the Creative Commons Attribution 4.0 License.
the Creative Commons Attribution 4.0 License.
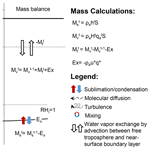
A model framework for atmosphere–snow water vapor exchange and the associated isotope effects at Dome Argus, Antarctica – Part 1: The diurnal changes
Tianming Ma
Zhuang Jiang
Minghu Ding
Pengzhen He
Yuansheng Li
Wenqian Zhang
Ice-core water isotopes contain valuable information on past climate changes. However, such information can be altered by post-depositional processing after snow deposition. Atmosphere–snow water vapor exchange is one such process, but its influence remains poorly constrained. Here we constructed a box model to quantify the atmosphere–snow water vapor exchange fluxes and the associated isotope effects at sites with low snow accumulation rates, where the effects of atmosphere–snow water vapor exchange are suspected to be large. The model reproduced the observed diurnal variations in δ18O, δD, and deuterium excess (d-excess) in water vapor at Dome C, East Antarctica. According to the same model framework, we found that under average summer clear-sky conditions, atmosphere–snow water vapor exchange at Dome A can cause diurnal variations in atmospheric water vapor δ18O and δD of 4.8 ‰ ± 2.6 ‰ and 29 ‰ ± 19 ‰, with corresponding diurnal variations in surface snow δ18O and δD of 0.80 ‰ ± 0.35 ‰ and 1.6 ‰ ± 2.7 ‰. The modeled results under summer cloudy conditions display similar patterns to those under clear-sky conditions but with much smaller magnitudes of diurnal variations. However, under winter conditions at Dome A, the model predicts few to no diurnal changes in snow isotopes, consistent with the stable boundary condition in winter that inhibits effective vapor exchange between the atmosphere and snow. In addition, after 24 h and continuous simulations of 11 d, the model predicts significant enrichments in snow isotopes under summer conditions, while in winter, the depletions also accumulate after each 24 h simulation but with a much smaller magnitude of change compared to the results from summer simulations. If the modeled snow isotope enrichments in summer conditions and the depletions in winter conditions represent the general situation at Dome A, this likely suggests that atmosphere–snow water vapor exchange tends to increase snow isotope seasonality, and the annual net effect would be overall enrichments in snow isotopes since the effects in summer appear to be greater than those in winter. This trend will need to be further explored in the future with more comprehensive model studies and/or field observations and experiments.
- Article
(5482 KB) - Full-text XML
-
Supplement
(726 KB) - BibTeX
- EndNote
Water-stable isotopes (δ18O and δD) in snow and rain are valuable proxies to inform researchers about atmospheric temperatures at the time of precipitation (Craig, 1961; Dansgaard, 1964). In Antarctica, the isotopic composition of snowfall, as well as that of surface snow, is correlated with local air temperature (Fujita and Abe, 2006; Masson-Delmotte et al., 2008; Stenni et al., 2016). These findings permit past temperature reconstructions using ice-core δ18O and δD records across different timescales (e.g., from millennial to glacial–interglacial) (Petit et al., 1999; EPICA community members, 2004; WAIS Divide project members, 2013). Temperature information at shorter timescales (e.g., seasonal to decadal or longer) is critical for understanding climate variabilities and probing driving forces, and thus many studies have focused on high-resolution temperature reconstructions using water isotope profiles (e.g., Stenni et al., 2017). However, there are an increasing number of observations indicating that air temperature and snow/ice-core water isotopes are not always co-varying, especially at decadal or shorter timescales, and the disconnection is particularly obvious at low snow accumulation rate sites such as Vostok, Dome F, and Dome C, Antarctica (Hoshina et al., 2014; Ekaykin et al., 2017; Casado et al., 2018). Such observations suggest changes in snow water isotopes after deposition, which not only inhibit temperature reconstructions at decadal or shorter timescales using ice-core δ18O and/or δD records but also undermine reconstructions at longer timescales such as millennial and glacial–interglacial climate changes (Touzeau et al., 2016; Casado et al., 2018; Laepple et al., 2018; Markle and Steig, 2022).
It is well known that after snow deposition, a combination of post-depositional processes can induce significant changes in the water isotopic composition of snow (Steen-Larsen et al., 2013; Casado et al., 2018; Laepple et al., 2018). Such changes have been demonstrated by the gradual weakening of snow isotope–temperature relationships as reflected by surface and buried snow samples (Casado et al., 2018). Atmosphere–snow water vapor exchange is one such process, but there are limited observations and modeling studies focusing on this process at the diurnal scale in polar summers (Ritter et al., 2016; Casado et al., 2018; Madsen et al., 2019; Hughes et al., 2021; Wahl et al., 2021, 2022; Hu et al., 2022). The isotopic effects associated with atmosphere–snow water vapor exchange at longer timescales have been investigated at Greenland Ice Sheet (Dietrich et al., 2023) but not yet in Antarctica.
Atmosphere–snow water vapor exchange is the snow sublimation–water vapor deposition cycle occurring at the atmosphere–snow interface. It is driven by near-surface vapor pressure gradients and influenced by temperature, wind speed, and humidity (Neumann et al., 2009; Sokratov and Golubev, 2009; Ritter et al., 2016; Wahl et al., 2021, 2022). Dansgaard et al. (1973) proposed that the layer-by-layer sublimation of snow and ice does not induce isotopic fractionation, but this was suggested to be invalid based on laboratory experiments and field observations in which sublimation was found to modify surface-snow isotopic compositions under natural conditions (Sokratov and Golubev, 2009; Ebner et al., 2017; Hughes et al., 2021; Wahl et al., 2021). Water vapor sublimated from snow can be transferred to the overlying atmosphere where it affects the atmospheric water vapor concentration and isotopic composition. Moreover, the inverse part of sublimation, i.e., deposition, can also lead to changes in the isotopic composition of surface snow as well as atmospheric water vapor due to preferential deposition of heavy isotopes (e.g., and HDO; Wahl et al., 2021). Given fluctuations in surface temperature, humidity, and other meteorological conditions, the relative degree of sublimation vs. deposition could vary, leading to variations in the isotopic compositions of surface snow and atmospheric boundary layer water vapor (Neumann et al., 2009; Sokratov and Golubev, 2009; Ritter et al., 2016; Wahl et al., 2021, 2022; Hughes et al., 2021). Parallel variations in the isotopic composition of atmospheric water vapor and surface snow (0.2–1.5 cm depth) have been observed at multiple polar sites (e.g., Dome C, Kohnen station, NEEM, and EastGrip) in summer for short durations (Steen-Larsen et al., 2013; Casado et al., 2016, 2018; Madsen et al., 2019; Bréant et al., 2019), and such co-variations have been suggested to be due to atmosphere–snow water vapor exchange.
Given the difficulties in conducting continuous high-resolution observations in polar regions, a model frame describing the atmosphere–snow water vapor exchange processes and the associated isotope effects would be useful in terms of snow and ice-core water isotope record interpretation across different sites. Such models, if fully resolving the physical mechanisms of atmosphere–snow water vapor exchange processes with appropriate parameterizations, can be incorporated into snowpack and climate models to assess the effects of atmosphere–snow water vapor exchange on the preservation of snow water isotope signals. Several empirical models have been developed to evaluate the isotope effects of atmosphere–snow water vapor exchange. They incorporate atmospheric stratification and climatological boundary conditions to calculate water mass and isotope exchanges at the atmosphere–snow interface by assuming a closed system with a one-dimensional box model (Ritter et al., 2016; Casado et al., 2018; Pang et al., 2019).
As the interior dome of East Antarctica, Dome Argus (80.42° S, 77.12° E; 4093 m above sea level, m a.s.l.), Dome A hereafter, has a more southerly moisture source than other sites on the eastern Antarctic Plateau (Wang et al., 2012). This makes ice-core records of water isotopes from Dome A special in terms of recording southern mid-altitude moisture influence. In addition, Dome A is a candidate site in the search for ancient ice as it is 1.0–1.5×106 years old (Sun et al., 2009; Van Liefferinge et al., 2018). Since 2009, the Kunlun deep-ice coring project has been conducted at Dome A. By the 2015/16 field season, an 800 m ice core had been drilled (Hu et al., 2021), and a preliminary analysis of water isotopic records of the top 109 m reflected a long-term cooling trend at Dome A over the last 2 kyr (Hou et al., 2012; Jiang et al., 2012; An et al., 2021). Given the extremely low snow accumulation rate (18–23 mm water equivalent per year from Ding et al., 2016) at Dome A, water isotopes preserved in firn and ice cores at this site are presumably influenced by post-depositional processing. In particular, the effects of atmosphere–snow water vapor exchange might become important, as snow can stay at the surface for a relatively long period. This characteristic not only means that water isotope records from Dome A should be carefully evaluated for the effects of atmosphere–snow water vapor exchange before interpretation but also makes Dome A a promising site for elucidating the isotopic effects of atmosphere–snow water vapor exchange. In addition, reanalysis data indicate that at Dome A the time interval between two precipitation events can reach ∼80 d (estimated based on the ERA5 reanalysis dataset), which means that snow can sit at the surface for a substantially long period before burial and is subject to extensive atmosphere–snow water vapor exchange that consequently affects the isotopic composition of the buried snow. Pang et al. (2019) estimated the potential influence of summer (November to January) sublimation on the isotopic composition of surface snow at Dome A using a simple Rayleigh distillation model. They found that on average surface snow δ18O was enriched by 2.0 ‰ compared to fresh snow δ18O. However, this evaluation may underestimate the isotopic effects since it did not consider the potential effects of atmospheric dynamic conditions and clouds. A new model is thus needed to provide a more comprehensive evaluation of the isotopic effect of atmosphere–snow water vapor exchange at Dome A, especially for seasons other than summer when observations are not available.
To provide a more comprehensive assessment on the effects of atmosphere–snow water vapor exchange for snow and atmospheric water isotope variations at Dome A, we constructed an improved one-dimensional box model based on previous work (Ritter et al., 2016; Casado et al., 2018; Touzeau et al., 2018) to predict changes in snow and water vapor isotopic compositions at Dome A at the diurnal scale. The main characteristics compared to models in the literature include the use of the bulk aerodynamic method to parameterize atmosphere–snow water vapor exchange. This model was first validated using observations at Dome C and then applied under Dome A conditions.
2.1 Model construction and description
Similar to the model developed by Casado et al. (2018), the model presented in this study contains three water reservoirs, i.e., the free-atmospheric water vapor layer, the atmospheric boundary layer, and the topmost snow layer (Fig. 1). Their masses and isotopic compositions are considered to be associated only with atmosphere–snow water vapor exchange occurring at the atmosphere–snow interface and the exchange of air masses occurring between the free atmosphere and boundary layer. These two processes can cause changes in the masses and isotopic compositions of water vapor in the boundary layer, whereas the masses and isotopic compositions of snow are influenced only by atmosphere–snow water vapor exchange.
