the potential influence of footprint changes on the sign of CH4 balances and further confirms that seasonal soil freezing and thawing differences may likely be the main explanation for seasonal CH4 variations.
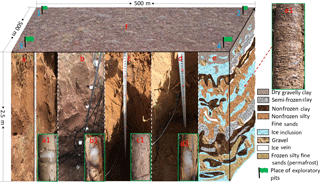
Figure 6Location of exploratory pits and drillings in this study in autumn: (f) photo of a typical ground surface (16 October 2014). Green flags represent the location for the soil survey using test pits and drilling. (a, b, c, d) Soil profiles of 0–250 cm of depth at the north (1), south (2), east (3), and west (4) corners of the eddy covariance footprint, respectively. (a1, b1, c1, d1) Drilling cores, with clear ice (white) in (a1, b1, d1), but not in (c1). (e) An illustration that combines results from drillings, test pits, and multichannel ground-penetrating radar (Malå Geoscience, Sweden) for active layer variations in permafrost area during the autumn season. (e1) A core sample of the same drilling (16 October 2014).
Furthermore, we suggested that the specific autumn soil vertical structure may help to explain why the site was a CH4 sink, unlike the CH4 source in spring. The sequential probing data enables us to establish a rough estimate on the soil vertical structure during the autumn thawing–freezing process, in which the vertical distribution of clay, sandy soils, and soil organic layers was mixed like a multilayer structure, rather than forming a gradual change (Fig. 6e). As the soil profile is vertically different in features such as soil density, thermal conductivity, latent heat, and soil salinity, we boldly conjecture that the Tsoil, SWC, and soil microbial activities also had a similar layer type of vertical distribution. As a result, layers of frozen and thawed soils were not changing gradually but appeared like a layered structure too. This soil vertical structure trapped high concentrations of soil water between the frozen layers and was therefore highly anaerobic and suitable for CH4 production. It may also allow speculation that biogenic CH4 between frozen layers could not escape in autumn. The biogenic CH4 would be trapped until the active soil layer was completely frozen in late autumn, and in some warmer years until early winter, and created frost cracks. This would enable it to escape and may explain why there was a large burst of CH4 emissions in late autumn and early winter and may also explain the constantly weak CH4 emission through the winter season, although methanogenic bacteria may have stopped functioning in the low temperatures of winter. Of course, further studies and direct data collection in the field will be needed to fully test the hypothesis.
4.2 Impacts of environmental, permafrost, and microbial activities on CH4 fluxes
Our results demonstrated the important roles of climate, freezing and thawing dynamics, and soil microbe activities in regulating the direction and amount of CH4 exchange between the atmosphere and ecosystems in permafrost areas. The key role of the above factors and processes was also confirmed by the better representation of seasonal CH4 cycles by our new seasonal division method based on soil microbes, temperature, and permafrost dynamics rather than Tair or vegetation phenology. Here, we further discuss potential mechanisms of how environmental (including air and soil heat and water), freezing and thawing processes, and soil microbes control the production and absorption of CH4.
First, it is noteworthy that both the strength and direction of correlations among CH4 fluxes, SWC, and Tsoil parameters changed with soil depths, particularly during spring and autumn, when active layer soils shifted between thawing and freezing regularly. The positive and negative CH4 flux correlations with Tsoil and SWC may suggest that the impacts of Tsoil and SWC on CH4 fluxes shall be treated as a holistic process (Table 3), rather than as separate ones. For instance, in autumn, the significant correlation between CH4 fluxes and Tsoil or SWC was positive at some soil depths, but negative at some other depths, reaching the maximum at the depth of 80 cm. Further, in situ observations suggested that soil organic matter and soil microbe number were also at a very high level at this depth, highlighting that the regulation of soil abiotic factors on CH4 cycling may be highly influenced by soil biotic activities. In addition, the holistic soil heat–water process could also determine the concentration of soil inorganic ions, particularly during spring and autumn, which were critical factors controlling the amount of soil unfrozen water. Earlier studies suggested that soil unfrozen water is important for maintaining soil microbial activities in winter (Panikov and Dedysh, 2000; Rivkina et al., 2004). In the future we will include data for soil unfrozen water to test its role in regulating CH4 exchanges in permafrost regions.
