the Creative Commons Attribution 4.0 License.
the Creative Commons Attribution 4.0 License.
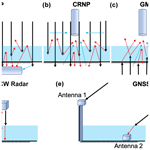
Review article: Performance assessment of radiation-based field sensors for monitoring the water equivalent of snow cover (SWE)
Alain Royer
Alexandre Roy
Sylvain Jutras
Alexandre Langlois
Continuous and spatially distributed data of snow mass (water equivalent of snow cover, SWE) from automatic ground-based measurements are increasingly required for climate change studies and for hydrological applications (snow hydrological-model improvement and data assimilation). We present and compare four new-generation sensors, now commercialized, that are non-invasive and based on different radiations that interact with snow for SWE monitoring: cosmic-ray neutron probe (CRNP), gamma ray monitoring (GMON) scintillator, frequency-modulated continuous-wave radar (FMCW radar) at 24 GHz and global navigation satellite system (GNSS) receivers (GNSSr). All four techniques have relatively low power requirements, provide continuous and autonomous SWE measurements, and can be easily installed in remote areas. A performance assessment of their advantages, drawbacks and uncertainties is discussed from experimental comparisons and a literature review. Relative uncertainties are estimated to range between 9 % and 15 % when compared to manual in situ snow surveys that are also discussed. Results show the following. (1) CRNP can be operated in two modes of functioning: beneath the snow, it is the only system able to measure very deep snowpacks (> 2000 mm w.e.) with reasonable uncertainty across a wide range of measurements; CRNP placed above the snow allows for SWE measurements over a large footprint (∼ 20 ha) above a shallow snowpack. In both cases, CRNP needs ancillary atmospheric measurements for SWE retrieval. (2) GMON is the most mature instrument for snowpacks that are typically up to 800 mm w.e. Both CRNP (above snow) and GMON are sensitive to surface soil moisture. (3) FMCW radar needs auxiliary snow-depth measurements for SWE retrieval and is not recommended for automatic SWE monitoring (limited to dry snow). FMCW radar is very sensitive to wet snow, making it a very useful sensor for melt detection (e.g., wet avalanche forecasts). (4) GNSSr allows three key snowpack parameters to be estimated simultaneously: SWE (range: 0–1000 mm w.e.), snow depth and liquid water content, according to the retrieval algorithm that is used. Its low cost, compactness and low mass suggest a strong potential for GNSSr application in remote areas.
- Article
(2179 KB) - Full-text XML
-
Supplement
(328 KB) - BibTeX
- EndNote
Snow cover on the ground surface plays an important role in the climate system due to its high albedo, heat insulation that affects the ground thermal regime, and contribution to snow runoff and soil moisture (Meredith et al., 2019). The water equivalent of snow cover (SWE, its mass per unit area) not only is expressed in kilogram per square metre (kg m−2) but also is commonly shown in units of millimetres of water equivalent (mm w.e). It is an essential climate variable (ECV) for monitoring climate change, as recognized by the Global Climate Observing System (GCOS-WMO, 2016; https://gcos.wmo.int/en/essential-climate-variables, last access: 25 October 2021), which aligns with the World Meteorological Organization's (WMO) Global Cryosphere Watch initiative (Key et al., 2016; https://globalcryospherewatch.org, last access: 25 October 2021). SWE monitoring is also of primary importance for hydrological forecasting and preventing flooding risks over snowmelt-dominated basins in mountainous and cold-climate regions. Snow station distributions are generally sparse in high-latitude regions, remote areas and high mountains (Bormann et al., 2013; Key et al., 2015, 2016; Pirazzini et al., 2018; Haberkorn, 2019; Brown et al., 2019, 2021; Royer et al., 2021), given that monitoring is generally based upon expensive and occasional (weekly to monthly) manual sampling. Automation of SWE measurement networks is an essential medium-term prospect, especially since reliable and automatic instrument alternatives exist (Dong, 2018; this study).
Various in situ field devices and approaches for measuring the temporal dynamics of SWE are available, all of which have their strengths and limitations (see the review by Rasmussen et al., 2012; Kinar and Pomeroy, 2015; Pirazzini et al., 2018). Some are invasive (i.e., destroying the snowpack or changing its properties), while others that are based on different remotely sensed approaches are non-invasive. Here, we focus on a new generation of radiation-based field sensors that directly measure SWE, i.e., measuring a signal that is proportional to the snow mass per unit area. In this study, we do not consider sensors that are based on pressure and load cell sensors (snow pillows), snowmelt lysimeters, dielectric sensors (e.g., the SNOWPOWER system, commercially available as the Snow Pack Analyzer) or acoustic sensors (see Kinar and Pomeroy, 2015). We do not consider indirect approaches, such as those based on snow-depth monitoring, combined with a model of snow density evolution (Yao et al., 2018). We also exclude satellite-based approaches.
The objective of this paper, therefore, is to present a performance review of four selected non-invasive sensors (Table 1), viz., the cosmic-ray neutron probe (CRNP), the gamma ray monitoring (GMON) scintillator, frequency-modulated continuous-wave radar (FMCW radar) and global navigation satellite system (GNSS) receivers (GNSSr). All four approaches have common features: easy to install, low power (e.g., powered by solar panels), provide continuous and autonomous SWE measurements, and deployable in remote areas. The continuous or quasi-continuous SWE measurement capability is defined here relative to the application, such as for seasonal SWE monitoring, for hydrological-model validation or for following a relatively short winter storm event. Surface-based radar scatterometers and microwave radiometers have not been considered in this study because (1) they are still in early stages of development or are currently not operational and (2) they have heavy maintenance demands (not autonomous) and are still relatively expensive. These include, for example, scatterometers (Werner et al., 2010; Wiesmann et al., 2010; King et al., 2015; Werner et al., 2019), microwave radiometers (Langlois, 2015; Roy et al., 2016, 2017; Wiesmann et al., 2021), radar interferometers (Werner et al., 2010; Leinss et al., 2015; Pieraccini and Miccinesi, 2019; GPRI brochure, 2021) and stepped-frequency continuous-wave radar (SFCW) instruments (Alonso et al., 2021).
Table 1SWE sensors that were studied and acronyms that were used. FMCW: frequency-modulated continuous-wave radar. GNSS: global navigation satellite system, including GPS (Global Positioning System, USA), GLONASS (Russia), Galileo (Europe) and BeiDou (China) satellite constellations. The frequency of the electromagnetic (EM) wave that was used and their approximate maximum water equivalent of snow (SWEmax) measurement limit capabilities are given. SD: snow depth. See Fig. 1 for measurement principle conceptualization and Fig. 2 for photos.
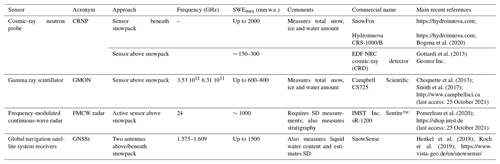
Section 2 provides background information on the basic principles of each of the four sensors that are presented in Table 1. Examples of SWE temporal series comparisons from four different instruments that were acquired in Quebec, eastern Canada, are given in Sect. 3.1 and 3.2: comparisons between EDF's (Électricité de France) CRNP (NRC sensor; nivomètre à rayonnement cosmique) and GMON on one hand and GNSSr, FMCW radar and GMON on the other hand. This permits performance evaluations for each system, including uncertainty analysis, compared to manual SWE measurements. We complement these uncertainty assessments with a review of additional results from previous studies (Sect. 3.3 and Table 2). Advantages and drawbacks of these sensors are then discussed in Sect. 4 (Table 3). Listing of references cited in this article sorted by the sensor name: CNRP, FMCW-Radar, GNSSr, GMON, Radar, Radiometer and Snow core can be found in the Supplement.
The main characteristics of the four reviewed sensors are summarized in Table 1, with the acronym that is used to denote them, together with their commercial names. There are two operation modes for the cosmic-ray neutron probe (CRNP); thus, five cases were considered. All of these sensors allow for quasi-continuous measurements throughout the winter without maintenance and are powered by solar panels and batteries. The measuring principles of each of the instruments are illustrated in Fig. 1 and shown in Fig. 2. In this section, we only recall the main principles of functioning and the key elements of SWE retrieval, given that all sensors are well described in detail in the cited references.
Aspects that are related to the measurement scale of each sensor are critical to SWE measurements, since SWE is generally highly variable spatially, depending upon the ecosystem and terrain (Kinar and Pomeroy, 2015; Dong, 2018). These questions are discussed in Sect. 4.
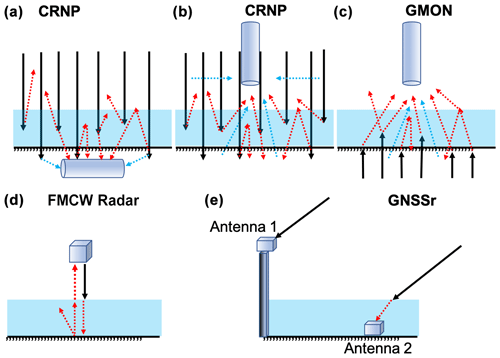
Figure 1Diagram of radiation paths for the five approaches (see Table 1). In all figures, black arrows correspond to natural (a, b, c) or emitted (d, e) signals, and dotted red arrows correspond to rays interacting with snow (the lower the signal reaching the sensor is, the higher the SWE is). The footprint of the sensor is defined by the area from which emanates the measured radiation having interacted with the snow. (a) Cosmic-ray neutron probe (CRNP) below the snow, buried in the ground. In this case, black arrows are ambient neutrons generated primarily by interactions of secondary cosmic-ray neutrons with terrestrial and atmospheric nuclei. Dotted red arrows are neutrons interacting with snow, which decrease when SWE increases. Dotted blue arrows are neutrons interacting with soil moisture. (b) CRNP above the snow, looking downward. Same as (a) for the arrow meanings, but dotted blue arrows are neutrons interacting with soil and atmospheric moisture. (c) Gamma ray monitoring (GMON) sensor. Same as (a) for the arrow meanings. (d) Frequency-modulated continuous-wave radar (FMCW radar) looking downward above the snow. The black arrows are the radar-emitted wave at 24 GHz. (e) Global navigation satellite system (GNSS) receivers. The two antennas receive signals emitted by all of the GNSS satellites in the antennas' field of view and at all incidence angles: only one incident ray (black arrow) at one angle is shown. According to the inversion algorithm, different rays that interact with the snow (dotted red arrows) are used. For the SnowSense system, independent measurements at antenna 1 and antenna 2 are analyzed.
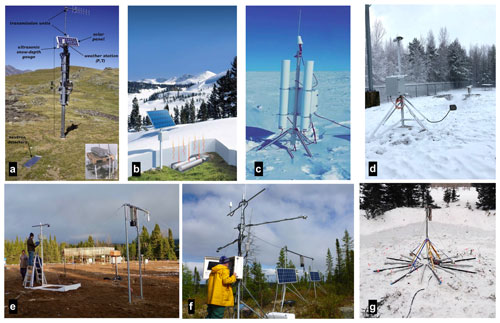
Figure 2Photographs of sensors that were analyzed. (a) Cosmic-ray neutron probe (CRNP) from the EDF French network (nivomètre à rayonnement cosmique, NRC) at the Lac Noir station in Ecrins-Pelvoux massif, France. One can see the neutron probe buried in the ground (also shown in inset) and the mast, which carries ancillary meteorological sensors. Credit: Delunel et al. (2014). (b) SnowFox CRNP set at ground level beneath the snow cover. Similar to (a), the system requires measurements of atmospheric conditions. Credit: Hydroinnova SnowFox manual. (c) Same sensor as in (b), but the Hydroinnova CRS-1000/B sensor is placed above the snow, measuring ambient and upward neutron counts, with the latter being attenuated by the snowpack. Credit: Philip Marsh, Wilfrid Laurier University, Waterloo, ON, Canada; sensor in the tundra at Trail Valley Creek, Changing Cold Regions Network (http://ccrnetwork.ca, last access: 25 October 2021; Jitnikovitch et al., 2021). (d) GNSSr installed at the Université de Sherbrooke SIRENE (Site interdisciplinaire de recherche en environnement extérieur) site. The antenna that was placed on the ground (beneath the snow) was made visible at 3 m from the mast, on top of which a second antenna was affixed. Credit: Alain Royer. (e) The FMCW radar (on the left) and the GMON scintillator (on the right) at the NEIGE-FM (NEIGE-Forêt Montmorency) site. A metallic plate on the ground in the field of view of the radar substantially increases radar echoes. In the background of panel (e), one can see the solid precipitation gauge, which is known as the Double Fence Intercomparison Reference (DFIR). Credit: Alain Royer. (f) Meteorological and snow (GMON) automatic station at the Le Moyne–James Bay, Quebec, Canada, site in a sub-Arctic environment (Prince et al., 2019). Credit: Alain Royer. (g) The GMON scintillator at the NEIGE-Forêt Montmorency site set up to boost 40K counts with pipes filled with potassium fertilizer. Credit: Sylvain Jutras.