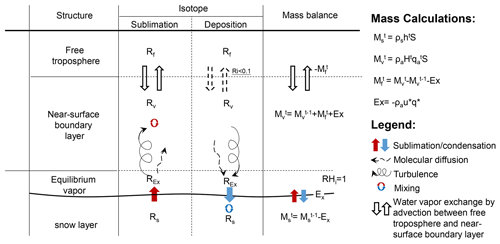
Figure 1Schematic diagram of the box model used in this study. Please note that the dotted arrows between the free atmosphere and the boundary layer indicate that exchange can only take place under the condition that the Richardson number (Ri) is less than 0.1.
The atmosphere–snow water vapor exchange consists of two processes: sublimation and deposition (Fig. 1). During sublimation, water vapor is released from snow, transported into the atmospheric layer via turbulent mixing and molecular diffusion, and immediately mixed with the water vapor already in the boundary layer. During deposition, water vapor is influenced by aerodynamic resistance from turbulence and molecular diffusion, and the deposit is mixed with the surface snow layer. While water vapor transportation at the atmosphere–snow interface relies on two different diffusion pathways, turbulence plays a more crucial role in mass and energy exchanges (Brun et al., 2011; Vignon et al., 2017).
In the box model, atmosphere–snow water vapor exchange flux is calculated by turbulent quantities at each time step of 1 h, as detailed in Sect. 2.1.1. Based on atmosphere–snow water vapor exchange flux parameterization, the model further calculates temporal variations in snow and water vapor isotopic compositions according to isotopic mass balance (detailed in Sect. 2.1.2).
Model inputs mainly include meteorological conditions, e.g., air temperature (Ta), surface temperature (Ts), humidity (relative humidity, RHw, or specific humidity, qa)), and wind speed (ua). Additional model inputs include the mixing-layer height (H0), snow layer thickness (h0), and the initial isotopic values, i.e., the snow isotopic composition (δs0), water vapor isotopic composition in the boundary layer (δv0), and water vapor isotopic composition in the free atmosphere (δf0).
2.1.1 Atmosphere–snow water vapor exchange flux parameterization
We used the bulk aerodynamic method and Monin–Obukhov similarity theory (Monin and Obukhov, 1954) to estimate turbulent fluxes. This approach calculates the net effects of sublimation and deposition at each time step using meteorological data, avoiding the parameterization of the individual fluxes of sublimation and deposition.
The bulk aerodynamic method estimates the atmosphere–snow water vapor exchange flux (Ex) through calculation of latent heat (LE) between the surface and one reference height (z) in the boundary layer (Berkowicz and Prahm, 1982). The expression is as follows:
where ρa is the dry-air density varying with the observed air temperature (Ta) and pressure (Pa), Ls is the sublimation heat constant, and u∗ and q∗ are the friction velocity and specific humidity turbulence scale, respectively. u∗ and q∗ are defined as
where k denotes the von Kármán constant, ua is the wind speed at the reference height in the boundary layer (z=4 m), q0 is the saturated specific humidity at the snow surface derived from the Clausius–Clapeyron equation, qa is the specific humidity that can be estimated from the observed relative humidity over the ice surface (RHi) once the saturated specific humidity at the reference height (qs) is known from the August–Roche–Magnus Formula at a given temperature (Ta), z0 represents the surface roughness length for humidity exchange, and ΨM is the diabatic correction term with respect to the ratio of the reference layer height (z) and Monin–Obukhov length (L). L is defined as
where is the mean potential temperature between the snow surface (θ0) and the reference height in the boundary layer (θa), g is the gravitational acceleration, and u∗ and θ∗ are the friction velocity and temperature turbulence scale, respectively. The θ∗ is analogous to u∗ and q∗, using θa, z0, and :
In Eqs. (2), (3), and (5), z0 can be estimated using least-squares fitting with the observed wind speed at three different heights under neutral atmospheric stratification. The ΨM is calculated for stable, unstable, and neutral boundary layers using the functions taken from Louis (1979). The determination of atmospheric stability depends on the Richardson number (Ri), which is defined as follows:
Based on Eqs. (1)–(6), the atmosphere–snow water vapor exchange flux, Ex, can be calculated in the model with appropriate inputs. A positive value of Ex represents net sublimation (i.e., sublimation > deposition), while a negative value of Ex corresponds to net deposition (i.e., sublimation < deposition).
2.1.2 Isotopic mass balance
Assuming that the snow reservoir is influenced only by atmosphere–snow water vapor exchange (Fig. 1), temporal variations in snow mass per unit of surface area (S) can be expressed as
where Ex is the exchange flux as calculated in the previous section, Ms is the mass of the defined surface snow, and the superscript t denotes time. From Eq. (7), Ms at time t can be calculated from the initial snow masses (i.e., masses at t=0) and the accumulated Ex at time t. In the model, Ms at t=0 relies on the initial snow height (h0) and snow density (ρs). The water vapor mass in the boundary layer (Mv) at time t and the unit area (S) can be computed from the initial boundary height (H0), dry air density (ρa), and specific humidity (qa) at the reference height in the boundary layer:
where qa at time t can be determined by direct measurements or the observed relative humidity (RHi). In Eq. (8), we neglect the temporal changes in the height of the boundary layer, given that the boundary heights in polar inland regions are relatively stable (Bonner et al., 2010; B. Ma et al., 2020). According to Eq. (8), the mass changes in the atmospheric boundary water vapor layer at each time interval are ). This quantity is influenced by atmosphere–snow water vapor exchange (Ex) and the water vapor exchange flux from the free atmosphere to the boundary layer (Mf). Thus, Mf at any time can be quantified as follows:
Note that the exchange between the boundary layer and the free atmosphere can occur under unstable conditions or weakly stable conditions (Zilitinkevich and Esau, 2007). In the model, we hold that Mf can contribute to the atmospheric boundary water vapor reservoir when the Richardson number is less than 0.1 (i.e., including weakly stable conditions in addition to unstable conditions).
Based on the calculation of mass changes in the three reservoirs (Eqs. 7–9), the isotopic mass equations are
where Rs, Rv, Rf, and REx represent the ratios of heavy isotopes (18O and D) and light isotopes (16O and H) in the snow layer, atmospheric boundary layer, free-atmospheric layer, and exchange flux, respectively.
The calculation of REx differs between the sublimation-dominated (i.e., net sublimation) period and deposition-dominated (i.e., net deposition) period. For the sublimation-dominated phase (Ex>0), kinetic fractionation is assumed to occur when the sub-saturation condition is considered. The isotopic composition of the sublimated vapor is calculated from Merlivat and Jouzel (1979), combining Rs, Rv, the diffusion coefficient (k′), the equilibrium coefficient (αe), and the relative humidity of the air with respect to the surface temperature (h) as follows:
The isotopic composition of the condensed vapor (Ex<0) is in equilibrium with that of the water vapor above −20 °C. However, kinetic fractionation will also occur due to vapor supersaturation over ice on the eastern Antarctic Plateau. This effect can reduce the effective fractionation of water isotopes. Therefore, the equilibrium coefficient (αe) is replaced by the effective fractionation coefficient (αf) when calculating the REx of condensed vapor. αf is defined as the product of the kinetic fractionation coefficient (αk) and αe. The REx of condensed vapor is thus expressed as
αe with respect to ice is given by Ellehoj et al. (2013) as a function of temperature (Eq. 13).
αf is deduced from αe as follows
where Di is the diffusivity of the water molecule and is the same as Di but for heavy isotopes. The ratios of are given by Jouzel and Merlivat (1984), with values of 1.0285 for 18O and 1.0251 for D.
The key variables in the model are summarized and listed in Table S1 in the Supplement.
2.2 Model simulations
We first used the abovementioned model to simulate atmosphere–snow water vapor exchange and the associated isotope effects at Dome C (75.10° S, 123.33° E; 3233 m a.s.l.), where diurnal variations in water vapor isotopic compositions as well as surface snow water isotopes are available from observations (Casado et al., 2016; Touzeau et al., 2016). We then applied the model to Dome A conditions to investigate the isotopic effects due to atmosphere–snow water vapor exchange at diurnal scales. The initial model values, including mixing-layer height (H0), snow layer height (h0), snow isotopic composition (δs0), water vapor isotopic composition in the boundary layer (δv0), water vapor isotopic composition in the free-atmosphere layer (δf0), and snow density (ρs) are listed in Table 1. These values were justified according to the conditions discussed in the following sections.
Table 1Key initial values for diurnal simulations.
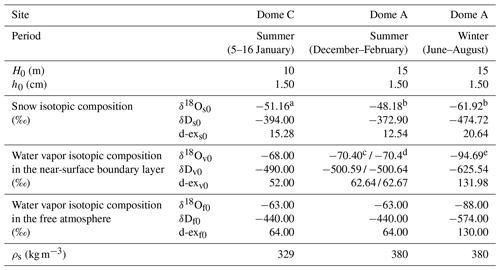
a, b Observations for surface snow isotopes and calculations for fresh snow isotopes, respectively. c, d Values correspond to clear-sky and highly cloudy conditions, respectively. e Some of the winter conditions were set the same as those in summer (see details in Sect. 2.2.3).
2.2.1 Diurnal simulations under Dome C conditions
At Dome C, previous observations revealed a clear diurnal cycle of water vapor isotopic composition from 5 to 16 January 2015 (Casado et al., 2016). This diurnal cycle was attributed to the effects of atmosphere–snow water vapor exchange under clear-sky conditions (Casado et al., 2018). To compare the modeled results with the observations, we performed a continuous simulation using observed meteorological data over the same period (11 d). Meteorological parameters (e.g., temperature, humidity, and wind speed) during the observed period were downloaded from the CALibration and VAlidation of meteorological and climate models and satellite retrievals (CALVA) program (Genthon et al., 2010). The surface snow temperature (Ts) data are available in a previous publication (Casado et al., 2016). The boundary height, H0, was determined by Doppler sodar measurements from an on-site iron tower at Dome C (Vignon et al., 2017). The surface snow layer height, h0, was set to be the thickness of surface snow collected (i.e., 1.5 cm) for isotopic composition analysis at this site (Casado et al., 2018). The initial vapor isotopic compositions in the boundary layer, δv0, were set as the observations of water vapor δ18O, δD, and deuterium excess (d-excess) at the beginning of the modeling period during the 2014/15 field season (Casado et al., 2016), while snow isotopes, δs0, were set as the mean isotopic values of summer surface snow samples (Casado et al., 2018). The water vapor isotopic composition in the free atmosphere layer (δf0) was not reported at this site. Here we expect that δf0 is greater than δv0. Although there are currently no vertical observations of water vapor isotopic composition in Antarctica, vertical isotopic profiles (δ18O) observed at Summit Camp, Greenland, have indicated that the isotopic composition of water vapor in the free atmosphere is slightly higher than that within the boundary layer (Berkelhammer et al., 2016). In order to explain the water vapor and snow isotope observations at Dome C, Casado et al. (2018) assumed that the contribution from the free atmosphere can increase the ratio of molecules in the boundary layer (Casado et al., 2018) and set δf0 as the highest observed value of water vapor isotopic composition at Dome C. Note that δf0 was a constant value for the simplicity of model calculations. The density of the topmost 5 cm of surface snow (ρs) was reported by Champollion et al. (2019).