Tair and precipitation impact CH4 fluxes indirectly through their influences on Tsoil and SWC (Zhuang et al., 2004; Lecher et al., 2015). Such indirect influences may often be characterized with time-lagged effects (Koven et al., 2011). For instance, post-drought rainfall events in summer can first promote soil CH4 consumption (summer of 2014). This is because certain soil moisture is needed for methanogenic bacteria to function (Del et al., 2000; Luo et al., 2012). Yet, prolonged rainfall will eventually cause CH4 fluxes to change from negative (soils consume CH4) to positive (soils emit CH4) fluxes (for example, day 168 to 183 of 2015; Fig. 3d). After rainfall events, CH4 flux gradually turned negative again with the decrease in SWC. As a result of these time-lagged effects, the correlation coefficient between CH4 fluxes and precipitation often appears very low, although still statistically significant.
Second, soil methanogenic and methanotrophic bacteria could coexist with different optimal niches (e.g., ranges of Tair/Tsoil and SWC; Zhuang et al., 2013; Lau et al., 2015; Wei et al., 2015a). For example, the CH4 diel cycle in summer was found to have two strong consumption peaks and one weak emission peak (Fig. 4a3, c3, d3, e3). The timing of these different peaks may well reflect the different environmental requirements for the dominance of methanogens and methanotrophic bacteria. Furthermore, methanogens may have a broader functional temperature range than methanotrophic bacteria (Kolb, 2009; Lau et al., 2015; Yang et al., 2016). This is also evident, for example, from the diel CH4 cycle in autumn when CH4 consumption was minimal at both the lowest and highest Tair (Fig. 4a4–e4).
The complex relationships between CH4 fluxes and environmental factors make it a grand challenge to predict the future of the QTP CH4 budget under a changing climate. For instance, it has been generally believed that the ALT will increase under projected warming (Wu and Liu, 2004). The positive correlation between CH4 fluxes and ALT found here suggests that the QTP permafrost CH4 sink may thus be weakened. However, the negative correlation between CH4 flux and Tair may lead to a different conclusion. Incorporating our findings and high-resolution data into mechanistic CH4 models is therefore needed to enhance our capacity in predicting future CH4 budgets. Earth system models have been introduced to estimate CH4 dynamics (Curry, 2007; Spahni et al., 2011; Bohn et al., 2015). For example, using a terrestrial ecosystem modeling approach, Zhuang et al. (2004) estimated the average QTP permafrost CH4 sink of −0.08 g C m−2 yr−1, much smaller than our field-based CH4 estimate ( g CH4-C m−2 yr−1). Current CH4 models focus on the regulation of CH4 processes by temperature and SWC and usually lack high-resolution data for model parameterization (Bohn et al., 2015). Data interpolation and the use of average values of certain environmental factors are normal practices in most models (Zhuang et al., 2004), which may overlook the impacts of environmental variations on CH4 dynamics. For example, at Beilu'he, Tair on a typical summer day (e.g., 6 July 2013) could vary between −6 and 28 ∘C, a difference of 34 ∘C. The resulting diel mean temperature, 17 ∘C, is beyond the range of methanotrophic bacteria's preferable temperature of 20–30 ∘C (Segers, 1998; Steinkamp et al., 2001; Yang et al., 2016). Therefore, models using diel mean temperature as an input may estimate the site as a net CH4 sink. However, field observations show a source with a sink only during a short period (08:30–11:30), on 6 July 2013, because the short period of the sink was offset by the source over the remaining 21 h.
Furthermore, half-hourly SWC was related with the waterproof role by the permafrost layer during spring and autumn (Fig. 6a). However, because of the shortage of high-temporal-resolution data, half-diel or diel mean SWC data are often used in many previous studies (Zhu et al., 2004; Jiang et al., 2010; Wei et al., 2015b), which could not correctly show the regulation of permafrost soil properties that are critical for CH4 dynamics. As another example, Tsoil of 0–50 cm in depth is one of the most important factors related to CH4 fluxes (Mastepanov et al., 2008). However, many studies used Tair or reanalyzed deep Tsoil instead (Zhu et al., 2004; Bohn et al., 2015; Oh et al., 2016). Because the active layer is not homogeneous, but with different thermal conductivities during the freezing and thawing process, the use of Tair or deep Tsoil brings in large uncertainties in CH4 modeling. Future research needs to improve mechanistic understanding of CH4 dynamics and their biotic and abiotic control factors and to conduct more high-resolution and long-term field monitoring.