2.1 Cosmic-ray neutron probe (CRNP)
CRNP measurement is based on the moderation of ambient neutrons by hydrogen in water, snow and ice. The intensity of natural low-energy cosmic-ray neutron emission is inversely correlated with the amount of hydrogen in the soil (Zreda et al., 2008; Andreasen et al., 2017) or snow cover (Desilets et al., 2010; Gottardi et al., 2013; Sigouin and Si, 2016; Gugerli et al., 2019; Bogena et al., 2020). Even though the principle of this approach has been known since the 1970s, it attained a level of operational maturity in the 2000s, especially with the use of commercialized soil moisture probes. Électricité de France (EDF) successfully used a network of cosmic-ray probes (denoted nivomètre à rayonnement cosmique, NRC; this sensor is composed of two neutron detector tubes filled with helium-3, 3He) that were buried under the snowpack to measure SWE for more than a decade in the French Alps and in the Pyrenees (Fig. 2a, sensor placed at 3.5 m from a 6 m mast) (Paquet and Laval, 2006; Paquet et al., 2008; Gottardi et al., 2013; Delunel et al., 2014). Ephemeral, shallow snow cover across the UK is monitored by the COSMOS-UK network of 46 sites equipped with the CRNP Hydroinnova CRS-2000 or CRS-1000/B models (https://cosmos.ceh.ac.uk, last access: 25 October 2021; Evans et al., 2016).
There are two experimental approaches for CRNP-based SWE monitoring (Fig. 1a, b): (1) with the probe at the ground level beneath the snow (such as EDF's NRC, Fig. 2a, and the SnowFox sensor for Hydroinnova, Fig. 2b) or (2) with the probe placed a few metres above the snow surface (Fig. 1b), such as the one proposed by Hydroinnova (Fig. 2c) (CRS-1000/B, Hydroinnova, Albuquerque, NM, USA; http://hydroinnova.com/snow_water.html, last access: 25 October 2021). Using a dual-channel set-up, the system is composed of two detector tubes filled with 10BF3; one is sensitive to neutrons with a maximum energy of ∼ 0.025 eV, whereas the second is sensitive to moderated energy neutrons from ∼ 0.2 eV to 100 keV. The cosmic-ray probe above the snowpack (Fig. 1b) is an attractive SWE measurement tool because it can provide direct estimates of SWE within a 20 to 40 ha measurement area, footprint (20 ha corresponds to a circle of 252 m radius) (Desilets and Zreda, 2013; Schattan et al., 2017). In contrast, the footprint of a probe that is installed under the snow is limited to a spot measurement above the sensor (Fig. 1a). While approach 1a permits measurements of very thick snow cover (> 1000 mm w.e.) (Gugerli et al., 2019), the drawback of approach 1b is that it is limited to low SWE measurements (typically < 150 mm w.e.) over homogeneous flat terrain. However, in the Austrian Alps, contrary to previous studies, Schattan et al. (2017) claim not to have measured saturation for a snowpack of the order of 600 mm w.e., over an estimated footprint with 230 m radius.
The CRNP method requires creating a function for converting neutron counts to SWE (Paquet et al., 2008; Gottardi et al., 2013; Sigouin and Si, 2016; Andreasen et al., 2017; Schattan et al., 2017; Delunel et al., 2014; Bogena et al., 2020). Desilets (2017) provides the calibration procedure in detail. Neutron counts must be accumulated over a specified period of time (e.g., from 6 to 24 h). The CRNP method requires that the counting rate must first be known (calibrated) and that disturbance effects on measured cosmic-rays at the site location have to be taken into account. Disturbance effects that need to be corrected include temporal variations in the natural cosmic-ray flux and variations in on-site measurements of air pressure and atmospheric water vapour during the count time. Temporal variation in cosmic-ray flux can be determined from the NMDB database (Real-Time Database for high-resolution Neutron Monitor measurements; https://www.nmdb.eu/, last access: 25 October 2021), thereby providing access to reference neutron monitor measurements from stations around the world. Corrections for air pressure (linked to the altitude of the station) and variations of atmospheric water vapour require ancillary standard meteorological sensors, which measure atmospheric pressure, air temperature and relative humidity.
While accuracy losses that are linked to atmospheric disturbances (pressure and humidity corrections) are relatively weak (a few percent), this is not the case for primary variations in the natural cosmic-ray flux (Andreasen et al., 2017), which may drastically change the results of SWE estimation. This flux can vary up to 30 % over long periods (weeks to months), thereby causing errors up to 50 % in SWE estimates when they are not considered (Paquet and Laval, 2006). Therefore, it is important to correct the measured signal using the closest world reference station in the vicinity of the measurement site. If not available, a second cosmic-ray sensor is required to produce accurate SWE estimates using normalized signals (above and beneath snow) as done by the cosmic-ray detector commercialized by Geonor Inc. (https://geonor.com/live/, last access: 25 October 2021).
In the case of the second approach, where the probe is installed above the ground surface (Fig. 1b), the probe must be calibrated for soil moisture. If soil moisture correction is not applied on the winter signal measurements, retrieved SWE values will be systematically overestimated. This bias can be corrected using measurements of CRNP signal without snow, just prior to the onset of snow cover, or using soil moisture probe during the winter (see Sect. 4).
2.1.1 Gamma ray scintillator (GMON)
Monitoring SWE by using natural soil radioactivity is a well-known approach (Bissell and Peck, 1973). Since 1980, an airborne snow survey program using this technology has successfully collected mean areal-SWE data for operational flood forecasting over the whole of northwestern North America, including the Rocky Mountains, Alaska and Great Plains (National Operational Hydrologic Remote Sensing Center, https://www.nohrsc.noaa.gov/snowsurvey/, last access: 25 October 2021). The mean area-SWE value is based on the difference between gamma radiation measurements over bare ground and snow-covered ground, the latter being attenuated by the snowpack (Carroll, 2001).
The principle of SWE measurements that are based on the gamma ray monitor (GMON) scintillator is the absorption by the water, regardless of its phase (liquid, snow or ice) of the natural radioactive emission of potassium-40 (40K) from soils (Ducharme et al., 2015). This naturally occurring radioactive isotope of potassium has a gamma emission of 1.46 MeV. The GMON probe also measures the emission of thallium-208 (208Tl), which emits gamma rays at a slightly higher energy (2.61 MeV) that originate from the decay of thorium-232 (Choquette et al., 2013; Wright, 2013; Stranden et al., 2015). Both of these elements are common to almost all types of surfaces, regardless of whether these are organic or non-organic soils. However, we observed that the isotope associated with the higher count (i.e., 40K) is generally the most reliable.
The GMON scintillator, which is manufactured by Campbell Scientific (Canada) (CS725; http://www.campbellsci.ca/cs725, last access: 25 October 2021), is composed of a tube 62 cm long and 13 cm in diameter, weighing 9 kg. The experimental set-up, which is illustrated in Fig. 1c, is based on the initial, snow-free measurement of the number of counts for 40K or 208Tl per period of time, which would be later decreased by the presence of the snowpack. Typically, 300 000 and 60 000 counts per 24 h for 40K and 208Tl, respectively, are suggested as minimal values to provide accurate SWE measurements (CS725 Snow Water Equivalent Instruction Manual, 2017, Campbell Scientific (Canada) Corporation, Edmonton, AB; https://s.campbellsci.com/documents/ca/manuals/cs725_man.pdf, last access: 25 October 2021). The observed rate of soil emission at each site allows the operator to define the minimum sampling time frequency. Seeding experiments conducted using potassium fertilizer show the potential for increasing potassium counts that are measured by the CS725 by up to 80 % at sites where low counts are found (Wright et al., 2011). As is the case for ground-pointing CRNP, measuring the baseline signal of the radiation energy emanating from the ground prior to the first snowfall is a critical step in signal processing, given that it also depends upon soil moisture (SM) during the winter and spring periods. SM attenuates the natural dry-ground emission, resulting in an overestimate of SWE during signal processing when SM increases (Choquette et al., 2013) (see Sect. 4).
The Campbell CS725 GMON sensor has been the subject of a detailed performance analysis within the framework of the WMO Solid Precipitation Intercomparison Experiment (Smith et al., 2017). Moreover, since the device is sensitive to water contained in soils, it can be successfully used to estimate soil moisture during snow-free seasons. An operational GMON network, with a sampling frequency of 6 h, is actually deployed across the southern part of Quebec and Labrador, northeastern Canada (45–55∘ N); it accounts for 116 stations that are operated by Hydro-Québec (87), Rio Tinto's hydropower (bauxite–aluminum smelters) (13), Ministère de l'Environnement et de la Lutte contre les changements climatiques of the government of Quebec (10), Parks Canada (4), and the government of Newfoundland and Labrador (2) and which are dedicated to water resource forecasting (Alexandre Vidal, Hydro-Québec, personal communication, November 2020). Also, these continuous measurements from the GMON Quebec network are demonstrably very useful for validating the assimilation of microwave observations into a snow model (Larue et al., 2018). Recently, GMON had also demonstrated its robustness in a research project on seasonal snow monitoring from a station that was installed at 4962 m a.s.l. in the Nepalese Himalayas (Langtang Valley) to quantify the evolution of SWE (Kirkham et al., 2019).
2.2 FMCW radar (FMCW radar)
The principle of frequency-modulated continuous-wave (FMCW) radar has been well known since the 1970s (see the reviews by Peng and Li, 2019, and by Pomerleau et al., 2020) and has been popularized for snow studies since Koh et al. (1996), Marshall et al. (2005), and Marshall and Koh (2008), among others, were published. FMCW radar is an active system design for distance measurements. The radar emits a wave at variable frequencies that are centered on a reference frequency. When the radar receives a return from a target, the frequency difference between the emitted and reflected signals is measured (Fig. 1d). Since the frequency change rate is known, the time between the emission and the reception of the echo can be measured, from which the radar–target distance is calculated.
The principle of SWE retrieval is based on the time measurement of wave propagation in the snowpack that is proportional to the snow refractive index (square of permittivity), which changes the wave-speed propagation. As the refractive index of snow can be linked to its density (Tiuri et al., 1984; Matzler, 1996; Pomerleau et al., 2020), SWE can be retrieved knowing the snow depth. The experimental set-up is shown in Fig. 1d and illustrated in Fig. 2e.
Two main FMCW radar specifications are required for SWE measurement: the radar central frequency and its bandwidth that is scanned. The central frequency specifies three parameters: (a) the loss in signal strength of an electromagnetic wave that would result from a line-of-sight path through free space (the higher the frequency, the greater the loss), (b) its penetration depth (the higher the frequency, the less penetration power it has) and (c) its sensitivity to liquid water content in the snowpack. The bandwidth specifies the distance resolution and, thus, the precision: the wider the bandwidth, the lower the resolution. There is negligible frequency dependency of the snow refractive index (n′), which governs wave propagation in the snowpack. The refractive index (n′) is linked to snow density (ρ) by a linear relationship: (Pomerleau et al., 2020).
For snow studies, several FMCW radars with different frequencies and resolutions are used, such as those common at the X-band (10 GHz), operating over 8–12 GHz (Ellerbruch and Boyne, 1980; Marshall and Koh, 2008). They provide a vertical resolution on the order of 3 cm. In contrast, L-band FMCW radar (1.12–1.76 GHz) allows for greater penetration but suffers from reduced vertical resolution (Yankielun et al., 2004). Multiband FMCW radars have also been developed (Rodriguez-Morales et al., 2014), such as an L-/C-band (2–8 GHz) one that was used to successfully retrieve snow depth (Fujino et al., 1985), a C-/Ku-band (8–18 GHz) large wideband FMCW radar that is capable of detecting crusts as thin as 0.2 mm within the snowpack (Marshall and Koh, 2005) or the improved (C-, X- and Ka-band) radar (Koh et al., 1996). Operating frequencies of commercial, low-cost radar systems, such as those that are adopted for automotive radar systems (Schneider, 2005), are now available for K-band (24 GHz) and W-band (77 GHz) applications.
