the Creative Commons Attribution 4.0 License.
the Creative Commons Attribution 4.0 License.
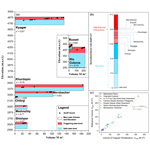
Refining lake volume estimation and critical depth identification for enhanced glacial lake outburst flood (GLOF) event anticipation
Nazir Ahmed Bazai
Peng Cui
Wang Hao
Zhang Guotao
Liu Dingzhu
Javed Hassan
Climate change leads to changes in glacier mass balance, including steady advancements and surges that reposition the glacier snouts. Glacier advancement can dam proglacial meltwater lakes. Within the Karakoram and surrounding regions, the positive feedback of climate change has resulted in more frequent ice-dammed glacial lake outburst floods (GLOFs), often facilitated by englacial conduits. However, the complex and multi-factor processes of conduit development are difficult to measure. Determining the lake depths that might trigger GLOFs and the numerical model specifications for breaching is challenging. Empirical estimates of lake volumes, along with field-based monitoring of lake levels and depths and the assessment of GLOF hazards, enable warnings and damage mitigation. Using historical data, remote sensing techniques, high-resolution imagery, cross-correlation feature tracking, and field-based data, we identified the processes of lake formation, drainage timing, and triggering depth. We developed empirical approaches to determine lake volume and trigger water pressure leading to a GLOF. A correlation, albeit a weak one, between glacier surge velocity and lake volume reveals that glacier surge may play a crucial role in lake formation and thus controls the size and volume of the lake. Lake volume estimation involves geometric considerations of the lake basin shape. A GLOF becomes likely when the lake's normalized depth (n′) exceeds 0.60, equivalent to a typical water pressure on the dam face of 510 kPa. These field and remotely sensed findings not only offer valuable insights for early warning procedures in the Karakoram but also suggest that similar approaches might be effectively applied to other mountain environments worldwide where GLOFs pose a hazard.
- Article
(9271 KB) - Full-text XML
-
Supplement
(784 KB) - BibTeX
- EndNote
Globally, glacier shrinkage is a strikingly visible sign of climate change, as is an apparent increase in the number of glacier hazards such as avalanches (Byers et al., 2023; Kääb and Girod, 2023; Li et al., 2024; You and Xu, 2022) and glacial lake outburst floods (GLOFs) (Bazai et al., 2021; Bhambri et al., 2019; Emmer, 2017; Zheng et al., 2021). However, within high-mountain Asia (HMA), particularly the Karakoram, Kunlun Shan, and eastern Pamir, the glaciers have gained mass since 1970 (Berthier and Brun, 2019; Gardelle et al., 2012; Kääb et al., 2015; Minora et al., 2013; Yao et al., 2012). This positive response to climate change may now be over (Jackson et al., 2023), with many glaciers more recently displaying stability (Ali et al., 2021) or retreat (Singh et al., 2023). Nonetheless, the mass gain has influenced glacier dynamic behaviors, with the Karakoram glaciers thickening, increasing glacier surges (Bazai et al., 2021, 2022; Mu et al., 2024) and advancing glacier termini throughout the region (Bhambri et al., 2013; Bolch et al., 2017). This behavior contrasts that found in neighboring regions with more sustained negative glacier mass budgets, such as the Himalaya, Hindu Kush, and Tibet (Bazai et al., 2021; Bolch et al., 2011; Frey et al., 2014). In the latter areas, glaciers continue to shrink, thin, and reduce in volume, showing no significant glacier advance (Dehecq et al., 2019; Farinotti et al., 2020; Yao et al., 2012). As a result, the increase in moraine lake formation has increased the number of GLOFs in the glacier-retreating regions (Nie et al., 2017). However, in regions where ice mass has increased, glacier advance has prompted the rapid formation of ice-dammed lakes accompanied by sudden releases of meltwater originating from these lakes (Zhang et. al., 1990; Hewitt, 1982, 1998; Hewitt and Liu, 2010; Singh et al., 2023). Although the number and size of GLOFs may decrease with progressive deglaciation (Veh et al., 2023), ice-dammed lake floods currently represent the dominant hazard in cryospheric regions (Veh et al., 2022), comprising 70 % of GLOFs through recorded history (Carrivick and Tweed, 2016). In contrast, moraine-dammed lakes contribute only 9 % (with the remaining 16 %, 3 %, and 2 % associated with unknown dam types, volcanic activity, and bedrock failure, respectively) (Carrivick and Tweed, 2016). Specific details of the GLOF hazard for HMA have been compiled by Shrestha et al. (2023).
Herein, the focus is upon ice-dammed lakes. The mechanisms and frequency of dam-related GLOFs remain poorly understood, hindering accurate prediction (Bazai et al., 2021; Cook et al., 2016; Harrison et al., 2018; Richardson and Reynolds, 2000). Recent studies have investigated changes in frequency due to climate change (Rick et al., 2023; Veh et al., 2023), and there are regional assessments of flood volume and hazards (Rick et al., 2023). Despite these efforts, understanding the drainage and predicting flood events from ice-dammed lakes remain challenging. Nonetheless, anticipating the risks associated with these events is crucial due to their potential to have devastating impacts on human lives and livelihoods, ecosystems, infrastructure (e.g., roads, bridges, hydropower systems), and river channel stability and to have effects on agriculture and fisheries (Carrivick and Tweed, 2016; Cook et al., 2016; Emmer, 2017; Clague et al., 2000; Neupane et al., 2019; Zhang et al., 2022). GLOFs have been recorded up to 500 km from ice-dammed lakes (Hewitt and Liu, 2010), resulting in hundreds of human fatalities and the other impacts noted above (Carrivick and Tweed, 2016; Cui et al., 2014, 2015; Kreutzmann, 1994; Mason, 1929; Stuart-Smith et al., 2021; Zhang et al., 1990; Zheng et al., 2021).
Whilst progress has been made in understanding the breaching mechanisms of moraine lake outburst floods, triggered by ice or debris falls, strong earthquake shaking, internal piping, or overtopping waves that exceed the shear resistance of the dam (Emmer and Vilímek, 2013; Richardson and Reynolds, 2000), understanding the mechanisms of ice-dammed lake outburst floods remains a challenge (Werder et al., 2010), making prediction using numerical modeling currently impossible. Therefore, there is an urgent need for simplified approaches to GLOF prediction to mitigate downstream impacts.
Despite the uncertainty related to the details of GLOF initiation, sudden glacier advances during surge cycles have a prominent role in the formation of ice-dammed lakes by creating an ice barrier in the valleys, particularly in narrow valley floor sections and at confluences (Bazai et al., 2021; Bhambri et al., 2019), that dams rivers (Singh et al., 2023). Glacier surges have resulted in the formation of ice-dammed lakes in the Swiss Alps (Haeberli, 1983), northern Norway (Xu et al., 2015), Argentinian Patagonia (Vandekerkhove, 2021), Alaska (Trabant et al., 2003), Karakoram, and the Pamir (Bazai et al., 2021; Hewitt and Liu, 2010) and Tian Shan regions (Ng et al., 2007; Shangguan et al., 2017). Recent studies have revealed that the draining processes of ice-dammed lakes potentially involve one or more mechanisms: subglacial breaching, overspill, rapid ice mass instability, and slow deformation of subglacial cavities (Björnsson, 2003; Haemmig et al., 2014; Round et al., 2017). Several attempts have been made to explore the drainage behavior of ice-dammed lake outburst floods (Hewitt and Liu, 2010). However, due to the remoteness, danger, and inhospitable terrain where such lakes can be found, real-time data are few, and significant gaps remain in our knowledge of these processes.
In the Karakoram, ice-dammed lakes are found in five major valleys, three of which are densely populated and highly vulnerable to unexpected GLOFs. Recent advances in understanding have been made (Bazai et al., 2021) concerning the formation of episodic ice-dammed lakes, which, due to ice mass transfer variations, are linked to changes in the glacier surface velocity and ice thickness (Singh et al., 2023) and fluctuations in the crevasse density during the surge cycle (Rea and Evans, 2011; Sharp, 1985). Consequently, herein, we explore two main hypotheses: (1) that lake volume is related to glacier velocity and (2) that there is a critical lake depth associated with ensuing GLOFs (Thoraninsson, 1939). As lake volume can dictate the characteristics of a GLOF, a third secondary hypothesis was addressed: (3) that ice-dammed lakes can exhibit geometries similar to regular geometric shapes such that, in the absence of detailed lake volume data, lake volumes might be estimated from geometric consideration. Despite advancements in knowledge globally, the techniques for measuring and estimating both the volume of the lake before an outburst and the critical depth (for GLOF release), as well as for the timely prediction of GLOFs, remain largely unexplored or unidentified (Round et al., 2017; Shangguan et al., 2016; Steiner et al., 2018). Very few ice-dammed lake volume data are available. These ice-dammed lake volumes were measured while the lake basin was either empty (after a GLOF event) or partially filled and thus shallow (Round et al., 2017; Shangguan et al., 2016; Steiner et al., 2018). Given that their potential full volumes are unknown, the downstream threat from such lakes remains high. To measure the flood volume and flood magnitude for a deep and potentially full lake, the lake volume measurement is recognized as a critical variable that needs to be accurately calculated or at least well-estimated (Bazai et al., 2021, 2022). An accurate estimate of lake volume will also help in exploring the GLOF timing, triggering depth of the lake, and frequency of ice-dammed lake outburst floods in relation to surge cycles. Timing information can be approximated by correlating glacier velocities and GLOF occurrences (Bazai et al., 2021, 2022), which should assist in timely hazard assessment. Herein, the primary objective of this study is to enhance predictive capabilities regarding GLOF event timing by refining empirical lake volume estimation and identifying critical depths for future hazard and risk reduction. We seek to achieve the objective within a framework of adjustment of lake volume to glacier surge speed, which has implications for changes in the depth of lakes relative to the heights of the ice barriers that impound the lakes.