4.3 The classification system of the four seasons for CH4 studies
Our study also differs from the majority of earlier studies regarding the definition of the seasons (Treat et al., 2014; Wang et al., 2014; Wei et al., 2015a; Song et al., 2015). Here, we adopted a new classification system of the four seasons based on 0–25 cm soil depth bacterial activities (Fig. 2), Tsoil of 0–40 cm (Fig. S6a), and ALT (Fig. S8), rather than the conventional methods based on Tair and vegetation dynamics (Chen et al., 2011; McGuire et al., 2012). Previous studies indicated that changes in CH4 fluxes are regulated by soil microbes, and activities of soil microbes are not limited to the warm season (Zhuang et al., 2004; Lau et al., 2015; Yang et al., 2016). For instance, in March and November, we found the amount of gene expression by methanogens and methanotrophs in 0–25 cm soils were about 16.8 % and 35.6 % (Fig. 2), respectively, suggesting there are still strong microbial activities during the cold and dry season. Therefore, our new method of defining the four seasons from the top soil biotic and abiotic features better captures the pattern of CH4 dynamics throughout a year.
Our field data indicate that there was a large CH4 sink in the QTP permafrost area during recent years. The strength of this CH4 sink is larger than found in previous studies in the same region and many high-latitude tundra ecosystems. This study highlights the complexity of environmental controls, including soil heat–water processes, permafrost freezing and thawing dynamics, and soil microbial activities, on CH4 cycling. This complexity implies that linear interpolation and extrapolation from site-level studies could introduce large uncertainties in CH4 flux estimation. Future quantification of CH4 dynamics in permafrost regions needs to account for the effects of complex environmental processes. Our findings also highlight the importance of conducting more high-resolution and long-term field monitoring in permafrost regions for better understanding and modeling of permafrost CH4 cycling under a changing climate.
The data are available through the corresponding authors and will be made publicly available through the State Key Laboratory of Frozen Soil Engineering Data Center in the near future (http://sklfse.casnw.net/).
The supplement related to this article is available online at: https://doi.org/10.5194/tc-12-2803-2018-supplement.
HY, QW, and HJ designed the research. HY, QZ, and AC performed eddy-covariance data analysis and calculations. HY and AC drafted the paper. TY, ZL, YQ, and LL contributed to the interpretation of the results and to the text. YY and GL performed the field data collection. All authors contributed to writing the paper.
The authors declare that they have no conflict of interest.
We would like to thank Yongzhi Liu, Jing Luo, Ji Chen, Guilong Wu, Wanan Zhu,
Zhipeng Xiao, and Chang Liao for their tremendous help in collecting field
data over all these years. We also want to pay tribute and show our gratitude
to the late Xiaowen Cui for his contribution to our many field adventures. We
thank John McCabe for reading over a previous version of the paper. This
study was supported by the National Natural Science Foundation of China
(41501083), Key Research Program of Frontier Sciences, Chinese Academy of
Sciences (QYZDJ-SSW-DQC011), Opening Research Foundation of Key Laboratory of
Land Surface Process and Climate Change in Cold and Arid Regions, Chinese
Academy of Sciences (LPCC201307), and Opening Research Foundation of Plateau
Atmosphere and Environment Key Laboratory of Sichuan Province
(PAEKL–2014–C3). Anping Chen acknowledges the support from a Purdue
University Forestry and Natural Resources research scholarship.
Edited by: Christian Hauck
Reviewed by: two anonymous referees
Bohn, T. J., Melton, J. R., Ito, A., Kleinen, T., Spahni, R., Stocker, B. D., Zhang, B., Zhu, X., Schroeder, R., Glagolev, M. V., Maksyutov, S., Brovkin, V., Chen, G., Denisov, S. N., Eliseev, A. V., Gallego-Sala, A., McDonald, K. C., Rawlins, M. A., Riley, W. J., Subin, Z. M., Tian, H., Zhuang, Q., and Kaplan, J. O.: WETCHIMP-WSL: intercomparison of wetland methane emissions models over West Siberia, Biogeosciences, 12, 3321–3349, https://doi.org/10.5194/bg-12-3321-2015, 2015.