2.2.2 Simulations under Dome A summer conditions
Previous studies have shown that a diurnal cycle clearly occurs in surface snow and water vapor isotopic compositions during clear-sky days, whereas this feature is not significant in highly cloudy periods (Casado et al., 2016; Ritter et al., 2016; Hughes et al., 2021). Clouds play an important role in modulating atmospheric thermal and dynamic conditions (Haynes et al., 2013), and cloudy conditions may also mean more moisture present in the atmosphere. Under cloudy conditions, extra moisture and downward radiation from clouds likely disturb local temperature and humidity variabilities, resulting in smaller differences between day and night atmosphere–snow water vapor exchange, and thus the isotopic effects are less pronounced. Therefore, in the model simulations for Dome A summer conditions, we not only simulated continuous changes in surface snow and water vapor isotopic composition over a multiday timescale but also incorporated two representative scenarios (i.e., cloudy vs. clear-sky conditions) to ensure a rigorous assessment of the isotopic variations associated with atmosphere–snow water vapor exchange processes.
The simulations with continuous meteorological input were conducted without considering the influence of clouds. The selected period for summer simulations was from 5 to 16 January for each year from 2006 to 2011 (with the exception of 2005 for which data were not available). The model was thus run for 11 d each year, consistent with the Dome C simulations. By averaging the six simulated results obtained from the simulations, we were able to estimate the continuous changes in water vapor and snow isotopic composition. This approach allowed for a more robust analysis of the simulated data and enabled a direct comparison of the results across different cases (results shown in Sect. 3.2.4 and Fig. 7).
The hourly averages of total cloud cover (Tcc) were used to select days with clear-sky and highly cloudy conditions. These data were retrieved from the ERA5 reanalysis dataset, with a spatial resolution of 1.25°×1.25°. Based on previous studies, the classification criteria are as follows: Tcc≤0.3 for clear-sky conditions and Tcc≥0.8 for highly cloudy conditions (Qian et al., 2012). Following this criterion, we selected 20 clear-sky days during the summer period (December to February) of 2005–2011. Then, the hourly meteorological data from those selected days were stacked to create a representative cycle for model initialization. For highly cloudy conditions, a stack of 102 diurnal cycles of meteorological variables was also produced for modeling at the diurnal scale.
Meteorological data were obtained from an automatic weather station (AWS) installed near the summit of Dome A. The hourly surface air pressure; air temperature at heights of 1, 2, and 4 m; relative humidity at 4 m; wind speed at heights of 1, 2, and 4 m; and wind direction are available for the period of 2005–2011 (Ma et al., 2010; Ding et al., 2022). The surface snow temperature (Ts) observations were not available at Dome A. Thus, we performed Ts calculations based on the method from Brun et al. (2011). The equation for Ts calculation is shown as follows:
where σ is the Stefan–Boltzmann constant, ϵ is the snow emissivity (0.93), and LWdn and LWup are the downward and upward longwave radiative fluxes, respectively. The hourly longwave radiative flux data were retrieved from the ERA5 reanalysis dataset.
The stacked hourly mean values of the meteorological conditions at Dome A are shown in Fig. 2a. During clear-sky conditions, the air temperature at the 4 m level (Ta) shows a diurnal cycle with an amplitude of 10 °C and an average of −31 °C. The diurnal Ts follows a similar pattern to that of Ta, varying between −39 and −28 °C. The ranges of diurnal cycles for specific humidity (qa) and relative humidity (RHi) are 1.8– kg kg−1 and 66 %–130 %, respectively. qa is also parallel to Ta, whereas RHi shows an opposite trend. In contrast to temperature and humidity, the daily air pressure near the surface is stable (∼584 hPa). The wind speed (ua) and latent heat flux reached daily maxima of 3.0 m s−1 and 3.3 W m−2, respectively, at 10:00 UTC, coinciding with the peaks in Ta, Ts, and qa at the diurnal scale. Under highly cloudy conditions, the latent heat exhibits less variability, yet qa and ua display greater diurnal variations (Fig. 2b).
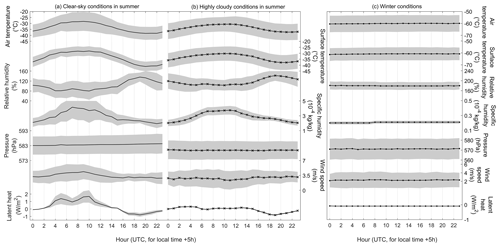
Figure 2Stacks of diurnal cycles of meteorological parameters and the calculated latent heat under summer clear-sky conditions (a), summer highly cloudy conditions (b), and winter conditions (c) at Dome A. The hourly air temperature, relative humidity, air pressure, and wind speed data were averaged from the AWS observations on the selected days. The diurnal variations in the other three parameters were calculated based on hourly observations. In each panel, the solid line with marks represents the average and the gray shadow represents the standard deviation (±1σ).
The initial model values of H0, h0, δs0, δv0, and ρs for the Dome A simulations are listed in Table 1. H0 was estimated as the median thickness of the boundary layer (15 m) based on sonic radar and visual observations of the angular size of stellar images during summer (Bonner et al., 2010; B. Ma et al., 2020). The surface snow thickness, h0, was set to 1.5 cm according to summer snow accumulation at Dome A (calculated from the annual mean snow accumulation of 18–23 mm yr−1). δs0 values were obtained from the average precipitation isotopic composition measurements during the 2009/10 field season at Dome A (Pang et al., 2019). The δv0 can be calculated from δs0 assuming atmosphere-snow equilibrium and using the equilibrium fractionation coefficient at the surface temperature of the beginning of the diurnal cycle. δf0 was set equal to the value at Dome C, since there are no measurements available from Dome A. The ρs in Table 1 was from the measurements taken during the 2014/15 field season (T. Ma et al., 2020).
2.2.3 Simulations under Dome A winter conditions
Given the different meteorological conditions in winter compared to summer, the degree of atmosphere–snow water vapor exchange and the associated isotope effects could be different. Therefore, we also conducted multiday and diurnal simulations for winter at Dome A, similar to the summer simulations. This may shed light on assessments of the effects of atmosphere–snow water vapor exchange on seasonal and annual scales.
Winter simulations that incorporated continuous meteorological data were executed for a duration of 11 d, spanning 5 to 16 July for each year between 2006 and 2011. This enabled the acquisition of six simulated results, which were subsequently averaged to provide a comprehensive understanding of the continuous changes in water vapor and snow isotopic composition over a multiday timescale.
The stacked hourly mean values of winter meteorological conditions at Dome A were extracted in the same way as we did for the summer conditions. As shown in Fig. 2c, the average temperature, specific humidity, and atmospheric pressure are lower than those in summer, but the relative humidity increases during winter. These changes result in the negative values of latent heat flux during winter. In addition, the winter meteorological parameters and latent heat flux do not show any apparent diurnal variations.
The initial model values for the winter simulations are also listed in Table 1. The initial value of the snow isotopic composition () is the average of the precipitation isotopic composition at the starting month for the winter season. Due to the lack of observations, was estimated from the monthly mean temperature and the δ–T slopes in non-summer seasons (0.64 ± 0.02) according to the compiled data from Pang et al. (2019). We also further evaluated these estimations of by a comparison with snowfall δ18O modeled using the water-isotope-enabled ECHAM5 general circulation model (ECHAM5-wiso; Werner et al., 2011). The results of the two methods agree with each other (Sect. S3 in the Supplement), suggesting that estimation using the regression line is reliable. The initial value for the water vapor isotopic composition () was also estimated assuming isotope equilibrium with . δf0 was set to the calculated using and the highest temperature observed in winter during the studied period. h0 is kept the same as that in summer to simplify the calculations. The median H0 at Dome A varies little throughout most of the year according to Bonner et al. (2010) and B. Ma et al. (2020), so in the model we used the same H0 in winter as that in summer. ρs is the annual mean snow density based on measurements (T. Ma et al., 2020), and we did not consider seasonal variations to simplify the calculations.
2.2.4 Sensitivity simulations
Changes in initial parameters could influence the isotopic effects of atmosphere–snow water vapor exchange. For example, previous field experiments have indicated that isotopic enrichment caused by atmosphere–snow water vapor exchange tends to decrease as snow thickness increases (Hughes et al., 2021). Ritter et al. (2016) noted that diurnal variations in water vapor isotopic composition decrease as the mixing-layer height (i.e., H0) increases. These previous findings motivate us to investigate the sensitivity of the modeled results to these boundary conditions and initial values.
The sensitivity tests included three groups of comparative experiments for the Dome A site and were run for a 24 h period under summer clear-sky conditions. The first group focuses on the sensitivity of surface and water vapor δ18O to varying h0 and H0. In the experiment, we vary h0 between 0.1 and 3.0 cm (Ritter et al., 2016; Hughes et al., 2021) and H0 from 1 to 100 m (Bonner et al., 2010; Ritter et al., 2016). The second group is designed to investigate how the uncertainties in and influence the isotopic effects of atmosphere–snow water vapor exchange, especially when and are not in equilibrium. We varied and from −53 ‰ to −43 ‰ (the range of summer precipitation δ18O at Dome A; Pang et al., 2019) and −85 ‰ to −55 ‰, respectively. The range of is estimated from and the equilibrium fractionation coefficient under summer conditions, and and in thermodynamic imbalance are included. In the third group, and snow density were varied to test their influence on the diurnal changes in surface snow and water vapor δ18O, respectively. The selection of −68 ‰ to −58 ‰ for the range refers to the summer observations of water vapor isotopic composition at Dome C (Casado et al., 2016). According to field observations at Dome A and other interior domes (Laepple et al., 2018), the range of snow density was set to 300–400 kg m−3 for sensitivity simulations. Note that the isotope effects are greater in summer than in winter; we only used summer conditions and values to illustrate the sensitivity of the modeled results to these parameters.