The availability of such new types of lightweight and very compact 24 GHz FMCW radar systems has motivated our research group to assess their ability to monitor the SWE continuously and autonomously (Fig. 2e) (Pomerleau et al., 2020). The FMCW radar that is used, which is centered on 24 GHz (K-band), is manufactured by IMST (IMST Sentire™, IMST, Kamp-Lintfort, Germany; http://www.radar-sensor.com/, last access: 25 October 2021; IMST, 2021); its housing module is very compact (114.0 mm × 87.0 mm × 42.5 mm) and weighs 280 g. This FMCW radar has a bandwidth of 2.5 GHz, scanning over 23–25.5 GHz, which provides a resolution of 6 cm in the air. These specifications appear to be a good compromise between penetration and resolution capabilities for SWE estimation while keeping the sensor affordable, light and compact, with low power consumption. The radar penetration depth (δPr) of dry snow significantly decreases with density following a power law, which varies with temperature (see Fig. A2; Pomerleau et al., 2020). At T= 0 ∘C, δPr decreases from 6.78 to 4.81, 3.26 and 2.05 m for respective snow densities of 150, 200, 275 and 400 kg m−3 (Pomerleau et al., 2020). Wet snow drastically reduces δPr, given that liquid water strongly absorbs the radar signal, leading to high reflectivity at the air–wet-snow interface, and weak transmissivity. For example, the two-way radar penetration depth decreases abruptly from 2 m for dry snow at a density of 400 kg m−3 to 0.05 m for wet snow with 0.5 % of liquid water content (as a volume fraction). It should be noted that this strong sensitivity to wet snow allows for the radar to precisely detect the onset of snowpack surface melt, a benefit that is discussed in Sect. 4.
One of the main interests of this approach is its potential capacity to estimate SWE from a small remotely piloted aircraft (RPA). Over the Arctic, snow cover can generally be characterized as a two-layer snowpack structure, which is composed of a dense wind slab overlaying a less dense depth hoar layer (Rutter et al., 2019; Royer et al., 2021). Thus, assumptions can be made regarding the mean refractive index of each of these layers, thereby allowing for SWE to be estimated (Pomerleau et al., 2020). Hu et al. (2019) also showed the usefulness of imaging FMCW synthetic aperture radar on board the RPA. Several studies have also shown the potential of FMCW radar for different applications, such as avalanche studies (Vriend et al., 2013; Okorn et al., 2014; Laliberté et al., 2021), snow stratigraphy based on successive FMCW echo analyses (Marshall and Koh, 2008; Marshall et al., 2007), snowpack tomography (Xu et al., 2018) and ice thickness monitoring (Yankielun et al., 1993; Gunn et al., 2015). Pomerleau et al. (2020) obtained highly accurate measurements of lake ice thickness using the 24 GHz FMCW radar, with a root-mean-square difference (RMSD) of 2 cm accuracy up to ≈ 1 m ice thickness (derived from 35 manual in situ measurements).
2.3 GNSS receivers (GNSSr)
The principle of SWE retrieval based on global navigation satellite system (GNSS) receivers is to use the signals that are emitted at 1.575 and 1.609 GHz by the GNSS satellite constellations. SWE can be related to the carrier phase change that is induced by the delay caused by the snowpack at ground level. With two static receivers (standard GNSS antennas), i.e., one placed under the snow and the other above the snow, carrier phase measurements of both receivers can be compared and SWE can be derived using the onboard measurement hardware (Fig. 1e) (Henkel et al., 2018). Comparing GNSS signal attenuation measurements at the two antennas (below and above the snowpack) also permits the retrieval of liquid water content (LWC) of the wet snow (Koch et al., 2019). Snow-depth retrieval has been operational for longer, based on interferometric reflectometry of GNSS signals (see Larson et al., 2009; Larson, 2016). Steiner et al. (2019) used a slightly simplified retrieval algorithm based on the path delay estimates of the GPS signals while propagating through the snow cover due to both refraction at the air–snow interface and decrease in wave velocity in the medium.
This relatively recent and novel approach has been validated (Henkel et al., 2018; Steiner et al., 2018; Koch et al., 2019; Appel et al., 2019). A system has now been commercialized by VISTA Remote Sensing in Geosciences GmbH, Munich, Germany (SnowSense©, https://www.vista-geo.de/en/snowsense/, last access: 25 October 2021). The experimental set-up is described in Fig. 1e, based on a low-cost and lightweight system. In this study, we used the SnowSense system for monitoring SWE and LWC throughout a winter, together with other sensors (see Results Sect. 3). We also developed our own system, shown in Fig. 2d.
Another promising way to monitor SWE, which is based on the same principle of GNSS, is the use of powerful satellite transmissions as illumination sources for bistatic radar. This so-called “signals-of-opportunity” (SoOp) approach covers a wide range of frequencies, such as emissions from United States Navy Ultra High Frequency (UHF) Follow-On (UFO) communication satellites in P-band frequencies (between 240–270 MHz). From two P-band antennas (one direct and one reflected), Shah et al. (2017) demonstrated the feasibility of retrieving SWE using the phase change in reflected waveforms, which is linearly related to the change in SWE. These methods were not included in this review, since they are still in the development stage and not sufficiently mature to be operational.
Continuous and simultaneous recordings of different instruments on different sites were analyzed to evaluate their behaviour in terms of their temporal evolution. Manual measurements were used to compare the data between them. First (Sect. 3.1 and 3.2), two experiments we conducted were compared: GMON and CRNP (Sect. 3.2.1) and GMON, radar and GNSSr (Sect. 3.2.2). A comprehensive literature review and evaluations of similar sensors are then presented in Sect. 3.3. This later section also includes uncertainty estimates of our experiments and from this review, which are synthesized in Table 2.
Table 2Uncertainty analysis for the four systems that were considered. The range measurement indicates the highest SWE (mm) value on which the analysis was performed. RMSD: root-mean-square difference. R2: determination coefficient of the linear regression analysis. Pts: number of in situ manual samples. –: no information available.
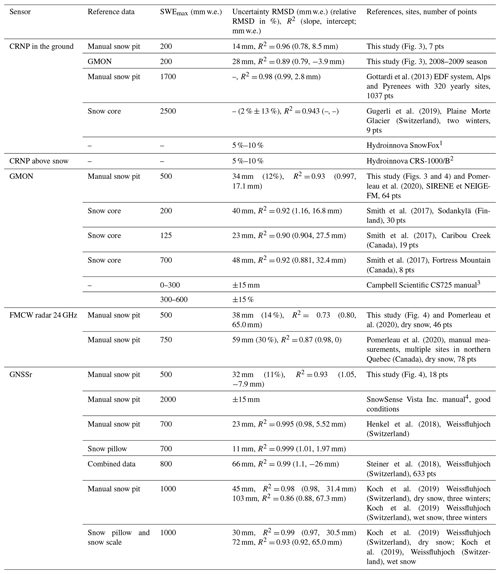
1 https://hydroinnova.com/_downloads/snowfox_v1.pdf (last access: 25 October 2021); Hydroinnova, Albuquerque, NM. 2 http://hydroinnova.com/snow_water.html (last access: 25 October 2021); Hydroinnova, Albuquerque, NM. 3 https://s.campbellsci.com/documents/ca/manuals/cs725_man.pdf (last access: 25 October 2021); Campbell Scientific (Canada) Corporation, CS725 manual. 4 https://www.vista-geo.de/en/snowsense/.
3.1 Experimental sites and methods
We compared four instruments at two snow research stations that were located in Quebec (Canada). The first was the SIRENE site (Site interdisciplinaire de recherche en environnement extérieur), which is situated on the main campus of the Université de Sherbrooke in a temperate forest environment (45.37∘ N, 71.92∘ W; 250 m a.s.l.) (Fig. 2d). The second site is the NEIGE-Forêt Montmorency (NEIGE-FM) research station. The instruments were located in an open area (Fig. 2e) of the Montmorency experimental forest (47.32∘ N, 71.15∘ W; 640 m a.s.l.) of Université Laval (Québec City), which is in the boreal forest. The NEIGE-FM snow research station is part of the World Meteorological Organization's (WMO) Global Cryosphere Watch (GCW) surface network, CryoNet (http://globalcryospherewatch.org/cryonet/sitepage.php?surveyid=191, last access: 25 October 2021).
Two methods were used to obtain in situ manual SWE measurements in the vicinity of the four SWE systems: the snow pit (SP) approach and snow tube core samplers (see Kinar and Pomeroy, 2015; López-Moreno et al., 2020). The SP-based SWE values (in mm w.e., i.e., kg m−2) were derived from vertical continuous density profiles, which were determined by weighing snow samples at a vertical resolution of 5 cm (height of the density cutter). Assuming an accuracy of density cutter measurements of about 9 % (Proksch et al., 2016), the mean relative SWE accuracy from a snow pit can be estimated to be of 6 %–12 %. SWE estimates were also obtained by weighing the extracted core sample of a known diameter (∅) and snow depth using a coring tube. In this study, the core sampling was performed using three different snow tube models, which were averaged: “Carpenter” (Federal standard sampler, 3.7 cm ∅ tube), the Hydro-Québec snow tube (12.07 cm ∅) and an in-house Université Laval snow tube (15.24 cm ∅). The uncertainty of tube core sampling that we carried out on snowpack up to 600 mm w.e. with large tubes on the order of 6 % but can be higher, up to 12 %. Such uncertainty is difficult to define, as discussed in Sect. 3.3 and in the Appendix. Furthermore, as manual measurements cannot be taken at the same location throughout a given winter period, this could generate uncertainty when compared to a fixed instrument, due to small-scale spatial variability of SWE and surface roughness (López-Moreno et al., 2020).
The snowpack properties were derived from GMON and CRNP systems throughout the entire winter season of 2008–2009 (Fig. 3) and from GMON, FMCW radar and GNSSr systems in 2017–2018 (Fig. 4). The CRNP probe that was used was the same as the French EDF probe that was placed on the ground (Paquet et al., 2008) and installed at about 5 m distance from the GMON footprint. The GMON scintillator was installed on a 2 m mast above the surface, located in a slight depression in comparison with the terrain where the CRNP was buried. The CRNP counts were accumulated over 1 h and normalized against an identical probe that was installed nearby, just above the snow surface. The GMON counts were accumulated over 6 h, and only 40K counts were considered (TI counts were similar but are not shown). The GMON sensor was adjusted to take into account the soil moisture prior to snowfall accumulation but not afterwards.
In addition to SWE measurements, continuous automatic snow-depth measurements were performed using an ultrasonic ranging sensor (Campbell Scientific SR50AT-L) and manually with a graduated probe around the sampling sites. LWC measurements were derived from GNSSr (Fig. 4). Air temperature (T) at 2 m height and total daily precipitation (tipping bucket rain gauge) were recorded at the SIRENE site; a threshold of T= 0 ∘C was used to separate solid and liquid phases.
In this section, we present comparisons between these sensors with manual snow pit validation data that were measured as close as possible to the automatic instruments. The uncertainty of measurements, including other measurements that we carried out (not shown), is reported in Table 2.
3.2 Validation of measurements
3.2.1 Comparison of GMON- and CRNP-derived SWE seasonal evolution
Figure 3 shows the SWE evolution of a shallow snowpack (maximum snow depth of 56 cm) at the SIRENE site that was derived from daily mean values of the GMON and CRNP data throughout the winter season of 2008–2009.
Results show that GMON and CRNP evolve similarly over the winter, with GMON SWE being slightly higher after the first winter month (SWE > 50 mm). This difference occurred after a pronounced melting spell (29–30 December 2008) and is explained by the water that has accumulated on the ground under the GMON scintillator and not on the CRNP, due to the local terrain configuration. The moisture beneath the GMON scintillator formed a significant ice layer that lasted all winter. As this ice layer was not present in snow pits (the amount of water in an ice crust being otherwise difficult to measure), this could possibly explain differences between GMON and manual measurements. Precipitation data (snowfall and rain) show how GMON and CRNP evolve with each event (Fig. 3). But for studying short meteorological events, the measurement period linked to a given instrument should be short enough to be able to account for rapid changes in SWE. This can be seen in Fig. 3, which shows higher variability in SWE derived from CRNP based on counts accumulated over 1 h than those derived from GMON based on counts accumulated over 6 h. Moreover, small snowfalls on top of a thick (denser or wet) snowpack were not always detected. Further studies are needed to address challenges related to sub-daily reliability of these instruments.
For that given winter, rain-on-snow events were frequent, leading to moisture accumulation on the ground. Note also that at the end of the winter, there was ice that had not yet melted and water accumulation under the GMON scintillator, resulting in a significant GMON overestimation in terms of SWE but not in terms of total water (snow plus ice). There was no more snow on the ground after 20 March 2009. The accuracy measurements are discussed in Sect. 4.2.