The Karakoram Range in HMA is known for its complex geology, climatic variability, and denudation processes, including debris flows, mudflows, landslides, rockfalls, avalanches, and GLOFs. As was noted in the preceding section, changes in glacier dynamics, increasing glacier surges, and a trend of increases in GLOF-related disasters characterize this region. These hazards are responsible for substantial economic losses, including the destruction of residences, infrastructure such as roads and bridges, and agricultural areas, as well as blockages of transportation routes like the Karakoram Highway and other expressways (Shrestha et al., 2023).
Glacier surges in the region have been recorded since the 15th century (Bazai et al., 2021). Since the application of remote sensing to the monitoring of the glaciers from 1970 to 2020, an increasing occurrence of glacier surges has been recorded since the 1990s, with some glacier surges being linked to the formation of ice-dammed lakes and subsequent GLOFs. Some lakes persist only seasonally, forming in the winter when temperatures are very low and draining slowly in the spring or summer. Other lakes are more persistent (Bhambri et al., 2019; Hewitt and Liu, 2010) and have the potential for catastrophic outbursts. The most frequent glacier surges and formation of lakes leading to outburst floods in the Karakoram region occur for the Khurdopin, Kyagar, and Shishper glaciers. For example, Round et al. (2017) concluded that surges were the main factor controlling the formation of ice-dammed lakes associated with the Kyagar Glacier, with the volumes of the lakes reaching a maximum 3 years after the surge period (Li et al., 2023). Similarly, Bazai et al. (2022) concluded that surge velocities have a significant effect on lake formation related to the Khurdopin Glacier.
Although the foreland of the Kyagar Glacier, situated in the Shaksgam Valley, is uninhabited, GLOFs have caused damage and losses further downstream. Conversely, GLOFs from the Khurdopin and Shishper glaciers, located in the densely populated Hunza area, have resulted in casualties and substantial economic losses. Consequently, these glaciers and their lakes are selected for study. The focus of the broader investigation is to obtain the data necessary to understand the complex behavior of the glaciers and their drainage systems with a view to anticipating when the occurrence of GLOFs is imminent. Therefore, there is an urgent need to identify triggering factors for GLOFs to provide downstream warnings in a timely fashion. A better understanding of the complex process behaviors should eventually lead to improved prediction of such events, not only within the Karakoram but also worldwide.
3.1 Remote sensing data
The identification and mapping of the Khurdopin, Kyagar, and Shishper ice-dammed lakes were accomplished using open and commercial satellite imagery sources from 1970 to 2022. The datasets include 590 images from Landsat 2–Landsat 5 and Landsat 7–Landsat 9 and 45 images from Sentinel-2, downloaded from the United States Geological Survey (USGS) website (http://earthexplorer.usgs.gov/, last access: 12 December 2023) (Table S1 in the Supplement). The commercial high-resolution images consisted of 35 images from Gaofen-1 (GF-1) and Gaofen-2 (GF-2), 11 images from SPOT 6 and SPOT 7, and 5 images from CRESDA (https://www.cresda.com/zgzywxyyzxeng/cooperation/odatad/list/odatad_1.html, last access: 20 July 2022; https://earth.esa.int/eogateway, last access: 23 July 2022; and https://www.planet.com/products/planet-imagery, last access: 10 August 2022, respectively). The following DEM datasets have been used to estimate lake volume, depth, and dam height: the Advanced Spaceborne Thermal Emission and Reflection Radiometer (ASTER) and the Phased Array type L-band Synthetic Aperture Radar (PALSAR) DEM data scenes from the National Aeronautics and Space Administration (NASA) Earthdata website (https://search.earthdata.nasa.gov/, last access: 10 December 2022) and KH-9 and Shuttle Radar Topography Mission (SRTM) data downloaded from http://earthexplorer.usgs.gov/ (last access: 12 December 2022) (Table S2 in the Supplement). Field surveys were conducted of the Shishper Glacier lakes in 2019, 2021, and 2022 and of the Khurdopin Glacier lakes in 2017 and 2018 using handheld GPS devices and uncrewed aerial vehicles (UAVs) (see Sect. 3.2) to determine annual lake extents, lake depths, glacier altitudes and thickness, terminus positions, and glacier surface displacements. The purpose of the field campaigns was to obtain (i) data on processes that could not be derived from remote sensing and (ii) field data to calibrate and validate remote-sensing-derived data. The glacier outlines were obtained from the Randolph Glacier Inventory (RGI 6.0) (RGI Consortium, 2017) and modified according to surge movements with time (https://www.planet.com/products/planet-imagery/, last access: 10 October 2022).
3.2 Glacier lake surface area mapping and glacier surface velocity
Satellite imagery had a spatial resolution of 0.8 to 30 m (Table S1). High-resolution imagery is used with the aim of obtaining accurate lake surface levels. The images were selected based on the visibility of the glacier surface and lake areas, and overall, 23 ice-dammed lakes from eight surge events were identified related to the Khurdopin, Kyagar, and Shishper glaciers (Table 1, Fig. 1a). The presence of lakes was determined based on the normalized difference water index (NDWI) (McFeeters, 1996), and the outlines of all 23 lakes were digitized manually using Landsat false-color composites (near-infrared, red, and green bands) to distinguish waterbodies from other objects (Huggel et al., 2002). The extents of six Shishper and Khurdopin lakes that occurred after 2017 were obtained in the field using GPS (G639; accuracy: single, 1–3 m; Satellite Based Augmentation System: 0.6 m) survey points along the lake shorelines (Fig. 2a–d), as well as UAV-generated digital surface models (DSMs). Alternatively, high-resolution satellite imagery from Planet (3 m), GF-1 and GF-2 (0.8 and 4 m resolution, respectively), and SPOT 6 and SPOT 7 (1.5 m) was used to extract the lake boundaries. The coupled lake extent and outlines help reduce the uncertainty in the lake extent obtained for Landsat 2–Landsat 5 images. The above-described method was used to extract the extent of the Khurdopin and Kyagar glacial lakes previously reported (Bazai et al., 2021, 2022), the data of which are incorporated into the current analysis.
Table 1GLOF and surge date and lake volume measurement since 1970 obtained using remote sensing techniques. The average surge velocity and volume related to each of the 23 GLOFs from eight surge cycles are presented; other detail is in Table S3 in the Supplement.
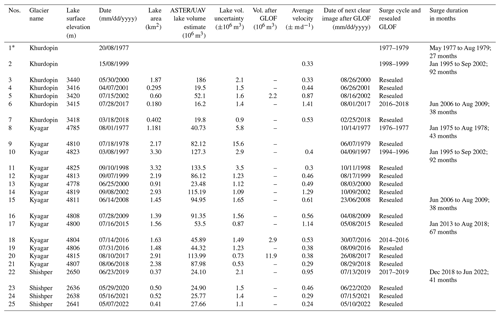
* Only the date of the GLOF is available; other details are not accessible.
The Khurdopin, Kyagar, and Shishper glaciers are surge-type glaciers (Copland et al., 2011; Hewitt, 1998). Since 1972, eight surge events have occurred in total at Khurdopin (three surges), Kyagar (three surges), and Shishper (two surges) (Table 1) at an interval of 17–20 years for each glacier. The Landsat 2–Landsat 4 images from 1970 to 1990 have errors in the selected glacier area. Therefore, the initial surges for Khurdopin and Kyagar between 1970 and 1989 were not considered when estimating the annual velocity. Orthorectified Landsat scenes from the Thematic Mapper (TM) and OLI-2 and Sentinel-2 were used to estimate the yearly and event-based velocities of all three glaciers from 1989 to 2022 to obtain information about the surge events and glacier front changes. Within this period, cloud-free images were chosen each year, although some satellite images were absent. Glacier velocities were recorded as annual averages, although daily measurements of glacier velocity were also determined to assess any effect on lake volume given the possible velocity sensitivity to the triggering time of GLOFs.