3.1 Modeled diurnal and multiday variations at Dome C
On the diurnal scale at Dome C, the modeled water vapor δ18O increases from −68 ‰ at 00:00 UTC to −66 ‰ at 09:00 UTC and then decreases to −75 ‰ at 16:00 UTC (Fig. 3a). The diurnal patterns in water vapor δD are similar to those in water vapor δ18O, and their max–min difference is ∼54 ‰ (Fig. 3b). The water vapor d-excess, defined as d-excess (‰) (Dansgaard, 1964), varies between 52 ‰ and 72 ‰ during the 24 h period (Fig. 3c). Its diurnal pattern is opposite to that of δ18O and δD. The modeled snow δ18O and δD also exhibit a diurnal pattern where higher values occur during the warming phase and lower values occur during the cooling phase (Fig. 3d). The diurnal range of simulated snow δ18O is ∼1.5 ‰ on average, but its value is close to that of the observations (2.0 ‰) during a typical frost event from 6 to 7 January 2015. In addition, the diurnal variations in snow d-excess are opposite to those in snow δ18O and δD, similar to the relationship between vapor δ18O and d-excess. Overall, the modeled diurnal variations in vapor δ18O and δD capture the observations well, while their magnitudes are slightly different from those in the observations.
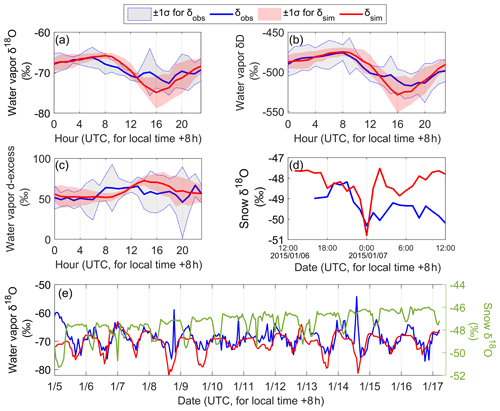
Figure 3Modeled variations in water vapor and snow isotopic compositions at Dome C along with the observations. (a) Diurnal variations in water vapor δ18O, (b) diurnal variations in water vapor δD, (c) diurnal variations in water vapor d-excess, (d) diurnal variations in snow isotopes during 6–7 January 2015, and (e) continuous variations in water vapor and snow δ18O during 5–16 January 2015. In all panels, the solid blue line represents the observations (δobs), with the light-gray shaded area as the uncertainties (±1σ). The solid red line and the light-red shaded area depict the simulation (δsim) and corresponding uncertainties (±1σ). In panel (e), the solid green line represents the modeled snow δ18O. Note that snow δ18O observations at Dome C are available only from 6 to 7 January 2015 (Casado et al., 2018). The method for uncertainty estimation can be found in Sect. S2 in the Supplement.
The continuous simulations at the multiday scale are shown in Fig. 3e. The simulated water vapor δ18O exhibits periodic changes on the diurnal scale, but its daily mean value remains unchanged over the course of the simulation. This trend is consistent with the observations reported by Casado et al. (2016), as evidenced by a high correlation coefficient (R>0.6). The snow δ18O values display a noticeable enrichment trend compared to their initial state, which is different from that of the water vapor δ18O.
3.2 Modeled results at Dome A
3.2.1 Diurnal variations under summer clear-sky conditions
At Dome A, the Richardson number (Ri) varies between −0.01 and 0.02 during the 24 h period (Fig. 4a). The friction velocity of water molecules (u∗) ranges from 0.11 to 0.19 m s−1, with a mean value of 0.14 m s−1 (Fig. 4b). The atmosphere–snow water vapor exchange flux (Ex) calculated from Ri and u∗ varies in parallel with temperature (Fig. 4c). In general, negative Ri values represent relatively unstable atmospheric conditions, which correspond to the phase of sublimation (i.e., net vapor flux from snow to the atmosphere; Fig. 4c). In contrast, Ri appears to be positive during most of the cooling phase (i.e., the net vapor flux from the atmosphere to snow; Fig. 4c), suggesting stable atmospheric conditions.
Figure 4d–f displays the modeled surface snow and water vapor isotopic compositions and the uncertainties. All the isotopes display apparent diurnal cycles. In particular, water vapor δ18O and δD indicate enrichments in the sublimation period, followed by depletions during the rest of the day when condensation (vapor deposition) dominates (Fig. 4d and e). The snow δ18O and δD values exhibit similar but somewhat opposite patterns within 24 h (Fig. 4d and e). The diurnal pattern of d-excess is opposite to that of δ18O and δD in snow and vapor (Fig. 4f). Overall, the diurnal patterns of snow and water vapor isotopes at Dome A are similar to those at Dome C during summer cloudless conditions.
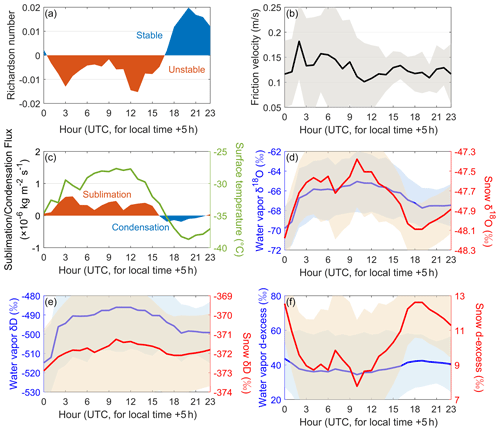
Figure 4The simulated hourly mean vapor exchange flux and variations in atmospheric water vapor and snow isotopes under summer clear-sky conditions at Dome A: (a) Richardson number, (b) friction velocity, (c) vapor exchange flux, (d) snow and water vapor δ18O, (e) snow and water vapor δD, and (f) snow and water vapor d-excess. The uncertainties for each variable are displayed by shaded areas in each panel.
The magnitudes of the diurnal range in water vapor isotopic composition are 4.8 ‰ for δ18O, 29 ‰ for δD, and 9.3 ‰ for d-excess. In comparison, the modeled diurnal isotope variations in surface snow are much smaller with magnitudes of 0.80 ‰ for δ18O, 1.6 ‰ for δD, and 4.9 ‰ for d-excess. In addition, after 24 h of model operation, the water vapor δ18O, δD, and d-excess increase by 2.4 ‰, 16 ‰, and 3.1 ‰, respectively (Fig. 4d–f). Moreover, after 24 h, the snow isotopic compositions display enrichments of 0.29 ‰ for δ18O and 1.1 ‰ for δD and a depletion of 1.3 ‰ for d-excess.
3.2.2 Diurnal variations under highly cloudy summer conditions
Under highly cloudy conditions, the Richardson number (Ri) is almost neutral or unstable at the diurnal scale (Fig. 5a). The friction velocity (u∗) exhibits a diurnal cycle varying between 0.11 and 0.13 m s−1 (Fig. 5b), which is much smaller than that under clear-sky conditions. We also found a diurnal cycle in the atmosphere–snow water vapor exchange flux (Ex), as shown in Fig. 5c. Overall, the diurnal changes in u∗, Ri, and Ex are less pronounced compared with those under clear-sky conditions.
The diurnal cycle patterns in water and surface snow isotopic compositions are also apparent under cloudy conditions (Fig. 5d–f), but the magnitudes are smaller than those under clear-sky conditions. In particular, the diurnal peak-to-valley differences in water vapor isotopic compositions are 3.0 ‰ for δ18O, 21 ‰ for δD, and 4.0 ‰ for d-excess. The diurnal variations in the surface snow isotopic composition have a magnitude of 0.28 ‰ for δ18O, 0.87 ‰ for δD, and 2.2 ‰ for d-excess. In addition, after 24 h, snow water isotopes were enriched in the model, the same as in clear-sky conditions.
3.2.3 Diurnal variations under winter conditions
The winter simulation results are plotted in Fig. 6. Under winter conditions, the Richardson number (Ri) and the friction velocity (u∗) remain stable over a full 24 h period (Fig. 6a and b). The atmosphere–snow water vapor exchange flux (Ex) shows negative values throughout 24 h (Fig. 6c), suggesting that sublimation does not occur under Dome A winter conditions. As a result, in contrast to the simulated results in summer, there are no significant diurnal variations in snow isotopes in winter, but the changes in water vapor isotopic composition in winter are comparable to those in summer. This can be associated with the almost-constant meteorological conditions and the relatively weak exchange between snow and atmospheric water vapor during a diurnal period, as displayed in Fig. 2c. In addition, because the isotopic composition of deposited vapor is much lower than that of surface snow, the winter snow layer experiences small but steady depletions in δ18O and δD (Fig. 6d and e). In contrast, snow d-excess becomes more enriched under the effects of the atmosphere–snow water vapor exchange flux (Fig. 6f). The water vapor isotopic composition also displays depletion because heavier isotopes tend to deposit faster.
3.2.4 Continuous changes at the multiday scale at Dome A
The continuous simulations presented in Fig. 7 reveal that the water vapor isotopic composition (δ18O) exhibits substantial interannual differences in absolute values, even during the same period (Fig. 7a and c). In addition, these simulations and their averages display distinct diurnal periodicity. On the multiday scale, the average water vapor δ18O values do not show a significant trend with increasing simulation time. Their values fluctuate around −72 ‰ in the summer and −105 ‰ in the winter. The diurnal cycles shown in the Dome A continuous simulations are consistent with the simulated results at Dome C.
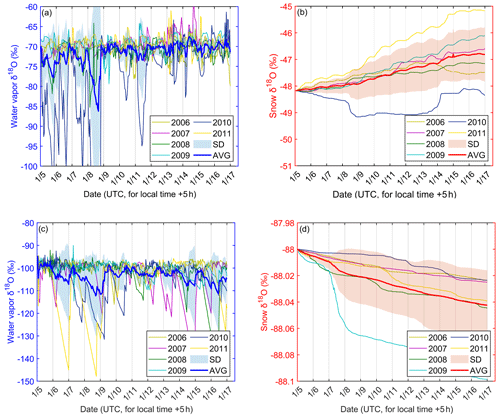
Figure 7Continuous simulations of snow and water vapor isotopes at Dome A. Panels (a) and (b) represent summer simulations over an 11 d period (5–16 January 2006–2011), and panels (c) and (d) are the same as panels (a) and (b) but for wintertime (5–16 July 2006–2011). In all panels, the colored lines represent the simulated results of water vapor δ18O for each year during the simulation period. The bold lines and the light-blue/red shadows are the averages (AVGs) and standard deviations (SDs) of the δ18O simulations in each year, respectively.