Burba, G. G., Mcdermitt, D. K., and Grelle, A.: Addressing the influence of instrument surface heat exchange on the measurements of CO2 flux from open–path gas analyzers, Glob. Change Biol., 14, 1854–1876, 2008.
Cao, B., Gruber, S., and Zhang, T.: Spatial variability of active layer thickness detected by ground–penetrating radar in the Qilian Mountains, Western China, J. Geophys. Res.-Earth, 122, 574–591, 2017.
Cao, G., Xu, X., and Long, R.: Methane emissions by alpine plant communities in the Qinghai–Tibet Plateau, Biol. Lett., 4, 681–684, 2008.
Cate, R. B. and Nelson, L. A.: A simple statistical procedure for partitioning soil test correlation data into two classes, Soil Sci. Soc. Am. J., 35, 658–660, 1971.
Chang, R., Miller, C., and Dinardo, S.: Methane emissions from Alaska in 2012 from CARVE airborne observations, P. Natl. Acad. Sci. USA, 111, 16694–16699, 2014.
Chang, S. and Shi, P.: A review of research on responses of leaf traits to climate change, Chinese Journal of Plant Ecology, 39, 206–216, 2015.
Chen, W., Wolf, B., and Zheng, X.: Annual methane uptake by temperate semiarid steppes as regulated by stocking rates, aboveground plant biomass and topsoil air permeability, Glob. Change Biol., 17, 2803–2816, 2011.
Curry, C.: Modeling the soil consumption at atmospheric methane at the global scale, Global Biogeochem. Cy., 21, GB4012, https://doi.org/10.1029/2006GB002818, 2007.
Del, G., Parton, W., and Mosier, A. R.: General CH4 oxidation model and comparisons of CH4 oxidation in natural and managed systems, Global Biogeochem. Cy., 14, 999–1019, 2000.
Dengel, S., Zona, D., Sachs, T., Aurela, M., Jammet, M., Parmentier, F. J. W., Oechel, W., and Vesala, T.: Testing the applicability of neural networks as a gap-filling method using CH4 flux data from high latitude wetlands, Biogeosciences, 10, 8185–8200, https://doi.org/10.5194/bg-10-8185-2013, 2013.
Falge, E., Baldocchi, D., and Olson, R.: Gap filling strategies for defensible annual sums of net ecosystem exchange, Agr. Forest Meteorol., 107, 43–69, 2001.
Foken, T. and Wichura, B.: Tools for quality assessment of surface-based flux measurements, Agr. Forest Meteorol., 78, 83–105, 1996.
Gažovič, M., Kutzbach, L., and Schreiber, P.: Diurnal dynamics of CH4 from a boreal peatland during snowmelt, Tellus B, 62, 133–139, 2010.
IPCC: Climate Change 2013: The Physical Science Basis. Contribution of Working Group I to the Fifth Assessment Report of the Intergovernmental Panel on Climate Change, edited by: Stocker, T. F., Qin, D., Plattner, G.-K., Tignor, M., Allen, S. K., Boschung, J., Nauels, A., Xia, Y., Bex, V., and Midgley, P.M., Cambridge University Press, Cambridge, United Kingdom and New York, NY, USA, 1535 pp., 2013.
Jansson, J. K. and Tas, N.: The microbial ecology of permafrost, Nat. Rev. Microbiol., 12, 414–425, 2014.
Jiang, C., Yu, G., and Fang, H.: Short–term effect of increasing nitrogen deposition on CO2, CH4 and N2O fluxes in an alpine meadow on the Qinghai–Tibetan Plateau, China, Atmos. Environ., 44, 2920–2926, 2010.
Jin, H., Li, S., and Cheng, G.: Permafrost and climatic change in China, Global Planet. Change, 26, 387–404, 2000.
Jørgensen, C. J., Johansen, K. M. L., and Westergaard-Nielsen, A.: Net regional methane sink in High Arctic soils of northeast Greenland, Nat. Geosci., 8, 20–23, 2015.
Kirschke, S., Bousquet, P., and Ciais, P.: Three decades of global methane sources and sinks, Nat. Geosci., 6, 813–823, 2013.