The surface velocities were extracted along the central line of the Khurdopin, Shishper, and Kyagar glaciers, highlighting the quiescent and surge phases obtained from published data (Bazai et al., 2021, 2022) using the image-to-image correlation, open-source software COSI-Corr (Leprince et al., 2012, 2007). The software effectively assesses the glacier surface velocity (Leprince et al., 2012; Steiner et al., 2018). Utilizing a displacement calculation, this technique was used to co-register and correlate surface features (Bazai et al., 2021; Steiner et al., 2018). The surface velocity and overall movement during the surge were measured by observing changes in the GPS-registered glacier front positions every 3 months from March 2019, and these changes were measured for 3 years for the Shishper Glacier in the field as well as for the Khurdopin Glacier from June 2017 to July 2019. When coupled with COSI-Corr-measured velocities, these latter procedures gave accurate results. The velocity estimation procedure generally yields an accuracy of of a pixel (Sattar et al., 2019). Velocity root-mean-square errors (RMSEs) were assessed to justify image processing accuracy. Examples of output are given in Fig. 1b–d.
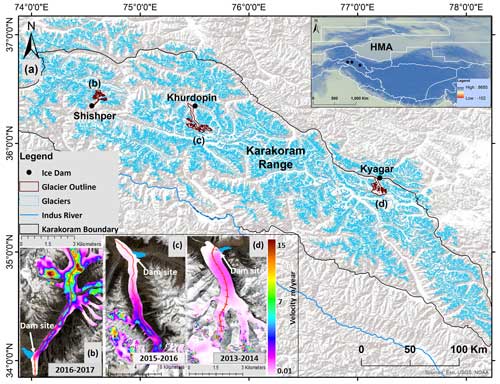
Figure 1Overview of the study site in the Karakoram (a) and the high-mountain Asia (HMA) region; panels (b)–(d) present the extent of each glacier at a given time when surge speed has led to ice-dammed lake formation. The associated ice flow velocities are indicated. The background of panels (b)–(d) is from © Google Earth images. Publisher's remark: please note that the above figure contains disputed territories.
3.3 Field observation and lake volume measurement
Six lakes were regularly monitored: four from the Shishper Glacier and two from the Khurdopin Glacier. Data for 23 GLOF events from eight surge cycles that occurred during or following the first year of each surge are presented in Table 1, with lakes resealing after each GLOF. The field data for six events from the Khurdopin and Shishper glaciers helped to reduce the uncertainty or validated data for 17 lakes for which data were obtained through remote sensing techniques (as explained in Sect. 3.2).
For the Kyagar Glacier, Li et al. (2023) suspected that the drainage conduit may not have been at the deepest part of the lake basin, and its configuration changed between GLOF events. All the previously recorded lakes from Khurdopin and Shishper were drained via single subglacial conduits with stable inlet positions (i.e., the inlet was seen to be in the same place on each occasion) and varying outlet positions and conduit lengths. Therefore, as closely as possible, we identified the inlet and outlet positions of the drainage conduits. As is shown in the Results section, the inlet position of the conduit in the ice-dammed lake basin was always in the deepest position. The lowest ice dam height also tended to be in the vicinity of the conduit. The conduit inlet positions were geolocated within the empty lake basins using GPS, and the lake depths were calculated for these locations with reference to shoreline elevations (Table 1 and Fig. 2). From the field survey, we noted that the presence of alignments of surface depressions in the glacier indicated the approximate position of curvilinear conduits, from which we estimated the conduit lengths between the inlet and outlet.
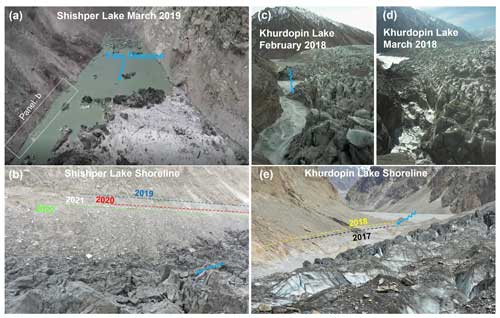
Figure 2Shishper and Khurdopin glacial lake views in the field. (a) Oblique view from a helicopter in March 2019 (image captured during lake monitoring by Gilgit-Baltistan Disaster Management Authority); (b) the Shishper shoreline elevations of four lakes that burst out in the given years; (c, d) successive oblique views of the Khurdopin lake in the field; (e) Khurdopin lake elevations in the given years. Wiggly blue lines are flow directions.
In addition, we used a UAV (DJI Mavic 2 Pro) equipped with a high-resolution camera (4000 pixels × 2250 pixels) to obtain multiple aerial photographs with a minimum of 85 % image overlap (Entwistle and Heritage, 2017, 2019; Tonkin and Midgley, 2016). The UAV flew at a low uniform height (500 m – to reduce the image distortion) to generate high-resolution orthomosaics and DSMs of the glacier lake surfaces, empty lake basins, and glacier termini. In addition to UAV data, we utilized data from KH-9 (1974), ASTER (2000–2019), PALSAR DEM (June 2008), and SRTM (February 2000) for the computation of lake volumes (Table S2). The SRTM DEM without voids serves as the reference dataset, and the vertical uncertainties in the SRTM DEM are reported to be ±10 m (Rodriguez et al., 2006). The corrected DEMs from the Karakoram region are those used by Bazai et al. (2021) and Gardelle et al. (2013).
3.4 Geometry of lake basin
Although, in this study, we have field-derived estimates of lake depth, basin geometry, and lake surface area to calculate lake volumes, in many other applications, only remote sensing data are available for undrained lakes. Consequently, considering that the lake area in satellite images often exhibits a triangular planform (e.g., Figs. 2a and 3a), we explored the possibility of using a geometric shape to approximate the volume of undrained lake basins. Such an approach would be valuable where the depths of lakes are unknown. To this end, we employ NDWI (as in Sect. 3.2) to identify lake outlines through Landsat false-color composites, which use near-infrared, red, and green bands to distinguish waterbodies from other features. We employ standard connected component analysis (Dillencourt et al., 1992) to manually calculate each lake's area, perimeter, and other surface dimensions (as given in Fig. 3b). Initial calculations are pixel-based and are later converted into metric units by multiplying pixel counts by their respective pixel sizes. The pixel size for high-resolution images varied from 0.8 to 3 m. The output was cross-validated with Khurdopin and Shishper glacial lake UAV data that had a pixel size of 0.063 m and with field survey evidence. Trials demonstrated that the known volume of the lakes determined using DEMs of the lake basins once drained (Sect. 3.3) could be approximated if the length of the lake from the upstream inlet to the ice dam face (Z) and the breadth of the lake at the ice dam (C) were known. Given the reported image resolution, uncertainties in the characteristic length measurements (Fig. 3b), measured using 3D GIS interpolation, would translate into uncertainty in lake volume estimates of only 3 % when applying Eqs. (1) and (2) if lake depth were known exactly. For undrained lakes, assuming the depth is the same as the width of the lake at the dam face (Fig. 3b) will likely overestimate lake volume. It might be expected that geometric estimates based on lake surface area alone would be improved if the lake's depth (h) is known at the deepest point close to the dam face. However, in our examples, there is uncertainty in the values of h obtained from DEMs of the drained basins such that the errors in lake depth estimates translate into errors in lake volume estimates of <14 %. Alternatively, where a lake is present, this latter parameter can be obtained by plumbing the depth from a boat.
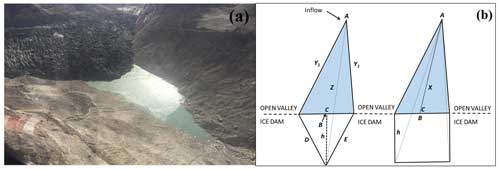
Figure 3(a) Example of Shishper glacier-dammed lake, with the surface exhibiting a roughly triangular 2D shape (see also Fig. 2a); (b) diagram for calculating the volume of the lakes assuming (left) an irregular tetrahedral shape and (right) an irregular pentahedral shape. The blue shading represents the horizontal surface of the lake, and the white area represents the vertical ice wall.
Given the triangular shape of the lake surface areas, the first consideration with regard to lake geometry was whether the valley sides might be regarded as providing a V-shaped lake cross-section or a rectangular cross-section (Fig. 3b); in either case, regular geometric shapes might provide an estimate of the lake volumes. A rectangular cross-section would be closer to the U-shaped valley cross-sections commonly associated with glaciated valleys. Thus, assuming a V-shaped valley, lake volume (V) can be approximated by an irregular tetrahedron (Fig. 3b, left-hand diagram) where the depth (h) is unknown but the distance from A to B (X in Fig. 3b) and the length C are known values. Assuming the lake surface is an isosceles triangle and the vertical face at the dam wall is an equilateral triangle, the volume can be obtained from
where the values for lakeside lengths Y1 and Y2, the main length Z, lakeside length D, and lakeside length E are defined in Fig. 3b and obtained from geometry.
Alternatively, considering a pentahedral, the volume is
These shape assumptions are addressed within the Results section.