The snow isotopic composition (δ18O) simulations in each year exhibit a striking similarity in their trends during the summer and winter seasons. Specifically, the snow δ18O values at the end of the simulation are consistently higher than the initial values during the summer (Fig. 7b). Conversely, a slightly negative trend can be observed in the winter simulations (Fig. 7d).
3.3 Sensitivity to model parameters
In the first group of sensitivity tests (Fig. 8a), the water vapor and snow isotopic composition displayed distinct patterns in response to variations in the snow depth (h0) and the boundary layer height (H0). The magnitude of the diurnal variations in water vapor δ18O () is highly influenced by H0 but not by h0. This finding aligns with previous calculations at Kohnen Station, which demonstrated a decrease in the magnitude of with increasing mixing-layer height (Ritter et al., 2016). On the other hand, the magnitude of diurnal variations in snow δ18O () exhibits a greater sensitivity to h0 (Fig. 8b). This finding is consistent with field experiments showing that isotopic enrichment induced by atmosphere–snow water vapor exchange tends to decrease with increasing snow thickness (Hughes et al., 2021). Similar to the magnitude of , the changes in after a diurnal cycle are more sensitive to h0 (Fig. 8c).
In the second group of tests, where and vary, the magnitude of diurnal changes is more sensitive to than to (Fig. 8d). As decreases, the magnitude of diurnal changes increases, emphasizing the influence of on snow isotopic variations (<0.05 ‰ in Fig. 8e). In addition, the value of after a diurnal cycle shows greater sensitivity to , while such a change remains small (<0.01 ‰ in Fig. 8f).
In the third group, with varying and snow density (ρs), changes in significantly influence the magnitude of diurnal variations in (Fig. 8g). In contrast, these changes have a lesser effect on the magnitude of diurnal variations and changes after a diurnal cycle (Fig. 8h and i). The snow density has a considerable effect on , while it induces only a small change in the magnitude of diurnal fluctuations.
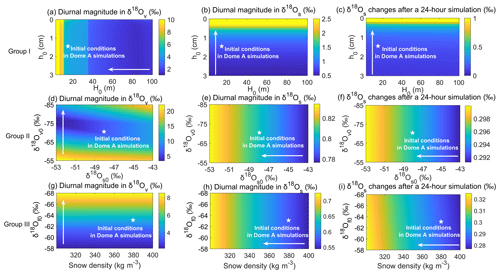
Figure 8Sensitivity of the modeled results to changes in initial conditions. Panels (a–c) display the modeled magnitude of δ18O diurnal variations in water vapor (), the modeled magnitude of δ18O diurnal variations in surface snow (), and differences between the ending and starting values varying with different surface snow thicknesses (h0) and boundary layer heights (H0). Panels (d–f) show the sensitivities of the simulated results to changes in initial water vapor () and surface snow isotopic composition (). Panels (g–i) are the same as (d–f) but show the sensitivities to changes in the water vapor isotopic composition of the free atmosphere () and snow density (ρs). In each panel, the white star indicates the initial conditions used in the Dome A simulations with summer clear-sky conditions. The white arrows correspond to the direction of the simulated results with higher sensitivity.
Despite differences in the magnitudes, under summer clear-sky and highly cloudy conditions the modeled isotopes in surface snow and water vapor display clear diurnal patterns at Dome A. In both of these cases, the water vapor isotopes show a smaller magnitude of diurnal variations with respect to the snow isotopes. In general, in the period of mass exchange dominated by sublimation, snow δ18O and δD are enriched because lighter isotopes are preferentially sublimated into the atmosphere. Moreover, sublimates mixing with water vapor leads to increases in vapor δ18O and δD because they have higher δ18O and δD values than atmospheric vapor. During periods of mass exchange dominated by deposition, water vapor δ18O and δD are significantly depleted (Ritter et al., 2016). Note that the effects on snow δ18O and δD are smaller than those on vapor δ18O and δD. This is because the surface snow mass reservoir is much larger than the mass of deposition, so the associated isotope effects on surface snow are very small (Steen-Larsen et al., 2013; Casado et al., 2018).
Based on Figs. 2, 4c, 4d, 5c, and 5d, it is evident that the diurnal isotope cycles in surface snow and water vapor have a strong correlation with surface temperature and humidity. As described in Sect. 2.1, surface temperature can modify local atmospheric dynamic conditions and specific humidity, leading to synchronous responses in atmosphere–snow water vapor exchange fluxes. Temperature can also affect isotope fractionation during phase exchange. Atmosphere–snow water vapor exchange is associated with equilibrium and kinetic isotope fractionation between snow and water vapor (Ritter et al., 2016; Hughes et al., 2021; Wahl et al., 2021). The degree of isotopic equilibrium fractionation is directly dependent on the local surface temperature (Ellehoj et al., 2013), while kinetic isotope fractionation is mainly driven by the vapor pressure gradient between the snow surface and atmosphere (Jouzel and Merlivat, 1984; Surma et al., 2021; Passey and Levin, 2021). The specific humidity is also crucial because it represents the size of the water vapor reservoir with which snow can exchange (Casado et al., 2018). However, it is only important for atmospheric vapor δ18O and δD, as surface snow is a much-larger mass reservoir that buffers the effects of atmospheric vapor change. Wind speed also plays a key role in driving isotopic variations at Dome A because its increase can amplify the variations in latent heat, leading to more pronounced diurnal changes in water vapor and snow isotopic composition (Sect. S5 in the Supplement, Bréant et al., 2019).
The diurnal variations in water vapor isotopic composition resulting from the exchange between the atmosphere and snow surface are subject to influences beyond mere meteorological conditions. Specifically, fluctuations in the boundary layer height (H0) can result in either an attenuation or an amplification of the magnitude of variations in water vapor isotopic composition (Ritter et al., 2016), as evidenced by Fig. 8a. Furthermore, the interaction between the free atmosphere and the boundary layer can significantly impact the diurnal variations in the water vapor isotopic composition (Casado et al., 2018). Specifically, during periods of intense mixing, the variations in water vapor isotopic composition become more pronounced (Fig. 8g and Sect. S4 in the Supplement). However, in the model employed for this study, the boundary layer height (H0) and water vapor isotopic composition in the free-atmosphere layer (δf0) are maintained as constants to simplify the calculations, whereas they vary daily in reality. This simplification for model calculations may lead to a reduction in the inter-day variability in simulated water vapor isotopic compositions (Fig. 3e).
We also compared our modeled water vapor δ18O, δD, and d-excess data at Dome A with water vapor δ18O, δD, and d-excess data from other East Antarctic interior sites from observations, such as the Kohnen station, Dome C, and a location approximately 100 km away from Dome A (Ritter et al., 2016; Casado et al., 2016; Liu et al., 2022). Both our simulations and observations have similar diurnal patterns, with high values occurring during daytime warming and low values occurring during nighttime cooling. However, it is worth noting that the magnitudes differ between the diurnal simulations at Dome A and the observations at other sites. Our modeled δD variations at Dome A (29 ‰ ± 19 ‰) are lower than the observed diurnal variations in water vapor δD at Kohnen station (36 ‰ ± 6 ‰ from Ritter et al., 2016) and at Dome C (38 ‰ ± 2 ‰ from Casado et al., 2016). This difference can be attributed to the atmospheric dynamic conditions at Dome A, which are characterized by a lower daily mean wind speed (2.8 m s−1) than that at Dome C (3.3 m s−1) and Kohnen station (4.5 m s−1) during the summer season (Casado et al., 2018). A lower wind speed corresponds to relatively weak air convection in the vertical direction. Due to the coupling between upper- and lower-atmospheric layers, vertical turbulent mixing may decrease with weakened air convection in the atmospheric near-surface layer (Casado et al., 2018). This change can attenuate molecular exchange between surface snow and water vapor, leading to a muted fluctuation in the modeled water vapor δD in combination with less mass exchange. In addition, the simulated diurnal changes in water vapor isotopic composition are lower than those observed at sites near Dome A (>40 ‰ for δ18O and 200 ‰ for d-excess). This large discrepancy may be due to calibration drifts caused by the low-water-vapor content during the measurements at the nearest Dome A site (Liu et al., 2022).
The magnitudes of the modeled diurnal changes in snow δ18O and δD are different between highly cloudy and clear-sky conditions, with apparently small magnitudes under cloudy conditions. It seems that when clouds are present, surface snow will receive longwave radiation from clouds and be less influenced by solar radiation. As a result, the diurnal radiation budget cycle is less variable than that on days without clouds, as otherwise, solar radiation with a strong diurnal cycle becomes the most significant variable. On days with clouds, the diurnal variations in air temperature and surface temperature are also smaller, and the differences between the air temperature and surface temperature during the day and night become less pronounced (Fig. 2). This could have a negative impact on the changes in atmospheric dynamics between day and night. The diurnal variations in the wind speed and friction velocity are thus not significant (Figs. 2, 4b, and 5b). The vertical turbulent mixing between surface snow and water vapor in a diurnal cycle is relatively stable. This results in reduced mass exchange and a decrease in the isotope effects occurring between these two reservoirs.
The model results for summer clear-sky and highly cloudy conditions also indicate that after a 24 h simulation, δ18O and δD in surface snow are enriched mainly due to isotope fractionation during sublimation. Notably, although water vapor with much-lighter δ18O and δD values than snow are deposited in the deposition period, the masses are negligible compared to those in the snow mass reservoir, so the effects on snow isotopes in the 24 h simulation period are dominated by the effects of sublimation. The enrichments in snow isotopes caused by sublimation are consistent with previous studies (e.g., Ritter et al., 2016; Casado et al., 2018; Hughes et al., 2021). In addition, sublimation is associated with snow mass loss. Many studies also indicate significant surface snow mass loss during summer due to sublimation at inland Antarctic sites including Dome A (e.g., Frezzotti et al., 2004; Ding et al., 2016). As such, at Dome A, surface snow isotopes are presumably enriched during summer. Using a simple Rayleigh distillation model, Pang et al. (2019) predicted that over summer ∼2 ‰ enrichments in surface snow δ18O can be caused under mean Dome A summer conditions.
Based on the results of the sensitivity tests, diurnal variations in isotopic composition of snow due to water vapor exchange processes can also be influenced by several parameters, such as snow thickness, snowfall isotopic composition, snowfall density, and surface roughness (refer to Fig. 8 and Sect. S5). Among these factors, changes in snowpack thickness exhibit the most pronounced impact on the isotopic effects of water vapor exchange processes. Specifically, when the snow thickness exceeds 3 cm, the water vapor exchange effect struggles to induce inter-day variations in snow isotopes. On the other hand, the effects of snowfall isotopic composition, snowfall density, and surface roughness on the isotopic composition of surface snow may be limited during the Dome A summer season (Sect. S5), given the realistic range of potential variations in snowpack parameters.