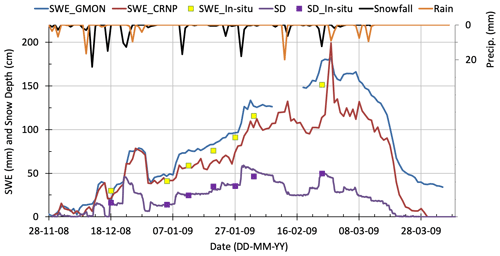
Figure 3GMON- and CRNP-derived water equivalent of snow cover (SWE, mm w.e.), snow depth (SD, cm), and recorded daily solid and liquid precipitation (precip., mm, right-hand scale), in comparison to validation data (in situ) at the SIRENE site for the winter season of 2008–2009. Continuous SD measurements (purple line) are from SR50AT-L, and SD in situ measurements (purple square; SD_In-Situ) are from snow pits. Open yellow squares correspond to manual in situ SWE measurements.
3.2.2 Comparison of GMON-, radar- and GNSSr-derived SWE seasonal evolution
Figure 4 shows the SWE evolution that was measured by the three instruments: GMON (40K counts only), FMCW radar and GNSSr, which had been placed in close proximity to one another at the NEIGE-FM research station for the winter season of 2017–2018. A maximum snow depth of 120 cm was measured during the season, corresponding to an SWE maximum of 500 mm w.e. at the end of April.
The three instruments were compared to manual in situ measurements that had been derived from SP (red squares) and core (red triangles) approaches in Fig. 4. We distinguished the two methods (SP and snow core) because they exhibit significant differences, with an RMSD of 33 mm (12 %). These discrepancies are the result of two problems: (1) SWE spatial variability, mainly due to snow-depth variability (López-Moreno et al., 2020), and (2) the method that was used, since the design of snow tubes and cutters has some influence on sampling errors and bias (Goodison et al., 1987; see Appendix). Therefore, uncertainty analyses (Sect. 3.3) were performed considering manual SP as the reference because the SP approach was used for both experiments.
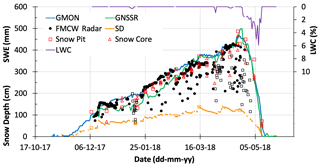
Figure 4GMON- (blue line), FMCW-radar- (closed black circles) and GNSSr-derived (green line) water equivalent of snow cover (SWE, mm w.e.); snow depth (orange line for SR50AT-L data and orange open squares for in situ data) (SD, cm); and GNSSr-derived liquid water content (LWC, % volumetric, purple line, right scale), in comparison to in situ snow pit (open red square) and snow core (open red triangle) SWE measurements at the NEIGE-FM site for the winter season of 2017–2018. For FMCW radar data (in black), plain circles are for dry snow, while open squares correspond to wet snow.
The continuous simultaneous recordings from the different instruments permit temporal evolution analysis (Fig. 4). During the accumulation period, the GMON scintillator shows relatively smooth and consistent evolution in SWE leading to a maximum of 465 mm on 19 April 2018, while the FMCW radar time series is more erratic and requires filtering to remove low SWE outliers. These points are mainly due to incorrect detection of the peak of the radar echo on the ground (snow–ground interface), sometimes with low amplitude, and which can be filtered with improved data quality processing of raw recordings (Pomerleau et al., 2020). In particular, all data that were acquired under wet-snow conditions (open black squares, Fig. 4), which correspond to melting periods with measured air temperature above 0 ∘C, are obviously underestimated as expected because of radar wave absorption by liquid water in the snowpack. Compared to the GMON scintillator, the GNSSr signal increases with values that are lower than the GMON scintillator until mid-March, at which point it continues to evolve with similar values, as the GMON SWEmax of 499 mm w.e. was reached on 23 April 2018. The behaviour of the three instruments, showing different patterns of snow evolution, always remains close to in situ observations (RMSE compared to the snow pit for GMON and GNSSr are, respectively, 34 and 32 mm; Table 2). It should be noted that in Fig. 4, there is a small difference (+4 d) between the disappearance of snow cover that was recorded with GNSSr (11 May 2018) compared to GMON (14 May 2018). The GNSSr sensor is not sensitive to soil moisture, while GMON is, despite the instruments being located on a well-drained sandy site (NEIGE-FM site). In the case shown here, the end of snowmelt is well captured by both instruments. The accuracy between instruments is analyzed in Sect. 3.4, including a second winter season of continuous measurements at the NEIGE-FM site (2016–2017, Pomerleau et al., 2020).
GNSSr also measures the liquid water content (LWC) of snow (purple line in Fig. 4). The non-zero LWC values correspond well to positive air temperatures that were recorded at this site and also to the drop in FMCW radar measurements (open black squares).
3.3 Analysis of measurement uncertainty
It is challenging to compare the accuracy of several instruments, given that there is no absolute reference for estimating SWE (see Kinar and Pomeroy, 2015). In situ manual measurements are themselves subject to error, with varying precision depending upon the method that is being used. Errors are incurred that depend upon the types of density cutter, tube diameter, sampling quality that is operator dependent and ice lenses in the snowpack, among other sources. This is a long-debated topic, with no actual established international standard protocol (Work et al., 1965; Goodison et al., 1981, 1987; Kinar and Pomeroy, 2015; López-Moreno et al., 2020). Commonly, the relative uncertainty for SWE measurement using snow core varies from 6 % for shallow snowpack (0–300 mm w.e.) to 8 % (300–1000 mm w.e.) for medium snowpack to 10 %–12 % for deeper snowpack (> 1000 mm w.e.) (see discussion in Appendix). Moreover, because manual measurements cannot be taken at the same location during a given winter period, uncertainty can be introduced by well-known local spatial variability in snow depth that can occur at fine scales around the sensors. Such variability depends upon several factors, such as the region and the environment (Arctic area and aspect and slope in mountainous areas, for example), the micro-topography and roughness, the vegetation, and snow redistribution by the wind (Clark et al., 2011; Bormann et al., 2013; Rutter et al., 2014; Meloche et al., 2021; Royer et al., 2021). Furthermore, temporal variability of snow depth and SWE during the winter requires regular validation measurements throughout the season.
The sensor uncertainties were evaluated from results of our experiments (Sect. 3.2) and from published studies at other experimental comparison sites (this section). These other sites are the Weissfluhjoch high-Alpine site near Davos, Switzerland (46.83∘ N, 9.81∘ E; 2 536 m a.s.l.); Sodankylä, Finland (67.37∘ N, 26.63∘ E; 185 m a.s.l.); Caribou Creek, SK, Canada (53.95∘ N, 104.65∘ W; 519 m a.s.l.); and the Fortress Mountain ski area, Kananaskis Country, Canadian Rocky Mountains, AB (50.82∘ N, 115.20∘ W; 2330 m a.s.l.). We also conducted a series of manual FMCW radar measurements (e.g., instrument operated by hand, rather than automatically) over dry snowpack and compared them with in situ SWE measurements over a wide range of conditions (snow depth and density) in boreal-forest (47∘ N, 18 points), sub-Arctic taiga (54–56∘ N, 32 points) and Arctic tundra (69∘ N, 28 points) environments along a northeastern Canadian transect (Pomerleau et al., 2020).
Note that we only consider here the differences between instruments in the field and do not address accuracies that were derived from instrument calibration by the manufacturer.
Table 2 summarizes the uncertainties of each instrument and protocol (five cases: CRNP in and above ground, GMON, FMCW radar, and GNSSr) in relation to in situ manual measurements (snow pit method), as well as against snow pillow and snow scale data that were considered reference measurements by the authors of the publications consulted. The results from the COSMOS-UK network (Wallbank et al., 2021) were not included in the overall uncertainty analysis because, in this study, depth-based SWE estimate of fresh snow was used to assess the uncertainty of CRNP (R2 of 0.53, in the range of 0–40 mm w.e.). Moreover, soil moisture is usually high and variable in the UK, which acts to increase uncertainties in the SWE estimate (Wallbank et al., 2021).
Even if the mechanical method is well known and has been proven over many years, the snow pillow can sometimes generate large errors when bridging processes occur that are linked to freeze–thaw cycles leading to disconnection of the weighing mechanism of the overlying snowpack and the surrounding snowpack (Kinar and Pomeroy, 2015). However, to compare measurements at a daily scale, they are worth looking at. In Table 2, the uncertainty that relates to the characterization of measurement dispersion compared to a reference was defined, when known. We used the root-mean-square difference (RMSD) between an instrument and a given reference and by a linear regression over the whole range of measured SWE data that were defined by the coefficient of determination (R2), the slope and the intercept. The number of points is also given.
Uncertainty analysis does not allow us to determine the “best” instrument, due to the diversity of experimental conditions, including the range of SWE, the number of experimental sites and point measurements, and the analyses that are performed over one or several seasons. It appears that all five methods show an RMSD in the range of 14 to 48 mm (mean 33±11 mm) against in situ snow pit manual measurements (Table 2). This represents a relative value of around 12 % on average, depending on the instruments. The mean coefficient of determination for the linear regression is also substantially high (mean R2= 0.92 ± 0.07). Calculated average slope is 0.976 ± 0.13, meaning that in general, the instruments slightly underestimate SWE for higher SWE values compared to in situ measurements, even if this is not always the case (Table 2). RMSD increases slightly when the analysis was performed over a deep snowpack (0–1000 mm w.e.) and decreases when compared to another continuous instrument instead of manual data (instrument vs. GMON and instrument vs. snow pillow; average RMSD = 23 ± 10 mm, Table 2).
For the GNSSr instrument that allows the operator to differentiate dry from wet snow, Koch et al. (2019) have shown that SWE RMSD is about 2.4-fold higher for wet snow than for dry snow. They did not provide information on LWC uncertainty. In late winter 2021, for very wet melting snow, we did a validation measurement using the WISe A2 Photonic probe (snow liquid water content sensor that is based on snow microwave permittivity measurements; https://a2photonicsensors.com/wise/, last access: 25 October 2021). The GNSSr LWC was of 0.44 % (in volume) (the retrieved GNSSr SWE was 149 mm w.e), and the LWC from the in situ probe was of 0.47 % for the upper half of the snowpack. The snowpack SWE that was measured manually was 133 mm. The lower half of the snowpack was saturated with water. The uncertainty in wet SWE retrieval could result from approximations in the retrieval algorithm that is used. For example, the wet-snow refractive index varies linearly with LWC, with a slope significantly dependent on the snow density (see the Appendix of Pomerleau et al., 2020). This aspect could probably be addressed further by improved inversion.
The uncertainty comparison in Table 2 must be weighted according to the analysis conditions. The accuracy estimates can actually depend upon the number of points being used and their distribution over time. High interannual variability of the snowpack state (see Bormann et al., 2013; Lejeune et al., 2019) ideally would necessitate several years of measurements over the winter. The uncertainties of each GMON and CRNP instrument were derived from huge datasets that were based on operational networks from the GMON Hydro-Québec network in Canada and the Alps' EDF network for the CRNP, respectively, with a very large number of samples taken over several years of experiments and from multiple sites. The accuracy of the GMON scintillator that is given by the manufacturer is ±15 mm for SWE < 300 mm and ±15 % for SWE of 300–600 mm, which is probably rather conservative. When SWE reference data and site adjustment processes are well done, the GMON scintillator is able to report SWE with an uncertainty as low as 5 % (Wright, 2011; Choquette et al., 2013; Wright et al., 2013). The accuracy of the SnowFox sensor (CRNP) that has been provided by the manufacturer (5 %–10 %) must be confirmed. The GNSSr approach has recently been the subject of two different comparative analyses showing very promising results (Henkel et al., 2018; Koch et al., 2019), which were confirmed by our own results. Over a full season, we obtained an excellent relationship between GNSSr and in situ manual measurements (relative RMSD = 11 %, Table 2) and compared with GMON (RMSD = 34 mm, 12 %, SWEGNSSr= 1.126 SWEGMON−59.3, R2= 0.97, 153 d).
In this section, we review the advantages and drawbacks of each of the instruments that are presented, summarized in Table 3. This analysis is based on our experience on instruments and their performances and a literature review on experimental results of measurements that were carried out with the same approaches. We only consider these field sensors for SWE measurements in terms of their continuous and autonomous capacities, from the perspective of an operational networking context, including criteria regarding low maintenance and relatively easy installation without requiring heavy infrastructure. The four instruments that we analyzed are CRNP with two experimental set-ups, i.e., instrument in the ground and above the snow; GMON; 24 GHz FMCW radar; and GNSSr (see Table 1 for acronyms and Fig. 1 for the experimental set-up). They are all capable of working on batteries and solar panels, by adjusting, if necessary in certain cases, the measurement protocol, i.e., by reducing the frequency of acquisition and onboard data processing. The following 10 criteria were considered (Table 3): the SWEmax capability; other measured parameters; whether ancillary data were required for SWE retrieval; the temporal sampling rate, i.e., whether they were capable of quasi-continuous SWE measurement capability, although the notion of continuous SWE measurements is relative to the application; the footprint of the sensor, i.e., taken here in the sense of the area from which emanates the measured radiation having interacted with the snow; the power consumption; the main strength of the approach; their critical drawbacks; the price of the instrument itself, knowing that the cost of the system may vary in case additional instruments are required for the SWE measurements, and the cost that is associated with on-site maintenance during winter should be considered here, but in our case, the four instruments are considered on the same basis, i.e., autonomous, with no need for intervention; and the possibility of other applications.