4.1 Surge velocity and ice-dammed lake volume
Figure 4a–c present the relationship between the glacier surge velocity (Khurdopin, Kyagar, and Shishper) and 23 GLOFs. The relationship between surge and GLOF was developed using annual average velocity data. The glacier's daily velocity was recorded on the day the GLOF was initiated, as detailed in Table 1. In Fig. 4 panels (a) to (c), it can be seen that the GLOF occurred after the peak of the glacier surge and the resealed lake formed while the surge velocity declined. These responses to slowing of the glacier velocity lasted for 2–4 years after the surge peak. Thus, GLOFs occur toward the end of a surge period or immediately afterward; the detail is presented in Table 1. The relationship between the timing of glacier surges and the timing of GLOFs is shown in Fig. 4a–c, wherein the dates of the GLOFs are given as the month in the year. The three Karakoram glaciers can be used as regional examples of surge behavior controlling GLOF occurrence, as there is a temporal relationship between the occurrence of periods of glacier surging and the occurrence of GLOFs (Fig. 4a–d). This pattern of behavior prompted the hypothesis that glacier thickening and thinning during surging might control the development of ice-dammed lakes (Bazai et al., 2022). Lake volumes would increase when the speed of the ice is low, the ice mass would be conserved or increased, and the fracturing of the ice would be reduced. The corollary pertains to when the ice speed increases, the glacier thins, and the fracturing of the ice mass increases, providing hydraulic drainage conduits (Gao et al., 2024). This sequence of events is shown schematically within Fig. 5. A thinning glacier also minimizes potential lake depth and might increase the likelihood of a GLOF occurring over the top of the ice dam.
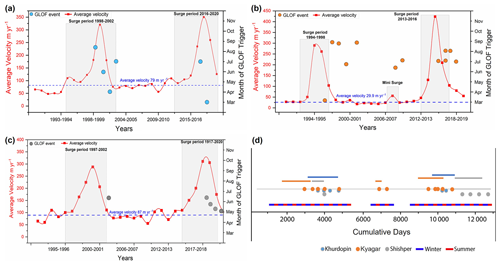
Figure 4Relationship between glacier surges and GLOFs, with average annual glacier velocity during the surge and quiescent phases for three glaciers: (a) Khurdopin, (b) Kyagar, and (c) Shishper. GLOFs for these glaciers occurred between the months of March and November. The combined analysis is presented in (d), illustrating the occurrences of GLOFs (dots) and related periods of glacier surging (bars) as cumulative days since 1 January 1990. Some points are plotted below the timeline to avoid coincident positions. The blue and red lines show the winter (October to April) and summer (May to September) seasons, respectively, with the GLOFs occurring predominantly in the summer months. Within panels (a) to (c), the average surge velocity is given as the red curves, and the average velocity during the study period is given in blue text.
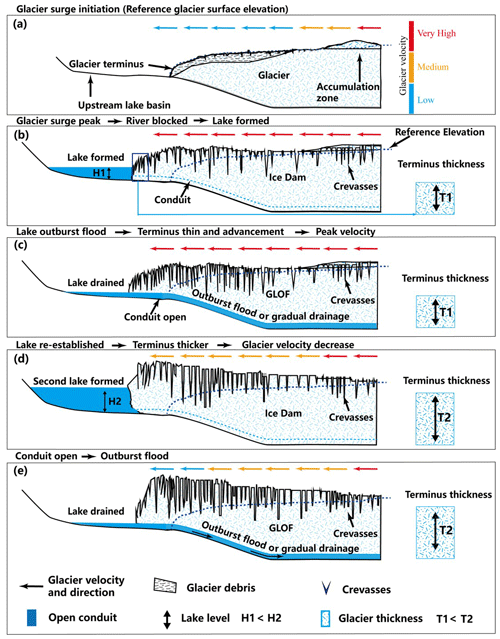
Figure 5The mechanism of glacier surge controls lake formation: (a) prior to the surge, there is no stream blockage in front of a thick terminus; (b) in the first year of the surge, stream blockage occurs, leading to lake formation behind a thinning terminus; (c) during the first year, peak glacier velocity is reached, the lake drains, and the terminus thins; (d) as surge velocity decreases, the lake reforms and the terminus thickens; (e) the second lake can drain as velocity continues to decrease and the terminus thickens.
As a first attempt to relate glacier behavior in a predictive sense to lake formation, we sought to determine the relationship between the resulting lake volume from the prior surge speed. Lake volume should be high when the glacier velocity is low and the ice mass thickens and vice versa; there is some support for this assertion (Fig. 6). Within Fig. 6, considering all the data (excluding the three drained lakes), the broad data spread prevents the fitting of a significant least-squares regression function. Nonetheless, trial curve fitting showed that a negative power function would be the best fit.
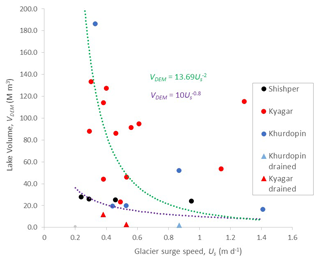
Figure 6Variation in glacial lake volume as a function of the glacier surge speed. Data from three glaciers. Most lakes drained completely, but three drained lakes had residual volumes (triangles). A −0.8 power function (purple curve) defines the lower limit to the data spread, while a −2.0 power least-squares function (green curve) defines the central tendency of the data trend (see text for explanation).
If the volume of a lake decreases as the glacier surge speed increases, as a negative power function would imply, both the lake depth and the surface area decrease; then, from an analogy with a pentahedron, the volume of a pentahedron reduces as the square of the characteristic length (Eq. 2), here the water depth. Consequently, assuming the pentahedral analogy applies, a least-squares trend line fitting procedure was used to define the constant α in the following function: fitted to all the data excluding the drained volumes. This equation, with α=13.6, is shown in Fig. 6 and visually is a good fit through data for a range of low values of Us when lake depths will be greatest. Given the data dispersion and the small sample number, there are no statistical outliers (defined objectively; Carling et al., 2022).
An eye-fitted power function has been added to Fig. 6 to tentatively define the lower limit of the data spread. These results, although clearly not definitive, indicate that there probably is a relationship between the volume of the lake and the control of the lake water level exerted by the surge speed. Therefore, surge speed should exert some control on lake depth, lake volume, and potential GLOF volumes.
Given the scatter in the data within Fig. 6, additional data would be required to determine if the relationship between surge speed and lake volume does follow a negative power trend, as we have suggested.
4.2 Tetrahedron assumption for lake volume
Using Eq. (1) and assuming the ice dam face was an equilateral triangle, only the values X and C are required such that the volume of the “tetrahedron” lakes is around 10 times greater than the volume of the lakes determined using the DEMs (Fig. 7a). This result indicates that the actual depth of the lake (h) must be much less than that value associated with an equilateral triangle of side length C (Fig. 3b, left-hand side). Nevertheless, this procedure provides a means to estimate lake volume from plan-view data alone.
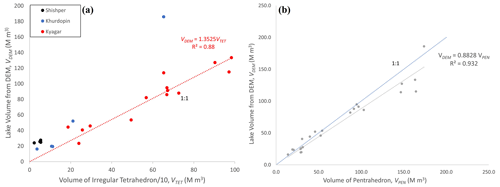
Figure 7(a) Relationship between the volumes of irregular tetrahedrons 10, derived from Eq. (1), and the volumes of the lakes determined using DEMs. (b) The relationship between the volumes of irregular pentahedrons and the volumes of the lakes determined using DEMs.
In contrast to the assumption of an equilateral triangle at the dam face, improved lake volume estimates were obtained considering the measured DEM-derived values of h along with the values of X and C. Once again, assuming an irregular tetrahedron as in Fig. 3b, the analysis demonstrated that the tetrahedral lake volume was roughly half that of the DEM volume (not illustrated). This latter result suggests that treating the valley cross-section as U-shaped (roughly quadrilateral) rather than V-shaped means that doubling the area of the triangular dam face section to form a quadrilateral should provide lake volume estimates, with the shape defined as an irregular pentahedron (square-based pyramid) (Fig. 3b, right-hand side), closer to the DEM-derived volume estimates.
4.3 Pentahedron assumption for lake volume
The pentahedral volume estimates (Eq. 2), as shown in Fig. 7b, are preferable to those values shown in Fig. 7a. They result in a nearly 1:1 relationship between VPEN and VDEM but require knowledge of the parameter depth h, as well as X and C. If it were assumed that a rectangular base of the pentahedron provides an exact match for the DEM volume, the correlation coefficient value would be unity. Thus, the coefficient of 0.88 reflects the deviation of the cross-sectional shape of the lake at the dam face from a rectangle. Note that the relationships between both determinations of lake volume (Fig. 7a and b) progressively deviate from a 1:1 relationship as lake volume increases. This trend might indicate that larger lakes are less well defined as tetrahedrons or pentahedrons as the volumes increase.
When assuming a tetrahedral shape for a lake, the lake volume can be estimated from remote sensing images alone as only the length of the lake (X) and the breadth of the lake (C) at the ice dam are needed to estimate the lake volume. When assuming a pentahedral shape, the depth (h) of the lake at the ice dam is required as well. Although, subsequent to GLOF drainage, h can be measured from a DEM or field survey, for the purposes of mitigation, a warning prior to a GLOF occurring is preferable.