Under the typical winter conditions at Dome A, temperature and humidity remain relatively constant throughout the day (i.e., during a 24 h simulation period). The Richardson number (Ri) is positive throughout the day, indicating stable atmospheric conditions. As a result, the diurnal variations in the exchange of atmospheric water vapor and snow isotopes are less pronounced. Specifically, the model simulations suggest that under these conditions only deposition occurs, leading to a depletion of snow isotopes (δ18O and δD) after the 24 h simulation period.
Because the diurnal variations in snow isotopic composition induced by atmosphere–snow water vapor exchange in summer and winter are different, seasonal snow isotopic changes can be affected. In particular, according to the modeled results, in summer surface snow δ18O and δD should become more enriched than fresh snow, while in winter surface snow isotopes should be less abundant than in fresh snow. This effect appears to be distinct from what can be expected from other post-depositional processes. For example, Town et al. (2008) demonstrated that wind-driven ventilation after snowfall can result in isotope enrichment in winter snow layers and depletion in summer snow layers, decreasing the magnitude of seasonal variations. Vapor diffusion in snow pores also contributes to the attenuation of δ18O or δD seasonal variations by smoothing (Johnsen et al., 2000; Casado et al., 2020). To evaluate the annual net effect of atmosphere–snow water vapor exchange, the potential mass loss in summer and gain in winter must be estimated. From just the continuous simulations of this study, it appears that the annual net effects should lead to isotopic enrichment in the snow layer since the magnitudes of isotopic changes in summer are much larger than those in winter. However, we note that the continuous simulation in this study was conducted without differentiating between clear-sky and cloudy conditions and was considerably affected by the abrupt temperature fluctuations observed at Dome A. Therefore, further exploration of continuous simulations is required, which can be achieved through improvements in model refinement and the capabilities of observational techniques with more-precise data available.
Atmosphere–snow water vapor exchange is important for snow isotope preservation as suggested by previous studies (Ritter et al., 2016; Hughes et al., 2021; Hu et al., 2022). In this study, we constructed a new box model based on the bulk aerodynamic method to predict changes in surface snow and water vapor isotopic compositions in response to diurnal fluctuations in local meteorological conditions. The model was validated by the agreement between the modeled and observed diurnal cycles of water vapor δ18O, δD, and d-excess at Dome C and then applied to investigate the degree of atmosphere–snow water vapor exchange and the associated isotope effects at Dome A at diurnal scales. The model results show that atmosphere–snow water vapor exchange at Dome A can also lead to similar diurnal isotope variations in atmospheric water vapor δ18O and δD under summer conditions, with corresponding diurnal variations in surface snow δ18O and δD. For clear-sky conditions, the magnitudes of the diurnal cycles in snow and water vapor isotopes are greater than those in simulations under highly cloudy conditions. In addition, we performed diurnal simulations under Dome A winter conditions. The results indicate that the diurnal isotope variations over the 24 h simulation period are less significant due to the stable atmospheric conditions with low and relatively stable air temperature and specific humidity. However, the model results suggest that snow isotope depletion can occur in winter. The modeled opposite isotope effects on snow after 24 h in winter and summer at Dome A suggest that atmosphere–snow water vapor exchange could increase the seasonal snow isotope variations. The modeled changes in winter are smaller than those in summer due to the highly stable boundary layer conditions in winter. This means that the effects in summer cannot be offset by those in winter, leading to overall enrichments of snow isotopes.
We also acknowledge the limitations inherent to our simulations with a one-dimensional model. The air mass exchange process between the free-atmosphere layer and boundary layer may play an important role in atmosphere–snow water vapor exchange as observed during some frost events (Casado et al., 2018). Although the influence of the free atmosphere has been incorporated into our model, it is worth refining the underlying assumptions for the air mass exchange process and improving the accuracy of the model simulations. Further, observational validation of the model results for the winter season is unavailable due to extremely harsh conditions at Dome A. Although it is currently difficult to conduct fieldwork at the diurnal scale there, observations at longer timescales (e.g., weekly resolved sampling of surface snow and precipitation over a year along with a snowpack to reconstruct the changes after deposition) could be possible. These results are important to validate the model's ability to predict the associated isotope effects of atmosphere–snow water vapor exchange, especially considering that the model implies that atmosphere–snow water vapor exchange may have few isotope effects at the annual scale but tends to increase snow water isotope seasonality. The latter is opposite to other post-depositional processes such as wind-driven ventilation (Town et al., 2008) and vapor diffusion in snow pores (Johnsen et al., 2000).
The simulated data and model code are available upon request to Tianming Ma (email: mtm@ustc.edu.cn). Other data and software used in this study are also available online. We also acknowledge the use of Dome C data from the CALVA project and CENECLAM and GLACIOCLIM observatories (https://doi.org/10.1594/PANGAEA.932512; Genthon et al., 2021a, and https://doi.org/10.1594/PANGAEA.932513; Genthon et al., 2021b). Meteorological observations at Dome A can be downloaded from the Australian Antarctic Data Centre (https://doi.org/10.26179/brjy-g225; Heil et al., 2017). The hourly averages of total cloud cover and longwave radiative fluxes were sourced from the ERA5 reanalysis dataset (https://doi.org/10.24381/cds.adbb2d47; Hersbach et al., 2023).
The supplement related to this article is available online at: https://doi.org/10.5194/tc-18-4547-2024-supplement.
LG and TM conceptualized this study. TM and PH performed the model simulations, analyzed the data, and wrote the paper with LG. LG and ZJ provided help with the model construction. MD, WZ, and YL provided available data for the simulations. All the authors contributed to data interpretation and writing.
At least one of the (co-)authors is a member of the editorial board of The Cryosphere. The peer-review process was guided by an independent editor, and the authors also have no other competing interests to declare.
Publisher's note: Copernicus Publications remains neutral with regard to jurisdictional claims made in the text, published maps, institutional affiliations, or any other geographical representation in this paper. While Copernicus Publications makes every effort to include appropriate place names, the final responsibility lies with the authors.
The authors are grateful for the data provided by the CALVA project and meteorological observations collected by Chinese National Antarctic Research Expedition during the summers of 2005–2011. The authors are also grateful to the editor Benjamin Smith and the reviewers including Hongxi Pang, Zoe R. Courville, and three anonymous experts for their constructive suggestions and comments, which improved the paper.
This research has been supported by the National Natural Science Foundation of China (grant no. 42206242 awarded to Tianming Ma, grant no. 42206245 awarded to Pengzhen He), the Open Fund of the State Key Laboratory of Cryospheric Science (grant no. SKLCS-OP-2020-06), the Natural Science Research Project of Anhui Province (grant no. 2108085QD158), and the Fundamental Research Funds for the Central Universities. Lei Geng received financial support from the National Natural Science Foundation of China (grant nos. W2411030 and 41822605), the Fundamental Research Funds for Central Universities, the Strategic Priority Research Program of Chinese Academy of Sciences (grant no. XDB 41000000), and the National Key R&D Program of China (grant no. 2019YFC1509100). This research was also supported in part by National Natural Science Foundation of China (grant nos. 49973006, 40773074, and 40703019 awarded to Yuansheng Li) and the Ministry of Science and Technology of China (grant no. 2006BAB18B01).
This paper was edited by Benjamin Smith and reviewed by Hongxi Pang, Zoe R. Courville, and three anonymous referees.
An, C., Hou, S., Jiang, S., Li, Y., Ma, T., Curran, M. A. J., Pang, H., Zhang, Z., Zhang, W., Yu, J., Liu, K., Shi, G., Ma, H., and Sun, B.: The long-term cooling trend in East Antarctic Plateau over the past 2000 years is only robust between 550 and 1550 CE, Geophys. Res. Lett., 48, e2021GL092923, https://doi.org/10.1029/2021GL092923, 2021.
Berkelhammer, M., Noone, D. C., Steen-Larsen, H. C., Bailey, A., Cox, C. J., O'Neill, M. S., Schneider, D., Steffen, K., and White, J. W. C.: Surface-atmosphere decoupling limits accumulation at Summit, Greenland, Sci. Adv., 2, e1501704, https://doi.org/10.1126/sciadv.1501704, 2016.
Berkowicz, R. and Prahm, L. P.: Evaluation of the profile method for estimation of surface fluxes of momentum and heat, Atmos. Environ., 16, 2809–2819, https://doi.org/10.1016/0004-6981(82)90032-4, 1982.
Bonner, C. S., Ashley, M. C. B., Cui, X., Feng, L., Gong, X., Lawrence, J. S., Luong-Van, D. M., Shang, Z., Storey, J. W. Y., Wang, L., Yang, H., Zhou, X., and Zhu, Z.: Thickness of the atmospheric boundary layer above Dome A, Antarctica, during 2009, Publ. Astron. Soc. Pac., 122, 1122–1131, https://doi.org/10.1086/656250, 2010.
Bréant, C., Leroy Dos Santos, C., Agosta, C., Casado, M., Fourré, E., Goursaud, S., Masson-Delmotte, V., Favier, V., Cattani, O., Prié, F., Golly, B., Orsi, A., Martinerie, P., and Landais, A.: Coastal water vapor isotopic composition driven by katabatic wind variability in summer at Dumont d'Urville, coastal East Antarctica, Earth Planet. Sc. Lett., 514, 37–47, https://doi.org/10.1016/j.epsl.2019.03.004, 2019.
Brun, E., Six, D., Picard, G., Vionnet, V., Arnaud, L., Bazile, E., Boone, A., Bouchard, A., Genthon, C., Guidard, V., Moigne, P. L., Rabier, F., and Seity, Y.: Snow/atmosphere coupled simulation at Dome C, Antarctica, J. Glaciol., 57, 721–736, https://doi.org/10.3189/002214311797409794, 2011.
Casado, M., Landais, A., Masson-Delmotte, V., Genthon, C., Kerstel, E., Kassi, S., Arnaud, L., Picard, G., Prie, F., Cattani, O., Steen-Larsen, H.-C., Vignon, E., and Cermak, P.: Continuous measurements of isotopic composition of water vapour on the East Antarctic Plateau, Atmos. Chem. Phys., 16, 8521–8538, https://doi.org/10.5194/acp-16-8521-2016, 2016.
Casado, M., Landais, A., Picard, G., Münch, T., Laepple, T., Stenni, B., Dreossi, G., Ekaykin, A., Arnaud, L., Genthon, C., Touzeau, A., Masson-Delmotte, V., and Jouzel, J.: Archival processes of the water stable isotope signal in East Antarctic ice cores, The Cryosphere, 12, 1745–1766, https://doi.org/10.5194/tc-12-1745-2018, 2018.