Table 3Pros and cons of the four systems that were considered for SWE monitoring. SM: soil moisture. FOV: field of view. LWC: liquid water content. RPA: remotely piloted aircraft. The approximate price is given (2021) but is subject to change according to exchange rate fluctuations.
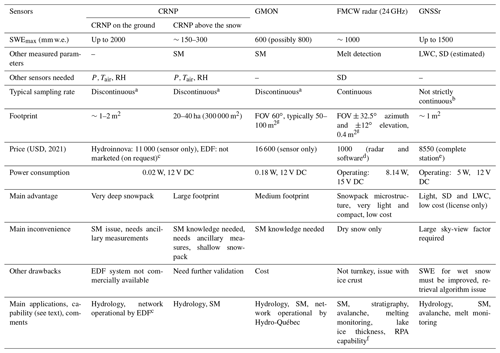
a Counts must be accumulated over a specified period, e.g., 6 h, 12 h or longer. b GNSS signals must be averaged over a period of time for noise reduction. The typical measurement cycle: 1 d−1 (possibly up to 6 d−1). c System based on a sensor that is not commercialized. d Software for sensor settings and reading/recording data but not for SWE retrievals. e Subscription license required. f Remotely piloted aircraft capability. g Depending on the height of the sensor on its support mast above snow, footprint given for 3 m mast.
The cost criterion is a very relative argument, which can influence the choice of decision-makers or researchers, depending upon the intended application (e.g., large network, in remote areas, among others) and also on the purchasers.
To complement the main criteria that are presented in Table 3, we include the following additional considerations, which are reported in the literature, by order of presentation rather than order of merit.
The CRNP approach is based on the neutron component that has an absorption mean free path about an order of magnitude larger than that for gamma radiation. This makes it the most efficient system for very deep snowpack analysis (Paquet et al., 2008). Measurements of snowpack of up to 2000 mm w.e. were performed using the SnowFox sensor at the UC Berkeley (University of California, Berkeley) Central Sierra Snow Lab in Soda Springs, CA (2120 m a.s.l.; https://vcresearch.berkeley.edu/research-unit/central-sierra-snow-lab, last access: 25 October 2021).
Regarding CRNP above the snow, Schattan et al. (2017) estimated the theoretical winter footprint over snow, which they defined as the distance from where neutrons originate. They found that 86 %, 63 % and 50 % of neutrons originate within respective distances of 273, 102 and 49 m. In practice, the authors found that the average footprint during the season, based on measurements over almost three snow seasons, was estimated to be around 230 m, possibly more.
Moreover, CRNP is inherently weakly sensitive to interference from vegetation compared to systems that are based on low EM frequencies (GMON, FMCW radar and GNSSr). This is in part because the attenuation coefficient for fast neutrons (∼ 0.01 m−2 kg in water, Murray and Holbert, 2020) is an order of magnitude smaller than the analogous attenuation coefficient in vegetation for GNSS microwaves (1.5 GHz) (e.g., Wigneron et al., 2017). Also, vegetation can itself be a significant source of electromagnetic emissions (Larson et al., 2014; Wigneron et al., 2017). The CRNP is affected by all sources of hydrogen within its measurement footprint. As biomass increases the hydrogen concentration in the CRNP's footprint, it is possible to monitor changes in biomass (Vather et al., 2020).
The instruments pointing toward the soil, CRNP and GMON above the surface, are sensitive to soil moisture. This can be a relatively large source of error with these measurement principles, given that these sensors are interpreting near-surface soil liquid content as SWE. This is especially the case during spring freshets and mid-season thaw cycles (see Fig. 3 and Smith et al., 2017). Heavy rainfall on snow also leads to erroneous SWE estimates due to the occurrence of water ponding beneath the snow (Fig. 3). Installation on well-drained soils can mitigate these effects, as shown in Fig. 4. By assuming that soil moisture levels remain stable throughout winter, which can be the case when soil remains frozen (see Gray et al., 1985, 2001), this soil moisture-induced bias can be adjusted prior to the first snowfall, or one must apply a correction based on soil moisture conditions that are otherwise known. Based upon 10+ years of experience with a large GMON network that is deployed in Quebec, Canada, over northern organic boreal soil, it has been shown that in most cases, SM does not vary substantially during the winter season (Choquette et al., 2013; Ducharme et al., 2015). To consider SM as constant, mathematical equations that are used in calculating SWE can be simplified. If the goal is to measure the total water that is available for hydrological purpose, this aspect can become an advantage.
Counter-based sensors such as CRNP and GMON need to accumulate enough counts for reliable SWE estimates. Thus, it may be necessary to accumulate the counts over an adjusted period of time (several hours, depending on the case) so that the measurement is not strictly continuous. This can prevent accurate detection of short events, for example sudden heavy snowfalls.
For the GMON scintillator, depending on the type of soil at the measurement site, gamma ray emissions may not be sufficient and could require a longer integration period, as is the case for sites with thick organic-soil layers. It is possible to enrich gamma emissions by using bags or pipes of potassium-rich fertilizer, thereby maintaining a shorter integration time. Wright et al. (2011) achieved success with this approach, which yielded significantly higher count strengths. Such a protocol is illustrated in Fig. 2g (data not yet processed). Over glaciers, GMON requires such an enriched gamma emission set-up. The size of the area that is effectively monitored by the GMON scintillator (footprint) extends to 10 m from the detector when there is no snow or water on the ground (Ducharme et al., 2015). The size of the sensed area exponentially decreases with increasing SWE and is estimated to be of the order of 5 m radius (50–100 m2) for 150–300 mm w.e. (Martin et al., 2008; Ducharme et al., 2015). This relatively large footprint is an advantage of this sensor.
With the FMCW radar technique, as previously stated, penetration depth strongly depends on the measurement frequency. Generally, high-frequency instruments result in higher-resolution measurements, but these are also affected by greater signal attenuation, i.e., by a reduced depth of penetration. A disadvantage of this approach is that it requires the measurement of snow height as close as possible to the radar sensor. Also, the algorithm for thresholding the radar echo peaks must be developed as well as the calculation of the SWE (see Pomerleau et al., 2020).
GNSS electromagnetic waves can be attenuated under the forest canopy, as the forest transmissivity at 1.5 GHz is not negligible (Wigneron et al., 2017). Yet, because we normalized the signal beneath the snow against the one acquired above the snowpack, when both antennas were placed under the canopy, this effect should not alter retrieval. GNSSr is not well suited to very steep mountainous terrain (e.g., deep-valley bottoms), given that a rather wide sky-view factor is needed by the instrument and that this view can be limited in such environments, depending on slope and location (Koch et al., 2019; Steiner et al., 2018).
The main conclusions that emerge from Table 3 and the aforementioned remarks are the following, recalling that each approach has its own advantages and limitations (by order of presentation rather than by order of merit):
-
The CRNP approach is based on measurements of natural cosmic-ray fluxes, which are variable in time, unfortunately requiring complementary atmospheric measurements (temperature, pressure and atmospheric humidity) at each site for correcting the signal, and must be normalized against a nearby reference site (available worldwide). CRNP on the ground is the most efficient system for very deep snowpack (> 2000 mm w.e., perhaps up to 7000 mm w.e.), as is the case in mountain environments or northerly areas that are witness to winter lake-effect snowfall. The most advantageous aspect of the CRNP is its ability to measure SWE through complex snow layers from shallow- to deep-snow conditions. This is a robust and mature approach, as demonstrated by the French EDF experience (Gottardi et al., 2013; Lejeune et al., 2019); however, the EDF's sensor is based on a system that is not exploited commercially. The alternative sensors are the CRNP-based sensor that is manufactured by Hydroinnova (SnowFox or CRS-1000/B, Hydroinnova, Albuquerque, NM) (https://hydroinnova.com, last access: 25 October 2021) and the CRD manufactured by Alpine Hydromet (https://www.alpinehydromet.com, last access: 25 October 2021) and marketed by Geonor Inc. These sensors are relatively new and still need to demonstrate their robustness. The cost of Hydroinnova system is about USD 11 000 for the sensor only. As previously mentioned, ancillary sensors (sensors of atmospheric humidity and barometric pressure) must be added, and the actual price could be up to USD 17 000 for the full set-up. The cost of the Geonor Inc. system is USD 15 000.
-
CRNP above the snow is most interesting system for measuring SWE over a large footprint, but it is limited to shallow snowpacks. It is the only approach that can provide an integrated spatial measurement. This approach also needs appropriate adjustment for each site in terms of soil moisture corrections, which can be difficult over a large area.
-
GMON is one of the most mature instruments for snowpacks that are not too deep (600 mm w.e. according to manufacturer specifications but up to 800 mm w.e based on our experience) and has a medium footprint (10 m). Yet, it needs systematic site adjustment for soil-moisture-induced error, which can increase the bias of measurements, particularly at the end of the winter when the soil becomes potentially saturated during snowmelt. It is the most expensive of the four instruments (around USD 16 600, CAD 20 000). This system has proved its robustness and accuracy within the operational Hydro-Québec Canadian network over a wide variety of environments for almost 10 years (Choquette et al., 2013).
-
The FMCW radar approach requires the measurement of the snow depth to be able to retrieve SWE. Its weak point is its limitation in measuring the SWE of wet snow. Yet, the instrument is very useful for dry snowpack characterization, in terms of stratigraphy or for avalanche studies and also for detection of snowmelt events. Moreover, it is not expensive (USD 1000, EUR 800). As it is very light weight and compact, one of its strengths is its potential capability to retrieve SWE from remotely piloted aircraft above Arctic snowpacks.
-
The potential of the GNSSr approach, which is a light and compact system, is strong, given its capability of measuring SWE and LWC with high accuracy and deriving snow depth. For SWE retrieval, its performance remains very good (relative RMSD of ∼ 10 % in the range of 0–1000 mm) and has the capacity to measure deep snowpack (up to 1500 mm w.e.). SWE accuracy for wet snow has yet to be improved, as it depends upon the GNSS signal processing. Its cost is USD 8550 (EUR 7000). The station includes the software/license, and processing is performed on board the station. The station comes with 1 year of Iridium communication for the retrieved SWE/LWC product (via VISTA). VISTA support allows customers to find an operational way to retrieve data in operational use for the future. The license alone for processing the raw data can also be directly purchased from ANAVS (https://anavs.com/, last access: 25 October 2021) for USD 2370 (EUR 2000).
In this paper, we evaluated four types of non-invasive sensors that have all reached a certain level of maturity in enabling deployments of autonomous networks for monitoring the water equivalent of snow cover (SWE). These include the cosmic-ray neutron probe (CRNP), the gamma ray monitoring (GMON) sensor, the frequency-modulated continuous-wave radar at 24 GHz (FMCW radar) and the global navigation satellite system receiver (GNSSr) (see Table 1). This new generation of light and practical systems that are based on radiation-wave measurement is now commercially available. The GMON scintillator is already operationally used in Quebec, Canada, for hydrological purposes (Hydro-Québec, Rio Tinto and governments).
The analysis of their performances that is summarized in Tables 2 (uncertainties of measurement) and 3 (pros and cons) shows that each approach has its strengths and weaknesses. The synthesis of their advantages and disadvantages shows that the overall uncertainties remain in the range of manual measurements, i.e., 9 % to 15 %. CRNP that is placed in the ground beneath the snow is the only system capable of measuring very deep snowpacks, while the GNSSr sensor is limited to SWE up to ∼1500 mm w.e., and the two others are up to ∼800 mm w.e. Both the CRNP and GMON approaches need systematic site adjustments for soil moisture characterization. In addition to SWE, an advantage of the sensor to be considered is its ability to measure other parameters, such as snowpack stratigraphy for the FMCW radar and the liquid water content for the GNSSr. The GNSSr approach, which has a relatively low cost and is light and very compact, appears to have a great potential in remote and difficult-to-access areas.
The requirement of automatic instrumentation networks for SWE measurements to improve seasonal snowpack monitoring is important for several applications, where spatially distributed SWE instruments are needed such as in remote and mountainous areas, for operational water resource and flood management over snow-driven watersheds. Networks of continuous SWE measurements are also required for calibrating satellite-derived SWE information or for winter transportation safety. This review of continuously monitoring SWE sensors is intended to help researchers and decision-makers choose the one system that is best suited to their needs.