4.4 Anticipating the timing of GLOF events
The timing of a GLOF remains difficult to determine, but for tunnel drainage, the main driver is the critical depth (Thorarinsson, 1969). The critical depth is important as it is the depth that exerts sufficient pressure at the ice dam wall to induce completed connectivity within the subglacial GLOF drainage conduit (Gao et al., 2024; Yasuda and Furuya, 2013). For the cases of Shishper, Khurdopin, and Kyagar, the glacial lake depths (h) have been normalized by dividing them by the minimum value of each ice dam height to give values (n′) of normalized lake depths that range between 0 for a fully drained lake and a hypothetic value of 1 if the lake level reaches the height of the ice barrier. At the approximate time of GLOF occurrence, the resulting values of n′ range between 0.32 and 0.95 (Fig. 8a–c). Most GLOFs occur for a range of n′ values between 0.61 and 0.95 (Fig. 8d). Thus, can be regarded as a warning level value with the potential for a GLOF occurring imminently increasing as n′ approaches unity.
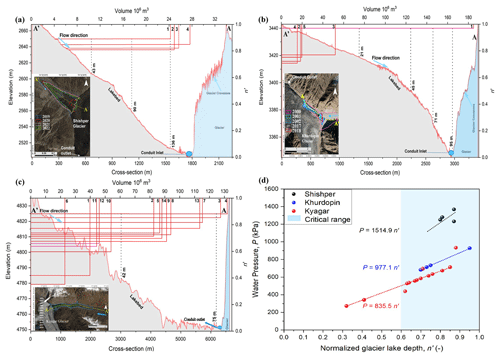
Figure 8The relationship between lake volume and elevation and the critical normalized lake depths for GLOFs: (a) Shishper, (b) Khurdopin, and (c) Kyagar. Red lines connect the relevant elevation and volume in each instance. (d) Water pressure at dam face as a function of n′. The cross-sections are denoted by A′ and A. The serial number and date of each lake flood event are shown in Table 1. The straight solid red lines relate specific lake elevations to volumes. A UAV photograph captured in the field was used for panel (a), the images captured by GF-2 were used for panel (b), and Landsat 8 OLI was used for panel (c).
As the water pressure (P) at the dam face increases linearly with water depth in each lake, any variation in the pressure with n′ that deviates from the linear trend reflects changes in the height of the ice dam (Fig. 8d). Thus, for example, the values of pressure for the Shishper lakes around an n′ value of 0.8 reflect greater overall deeper lakes and higher ice dam heights in contrast to the Kyagar lakes for similar values of n′. The two values of low pressure for are associated with relatively low ice dams and consequently reflect presumed low structural integrity within the ice mass, allowing ready conduit development. In the present examples, low values of n′ (<0.6) probably are associated with shallow lakes of low hazard potential. Overall, the Kyagar data (Fig. 8d) indicate that a minimum water pressure of around 510 kPa should be regarded as a threshold for general concern for GLOF occurrence in the region. However, consideration should be given to local conditions when applying the findings of this study to other locations around the globe.
Although predictive models related to ice-dammed lake development and subsequent GLOF hazard would best be based on modeling the physics of the systems, the controlling parameters are numerous and complex. For example, the mechanisms of glacial sliding, overburden pressure, and tensile and driving stresses require consideration, as do flexure and ice fracture mechanics, thermal erosion, and water pressure, amongst other controls (Carrivick et al., 2020), along with climatic influences (Ng et al., 2007; Richardson and Reynolds, 2000). Few of these controls are understood well, and, importantly, even where there is an adequate theory, the field data required to inform modeling are absent for specific potential GLOF locations. In this study, the glacier and lake interactions and their empirical relationships have been explored, and their effect on lake volume and draining processes has been examined. Understanding glacier surges, lake formation, and the interactions between lakes and glaciers is crucial for advancing knowledge and developing empirical or numerical GLOF models in mountainous regions (Carrivick et al., 2020; Quincey and Luckman, 2014). Glacier surge speed is routinely determined using remote sensing imagery (Paul, 2015), as is lake surface area (Quincey and Luckman, 2014). Thus, remote sensing provides a means to develop graphs similar to the graph of Fig. 6 for specific locations around the globe where ice-dammed lakes form due to glacier surging. Although the data within Fig. 6 are scattered, a negative relationship between surge velocity and lake volume is implied. Specifically, data points scatter around a median trend according to a theoretical −2 power function (Fig. 6). Clearly, more data points within Fig. 6 would be desirable so that the relationship (if any) between ice surge velocity and lake volume might be better defined.
There is an urgent need for simpler methods to predict the probable triggering water levels that lead to GLOFs and the likely volume of the ice-dammed lakes that translate into GLOF hydrographs. Given that requirement, it is acknowledged that the relationships proposed herein are empirical and apply specifically to glaciers within the Karakoram region. However, there is no reason to suppose that similar functions based on geometric considerations (Zhang et al., 2023) and a critical depth (Zhao et al., 2017) might not be developed elsewhere, including for moraine-dammed lakes (Yao et al., 2010). Below, the approach is explored for glacial ice-dammed lakes worldwide.
Despite the absence of long-term records, those that are available indicate that glacial ice-dammed lakes worldwide exhibit consistent behavior in terms of lake formation, filling, and volume gain in response to low glacier velocity (Bazai et al., 2021). Additionally, specific water pressure and critical normalized lake depth values for initiating outburst floods are evident (Fig. 8).
Building upon the information presented in Fig. 8 for the Karakoram, Fig. 9, based on 50 GLOF events from 10 glacial ice-dammed lakes, offers a depiction of the conditions under which glacier lake volume measurements are estimated with high accuracy. Considering lakes other than those within the Karakoram, the lake elevation (Fig. 9a) at the time of a GLOF was available for five lakes that have triggered a GLOF more than twice (Chilinji, Medvezhiy, Merzbacher, Russell, Rio Colonia), enabling the calculation of the normalized lake depths (Fig. 9b). The data points in Fig. 9c represent 27 lakes within the Pamir, Tian Shan, Greenland, and northern Patagonia for which lake volume data are available in the literature. These latter data were used to establish a relationship between the lake volume estimated using Eq. (2) and the reported volumes (R2=0.972; Fig. 9c).
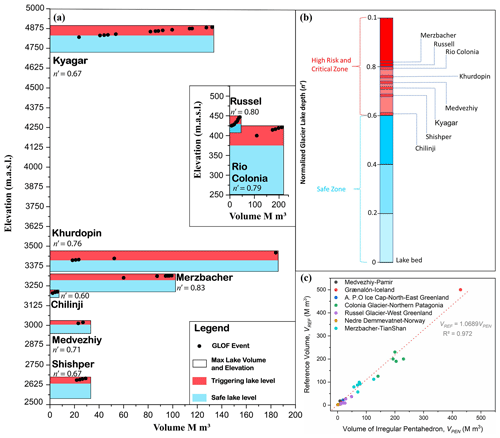
Figure 9(a) The maximum volume of ice-dammed glacier lakes that results in GLOFs related to water surface elevation. In this representation, the red bands are defined by the range of n′ values recorded just before each GLOF event was initiated. The blue bands represent lakes with volumes below the normalized lake depth of 0.60. (b) Normalized critical lake depths and the high-risk and critical zones for GLOFs, with an inferred gradation for risk within the safe zone, shown by shades of blue. (c) The relationship between the reference volume (VREF) and the measured volume (VPEN) obtained through a geometric approach. The locations of these glaciers are presented in Fig. S1 in the Supplement.
Figure 9a and b serve as key components and summaries for this discussion, identifying the lake volumes, elevations, and critical normalized depth values for GLOF outbursts. Critical normalized lake depth values (n′) exceed 0.60 in all cases of GLOFs (Fig. 9b). From this result, we infer that a safe lake level can be defined as <0.60, while the trigger level is ≥0.60. Values of were associated with slow, non-catastrophic lake drainage. Therefore, in the case of future ice-dammed lakes, values of critical depth (n′) exceeding 0.60 should be a cause for concern, and 0.60 would serve as a warning level.
Estimating the volume of an undrained ice-dammed lake from a field survey is dangerous due to floating ice, rugged terrain, and sudden drawdowns. The utilization of DEM measurements for lake volume estimation may also introduce high uncertainties or errors due to the difficulty in defining the lake depths (Carrivick et al., 2020; Emmer, 2018). However, for rapid response or mitigation policy purposes, the empirical model (Eq. 1 or Eq. 2) used in the current study proves to be quite efficient when applied to estimate the lake volume before a GLOF, not least because the errors in measurements from both satellite and UAV images are now quite small, as noted within the “Data and methods” section.
Although GLOFs cannot be predicted from this approach, the likely volume of water that might be released catastrophically can be determined. For sites that are deemed to pose a threat to human life and infrastructure, once the lake volume is better constrained, through either DEM analysis or geometric considerations, the value of n′ for any specific lake provides a ready indicator of the probability of an imminent GLOF. In contrast to the lower trend for water pressures associated with the Kyagar lakes, the higher water pressures required to cause the Khurdopin and Shishper lakes to empty may reflect greater structural integrity, possibly related to a greater downstream extent of the glacier dams. These structural issues can be examined in the future. Still, at this stage, if n′ exceeds 0.6, an initial general warning could be issued to communities downstream of the ice dam. In principle, the estimated volume of a potential GLOF can then be routed downstream using standard hydrodynamic flood routing procedures to determine the timing, depth, and extent of flooding at locations where inundation is forecast. Thus, the severity of the likely impact on humankind can be determined, and specific warning times can be derived from the modeled rate of travel of the GLOFs. These results represent a step forward from the observations made by Carrivick et al. (2020), who proposed the exploration of the interaction between lake water and glaciers to understand the lake formation process and identify lake depth, level, and volume. Based on this understanding, empirical models can be generated to predict GLOFs in a timely manner.