Casado, M., Münch, T., and Laepple, T.: Climatic information archived in ice cores: impact of intermittency and diffusion on the recorded isotopic signal in Antarctica, Clim. Past, 16, 1581–1598, https://doi.org/10.5194/cp-16-1581-2020, 2020.
Champollion, N., Picard, G., Arnaud, L., Lefebvre, É., Macelloni, G., Rémy, F., and Fily, M.: Marked decrease in the near-surface snow density retrieved by AMSR-E satellite at Dome C, Antarctica, between 2002 and 2011, The Cryosphere, 13, 1215–1232, https://doi.org/10.5194/tc-13-1215-2019, 2019.
Craig, H.: Isotope Variations in Meteoric Waters, Science, 133, 1702–1703, https://doi.org/10.1126/science.133.3465.1702, 1961.
Dansgaard, W.: Stable isotopes in precipitation, Tellus, 16, 436–468, https://doi.org/10.1111/j.2153-3490.1964.tb00181.x, 1964.
Dansgaard, W., Johnsen, S., Clausen, H., and Gundestrup, N.: Stable isotope glaciology, Meddelelser om Gronland, 197, 1–53, 1973.
Dietrich, L. J., Steen-Larsen, H. C., Wahl, S., Jones, T. R., Town, M. S., and Werner, M.: Snow-Atmosphere Humidity Exchange at the Ice Sheet Surface Alters Annual Mean Climate Signals in Ice Core Records, Geophys. Res. Lett., 50, e2023GL104249, https://doi.org/10.1029/2023GL104249, 2023.
Ding, M., Xiao, C., Yang, Y., Wang, Y., Li, C., Yuan, N., Shi, G., Sun, W., and Ming, J.: Re-assessment of recent (2008–2013) surface mass balance over Dome Argus, Antarctica, Polar Res., 35, 26133, https://doi.org/10.3402/polar.v35.26133, 2016.
Ding, M., Zou, X., Sun, Q., Yang, D., Zhang, W., Bian, L., Lu, C., Allison, I., Heil, P., and Xiao, C.: The PANDA automatic weather station network between the coast and Dome A, East Antarctica, Earth Syst. Sci. Data, 14, 5019–5035, https://doi.org/10.5194/essd-14-5019-2022, 2022.
Ebner, P. P., Steen-Larsen, H. C., Stenni, B., Schneebeli, M., and Steinfeld, A.: Experimental observation of transient δ18O interaction between snow and advective airflow under various temperature gradient conditions, The Cryosphere, 11, 1733–1743, https://doi.org/10.5194/tc-11-1733-2017, 2017.
Ekaykin, A. A., Vladimirova, D. O., Lipenkov, V. Y., and Masson-Delmotte, V.: Climatic variability in Princess Elizabeth Land (East Antarctica) over the last 350 years, Clim. Past, 13, 61–71, https://doi.org/10.5194/cp-13-61-2017, 2017.
Ellehoj, M. D., Steen-Larsen, H. C., Johnsen, S. J., and Madsen, M. B.: Ice-vapor equilibrium fractionation factor of hydrogen and oxygen isotopes: experimental investigations and implications for stable water isotope studies, Rapid Commun. Mass Sp., 27, 2149–2158, https://doi.org/10.1002/rcm.6668, 2013.
EPICA community members: Eight glacial cycles from an Antarctic ice core, Nature, 429, 623–628, https://doi.org/10.1038/nature02599, 2004.
Frezzotti, M., Pourchet, M., Flora, O., Gandolfi, S., Gay, M., Urbini, S., Vincent, C., Becagli, S., Gragnani, R., Proposito, M., Severi, M., Traversi, R., Udisti, R., and Fily, M.: New estimations of precipitation and surface sublimation in East Antarctica from snow accumulation measurements, Clim. Dynam., 23, 803–813, https://doi.org/10.1007/s00382-004-0462-5, 2004.
Fujita, K. and Abe, O.: Stable isotopes in daily precipitation at Dome Fuji, East Antarctica, Geophys. Res. Lett., 33, L18503, https://doi.org/10.1029/2006gl026936, 2006.
Genthon, C., Town, M. S., Six, D., Favier, V., Argentini, S., and Pellegrini, A.: Meteorological atmospheric boundary layer measurements and ECMWF analyses during summer at Dome C, Antarctica, J. Geophys. Res.-Atmos., 115, D05104, https://doi.org/10.1029/2009JD012741, 2010.
Genthon, C., Veron, D., Vignon, E., Six, D., Dufresnes; J.-L., Sultan, E., and Forget, F.: Ten years of shielded ventilated atmospheric temperature observation on a 45-m tower at Dome C, East Antarctic plateau, East Antarctic plateau, PANGAEA [data set], https://doi.org/10.1594/PANGAEA.932512, 2021a.
Genthon, C., Veron, D., Vignon, E., Six, D., Dufresnes, J.-L., Sultan, E., and Forget, F.: Ten years of wind speed observation on a 45-m tower at Dome C, East Antarctic plateau, PANGAEA [data set], https://doi.org/10.1594/PANGAEA.932513, 2021b.
Haynes, J. M., Vonder Haar, T. H., L'Ecuyer, T., and Henderson, D.: Radiative heating characteristics of earth's cloudy atmosphere from vertically resolved active sensors, Geophys. Res. Lett., 40, 624–630, https://doi.org/10.1002/grl.50145, 2013.
Heil, P., Hyland, G., and Alison, I.: Automatic Weather Station Data obtained at Dome A (Argus), Antarctica, Ver. 1, Australian Antarctic Data Centre [data set], https://doi.org/10.26179/brjy-g225, 2017.
Hersbach, H., Bell, B., Berrisford, P., Biavati, G., Horányi, A., Muñoz Sabater, J., Nicolas, J., Peubey, C., Radu, R., Rozum, I., Schepers, D., Simmons, A., Soci, C., Dee, D., and Thépaut, J.-N.: ERA5 hourly data on single levels from 1940 to present, Copernicus Climate Change Service (C3S) Climate Data Store (CDS) [data set], https://doi.org/10.24381/cds.adbb2d47, 2023.
Hoshina, Y., Fujita, K., Nakazawa, F., Iizuka, Y., Miyake, T., Hirabayashi, M., Kuramoto, T., Fujita, S., and Motoyama, H.: Effect of accumulation rate on water stable isotopes of near-surface snow in inland Antarctica, J. Geophys. Res.-Atmos., 119, 274–283, https://doi.org/10.1002/2013jd020771, 2014.
Hou, S., Wang, Y., and Pang, H.: Climatology of stable isotopes in Antarctic snow and ice: Current status and prospects, Chinese Sci. Bull., 58, 1095–1106, https://doi.org/10.1007/s11434-012-5543-y, 2012.
Hu, J., Yan, Y., Yeung, Y., and Dee, S.: Sublimation Origin of Negative Deuterium Excess Observed in Snow and Ice Samples from McMurdo Dry Valleys and Allan Hills Blue Ice Areas, East Antarctica, J. Geophys. Res.-Atmos., 127, e2021JD035950, https://doi.org/10.1029/2021JD035950, 2022.
Hu, Z., Shi, G., Talalay, P., Li, Y., Fan, X., An, C., Zhang, N., Liu, K., Yu, J., Yang, C., Li, B., Liu, B., and Ma, T.: Deep ice-core drilling to 800 m at Dome A in East Antarctica, Ann. Glaciol., 62, 293–304, https://doi.org/10.1017/aog.2021.2, 2021.
Hughes, A. G., Wahl, S., Jones, T. R., Zuhr, A., Hörhold, M., White, J. W. C., and Steen-Larsen, H. C.: The role of sublimation as a driver of climate signals in the water isotope content of surface snow: laboratory and field experimental results, The Cryosphere, 15, 4949–4974, https://doi.org/10.5194/tc-15-4949-2021, 2021.
Jiang, S., Cole-Dai, J., Li, Y., Ferris, D. G., Ma, H., An, C., Shi, G., and Sun, B.: A detailed 2840 year record of explosive volcanism in a shallow ice core from Dome A, East Antarctica, J. Glaciol., 58, 65–75, https://doi.org/10.3189/2012JoG11J138, 2012.
Johnsen, S. J., Clausen, H. B., Cuffey, K. M., Hoffmann, G., Schwander, J., and Creyts, T.: Diffusion of stable isotopes in polar firn and ice: the isotope effect in firn diffusion, in: Physics of ice core records, pp. 121–140, Hokkaido University Press, 2000.
Jouzel, J. and Merlivat, L.: Deuterium and oxygen 18 in precipitation: Modeling of the isotopic effects during snow formation, J. Geophys. Res.-Atmos., 89, 11749–11757, https://doi.org/10.1029/JD089iD07p11749, 1984.
Laepple, T., Münch, T., Casado, M., Hoerhold, M., Landais, A., and Kipfstuhl, S.: On the similarity and apparent cycles of isotopic variations in East Antarctic snow pits, The Cryosphere, 12, 169–187, https://doi.org/10.5194/tc-12-169-2018, 2018.
Liu, J., Du, Z., Zhang, D., and Wang, S.: Diagnoses of Antarctic inland water cycle regime: Perspectives from atmospheric water vapor isotope observations along the transect from Zhongshan Station to Dome A, Front. Earth Sci., 10, 823515, https://doi.org/10.3389/feart.2022.823515, 2022.
Louis, J.: A parametric model of vertical eddy fluxes in the atmosphere, Bound.-Lay. Meteorol., 17, 187–202, https://doi.org/10.1007/BF00117978, 1979.
Ma, B., Shang, Z., Hu, Y., Hu, K., Wang, Y., Yang, X., Ashley, M. C. B., Hickson, P., and Jiang, P.: Night-time measurements of astronomical seeing at Dome A in Antarctica, Nature, 583, 771–774, https://doi.org/10.1038/s41586-020-2489-0, 2020.
Ma, T., Li, L., Li, Y., An, C., Yu, J., Ma, H., Jiang, S., and Shi, G.: Stable isotopic composition in snowpack along the traverse from a coastal location to Dome A (East Antarctica): Results from observations and numerical modelling, Polar Sci., 24, 100510, https://doi.org/10.1016/j.polar.2020.100510, 2020.
Ma, Y., Bian, L., Xiao, C., Allison, I., and Zhou, X.: Near surface climate of the traverse route from Zhongshan Station to Dome A, East Antarctica, Antarct. Sci., 22, 443–459, https://doi.org/10.1017/s0954102010000209, 2010.