In situ field measurements of the water equivalent of snow cover (SWE) are accompanied by uncertainties from a variety of sources, which include (1) instrumental uncertainties, i.e., the size and type of sampling tubes according to snow depth and weight scale; (2) the sampling technique and extracting the snow core; (3) error that is induced by observer; (4) snow conditions, i.e., local natural variability, ice lenses and hard snow crusts within the snowpack; and (5) soil conditions, i.e., irregular soil surface and identification of the snow–ground interface. Snow depth is sometimes difficult to estimate over a thawed organic snow–ground interface because surface organic material is often taken into account in the snowpack depth estimate using a snow height probe.
In general, the uncertainty in the SWE depends mainly upon the diameter of the snow core according to the snow depth (the deeper the snow, the smaller the snow core that is required). Few studies discuss the accuracy of in situ SWE measurements comprehensively over a large range of conditions, from 100 to more than 2000 mm w.e. For example, the standard protocol that is implemented by Environment and Climate Change Canada is to attain 5 to 10 measurements along a pre-determined survey line of about 150 to 300 m using a translucent plastic ESC-30 sampler (6.2 cm ∅, which is commonly employed in Canada) (Brown et al., 2019). Each study is generally focused on one type of snowpack. Commonly, relative uncertainty varies from 6 % for shallow snowpack (0–300 mm w.e.) to 8 % (300–1000 mm w.e.) for medium snowpack to 10 %–12 % for deeper snowpack (> 1000 mm w.e.) (see references in the recent review by López-Moreno et al., 2020; also see Work et al., 1965; Turcan and Loijens, 1975; Peterson and Brown, 1975; Goodison et al., 1981, 1987; Sturm et al., 2010; Berezovskaya and Kane, 2007; Dixon and Boon, 2012; Stuefer et al., 2013; Steiner et al., 2018; Gugerli et al., 2019; Brown et al., 2019). Among recent studies, Stuefer et al. (2013) and López-Moreno et al. (2020) are limited to shallow Arctic snowpack, and Steiner et al. (2018) are limited to medium snowpack (up to 1200 mm w.e.), while Gugerli et al. (2019) discuss the problem across a large SWE range of Alpine snowpacks over a glacier from 200 to 2300 mm w.e. but with the same snow core (Fig. A1).
In summary, it is well known that SWE uncertainty decreases for shallow snowpack with a larger snow core diameter (typically above 6 cm diameter), given that a larger volume of snow is sampled. Yet, on the other hand, the coring technique is more difficult when snow depth increases. For thicker snowpack, it requires the digging of a pit, because a larger core diameter impeded the retrieval of the snow sample directly from the top of the snow surface. Thus, a large snow corer is limited to shallow snowpacks (snow depth less than 1.5–2 m). Moreover, common remarks from both our experience and the above-cited studies agree in that uncertainties in SWE estimates increase with thicker snowpacks. A small diameter snow core is required for thick snowpacks (snow depth above 2 m).
Figure A2 illustrates the underestimation of SWE with a large-diameter snow corer when SWE increases, from a large dataset that was derived from our International Polar Year experiments (Langlois et al., 2010).
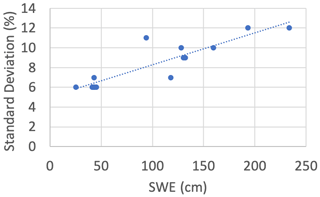
Figure A1Relationship between the standard deviation (%) of SWE measurements as a function of SWE (mm) based on snow core, derived from Gugerli et al. (2019) (data from Plaine Morte Glacier, Switzerland). Results show an uncertainty of 6 % for SWE of the order of 250–500 mm, about 10 % for SWE between 1000 and 1500 mm, and 12 % for SWE between 2000 and 2500 mm.
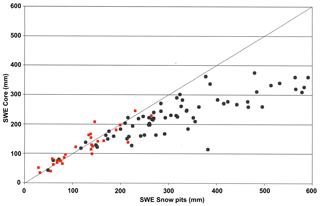
Figure A2Comparison between SWE measurements (in mm) from snow core and snow pit methods. Red squares are for small-diameter snow cores (ESC-30 type core, 6.2 cm), and black points are for large-diameter snow cores (9.5 cm). The black line is Y=X. Measured SWE core values are clearly underestimated above 250–300 mm w.e.. Unfortunately, no measurements with small-diameter snow cores above 280 mm w.e. are present in this example. The database (94 points) is derived from the International Polar Year project (Langlois et al., 2010), including sampling sites at Sherbrooke (SIRENE; 45.37∘ N, 71.92∘ W), Sept-Îles (50.30∘ N, 66.28∘ W), Schefferville (54.90∘ N, 66.70∘ W) and Kuujjuaq (58.06∘ N, 71.95∘ W) (also see Royer et al., 2021).
The data are available on request to the corresponding author.
The supplement related to this article is available online at: https://doi.org/10.5194/tc-15-5079-2021-supplement.
AlaR conceptualized the study and review. AlaR carried out the main analysis and wrote the paper. AlaR, AleR, SJ and AL contributed to the experiments, analysis of the results and revision of the manuscript.
The contact author has declared that neither they nor their co-authors have any competing interests. The mention of commercial companies or products does not constitute a commercial endorsement of any instrument or manufacturer by the authors.
Publisher’s note: Copernicus Publications remains neutral with regard to jurisdictional claims in published maps and institutional affiliations.
We acknowledge all of the students who have contributed to the field measurements, including Amandine Pierre, Maxime Beaudoin-Galaise and Benjamin Bouchard from Université Laval and Patrick Pomerleau, Fannie Larue and Alex Mavrovic from Université de Sherbrooke. We thank for their support the staff from Forêt Montmorency and from the Université de Sherbrooke Patrick Cliche, Patrick Ménard and Gabriel Diab. We also thank Alexandre Vidal of Hydro-Québec and Vincent Fortin of Environment and Climate Change Canada (ECCC). Finally, the reviewers, together with the following individuals, are thanked for their helpful comments, which improved the article: Craig Smith, ECCC; Charles Fierz, SLF, Davos; Yves Choquette, formerly of IREQ Hydro-Québec; Florian Appel, VISTA; and Reinhard Kulke, IMST.
This project was funded by the Natural Sciences and Engineering Research Council of Canada (NSERC), the Canadian Foundation for Innovation (CFI), Environment and Climate Change Canada (ECCC), and Fonds de recherche du Québec – Nature et technologies (FQRNT) of the government of Quebec. The European Space Agency (ESA) contributed to the installation of the GNSSr sensor within the ESA business development demonstration project SnowSense.
This paper was edited by Chris Derksen and reviewed by Craig Smith and Charles Fierz.
Alonso, R., del Pozo, J. M. G., Buisaìn, S. T., and Aìlvarez, J. A: Analysis of the Snow Water Equivalent at the AEMet-Formigal Field Laboratory (Spanish Pyrenees) during the 2019/2020 winter season using a Stepped-Frequency Continuous Wave Radar (SFCW), Remote Sens., 13, 616, https://doi.org/10.3390/rs13040616, 2021.
Andreasen, M., Jensen, K. H., Desilets, D., Franz, T., Zreda, M., Bogena, H., and Looms, M. C.: Status and perspectives of the cosmic-ray neutron method for soil moisture estimation and other environmental science applications, Vadose Zone J., 16, 1–11, https://doi.org/10.2136/vzj2017.04.0086, 2017.
Appel, F., Koch, F., Rösel, A., Klug, P., Henkel, P., Lamm, M., Mauser, W., and Bach, H.: Advances in Snow Hydrology Using a Combined Approach of GNSS In Situ Stations, Hydrological Modelling and Earth Observation – A Case Study in Canada, Geosciences, 9, 44, https://doi.org/10.3390/geosciences9010044, 2019.
Berezovskaya, S. and Kane, D. L.: Strategies for measuring snow water equivalent for hydrological applications: Part 1, accuracy of measurements. Proceedings of 16th Northern Research Basin Symposium, Petrozavodsk, Russia, 22–35, 2007.
Bissell, V. C. and Peck, E. L.: Monitoring snow water equivalent by using natural soil radioactivity, Water Resour. Res., 9, 885–890, 1973.
Bogena, H. R., Herrmann, F., Jakobi, J., Brogi, C., Ilias, A., Huisman, J. A., Panagopoulos, A., and Pisinaras, V.: Monitoring of Snowpack Dynamics with Cosmic-Ray Neutron Probes: A Comparison of Four Conversion Methods, Front. Water, 2, 19, https://doi.org/10.3389/frwa.2020.00019, 2020.
Bormann, K. J., Westra, S., Evans, J. P., and McCabe, M. F: Spatial and temporal variability in seasonal snow density, J. Hydrol., 484, 63–73, 2013.
Brown, R. D., Fang, B., and Mudryk, L.: Update of Canadian Historical Snow Survey Data and Analysis of Snow Water Equivalent Trends, 1967–2016, Atmos.-Ocean, 57, 149–156, https://doi.org/10.1080/07055900.2019.1598843, 2019.
Brown, R. D., Smith, C., Derksen, C., and Mudryk, L.: Canadian In Situ Snow Cover Trends for 1955–2017 Including an Assessment of the Impact of Automation, Atmos.-Ocean, 59, 77–92, https://doi.org/10.1080/07055900.2021.1911781, 2021.
Carroll, T. R.: Airborne Gamma Radiation Snow Survey Program: A user's guide, Version 5.0. National Operational Hydrologic Remote Sensing Center (NOHRSC), Chanhassen, 14, available at: https://www.nohrsc.noaa.gov/snowsurvey/ (last access: 25 October 2021), 2001.
Choquette, Y., Ducharme, P., and Rogoza, J.: CS725, an accurate sensor for the snow water equivalent and soil moisture measurements, in: Proceedings of the International Snow Science Workshop, Grenoble, France, 7–11 October 2013, 2013.
Clark, M. P., Hendrikx, J., Slater, A. G., Kavetski, D., Anderson, B., Cullen, N. J., Kerr, T., Hreinsson, E. Ö., and Woods, R. A.: Representing spatial variability of snow water equivalent in hydrologic and land-surface models: A review, Water Resour. Res., 47, W07539, https://doi.org/10.1029/2011WR010745, 2011.
Delunel, R., Bourles, D. L., van der Beek, P. A., Schlunegger, F., Leya, I., Masarik, J., and Paquet, E.: Snow shielding factors for cosmogenic nuclide dating inferred from long-term neutron detector monitoring, Quat. Geochronol., 24, 16–26, https://doi.org/10.1016/j.quageo.2014.07.003, 2014.
Desilets, D.: Calibrating a non-invasive cosmic ray soil moisture probe for snow water equivalent, Hydroinnova Technical Document 17-01, Zenodo, https://doi.org/10.5281/zenodo.439105, 2017.
Dixon, D. and Boon, S.: Comparison of the SnowHydro snow sampler with existing snow tube designs, Hydrol. Process., 20, 2555–2562, https://doi.org/10.1002/hyp.9317, 2012.
Desilets, D. and Zreda, M.: Footprint diameter for a cosmic-ray soil moisture probe: Theory and Monte Carlo simulations, Water Resour. Res., 49, 3566–3575, 2013.
Desilets, D., Zreda, M., and Ferreì, T. P. A.: Nature's neutron probe: Land surface hydrology at an elusive scale with cosmic rays, Water Resour. Res., 46, 1–7, 2010.
Dong, C.: Remote sensing, hydrological modeling and in situ observations in snow cover research: A review, J. Hydrol., 561, 573–583, 2018.
Ducharme, P., Houdayer, A., Choquette, Y., Kapfer, B., and Martin, J. P.: Numerical Simulation of Terrestrial Radiation over A Snow Cover, J. Atmos. Ocean. Tech., 32, 1478–1485, 2015.
Ellerbruch, D. and Boyne, H.: Snow Stratigraphy and Water Equivalence Measured with an Active Microwave System, J. Glaciol., 26, 225–233, 1980.
Evans, J. G., Ward, H. C., Blake, J. R., Hewitt, E. J., Morrison, R., Fry, M., Ball, L. A., Doughty, L. C., Libre, J. W., Hitt, O. E., Rylett, D., Ellis, R. J., Warwick, A. C., Brooks., M., Parkes, M. A., Wright, G. M. H., Singer, A. C., Boorman, D. B., and Jenkins, A.: Soil water content in southern England derived from a cosmic-ray soil moisture observing system – COSMOS-UK, Hydrol. Process., 30, 4987–4999, https://doi.org/10.1002/hyp.10929, 2016.
Fujino, K., Wakahama, G., Suzuki, M., Matsumoto, T., and Kuroiwa, D.: Snow stratigraphy measured by an active microwave sensor, Ann. Glaciol., 6, 207–210, 1985.