Kolb, S.: The quest for atmospheric methane oxidizers in forest soils, Env. Microbiol. Rep., 1, 336–346, 2009
Koven, C. D., Ringeval, B., and Friedlingstein, P.: Permafrost carbon–climate feedbacks accelerate global warming, P. Natl. Acad. Sci. USA, 108, 14769–14774, 2011.
Lau, M., Stackhouse, B. T., and Layton, A. C.: An active atmospheric methane sink in high Arctic mineral cryosols, ISME J., 9, 1880–1891, 2015.
Lecher, A. L., Dimova, N., and Sparrow K.,J.: Methane transport from the active layer to lakes in the Arctic using Toolik Lake, Alaska, as a case study, P. Natl. Acad. Sci. USA, 112, 3636–3640, 2015.
Lee, X., Massman, W., and Law, B. (Eds.): Handbook of Micrometeorology: A Guide for Surface Flux Measurement and Analysis, Springer Science and Business Media, Part of the Atmospheric and Oceanographic Sciences Library book series (ATSL), 29, https://doi.org/10.1007/1-4020-2265-4, 2006.
Li, K., Gong, Y., and Song, W.: Responses of CH4, CO2 and N2O fluxes to increasing nitrogen deposition in alpine grassland of the Tianshan Mountains, Chemosphere, 88, 140–143, 2012.
Liebner, S., Zeyer, J., and Wagner, D.: Methane oxidation associated with submerged brown mosses reduces methane emissions from Siberian polygonal tundra, J. Ecol., 99, 914–922, 2011.
Lin, Z., Burn, C. R., and Niu, F.: The Thermal Regime, including a Reversed Thermal Offset, of Arid Permafrost Sites with Variations in Vegetation Cover Density, Wudaoliang Basin, Qinghai–Tibet Plateau, Permafrost Periglac., 26, 142–159, 2015.
Loescher, H. W., Law, B. E., and Mahrt, L: Uncertainties in, and interpretation of, carbon flux estimates using the eddy covariance technique, J. Geophys. Res., 111, D21S90, https://doi.org/10.1029/2005JD006932, 2006.
Lund, M., Lafleur, P. M., and Roulet, N. T.:Variability in exchange of CO2 across 12 northern peatland and tundra sites. Glob. Change Biol., 16, 2436–2448, 2010.
Luo, G. J., Brüggemann, N., Wolf, B., Gasche, R., Grote, R., and Butterbach-Bahl, K.: Decadal variability of soil CO2, NO, N2O, and CH4 fluxes at the Höglwald Forest, Germany, Biogeosciences, 9, 1741–1763, https://doi.org/10.5194/bg-9-1741-2012, 2012.
Mastepanov, M., Sigsgaard, C., and Dlugokencky, E. J.: Large tundra methane burst during onset of freezing, Nature, 456, 628–630, 2008.
Mastepanov, M., Sigsgaard, C., Tagesson, T., Ström, L., Tamstorf, M. P., Lund, M., and Christensen, T. R.: Revisiting factors controlling methane emissions from high-Arctic tundra, Biogeosciences, 10, 5139–5158, https://doi.org/10.5194/bg-10-5139-2013, 2013.
Mauder, M., Cuntz, M., and Drüe, C.: A strategy for quality and uncertainty assessment of long–term eddy–covariance measurements, Agr. Forest Meteorol., 169, 122–135, 2013.
McGuire, A. D., Christensen, T. R., Hayes, D., Heroult, A., Euskirchen, E., Kimball, J. S., Koven, C., Lafleur, P., Miller, P. A., Oechel, W., Peylin, P., Williams, M., and Yi, Y.: An assessment of the carbon balance of Arctic tundra: comparisons among observations, process models, and atmospheric inversions, Biogeosciences, 9, 3185–3204, https://doi.org/10.5194/bg-9-3185-2012, 2012.
Moncrieff, J., Clement, R., and Finnigan, J.: Averaging, detrending, and filtering of eddy covariance time series, in: Handbook of micrometeorology, edited by: Lee, X., Massman, W., and Law, B., Springer Netherlands, 29, 7–31, 2004.
Muller, S. W.: Permafrost or permanently frozen ground and related engineering problems, Military Intelligence Division Office, Chief of Engineers, U. S. Army, 2, 6–10, 1947.
