the Creative Commons Attribution 4.0 License.
the Creative Commons Attribution 4.0 License.
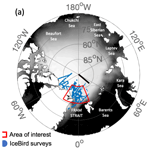
Interannual variability in Transpolar Drift summer sea ice thickness and potential impact of Atlantification
Thomas Krumpen
Luisa von Albedyll
Tatiana A. Alekseeva
Gerit Birnbaum
Sergei V. Frolov
Stefan Hendricks
Andreas Herber
Igor Polyakov
Ian Raphael
Robert Ricker
Sergei S. Serovetnikov
Melinda Webster
Christian Haas
Changes in Arctic sea ice thickness are the result of complex interactions of the dynamic and variable ice cover with atmosphere and ocean. Most of the sea ice exiting the Arctic Ocean does so through Fram Strait, which is why long-term measurements of ice thickness at the end of the Transpolar Drift provide insight into the integrated signals of thermodynamic and dynamic influences along the pathways of Arctic sea ice. We present an updated summer (July–August) time series of extensive ice thickness surveys carried out at the end of the Transpolar Drift between 2001 and 2020. Overall, we see a more than 20 % thinning of modal ice thickness since 2001. A comparison of this time series with first preliminary results from the international Multidisciplinary drifting Observatory for the Study of Arctic Climate (MOSAiC) shows that the modal summer thickness of the MOSAiC floe and its wider vicinity are consistent with measurements from previous years at the end of the Transpolar Drift. By combining this unique time series with the Lagrangian sea ice tracking tool, ICETrack, and a simple thermodynamic sea ice growth model, we link the observed interannual ice thickness variability north of Fram Strait to increased drift speeds along the Transpolar Drift and the consequential variations in sea ice age. We also show that the increased influence of upward-directed ocean heat flux in the eastern marginal ice zones, termed Atlantification, is not only responsible for sea ice thinning in and around the Laptev Sea but also that the induced thickness anomalies persist beyond the Russian shelves and are potentially still measurable at the end of the Transpolar Drift after more than a year. With a tendency towards an even faster Transpolar Drift, winter sea ice growth will have less time to compensate for the impact processes, such as Atlantification, have on sea ice thickness in the eastern marginal ice zone, which will increasingly be felt in other parts of the sea-ice-covered Arctic.
- Article
(2726 KB) - Companion paper
-
Supplement
(4603 KB) - BibTeX
- EndNote
The Arctic sea ice cover is undergoing rapid changes. Besides the continuous decline in annual mean sea ice extent by almost 14 % per decade from 1979 to 2010 (Cavalieri and Parkinson, 2012; Stroeve et al., 2012), sea ice volume has decreased as well. Based on a combination of submarine sea ice draft and satellite sea ice thickness (SIT) measurements from ICESat and CryoSat-2 from 1958 to 2018, Kwok (2018) found that central Arctic summer mean SIT decreased by about 60 % over 6 decades. This thinning was accompanied by a reduction in second-year and multi-year ice (SYI and MYI) fraction of more than 50 %, which resulted in substantial sea ice volume loss (Kwok, 2018; Spreen et al., 2020).
The importance of continuous measurements of Arctic SIT change is demonstrated by the implications these changes have on the Arctic summer sea ice energy and mass balance. Changing optical properties and thinning of sea ice allow increased penetration of solar energy into the ocean (Nicolaus et al., 2012; Katlein et al., 2019), with implications for ocean heat deposition (Perovich et al., 2007; Pinker et al., 2014) and primary productivity (Assmy et al., 2017; Horvat et al., 2017). Thinning of Arctic sea ice also impacts the pathways of sea ice from the major source regions on the Russian shelves. Due to the thinner ice cover sea ice drift is increased and sea ice is transported faster along the Transpolar Drift system (Spreen et al., 2011; Krumpen et al., 2019). However, the intensified summer melt and the initially thinner ice cover in the Siberian Arctic also lead to more frequent interruptions of the long-range transports of ice and ice-rafted matter from the shallow Russian shelves to the central Arctic Ocean (Krumpen et al., 2019). In order to predict the future development of these mechanisms, reliable measurements of sea ice parameters, like SIT, are vital.