Despite an escalating hazard from glacier lake outburst floods (GLOFs), understanding these hazards remains limited. It is imperative to determine the causes of these hazards, make timely predictions, and formulate new mitigation policies to minimize losses. Herein, it has been shown tentatively that glacier surge speed may correlate negatively with ice-dammed lake volumes such that glacier dynamics control lake volumes. Consequently, in those cases where glacier surge speed is monitored, trends in surge speed can provide a timely indication that a lake might form, allowing the risk of a potential GLOF to be considered and mitigation measures to be reviewed. Identifying the critical depths, lake volumes, and pressures of ice-dammed lakes worldwide associated with GLOFs has indicated that GLOFs may be imminent when the normalized depth (n′) for lakes exceeds a critical value () with a typical water pressure on the dam face exceeding 510 kPa. Identifying a critical depth that might lead to a GLOF is relatively straightforward and, thus, is a useful measure that provides a timely warning for downstream communities. Comparing published surveyed lake volumes with geometric volume estimates for 23 GLOF events from the Karakoram and 27 events from around the world, linear least-squares regression (R2=0.972) demonstrated that geometric estimates can be robust in the absence of detailed field or remote sensing surveys. Such an approach to determining lake volumes is useful on two accounts. Firstly, the approach can provide a quick estimation of lake volume. Then, if the lake volume is considered to be of concern, more detailed survey work can be commissioned to obtain a more accurate estimate of the volume. Secondly, in those situations where sufficient resources may not be available to conduct detailed volumetric surveys, the geometric approach provides a ready tool to obtain a reasonable volume estimate. Taken together, these findings suggest that future exploration should concentrate on specific volume and depth parameters to determine critical thresholds associated with normalized depth and the associated lake volume for future predictive purposes. In this respect, it should be noted that the water pressure recommended herein as potentially of concern (510 kPa) pertains to lake depths of ca. 50 m, whereas deeper lakes in other regions might drain at different values of pressure.
Data and/or further information regarding the data used can be obtained from the corresponding authors upon request.
Supplementary information for this paper is available in the Supplement and at https://doi.org/10.1016/j.earscirev.2020.103432 (Bazai et al., 2021) and https://doi.org/10.1016/j.gloplacha.2021.103710 (Bazai et al., 2022). The supplement related to this article is available online at: https://doi.org/10.5194/tc-18-5921-2024-supplement.
NAB conceptualized and designed the study and methodology, generated and compiled field data, processed data visualization, and drafted the manuscript. PAC contributed to conceptualization, methodology, interpretation, discussion, review, and editing. PC supervised funding acquisition and commented on the paper, and WH contributed to compiling the field data. NAB, ZG, LD, and JH contributed to remote sensing data analysis.
The contact author has declared that none of the authors has any competing interests.
Publisher's note: Copernicus Publications remains neutral with regard to jurisdictional claims made in the text, published maps, institutional affiliations, or any other geographical representation in this paper. While Copernicus Publications makes every effort to include appropriate place names, the final responsibility lies with the authors.
Special thanks go to the monitoring team of the Gilgit-Baltistan Disaster Management Authority (GBDMA), Quaid-i-Azam University, and Karakoram International University for their support, data sharing, and technical assistance. We are also grateful to Iqtidar Hussain for sharing his expertise and to the Special Research Assistant Program of the Chinese Academy of Sciences. Additionally, we deeply thank the editor and the three reviewers for their valuable and constructive comments.
This study was supported by the National Natural Science Foundation of China (grant no. 42350410445) and the Second Tibetan Plateau Scientific Expedition and Research Program (STEP) (grant no. 2019QZKK0906).
This paper was edited by Tobias Sauter and reviewed by Wilhelm Furian and two anonymous referees.
Ali, S., Khan, G., Hassan, W., Qureshi, J. A., and Bano, I.: Assessment of glacier status and its controlling parameters from 1990 to 2018 of Hunza Basin, Western Karakorum, Environ. Sci. Pollut. R., 28, 63178–63190, 2021.
Bazai, N. A., Cui, P., Carling, P. A., Wang, H., Hassan, J., Liu, D., Zhang, G., and Jin, W.: Increasing glacial lake outburst flood hazard in response to surge glaciers in the Karakoram, Earth-Sci. Rev., 212, 103432, https://doi.org/10.1016/j.earscirev.2020.103432, 2021.
Bazai, N. A., Cui, P., Liu, D., Carling, P. A., Wang, H., Zhang, G., Li, Y., and Hassan, J.: Glacier surging controls glacier lake formation and outburst floods: The example of the Khurdopin Glacier, Karakoram, Global Planet. Change, 208, 103710, https://doi.org/10.1016/j.gloplacha.2021.103710, 2022.
Berthier, E. and Brun, F.: Karakoram geodetic glacier mass balances between 2008 and 2016: persistence of the anomaly and influence of a large rock avalanche on Siachen Glacier, J. Glaciol., 65, 494–507, 2019.
Bhambri, R., Bolch, T., Kawishwar, P., Dobhal, D. P., Srivastava, D., and Pratap, B.: Heterogeneity in glacier response in the upper Shyok valley, northeast Karakoram, The Cryosphere, 7, 1385–1398, https://doi.org/10.5194/tc-7-1385-2013, 2013.
Bhambri, R., Hewitt, K., Kawishwar, P., Kumar, A., Verma, A., Tiwari, S., and Misra, A.: Ice-dams, outburst floods, and movement heterogeneity of glaciers, Karakoram, Global Planet. Change, 180, 100–116, 2019.
Björnsson, H.: Subglacial lakes and jökulhlaups in Iceland, Global Planet. Change, 35, 255–271, 2003.
Bolch, T., Pieczonka, T., and Benn, D. I.: Multi-decadal mass loss of glaciers in the Everest area (Nepal Himalaya) derived from stereo imagery, The Cryosphere, 5, 349–358, https://doi.org/10.5194/tc-5-349-2011, 2011.
Bolch, T., Pieczonka, T., Mukherjee, K., and Shea, J.: Brief communication: Glaciers in the Hunza catchment (Karakoram) have been nearly in balance since the 1970s, The Cryosphere, 11, 531–539, https://doi.org/10.5194/tc-11-531-2017, 2017.
Byers, A. C., Somos-Valenzuela, M., Shugar, D. H., McGrath, D., Chand, M. B., and Avtar, R.: Brief communication: An ice-debris avalanche in the Nupchu Valley, Kanchenjunga Conservation Area, eastern Nepal, The Cryosphere, 18, 711–717, https://doi.org/10.5194/tc-18-711-2024, 2024.
Carling, P. A., Jonathan, P., and Su, T.: Fitting limit lines (envelope curves) to spreads of geoenvironmental data, Prog. Phys. Geogr. Earth Environ., 46, 272–290, 2022.
Carrivick, J. L. and Tweed, F. S.: A global assessment of the societal impacts of glacier outburst floods, Global Planet. Change, 144, 1–16, 2016.
Carrivick, J. L., Tweed, F. S., Sutherland, J. L., and Mallalieu, J.: Toward numerical modeling of interactions between ice-marginal proglacial lakes and glaciers, Front. Earth Sci., 8, 577068, https://doi.org/10.3389/feart.2020.577068, 2020.
Clague, J. J. and Evans, S. G: A review of catastrophic drainage of moraine-dammed lakes in British Columbia, Quaternary Sci. Rev., 19, 1763–1783, 2000.
Cook, S. J., Kougkoulos, I., Edwards, L. A., Dortch, J., and Hoffmann, D.: Glacier change and glacial lake outburst flood risk in the Bolivian Andes, The Cryosphere, 10, 2399–2413, https://doi.org/10.5194/tc-10-2399-2016, 2016.
Copland, L., Sylvestre, T., Bishop, M. P., Shroder, J. F., Seong, Y. B., Owen, L. A., Bush, A., and Kamp, U.: Expanded and recently increased glacier surging in the Karakoram, Arct. Antarct. Alp. Res., 43, 503–516, 2011.
Cui, P., Chen, R., Xiang, L., and Su, F.: Risk analysis of mountain hazards in Tibetan plateau under global warming, Progressus Inquisitiones De Mutatione Climatis, 2, 103–109, 2014.
Cui, P., Su, F., Zou, Q., Chen, N., and Zhang, Y.: Risk assessment and disaster reduction strategies for mountainous and meteorological hazards in Tibetan Plateau, Chinese Sci. Bull., 60, 3067–3077, 2015.
Dehecq, A., Gourmelen, N., Gardner, A. S., Brun, F., Goldberg, D., Nienow, P. W., Berthier, E., Vincent, C., Wagnon, P., and Trouvé, E.: Twenty-first century glacier slowdown driven by mass loss in High Mountain Asia, Nat. Geosci., 12, 22–27, 2019.