Oh, Y., Stackhouse, B., and Lau, M.: A scalable model for methane consumption in arctic mineral soils, Geophys. Res. Lett., 43, 5143–5150, 2016.
Panikov, N. S. and Dedysh, S. N.: Cold season CH4 and CO2 emission from boreal peat bogs (West Siberia): Winter fluxes and thaw activation dynamics, Global Biogeochem. Cy., 14, 1071–1080, 2000.
Papale, D., Reichstein, M., Aubinet, M., Canfora, E., Bernhofer, C., Kutsch, W., Longdoz, B., Rambal, S., Valentini, R., Vesala, T., and Yakir, D.: Towards a standardized processing of Net Ecosystem Exchange measured with eddy covariance technique: algorithms and uncertainty estimation, Biogeosciences, 3, 571–583, https://doi.org/10.5194/bg-3-571-2006, 2006.
Patra, P. K. and Kort, E. A.: Regional Methane Emission Estimation Based on Observed Atmospheric Concentrations (2002–2012), J. Meteorol. Soc. Jpn., Ser. II, 94, 91–113, 2016.
Qin, Y., Wu, T., and Li, R.: Using ERA-Interim reanalysis dataset to assess the changes of ground surface freezing and thawing condition on the Qinghai–Tibet Plateau, Environ. Earth Sci., 75, 1–13, 2016.
Rigby, M., Prinn, R. G., and Fraser, P. J.: Renewed growth of atmospheric methane, Geophys. Res. Lett., 35, 2–7, 2008.
Rivkina, E., Laurinavichius, K., and McGrath, J.: Microbial life in permafrost, Adv. Space Res., 33, 1215–1221, 2004.
Segers, R.: Methane production and methane consumptiona–review of processes underlying wetland methane fluxes [Review], Biogeochemistry, 41, 23–51, 1998.
Shi, P., Sun, X., and Xu, L.: Net ecosystem CO2 exchange and controlling factors in a steppe–Kobresia meadow on the Tibetan Plateau, Sci. China Ser. D, 49, 207–218, 2006.
Song, W., Wang, H., and Wang, G.: Methane emissions from an alpine wetland on the Tibetan Plateau: Neglected but vital contribution of the nongrowing season, J. Geophys. Res.-Biogeo., 120, 1475–1490, 2015.
Spahni, R., Wania, R., Neef, L., van Weele, M., Pison, I., Bousquet, P., Frankenberg, C., Foster, P. N., Joos, F., Prentice, I. C., and van Velthoven, P.: Constraining global methane emissions and uptake by ecosystems, Biogeosciences, 8, 1643–1665, https://doi.org/10.5194/bg-8-1643-2011, 2011.
Steinkamp, R., Butterbach-Bahl, K., and Papen, H.: Methane oxidation by soils of an N limited and N fertilized spruce forest in the Black Forest, Germany, Soil. Biol. Biochem., 33, 145–153, 2001.
Sturtevant, C. S., Oechel, W. C., Zona, D., Kim, Y., and Emerson, C. E.: Soil moisture control over autumn season methane flux, Arctic Coastal Plain of Alaska, Biogeosciences, 9, 1423–1440, https://doi.org/10.5194/bg-9-1423-2012, 2012.
Tang, Y., Wan, S., and He, J.: Foreword to the special issue: looking into the impacts of global warming from the roof of the world, J. Plant Ecol., 2, 169–171, 2009.
Treat, C. C., Wollheim, W. M., and Varner, R. K.: Temperature and peat type control CO2 and CH4 production in Alaskan permafrost peats, Glob. Change Biol., 20, 2674–2686, 2014.
Vickers, D. and Mahrt, L.: Quality control and flux sampling problems for tower and aircraft data, J. Atmos. Ocean. Tech., 14, 512–526, 1997.
Wang, G., Li, Y., and Wang, Y.: Effects of permafrost thawing on vegetation and soil carbon pool losses on the Qinghai–Tibet Plateau, China, Geoderma, 143, 143– 52, 2008.
Wang, S., Jin, H., and Li, S.: Permafrost degradation on the Qinghai–Tibet Plateau and its environmental impacts, Permafrost Periglac., 11, 43–53, 2000.
Wang, Y., Liu, H., and Chung, H.: Non-growing season soil respiration is controlled by freezing and thawing processes in the summer monsoon-dominated Tibetan alpine grassland, Global Biogeochem. Cy., 28, 1081–1095, 2014.