GCOS-WMO: The global observing system for climate: implementation needs, World Meteorological Organization, Geneva, Switzerland, available at: https://public.wmo.int/en/programmes/global-climate-observing-system (last access: 25 October 2021), 2016.
Goodison, B., Ferguson, H., and McKay, G.: Measurement and data analysis, in handbook of snow: principles, processes, management, and use, Pergamon press Canada, Toronto, Canada, 191–274, 1981.
Goodison, B. E., Glynn, J. E., Harvey, K. D., and Slater, J. E.: Snow Surveying in Canada: A Perspective, Can. Water Resour. J., 12, 27–42, https://doi.org/10.4296/cwrj1202027, 1987.
Gottardi, F., Carrier, P., Paquet, E., Laval, M.-T., Gailhard, J., and Garçon, R.: Le NRC: Une décennie de mesures de l’équivalent, in: Proceedings of the International Snow Science Workshop Grenoble, 7–11 October 2013, 926–930, 2013.
Gray, D. M., Granger, R. J., and Dyck, G. E.: Over winter soil moisture changes, T. ASAE, 28, 442–447, 1985.
Gray, D. M., Toth, B., Zhao, L., Pomeroy, J. W., and Granger, R. J.: Estimating areal snowmelt infiltration into frozen soils, Hydrol. Process., 15, 3095–3111, 2001.
GPRI brochure: GAMMA Portable Radar Interferometer (GPRI), available at: https://gamma-rs.ch/uploads/media/Instruments_Info/gpri2_brochure_20160708.pdf, last access: 25 October 2021.
Gugerli, R., Salzmann, N., Huss, M., and Desilets, D.: Continuous and autonomous snow water equivalent measurements by a cosmic ray sensor on an alpine glacier, The Cryosphere, 13, 3413–3434, https://doi.org/10.5194/tc-13-3413-2019, 2019.
Gunn, G. E., Duguay, C. R., Brown, L. C., King, J., Atwood, D., and Kasurak, A.: Freshwater Lake Ice Thickness Derived Using Surface-based X- and Ku-band FMCW Scatterometers, Cold Reg. Sci. Technol., 120, 115–126, 2015.
Haberkorn, A. (Eds.): European Snow Booklet – an Inventory of Snow Measurements in Europe, 363 pp., 2019.
Henkel, P., Koch, F., Appel, F., Bach, H., Prasch, M., Schmid, L., Schweizer, J., and Mauser, W.: Snow water equivalent of dry snow derived from GNSS Carrier Phases. IEEE T. Geosci. Remote, 56, 3561–3572, https://doi.org/10.1109/TGRS.2018.2802494, 2018.
Hu, X., Ma, C., Hu, R., and Yeo, T. S.: Imaging for Small UAV-Borne FMCW SAR. Sensors, 19, 87, https://doi.org/10.3390/s19010087, 2019.
IMST: IMST sentireTM Radar Module 24 GHz sR-1200 Series User Manual. available at: http://www.radar-sensor.com/, last access: 25 October 2021.
Jitnikovitch, A., Marsh, P., Walker, B., and Desilets, D.: Cosmic-ray neutron method for the continuous measurement of Arctic snow accumulation and melt, The Cryosphere Discuss. [preprint], https://doi.org/10.5194/tc-2021-124, in review, 2021.
Key, J., Goodison, B., Schöner, W., Godøy, Ø., Ondráš, M., and Snorrason, Á.: A Global Cryosphere Watch. Arctic, 68, 1, 48–58, https://doi.org/10.14430/arctic4476, 2015.
Key, J., Schöner, W., Fierz, C., Citterio, M., and Ondráš, M.: Global Cryosphere Watch (GCW) implementation plan, World Meteorological Organization, Geneva, Switzerland, available at: https://globalcryospherewatch.org/reference/documents/files/GCW_IP_v1.7.pdf (last access: 25 October 2021), 2016.
Kinar, N. J. and Pomeroy, J. W.: Measurement of the physical properties of the snowpack, Rev. Geophys., 53, 481–544, https://doi.org/10.1002/2015RG000481, 2015.
King, J., Kelly, R., Kasurak, A., Duguay, C., Gunn, G., Rutter, N., Watts, T., and Derksen, C.: Spatio-temporal influence of tundra snow properties on Ku-band (17.2 GHz) backscatter, J. Glaciol., 61, 267–279, https://doi.org/10.3189/2015JoG14J020, 2015.
Kirkham, J. D., Koch, I., Saloranta, T. M., Litt, M., Stigter, E. E., Møen, K., Thapa, A., Melvold, K., and Immerzeel, W. W.: Near Real-Time Measurement of Snow Water Equivalent in the Nepal Himalayas, Front. Earth Sci., 7, 177, https://doi.org/10.3389/feart.2019.00177, 2019.
Koch, F., Henkel, P., Appel, F., Schmid, L., Bach, H., Lamm, M., Prasch, M., Schweizer, J., and Mauser, W.: Retrieval of snow water equivalent, liquid water content, and snow height of dry and wet snow by combining GPS signal attenuation and time delay, Water Resour. Res., 55, 4465–4487, https://doi.org/10.1029/2018WR024431, 2019.
Koh, G., Yankielun, N. E., and Baptista, A. I.: Snow cover characterization using multiband FMCW radars, Hydrol. Process., 10, 1609–1617, 1996.
Laliberté, J., Langlois, A., Royer, A., Madore, J.-B., and Gauthier, F.: Retrieving high contrasted interfaces in dry snow using a frequency modulated continuous wave (FMCW) Ka-band radar: a context for dry snow stability, Phys. Geogr., in press, 2021.
Langlois, A.: Applications of the PR Series Radiometers for Cryospheric and Soil Moisture Research, Radiometrics Corporation, available at: https://www.researchgate.net/publication/299372180_Applications_ of_the_PR_Series_Radiometers_for_Cryospheric_ and_Soil_Moisture_Research (last access: 25 October 2021), 2015.
Langlois, A., Royer, A., and Goïta, K.: Analysis of simulated and spaceborne passive microwave brightness temperature using in situ measurements of snow and vegetation properties, Can. J. Remote Sens., 36, 135–148, https://doi.org/10.5589/m10-016, 2010.
Larson, K., Gutmann, E., Zavorotny, V., Braun, J., Williams, M., and Nievinski, F.: Can we measure snow depth with GPS receivers? Geophys. Res. Lett., 36, L17502, https://doi.org/10.1029/2009GL039430, 2009.
Larson, K. M.: GPS interferometric reflectometry: Applications to surface soil moisture, snow depth, and vegetation water content in the western United States, Wiley Interdisciplinary Reviews: Water, 3, 775–787, https://doi.org/10.1002/wat2.1167, 2016.
Larson, K. M. and Small, E. E.: Normalized microwave reflection index: A vegetation measurement derived from GPS networks, IEEE J. Sel. Top. Appl., 7, 1501–1511, https://doi.org/10.1109/JSTARS.2014.2300116, 2014.
Larue, F., Royer, A., De Sève, D., Roy, A., Picard, G., and Vionnet, V.: Simulation and assimilation of passive microwave data using a snowpack model coupled to a calibrated radiative transfer model over North-Eastern Canada, Water Resour. Res., 54, 4823–4848, https://doi.org/10.1029/2017WR022132, 2018.
Leinss, S., Wiesmann, A., Lemmetyinen, J., and Hajnsek, I.: Snow Water Equivalent of Dry Snow Measured by Differential Interferometry, IEEE J. Sel. Top. Appl., 8, 3773–379, 2015.
Lejeune, Y., Dumont, M., Panel, J.-M., Lafaysse, M., Lapalus, P., Le Gac, E., Lesaffre, B., and Morin, S.: 57 years (1960–2017) of snow and meteorological observations from a mid-altitude mountain site (Col de Porte, France, 1325 m of altitude), Earth Syst. Sci. Data, 11, 71–88, https://doi.org/10.5194/essd-11-71-2019, 2019.
López-Moreno, J. I., Leppänen, L., Luks, B., Holko, L., Picard, G., Sanmiguel-Vallelado, A., Alonso-González, E., Finger, D.C., Arslan, A. N., Gillemot, K., Sensoy, A., Sorman, A., Ertaş, M. C., Fassnacht, S. R., Fierz, C., and Marty, C.: Intercomparison of measurements of bulk snow density and water equivalent of snow cover with snow core samplers: Instrumental bias and variability induced by observers, Hydrol. Process., 34, 3120-3133, https://doi.org/10.1002/hyp.13785, 2020.
Marshall, H.-P. and Koh, G.: FMCW radars for snow research, Cold Reg. Sci. Technol., 52, 118–131, 2008.
Marshall, H.-P., Koh, G., and Forster, R.: Estimating alpine snowpack properties using FMCW radar, Ann. Glaciol., 40, 157–162, 2005.
Marshall, H.-P., Schneebeli, M., and Koh, G.: Snow stratigraphy measurements with high-frequency FMCW radar: Comparison with snow micro-penetrometer, Cold Reg. Sci. Technol., 47, 108–117, 2007.
Martin, J.-P., Houdayer, A., Lebel, C., Choquette, Y., Lavigne, P., and Ducharme, P.: An unattended gamma monitor for the determination of snow water equivalent (SWE) using the natural ground gamma radiation. 2008 IEEE Nuclear Science Symposium and Medical Conference, edited by: Sellin, P., IEEE, 983–988, 2008.
Matzler, C.: Microwave permittivity of dry snow, IEEE T. Geosci. Remote Sens., 34, 573–581, https://doi.org/10.1109/36.485133, 1996.
Meloche, J., Langlois, A., Rutter, N., Royer, A., King, J., and Walker, B.: Characterizing Tundra snow sub-pixel variability to improve brightness temperature estimation in satellite SWE retrievals, The Cryosphere Discuss. [preprint], https://doi.org/10.5194/tc-2021-156, in review, 2021.
Meredith, M., Sommerkorn, M., Cassotta, S., Derksen, C., Ekaykin, A., Hollowed, A., Kofinas, G., Mackintosh, A., Melbourne-Thomas, J., Muelbert, M. M. C., Ottersen, G., Pritchard, H., and Schuur, E. A. G.: Polar Regions, in: IPCC Special Report on the Ocean and Cryosphere in a Changing Climate, edited by: Pörtner, H.-O., Roberts, D. C., Masson-Delmotte, V., Zhai, P., Tignor, M., Poloczanska, E., Mintenbeck, K., Alegriìa, A., Nicolai, M., Okem, A., Petzold, J., Rama, B., and Weyer, N. M., available at: https://www.ipcc.ch/srocc/chapter/chapter-3-2/ (last access: 25 October 2021), 2019.
Murray, R. M. and Holbert, K. E.: Nuclear Energy: An Introduction to the Concepts, Systems, and Applications of Nuclear Processes, Eighth Edition, Imprint Butterworth-Heinemann, Elsevier Inc., 624 pp., https://doi.org/10.1016/C2016-0-04041-X, 2020.
Okorn, R., Brunnhofer, G., Platzer, T., Heilig, A., Schmid, L., Mitterer, C., Schweizer, J., and Eisen, O.: Upward-looking L-band FMCW radar for snow cover monitoring, Cold Reg. Sci. Technol., 103, 31–40, 2014.
Paquet, E. and Laval, M. T.: Retour d'expeìrience et perspectives d'exploitation des NivomeÌtres aÌ Rayonnement Cosmique d'EDF/Operation feedback and prospects of EDF Cosmic-Ray Snow Sensors, Houille Blanche, 2, 113–119, 2006.
Paquet, E., Laval, M., Basalaev, L. M., Belov, A., Eroshenko, E., Kartyshov, V., Struminsky, A., and Yanke, V.: An application of cosmic-ray neutron measurements to the determination of the snow-water equivalent, Proc. 30th Int. Cosm. Ray Conf., Mexico City, Mexico, 2008, 1, 761–764, 2008.
Peng, Z. and Li, C.: Portable Microwave Radar Systems for Short-Range Localization and Life Tracking: A Review, Sensors, 19, 1136, https://doi.org/10.3390/s19051136, 2019.
Peterson, N. and Brown, J.: Accuracy of snow measurements, in: Proceedings of the 43rd Annual Meeting of the Western Snow Conference, Coronado, California, 1–5, 1975.
Pieraccini, M. and Miccinesi, L.: Ground-Based Radar Interferometry: A Bibliographic Review, Remote Sens., 11, 1029, https://doi.org/10.3390/rs11091029, 2019.