Dillencourt, M. B., Samet, H., and Tamminen, M.: A general approach to connected-component labeling for arbitrary image representations, J. ACM, 39, 253–280, 1992.
Emmer, A.: Glacier retreat and glacial lake outburst floods (GLOFs), in: Oxford Research Encyclopedia of Natural Hazard Science, Oxford University Press, Oxford, https://doi.org/10.1093/acrefore/9780199389407.013.275, 2017.
Emmer, A.: GLOFs in the WOS: bibliometrics, geographies and global trends of research on glacial lake outburst floods (Web of Science, 1979–2016), Nat. Hazards Earth Syst. Sci., 18, 813–827, https://doi.org/10.5194/nhess-18-813-2018, 2018.
Emmer, A. and Vilímek, V.: Review Article: Lake and breach hazard assessment for moraine-dammed lakes: an example from the Cordillera Blanca (Peru), Nat. Hazards Earth Syst. Sci., 13, 1551–1565, https://doi.org/10.5194/nhess-13-1551-2013, 2013.
Entwistle, N. and Heritage, G.: An evaluation DEM accuracy acquired using a small unmanned aerial vehicle across a riverine environment, Int. J. New Technol. Res., 3, 43–48, 2017.
Entwistle, N. S. and Heritage, G. L.: Small unmanned aerial model accuracy for photogrammetrical fluvial bathymetric survey, J. Appl. Remote Sens., 13, 014523, https://doi.org/10.1117/1.JRS.13.014523, 2019.
Farinotti, D., Immerzeel, W. W., de Kok, R. J., Quincey, D. J., and Dehecq, A.: Manifestations and mechanisms of the Karakoram glacier Anomaly, Nat. Geosci., 13, 8–16, 2020.
Frey, H., Machguth, H., Huss, M., Huggel, C., Bajracharya, S., Bolch, T., Kulkarni, A., Linsbauer, A., Salzmann, N., and Stoffel, M.: Estimating the volume of glaciers in the Himalayan–Karakoram region using different methods, The Cryosphere, 8, 2313–2333, https://doi.org/10.5194/tc-8-2313-2014, 2014.
Gao, Y., Liang, P., Qi, M., Yao, X., Ma, X., Mu, J., and Li, L.: Topography and accumulation rate as controls of asynchronous surging behaviour in the eastern and western branches of the Western Kunlun Glacier, Northwestern Tibetan Plateau, Int. J. Digit. Earth, 17, 2353112, https://doi.org/10.1080/17538947.2024.2353112, 2024.
Gardelle, J., Berthier, E., and Arnaud, Y.: Slight mass gain of Karakoram glaciers in the early twenty-first century, Nat. Geosci., 5, 322–325, 2012.
Gardelle, J., Berthier, E., Arnaud, Y., and Kääb, A.: Region-wide glacier mass balances over the Pamir-Karakoram-Himalaya during 1999–2011, The Cryosphere, 7, 1263–1286, https://doi.org/10.5194/tc-7-1263-2013, 2013.
Haeberli, W.: Frequency and characteristics of glacier floods in the Swiss Alps, Ann. Glaciol., 4, 85–90, 1983.
Haemmig, C., Huss, M., Keusen, H., Hess, J., Wegmüller, U., Ao, Z., and Kulubayi, W.: Hazard assessment of glacial lake outburst floods from Kyagar glacier, Karakoram mountains, China, Ann. Glaciol., 55, 34–44, 2014.
Harrison, S., Kargel, J. S., Huggel, C., Reynolds, J., Shugar, D. H., Betts, R. A., Emmer, A., Glasser, N., Haritashya, U. K., Klimeš, J., Reinhardt, L., Schaub, Y., Wiltshire, A., Regmi, D., and Vilímek, V.: Climate change and the global pattern of moraine-dammed glacial lake outburst floods, The Cryosphere, 12, 1195–1209, https://doi.org/10.5194/tc-12-1195-2018, 2018.
Hewitt, K.: Natural dams and outburst floods of the Karakoram Himalaya, in: Hydrological Aspects of Alpine and High Mountain Areas, edited by: Glen, J. International Hydrological Association. (I.A.H.S.) Publication No. 138, Exeter, UK, 138, 259–269, 1982.
Hewitt, K.: Recent glacier surges in the Karakoram Himalaya, south central Asia, Eos, Transactions, American Geophysical Union, 78, 46, 1998.
Hewitt, K. and Liu, J.: Ice-dammed lakes and outburst floods, Karakoram Himalaya: historical perspectives on emerging threats, Phys. Geogr., 31, 528–551, 2010.
Huggel, C., Kääb, A., Haeberli, W., Teysseire, P., and Paul, F.: Remote sensing based assessment of hazards from glacier lake outbursts: a case study in the Swiss Alps, Can. Geotech. J., 39, 316–330, 2002.
Jackson, M., Azam, M., Baral, P., Benestad, R., and Brun, F.: Consequences of climate change for the cryosphere in the Hindu Kush Himalaya, in: Water, ice, society, and ecosystems in the Hindu Kush Himalaya: An outlook ICIMOD, edited by: Wester, P., Chaudhary, S., Chettri, N., Jackson, M., Maharjan, A., Nepal, S., and Steiner, J. F., International Centre for Integrated Mountain Development (ICIMOD), Kathmandu, Nepal, 17–71, https://doi.org/10.53055/ICIMOD.1030, 2023.
Kääb, A. and Girod, L.: Brief communication: Rapid m3 bed erosion after detachment of the Sedongpu Glacier (Tibet), The Cryosphere, 17, 2533–2541, https://doi.org/10.5194/tc-17-2533-2023, 2023.
Kääb, A., Treichler, D., Nuth, C., and Berthier, E.: Brief Communication: Contending estimates of 2003–2008 glacier mass balance over the Pamir–Karakoram–Himalaya, The Cryosphere, 9, 557–564, https://doi.org/10.5194/tc-9-557-2015, 2015.
Kreutzmann, H.: Habitat conditions and settlement processes in the Hindukush-Karakoram, Petermann. Geogr. Mitt., 138, 337–356, 1994.
Leprince, S., Barbot, S., Ayoub, F., and Avouac, J.-P.: Automatic and precise orthorectification, coregistration, and subpixel correlation of satellite images, application to ground deformation measurements, IEEE T. Geosci. Remote, 45, 1529–1558, 2007.
Leprince, S., Avouac, J.-P., and Ayoub, F.: Ortho-rectification, coregistration, and subpixel correlation of optical satellite and aerial images, Google Patents, US 8,121,433 B2, https://patents.google.com/patent/US8121433B2/en (last access: 23 August 2020), 2012.
Li, G., Lv, M., Quincey, D. J., Taylor, L. S., Li, X., Yan, S., Sun, Y., and Guo, H.: Characterizing the surge behaviour and associated ice-dammed lake evolution of the Kyagar Glacier in the Karakoram, The Cryosphere, 17, 2891–2907, https://doi.org/10.5194/tc-17-2891-2023, 2023.
Li, Y., Cui, Y., Hu, X., Lu, Z., Guo, J., Wang, Y., Wang, H., Wang, S., and Zhou, X.: Glacier retreat in Eastern Himalaya drives catastrophic glacier hazard chain, Geophys. Res. Lett., 51, e2024GL108202, https://doi.org/10.1029/2024GL108202, 2024.
Mason, K.: Indus floods and Shyok glaciers, Himal. J, 1, 10–29, 1929.
McFeeters, S. K.: The use of the Normalized Difference Water Index (NDWI) in the delineation of open water features, Int. J. Remote Sens., 17, 1425–1432, 1996.
Minora, U., Bocchiola, D., D'Agata, C., Maragno, D., Mayer, C., Lambrecht, A., Mosconi, B., Vuillermoz, E., Senese, A., Compostella, C., Smiraglia, C., and Diolaiuti, G.: 2001–2010 glacier changes in the Central Karakoram National Park: a contribution to evaluate the magnitude and rate of the “Karakoram anomaly”, The Cryosphere Discuss., 7, 2891–2941, https://doi.org/10.5194/tcd-7-2891-2013, 2013.
Mu, J., Gao, Y., and Liang, P.: Hydrological control of the surging behaviour of the Ghujerab River Head Glacier, Karakoram (2019–2023): Insights from high-temporal-resolution remote sensing monitoring, J. Hydrol.: Reg. Stud., 53, 101768, https://doi.org/10.1016/j.ejrh.2024.101768, 2024.
Neupane, R., Chen, H., and Cao, C.: Review of moraine dam failure mechanism, Geomat. Nat. Haz. Risk, 10, 1948–1966, 2019.
Ng, F., Liu, S., Mavlyudov, B. and Wang, Y.:: Climatic control on the peak discharge of glacier outburst floods, Geophys. Res. Lett., 34, L21503, https://doi.org/10.1029/2007GL031426, 2007.
Nie, Y., Sheng, Y., Liu, Q., Liu, L., Liu, S., Zhang, Y., and Song, C.: A regional-scale assessment of Himalayan glacial lake changes using satellite observations from 1990 to 2015, Remote Sens. Environ., 189, 1–13, 2017.