Webb, E. K., Pearman, G. I., and Leuning, R.: Correction of flux measurements for density effects due to heat and water vapor transfer, Q. J. Roy. Meteorol. Soc., 106, 85–100, 1980.
Wei, D., Ri, X., and Wang, Y.: Responses of CO2, CH4 and N2O fluxes to livestock exclosure in an alpine steppe on the Tibetan Plateau, China, Plant Soil, 359, 45–55, 2012.
Wei, D., Ri, X., and Tarchen, T.: Considerable methane uptake by alpine grasslands despite the cold climate: In situ measurements on the central Tibetan Plateau, 2008–2013, Glob. Change Biol., 21, 777–788, 2015a.
Wei, D., Tarchen, T., and Dai, D.: Revisiting the role of CH4 emissions from alpine wetlands on the Tibetan Plateau: Evidence from two in situ measurements at 4758 and 4320 m above sea level, J. Geophys. Res.-Biogeo., 120, 1741–1750, 2015b.
Whalen, S. C.: Biogeochemistry of Methane Exchange between Natural Wetlands and the Atmosphere, Environ. Eng. Sci., 22, 73–94, 2005.
Whalen, S. C. and Reeburgh, W. S.: Consumption of atmospheric methane by tundra soils, Nature, 346, 160–162, 1990.
Whalen, S. C., Reeburgh, W. S., and Barber, V. A.: Oxidation of methane in boreal forest soils: a comparison of seven measures, Biogeochemistry, 16, 181–211, 1992.
Wilson K., Goldstein, A., Falge, E., Aubinet, M., Baldocchi, D., P., Berbigier, Bernhofer, C., Ceulemans, R., Dolman, H., Field, C., Grelle, A., Ibrom, A., Law, B. E., Kowalski, A., Meyers, T., Moncrieff, J., Monson, R., Oechel, W., Tenhunen, J., Valentini, R., and Verma, S.: Energy balance closure at FLUXNET sites, Agr. Forest Meteorol., 113, 223–243, 2002.
Wu, Q. and Liu, Y.: Ground temperature monitoring and its recent change in Qinghai–Tibet Plateau, Cold Reg. Sci. Technol., 38, 85–92, 2004.
Wu, Q. and Zhang, T.: Recent permafrost warming on the Qinghai–Tibetan Plateau, J. Geophys. Res., 113, D13108, https://doi.org/10.1029/2007JD009539, 2008.
Wu, Q. and Zhang, T.: Changes in active layer thickness over the Qinghai–Tibetan Plateau from 1995 to 2007, J. Geophys. Res., 115, D09107, https://doi.org/10.1029/2009JD012974, 2010a.
Wu, Q. Zhang, T., and Liu, Y.: Permafrost temperatures and thickness on the Qinghai–Tibet Plateau, Global Planet. Change, 72, 32–38, 2010b.
Yang, S., Wen, X., and Shi, Y.: Hydrocarbon degraders establish at the costs of microbial richness, abundance and keystone taxa after crude oil contamination in permafrost environments, Sci. Rep., 6, 37473, https://doi.org/10.1038/srep37473, 2016.
Zhu, X., Zhuang, Q., and Chen, M.: Net exchanges of methane and carbon dioxide on the Qinghai–Tibetan Plateau from 1979 to 2100, Environ. Res. Lett., 10, 085007, https://doi.org/10.1088/1748-9326/10/8/085007, 2004.
Zhuang, Q., Melillo, J. M., and Kicklighter, D. W.: Methane fluxes between terrestrial ecosystems and the atmosphere at northern high latitudes during the past century: A retrospective analysis with a process–based biogeochemistry model, Global Biogeochem. Cy., 18, GB3010, https://doi.org/10.1029/2004GB002239, 2004.
Zhuang, Q., Chen, M., and Xu, K.: Response of global soil consumption of atmospheric methane to changes in atmospheric climate and nitrogen deposition, Global Biogeochem. Cy., 27, 650–663, 2013.
Zona, D., Gioli, B., and Commane, R.: Cold season emissions dominate the Arctic tundra methane budget, P. Natl. Acad. Sci. USA, 113, 40–45, 2016.