Madsen, M. V., Steen-Larsen, H. C., Horhold, M., Box, J., Berben, S. M. P., Capron, E., Faber, A. K., Hubbard, A., Jensen, M. F., Jones, T. R., Kipfstuhl, S., Koldtoft, I., Pillar, H. R., Vaughn, B. H., Vladimirova, D., and Dahl-Jensen, D.: Evidence of Isotopic Fractionation During Vapor Exchange Between the Atmosphere and the Snow Surface in Greenland, J. Geophys. Res.-Atmos., 124, 2932–2945, https://doi.org/10.1029/2018JD029619, 2019.
Markle, B. R. and Steig, E. J.: Improving temperature reconstructions from ice-core water-isotope records, Clim. Past, 18, 1321–1368, https://doi.org/10.5194/cp-18-1321-2022, 2022.
Masson-Delmotte, V., Hou, S., Ekaykin, A., Jouzel, J., Aristarain, A., Bernardo, R. T., Bromwich, D., Cattani, O., Delmotte, M., Falourd, S., Frezzotti, M., Gallée, H., Genoni, L., Isaksson, E., Landais, A., Helsen, M. M., Hoffmann, G., Lopez, J., Morgan, V., Motoyama, H., Noone, D., Oerter, H., Petit, J. R., Royer, A., Uemura, R., Schmidt, G. A., Schlosser, E., Simões, J. C., Steig, E. J., Stenni, B., Stievenard, M., van den Broeke, M. R., van de Wal, R. S. W., van de Berg, W. J., Vimeux, F., and White, J. W. C.: A review of Antarctic surface snow isotopic composition: observations, atmospheric circulation, and isotopic modeling, J. Climate, 21, 3359–3387, https://doi.org/10.1175/2007jcli2139.1, 2008.
Merlivat, L. and Jouzel, J.: Global climatic interpretation of the deuterium-oxygen 18 relationship for precipitation, J. Geophys. Res.-Oceans, 84, 5029, https://doi.org/10.1029/JC084iC08p05029, 1979.
Monin, A. S. and Obukhov, A. M.: Basic laws of turbulent mixing in the atmosphere near the ground, Tr. Geophiz. Inst. Akad. Nauk. SSSR, 24, 163–187, 1954.
Neumann, T. A., Albert, M. R., Engel, C., Courville, Z., and Perron, F.: Sublimation rate and the mass-transfer coefficient for snow sublimation, Int. J. Heat Mass Tran., 52, 309–315, https://doi.org/10.1016/j.ijheatmasstransfer.2008.06.003, 2009.
Pang, H., Hou, S., Landais, A., Masson-Delmotte, V., Jouzel, J., Steen-Larsen, H. C., Risi, C., Zhang, W., Wu, S., Li, Y., An, C., Wang, Y., Prie, F., Minster, B., Falourd, S., Stenni, B., Scarchilli, C., Fujita, K., and Grigioni, P.: Influence of Summer Sublimation on δD, δ18O, and δ17O in Precipitation, East Antarctica, and Implications for Climate Reconstruction from Ice Cores, J. Geophys. Res.-Atmos., 124, 7339–7358, https://doi.org/10.1029/2018JD030218, 2019.
Passey, B. H. and Levin, N. E.: Triple Oxygen Isotopes in Meteoric Waters, Carbonates, and Biological Apatites: Implications for Continental Paleoclimate Reconstruction, Rev. Mineral. Geochem., 86, 429–462, https://doi.org/10.2138/rmg.2021.86.13, 2021.
Petit, J. R., Jouzel, J., Raynaud, D., Barkov, N. I., Barnola, J. M., Basile, I., Bender, M., Chappellaz, J., Davis, M., and Delaygue, M.: Climate and atmospheric history of the past 420 000 years from the Vostok ice core, Antarctica, Nature, 399, 429–436, https://doi.org/10.1038/20859, 1999.
Qian, Y., Long, C. N., Wang, H., Comstock, J. M., McFarlane, S. A., and Xie, S.: Evaluation of cloud fraction and its radiative effect simulated by IPCC AR4 global models against ARM surface observations, Atmos. Chem. Phys., 12, 1785–1810, https://doi.org/10.5194/acp-12-1785-2012, 2012.
Ritter, F., Steen-Larsen, H. C., Werner, M., Masson-Delmotte, V., Orsi, A., Behrens, M., Birnbaum, G., Freitag, J., Risi, C., and Kipfstuhl, S.: Isotopic exchange on the diurnal scale between near-surface snow and lower atmospheric water vapor at Kohnen station, East Antarctica, The Cryosphere, 10, 1647–1663, https://doi.org/10.5194/tc-10-1647-2016, 2016.
Sokratov, S. A. and Golubev, V. N.: Snow isotopic content change by sublimation, J. Glaciol., 55, 823–828, https://doi.org/10.3189/002214309790152456, 2009.
Steen-Larsen, H. C., Johnsen, S. J., Masson-Delmotte, V., Stenni, B., Risi, C., Sodemann, H., Balslev-Clausen, D., Blunier, T., Dahl-Jensen, D., Ellehøj, M. D., Falourd, S., Grindsted, A., Gkinis, V., Jouzel, J., Popp, T., Sheldon, S., Simonsen, S. B., Sjolte, J., Steffensen, J. P., Sperlich, P., Sveinbjörnsdóttir, A. E., Vinther, B. M., and White, J. W. C.: Continuous monitoring of summer surface water vapor isotopic composition above the Greenland Ice Sheet, Atmos. Chem. Phys., 13, 4815–4828, https://doi.org/10.5194/acp-13-4815-2013, 2013.
Stenni, B., Scarchilli, C., Masson-Delmotte, V., Schlosser, E., Ciardini, V., Dreossi, G., Grigioni, P., Bonazza, M., Cagnati, A., Karlicek, D., Risi, C., Udisti, R., and Valt, M.: Three-year monitoring of stable isotopes of precipitation at Concordia Station, East Antarctica, The Cryosphere, 10, 2415–2428, https://doi.org/10.5194/tc-10-2415-2016, 2016.
Stenni, B., Curran, M. A. J., Abram, N. J., Orsi, A., Goursaud, S., Masson-Delmotte, V., Neukom, R., Goosse, H., Divine, D., van Ommen, T., Steig, E. J., Dixon, D. A., Thomas, E. R., Bertler, N. A. N., Isaksson, E., Ekaykin, A., Werner, M., and Frezzotti, M.: Antarctic climate variability on regional and continental scales over the last 2000 years, Clim. Past, 13, 1609–1634, https://doi.org/10.5194/cp-13-1609-2017, 2017.
Sun, B., Siegert, M., Mudd, S., Sugden, D., Fujita, S., and Cui, X.: The Gamburtsev mountains and the origin and early evolution of the Antarctic Ice Sheet, Nature, 459, 690–693, https://doi.org/10.1038/nature08024, 2009.
Surma, J., Assonov, S., and Staubwasser, M.: Triple Oxygen Isotope Systematics in the Hydrologic Cycle, Rev. Mineral. Geochem., 86, 401–428, https://doi.org/10.2138/rmg.2021.86.12, 2021.
Touzeau, A., Landais, A., Stenni, B., Uemura, R., Fukui, K., Fujita, S., Guilbaud, S., Ekaykin, A., Casado, M., Barkan, E., Luz, B., Magand, O., Teste, G., Le Meur, E., Baroni, M., Savarino, J., Bourgeois, I., and Risi, C.: Acquisition of isotopic composition for surface snow in East Antarctica and the links to climatic parameters, The Cryosphere, 10, 837–852, https://doi.org/10.5194/tc-10-837-2016, 2016.
Touzeau, A., Landais, A., Morin, S., Arnaud, L., and Picard, G.: Numerical experiments on vapor diffusion in polar snow and firn and its impact on isotopes using the multi-layer energy balance model Crocus in SURFEX v8.0, Geosci. Model Dev., 11, 2393–2418, https://doi.org/10.5194/gmd-11-2393-2018, 2018.
Town, M. S., Warren, S. G., Walden, V. P., and Waddington, E. D.: Effect of atmospheric water vapor on modification of stable isotopes in near-surface snow on ice sheets, J. Geophys. Res., 113, D24303, https://doi.org/10.1029/2008JD009852, 2008.
Van Liefferinge, B., Pattyn, F., Cavitte, M. G. P., Karlsson, N. B., Young, D. A., Sutter, J., and Eisen, O.: Promising Oldest Ice sites in East Antarctica based on thermodynamical modelling, The Cryosphere, 12, 2773–2787, https://doi.org/10.5194/tc-12-2773-2018, 2018.
Vignon, E., Genthon, C., Barral, H., Amory, C., Picard, G., Gallée, H., Casasanta, G., and Argentini, S.: Momentum- and Heat-Flux Parametrization at Dome C, Antarctica: A Sensitivity Study, Bound.-Lay. Meteorol., 162, 341–367, https://doi.org/10.1007/s10546-016-0192-3, 2017.
Wahl, S., Steen-Larsen, H. C., Reuder, J., and Hörhold, M.: Quantifying the Stable Water Isotopologue Exchange Between the Snow Surface and Lower Atmosphere by Direct Flux Measurements, J. Geophys. Res.-Atmos., 126, e2020JD034400, https://doi.org/10.1029/2020jd034400, 2021.
Wahl, S., Steen-Larsen, H. C., Hughes, G., Dietrich, L., Zuhr, A., Behrens, M., Faber, A.-K., and Hörhold, M.: Atmosphere-Snow Exchange Explains Surface Snow Isotope Variability, Geophys. Res. Lett., 49, e2022GL099529, https://doi.org/10.1029/2022GL099529, 2022.
WAIS Divide project members: Onset of deglacial warming in West Antarctica driven by local orbital forcing, Nature, 500, 440–444, https://doi.org/10.1038/nature12376, 2013.
Wang, Y., Sodemann, H., Hou, S., Masson-Delmotte, V., Jouzel, J., and Pang, H.: Snow accumulation and its moisture origin over Dome Argus, Antarctica, Clim. Dynam., 40, 731–742, https://doi.org/10.1007/s00382-012-1398-9, 2012.
Werner, M., Langebroek, P. M., Carlsen, T., Herold, M., and Lohmann, G.: Stable water isotopes in the ECHAM5 general circulation model: Toward high-resolution isotope modeling on a global scale, J. Geophys. Res.-Atmos., 116, D15109, https://doi.org/10.1029/2011jd015681, 2011.
Zilitinkevich, S. S. and Esau, I. N.: Similarity theory and calculation of turbulent fluxes at the surface for the stably stratified atmospheric boundary layer, Bound.-Lay. Meteorol., 125, 193–205, https://doi.org/10.1007/s10546-007-9187-4, 2007.