Paul, F.: Revealing glacier flow and surge dynamics from animated satellite image sequences: examples from the Karakoram, The Cryosphere, 9, 2201–2214, https://doi.org/10.5194/tc-9-2201-2015, 2015.
Quincey, D. J. and Luckman, A.: Brief Communication: On the magnitude and frequency of Khurdopin glacier surge events, The Cryosphere, 8, 571–574, https://doi.org/10.5194/tc-8-571-2014, 2014.
Rea, B. R. and Evans, D. J. A.: An assessment of surge-induced crevassing and the formation of crevasse squeeze ridges, J. Geophys. Res.-Earth, 116, https://doi.org/10.1029/2011JF001970, 2011.
RGI Consortium: Randolph glacier inventory – a dataset of global glacier outlines: Version 6.0: technical report, global land ice measurements from space, Boulder, Colorado, USA, National Snow and Ice Data Center [data set], https://doi.org/10.7265/4m1f-gd79, 2017.
Richardson, S. D. and Reynolds, J. M.: An overview of glacial hazards in the Himalayas, Quatern. Int., 65, 31–47, 2000.
Rick, B., McGrath, D., McCoy, S., and Armstrong, W.: Unchanged frequency and decreasing magnitude of outbursts from ice-dammed lakes in Alaska, Nat. Commun., 14, 6138, https://doi.org/10.1038/s41467-023-41794-6, 2023.
Rodriguez, E., Morris, C. S., and Belz, J. E.: A global assessment of the SRTM performance, Photogramm. Eng. Rem. S., 72, 249–260, 2006.
Round, V., Leinss, S., Huss, M., Haemmig, C., and Hajnsek, I.: Surge dynamics and lake outbursts of Kyagar Glacier, Karakoram, The Cryosphere, 11, 723–739, https://doi.org/10.5194/tc-11-723-2017, 2017.
Sattar, A., Goswami, A., Kulkarni, A. V., and Das, P.: Glacier-surface velocity derived ice volume and retreat assessment in the Dhauliganga Basin, Central Himalaya – A remote sensing and modeling based approach, Front. Earth Sci., 7, 105, https://doi.org/10.3389/feart.2019.00105, 2019.
Shangguan, D., Liu, S., Ding, Y., Guo, W., Xu, B., Xu, J., and Jiang, Z.: Characterizing the May 2015 Karayaylak Glacier surge in the eastern Pamir Plateau using remote sensing, J. Glaciol., 62, 944–953, 2016.
Shangguan, D., Ding, Y., Liu, S., Xie, Z., Pieczonka, T., Xu, J., and Moldobekov, B.: Quick release of internal water storage in a glacier leads to underestimation of the hazard potential of glacial lake outburst floods from lake merzbacher in central tian shan mountains, Geophys. Res. Lett., 44, 9786–9795, 2017.
Sharp, M.: “Crevasse-fill” ridges – a landform type characteristic of surging glaciers?, Geogr. Ann. A, 67, 213–220, 1985.
Shrestha, F., Steiner, J. F., Shrestha, R., Dhungel, Y., Joshi, S. P., Inglis, S., Ashraf, A., Wali, S., Walizada, K. M., and Zhang, T.: A comprehensive and version-controlled database of glacial lake outburst floods in High Mountain Asia, Earth Syst. Sci. Data, 15, 3941–3961, https://doi.org/10.5194/essd-15-3941-2023, 2023.
Singh, H., Varade, D., de Vries, M. V. W., Adhikari, K., Rawat, M., Awasthi, S., and Rawat, D.: Assessment of potential present and future glacial lake outburst flood hazard in the Hunza valley: A case study of Shisper and Mochowar glacier, Sci. Total Environ., 868, 161717, https://doi.org/10.1016/j.scitotenv.2023.161717, 2023.
Steiner, J. F., Kraaijenbrink, P. D. A., Jiduc, S. G., and Immerzeel, W. W.: Brief communication: The Khurdopin glacier surge revisited – extreme flow velocities and formation of a dammed lake in 2017, The Cryosphere, 12, 95–101, https://doi.org/10.5194/tc-12-95-2018, 2018.
Stuart-Smith, R., Roe, G., Li, S., and Allen, M.: Increased outburst flood hazard from Lake Palcacocha due to human-induced glacier retreat, Nat. Geosci., 14, 85–90, 2021.
Thorarinsson, S.: The ice-dammed lakes of Iceland, with particular reference to their value as indicators of glacier oscillations, Geogr. Ann., 21A, 216–242, 1939.
Thorarinsson, S.: Glacier surges in Iceland, with special reference to the surges of Brúarjökull, Can. J. Earth Sci., 6, 875–882, 1969.
Tonkin, T. N. and Midgley, N. G.: Ground-control networks for image based surface reconstruction: An investigation of optimum survey designs using UAV derived imagery and structure-from-motion photogrammetry, Remote Sensing, 8, 786, https://doi.org/10.3390/rs8090786, 2016.
Trabant, D. C., March, R., and Thomas, D.: Hubbard Glacier, Alaska: Growing and advancing in spite of global climate change and the 1986 and 2002 Russell Lake outburst floods, US Geological Survey, Fact Sheet 001–03, 1–4, https://doi.org/10.3133/fs00103, 2003.
Vandekerkhove, E.: Impact of climate change on the occurrence of late Holocene glacial lake outburst floods in Patagonia: A sediment perspective, Ghent University, http://hdl.handle.net/1854/LU-8702177 (last access: 5 February 2022), 2021.
Veh, G., Lützow, N., Kharlamova, V., Petrakov, D., Hugonnet, R., and Korup, O.: Trends, breaks, and biases in the frequency of reported glacier lake outburst floods, Earths Future, 10, e2021EF002426, https://doi.org/10.1029/2021EF002426, 2022.
Veh, G., Lützow, N., Tamm, J., Luna, L. V., Hugonnet, R., Vogel, K., Geertsema, M., Clague, J. J., and Korup, O.: Less extreme and earlier outbursts of ice-dammed lakes since 1900, Nature, 614, 701–707, 2023.
Werder, M. A., Bauder, A., Funk, M., and Keusen, H.-R.: Hazard assessment investigations in connection with the formation of a lake on the tongue of Unterer Grindelwaldgletscher, Bernese Alps, Switzerland, Nat. Hazards Earth Syst. Sci., 10, 227–237, https://doi.org/10.5194/nhess-10-227-2010, 2010.
Xu, M., Bogen, J., Wang, Z., Bønsnes, T. E., and Gytri, S.: Pro-glacial lake sedimentation from jökulhlaups (GLOF), Blåmannsisen, northern Norway, Earth Surf. Proc. Land., 40, 654–665, 2015.
Yao, T., Thompson, L. G., Mosbrugger, V., Zhang, F., Ma, Y., Luo, T., Xu, B., Yang, X., Joswiak, D. R., and Wang, W.: Third pole environment (TPE), Environ. Dev., 3, 52–64, 2012.
Yao, X., Liu, S., and Wei, J.: Reservoir capacity calculation and variation of Moraine-dammed Lakes in the North Himalayas: a case study of Longbasaba Lake, Acta Geographica Sinica, 65, 1381–1390, 2010.
Yasuda, T. and Furuya, M.: Short-term glacier velocity changes at West Kunlun Shan, Northwest Tibet, detected by synthetic aperture radar data, Remote Sens. Environ., 128, 87–106, 2013.
You, C. and Xu, C.: Himalayan glaciers threatened by frequent wildfires, Nat. Geosci., 15, 956–957, 2022.
Zhang, G., Bolch, T., Yao, T., Rounce, D. R., Chen, W., Veh, G., King, O., Allen, S. K., Wang, M., and Wang, W.: Underestimated mass loss from lake-terminating glaciers in the greater Himalaya, Nat. Geosci., 16, 333–338, 2023.
Zhang, T., Li, D., East, A. E., Walling, D. E., Lane, S., Overeem, I., Beylich, A. A., Koppes, M., and Lu, X.: Warming-driven erosion and sediment transport in cold regions, Nature Reviews Earth & Environment, 3, 832–851, 2022.
Zhang, X. S., Li, N.-J., You, X.-Y., and Wang, W.-X.: The Researches Of Glacier Lake Outburst Floods Of The Yarkant River In Xinjiang, journal Science in China Series B: Chemistry, 33, 1014–1024, https://engine.scichina.com/doi/pdf/4988eae19a964ff491d9eb66e7be660d (last access: 15 April 2023), 1990.
Zhao, T.-Y., Yang, M.-Y., Walling, D. E., Zhang, F.-B., and Zhang, J.-Q.: Using check dam deposits to investigate recent changes in sediment yield in the Loess Plateau, China, Global Planet. Change, 152, 88–98, 2017.
Zheng, G., Allen, S. K., Bao, A., Ballesteros-Cánovas, J. A., Huss, M., Zhang, G., Li, J., Yuan, Y., Jiang, L., and Yu, T.: Increasing risk of glacial lake outburst floods from future Third Pole deglaciation, Nat. Clim. Change, 11, 411–417, 2021.