Pirazzini, R., Leppänen, L., Picard, G., López-Moreno, J. I., Marty, C., Macelloni, G., Kontu, A., von Lerber, A., Tanis, C. M., Schneebeli, M., de Rosnay, P., and Arslan, A. N.: European in-situ snow measurements: practices and purposes, Sensors, 8, 2016, https://doi.org/10.3390/s18072016, 2018.
Pomerleau, P., Royer, A., Langlois, A., Cliche, P., Courtemanche, B., Madore, J.B., Picard, G., and Lefebvre, É.: Low Cost and Compact FMCW 24 GHz Radar Applications for Snowpack and Ice Thickness Measurements, Sensors, 20, 3909, https://doi.org/10.3390/s20143909, 2020.
Prince, M., Roy, A., Royer, A., and Langlois, A.: Timing and Spatial Variability of Fall Soil Freezing in Boreal Forest and its Effect on SMAP L-band Radiometer Measurements, Remote Sens. Environ., 231, 111230, https://doi.org/10.1016/j.rse.2019.111230, 2019.
Proksch, M., Rutter, N., Fierz, C., and Schneebeli, M.: Intercomparison of snow density measurements: bias, precision, and vertical resolution, The Cryosphere, 10, 371–384, https://doi.org/10.5194/tc-10-371-2016, 2016.
Rasmussen, R., Baker, B., Kochendorfer, J., Meyers, T., Landolt, S., Fischer, A. P., Black, J., Theìriault, J. M., Kucera, P., Gochis, D., Smith, C., Nitu, R., Hall, M., Ikeda, K., and Gutmann, E.: How Well Are We Measuring Snow: The NOAA/FAA/NCAR Winter Precipitation Test Bed, B. Am. Meteorol. Soc., 93, 811–829, 2012.
Rodriguez-Morales, F., Gogineni, S., Leuschen, C. J., Paden, J. D., Li, J., Lewis, C. C., Panzer, B., Alvestegui, D. G.-G., Patel, A., Byers, K., Crowe, R., Player, K., Hale, R., Arnold, E., Smith, L., Gifford, C., Braaten, D., and Panton, C.: Advanced multifrequency radar instrumentation for polar research, IEEE T. Geosci. Remote, 52, 2824–2842, 2014.
Roy, A., Royer, A., St-Jean-Rondeau, O., Montpetit, B., Picard, G., Mavrovic, A., Marchand, N., and Langlois, A.: Microwave snow emission modeling uncertainties in boreal and subarctic environments, The Cryosphere, 10, 623–638, https://doi.org/10.5194/tc-10-623-2016, 2016.
Roy, A., Toose, P., Williamson, M., Rowlandson, T., Derksen, C., Royer, A., Berg, A., Lemmetyinen, J., and Arnold, L.: Response of L-Band brightness temperatures to freeze/thaw and snow dynamics in a prairie environment from ground-based radiometer measurements, Remote Sens. Environ., 191, 67–80, 2017.
Royer, A., Domine, F., Roy, A., Langlois, A., Marchand, N., and Davesne G.: New northern snowpack classification linked to vegetation cover on a latitudinal mega-transect across northeastern Canada, Écoscience, https://doi.org/10.1080/11956860.2021.1898775, 2021.
Rutter, N., Sandells, M., Derksen, C., Toose, P., Royer, A., Montpetit, B., Lemmetyinen, J., and Pulliainen, J.: Snow stratigraphic heterogeneity within ground-based passive microwave radiometer footprints: implications for emission modeling, J. Geophys. Res.-Earth, 199, 550–565, https://doi.org/10.1002/2013JF003017, 2014.
Rutter, N., Sandells, M. J., Derksen, C., King, J., Toose, P., Wake, L., Watts, T., Essery, R., Roy, A., Royer, A., Marsh, P., Larsen, C., and Sturm, M.: Effect of snow microstructure variability on Ku-band radar snow water equivalent retrievals, The Cryosphere, 13, 3045–3059, https://doi.org/10.5194/tc-13-3045-2019, 2019.
Schattan, P., Baroni, G., Oswald, S. E., Schöber, J., Fey, C., Kormann, C., Huttenlau, M., and Achleitner, S.: Continuous monitoring of snowpack dynamics in alpine terrain by aboveground neutron sensing, Water Resour. Res., 53, 3615–3634, https://doi.org/10.1002/2016WR020234, 2017.
Schneider, M.: Automotive radar – Status and trends, in: Proceedings of the German Microwave Conference, Ulm, Germany, 5–7 April 2005, 144–147, 2005.
Shah, R., Xiaolan Xu, Yueh, S., Sik Chae, C., Elder, K., Starr, B., and Kim, Y.: Remote Sensing of Snow Water Equivalent Using P-Band Coherent Reflection, IEEE Geosci. Remote S., 14, 309–313, https://doi.org/10.1109/LGRS.2016. 2636664, 2017.
Sigouin, M. J. P. and Si, B. C.: Calibration of a non-invasive cosmic-ray probe for wide area snow water equivalent measurement, The Cryosphere, 10, 1181–1190, https://doi.org/10.5194/tc-10-1181-2016, 2016.
Smith, C. D., Kontu, A., Laffin, R., and Pomeroy, J. W.: An assessment of two automated snow water equivalent instruments during the WMO Solid Precipitation Intercomparison Experiment, The Cryosphere, 11, 101–116, https://doi.org/10.5194/tc-11-101-2017, 2017.
Steiner, L., Meindl, M., Fierz, C., and Geiger, A.: An assessment of sub-snow GPS for quantification of snow water equivalent, The Cryosphere, 12, 3161–3175, https://doi.org/10.5194/tc-12-3161-2018, 2018.
Steiner, L., Meindl, M., and Geiger, A.: Characteristics and limitations of GPS L1 observations from submerged antennas, J. Geodesy, 93, 267–280, https://doi.org/10.1007/s00190-018-1147-x, 2019.
Stranden, H. B., Ree, B. L., and Møen, K. M.: Recommendations for Automatic Measurements of Snow Water Equivalent in NVE. Report of the Norwegian Water Resources and Energy Directorate, Majorstua, Oslo, Noway, 34 pp., 2015.
Stuefer, S., Kane, L. D., and Liston, G. E.: In situ snow water equivalent observations in the US Arctic, Hydrol. Res., 44, 21–34, https://doi.org/10.2166/nh.2012.177, 2013.
Sturm, M., Taras, B., Liston, G., Derksen, C., Jones, T., and Lea, J.: Estimating snow water equivalent using snow depth data and climate classes, J. Hydrometeorol., 11, 1380–1394, 2010.
Tiuri, M., Sihvola, A., Nyfors, E., and Hallikainen, M.: The complex dielectric constant of snow at microwave frequencies, IEEE J. Oceanic Eng., 9, 377–382, 1984.
Turcan, J. and Loijens, J.: Accuracy of snow survey data and errors in snow sampler measurements, Proc. 32nd East. Snow. Conf., 2–11, 1975.
Vather, T., Everson, C. S., and Franz, T. E.: The applicability of the cosmic ray neutron sensor to simultaneously monitor soil water content and biomass in an Acacia mearnsii Forest, Hydrology, 7, 48, https://doi.org/10.3390/hydrology7030048, 2020.
Vriend, N. M., McElwaine, J. N., Sovilla, B., Keylock, C. J., Ash, M., and Brennan, P. V.: High-resolution radar measurements of snow avalanches, Geophys. Res. Lett., 40, 727–731, 2013.
Wallbank, J. R., Cole, S. J., Moore, R. J., Anderson, S. R., and Mellor, E. J.: Estimating snow water equivalent using cosmic-ray neutron sensors from the COSMOS-UK network, Hydrol. Process., 35, e14048. https://doi.org/10.1002/hyp.14048, 2021.
Werner, C., Wiesmann, A., Strozzi, T., Schneebeli, M., and Mätzler, C.: The SnowScat ground-based polarimetric scatterometer: Calibration and initial measurements from Davos Switzerland, in: Proc. IEEE Int. Geosci. Remote Sens. Symp. (IGARSS'10), Jul. 2010, 2363–2366, 2010.
Werner, C., Suess, M., Wegmüller, U., Frey, O., and Wiesmann A.: The Esa Wideband Microwave Scatterometer (Wbscat): Design and Implementation, in: Proc. IGARSS 2019 – IEEE International Geoscience and Remote Sensing Symposium, 8339–8342, https://doi.org/10.1109/IGARSS.2019.8900459, 2019.
Wiesmann, A., Werner, C., Strozzi, T., Matzler, C., Nagler, T., Rott, H., Schneebeli, M., and Wegmüller, U.: SnowScat, X- to Ku-Band Scatterometer Development, in Proc. of ESA Living Planet Symposium, Bergen 28.6.–2.7., available at: https://gamma-rs.ch/uploads/media/Instruments_Info/gamma_snowscat.pdf (last access: 25 October 2021), 2010.
Wiesmann, A., Werner, C., Wegmüller, U., Schwank, M., and Matzler, C.: ELBARA II, L-band Radiometer for SMOS Cal/Val Purposes, available at: https://gamma-rs.ch/uploads/media/Instruments_Info/ELBARAII_poster.pdf, last access: 25 October 2021.
Wigneron, J. P., Jackson, T. J., O'Neill, P., De Lannoy, G. J., de Rosnay, P., Walker, J. P., Ferrazzoli, P., Mironov, V., Bircher, S., Grant, J. P., Kurum, M., Schwank, M., Munoz-Sabater, J., Das, N., Royer, A., Al-Yaari, A., Bitar, A. Fernandez-Moran, R., Lawrence, H., Mialon, A., Parrens, M., Richaume, P., Delwart, S., and Kerr, Y.: Modelling the passive microwave signature from land surfaces: A review of recent results and application to the L-Band SMOS & SMAP soil moisture retrieval algorithms, Remote Sens. Environ., 192, 238–262, 2017.
Work, R. A., Stockwell, H. J., Freeman, T. G., and Beaumont, R. T.: Accuracy of field snow surveys, western United States, including Alaska, Cold Regions Research and Engineering Laboratory (U.S.) Technical report, 163, 49 pp., available at: https://hdl.handle.net/11681/5580 (last access: 25 October 2021), 1965.
Wright, M., Kavanaugh, K., and Labine, C.: Performance Analysis of the GMON3 Snow Water Equivalency Sensor. Proceedings of The Western Snow Conference. Stateline, NV, USA, April 2011, Poster on line, available at: https://s.campbellsci.com/documents/us/miscellaneous/performance-analysis-cs725.pdf (last access: 25 October 2021), 2011.
Wright, M.: CS725 Frozen Potential: The Ability to Predict Snow Water Equivalent is Essential. METEOROLOGICAL TEChnOLOGy InTERnATIOnAL, August 2013, 122–123, available at: https://www.meteorologicaltechnologyinternational.com (last access: 25 October 2021), 2013.
Xu, X., Baldi, C., Bleser, J.-W., Lei, Y., Yueh, S., and Esteban-Fernandez, D.: Multi-Frequency Tomography Radar Observations of Snow Stratigraphy at Fraser During SnowEx, in Proceedings of the IGARSS 2018-2018 IEEE International Geoscience and Remote Sensing Symposium, Valencia, Spain, 22–27 July 2018, 2018.
Yankielun, N., Rosenthal, W., and Robert, D.: Alpine snow depth measurements from aerial FMCW radar, Cold Reg. Sci. Technol., 40, 123–134, 2004.
Yankielun, N. E., Ferrick, M. G., and Weyrick, P. B.: Development of an airborne millimeter-wave FM-CW radar for mapping river ice, Can. J. Civil. Eng., 20, 1057–1064, 1993.
Yao, H., Field, T., McConnell, C., Beaton, A., and James, A.L.: Comparison of five snow water equivalent estimation methods across categories, Hydrol. Process., 32, 1894–1908, https://doi.org/10.1002/hyp.13129, 2018.
Zreda, M., Desilets, D., Ferré, T. P., and Scott, R. L.: Measuring soil moisture content non‐invasively at intermediate spatial scale using cosmic‐ray neutrons, Geophys. Res. Lett., 35, L21402, https://doi.org/10.1029/2008GL035655, 2008.
- Abstract
- Introduction
- Radiation-based SWE sensor review
- Results
- Strengths and weaknesses of instruments
- Conclusions
- Appendix A: Estimating the uncertainty of in situ field measurements
- Data availability
- Author contributions
- Competing interests
- Disclaimer
- Acknowledgements
- Financial support
- Review statement
- References
- Supplement
- Abstract
- Introduction
- Radiation-based SWE sensor review
- Results
- Strengths and weaknesses of instruments
- Conclusions
- Appendix A: Estimating the uncertainty of in situ field measurements
- Data availability
- Author contributions
- Competing interests
- Disclaimer
- Acknowledgements
- Financial support
- Review statement
- References
- Supplement