Satellite-based radar altimeters provide the means to investigate Arctic-wide SIT changes, but due to the influence of warm snow and ice and the formation of melt ponds during the melt season, these data sets are only available from October through April (Ricker et al., 2017; Hendricks and Ricker, 2019). However, in light of recent model predictions of a nearly ice-free Arctic in summer (Johannessen et al., 2004; Holland et al., 2006; Wang and Overland, 2009; Overland and Wang, 2013; Overland et al., 2019), long-term and large-scale melt season SIT observations are more important than ever. Melt season SIT measurements from upward-looking sonar (ULS) (Hansen et al., 2013; WHOI, 2014; NPI, 2018; Belter et al., 2020a; Spreen et al., 2020), ground-based and airborne electromagnetic induction (EM) measurements (Haas, 2004; Haas et al., 2008, 2010), airborne remote sensing (Kurtz et al., 2013), and in situ drill holes (Kern et al., 2018) are spatially or temporally limited and therefore not sufficient for the investigation of long-term and large-scale variability.
Here we present an extended long-term summer SIT time series with data obtained within the framework of the IceBird, TIFAX, and previous summer air- and shipborne campaigns between 2001 and 2020. These summer campaigns, led by the Alfred Wegener Institute, Helmholtz Centre for Polar and Marine Research, were designed to provide a long-term time series of large-scale SIT measurements in, but not limited to, the vicinity of the main exit gate of Arctic sea ice – Fram Strait. The extensive airborne and ground-based survey activities provide a unique basis for the investigation of large-scale SIT distributions. In this framework, SIT is measured using airborne EM (AEM) and ground-based EM (GEM) devices, which make use of the contrasting electromagnetic conductivities between sea ice and seawater (Haas et al., 2008). Since data are only available from a number of years and the area covered during these campaigns (Fig. 1a)) includes a wide range of different ice types from various sources, a careful analysis is required for the investigation of interannual SIT variability.
For the current study we focus on the SIT measurements from a selected area of interest (AOI, enclosed area in Fig. 1a) north of Fram Strait. Sea ice reaching Fram Strait originates from multiple regions of the Arctic, which means long-term observations of SIT in its vicinity provide insight into integrated Arctic-wide thermodynamic and dynamic changes in the sea ice cover (Hansen et al., 2013). While previous studies recorded substantial thinning in Fram Strait in summer (Hansen et al., 2013; Spreen et al., 2020) and across Fram Strait (79∘ N) SIT gradients in spring during the first decade of the 21st century (Renner et al., 2014), we focus on the evolution of summer (July–August) SIT further upstream of the Transpolar Drift. With the AEM being towed by a fixed-wing aircraft, longer transects and ultimately a greater areal distribution of the measurements are achieved compared to other in situ observations.
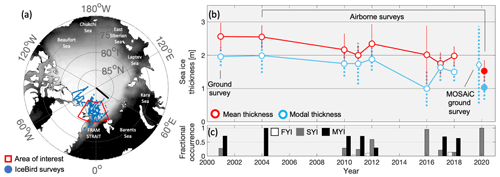
Figure 1(a) Map showing all EM-based summer (July–August–September) SIT measurements (dark blue circles) obtained between 2001 and 2020, as well as July–August mean sea ice concentration (OSI-450 and OSI-430-b, 25×25 km) for the period from 2000 to 2019 (Lavergne et al., 2019). Enclosed area (red, 80.5–86∘ N and 30∘ W–20∘ E) indicates the selected area of interest (AOI; see Table 1 for an overview of the corresponding expeditions and basic SIT statistics for the selected AOI). The black line (86–90∘ N, 50∘ E) shows the transect of Russian sea ice observations. (b) Summer (July–August) mean (red circles) and modal (light blue circles) SIT based on EM measurements conducted in the AOI (dark blue circles in a). The diamond shows modal EM SIT measured on the MOSAiC floe in July 2020, and filled circles indicate mean and modal EM SIT values obtained during the IceBird MOSAiC campaign in September 2020. Vertical lines indicate standard deviations of mean (red) and modal (light blue) SIT values of the individual profiles from each year. Panel (c) shows the fractional occurrence of first-year (FYI, white), second-year (SYI, grey), and multi-year ice (MYI, black, ice older than 2 years) for the individual years. The age classification is based on ICETrack calculations of the number of days the ice particles travelled along their trajectories.
Table 1Summary of used research platforms, sampling periods, profile lengths, and basic sea ice thickness statistics for the selected area of interest (see Fig. 1a) and individual research campaigns.
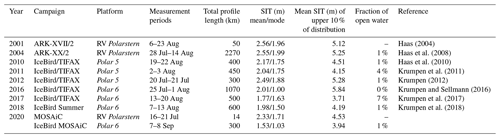
The objectives of this study are to extend the summer SIT time series (from 2012 to 2020), first published by Krumpen et al. (2016), at the end of the Transpolar Drift and investigate the interannual variability in SIT in the selected AOI close to the export gate of Arctic sea ice. We will use the Lagrangian sea ice tracking tool, ICETrack (Krumpen, 2018), to determine the source regions and drift trajectories of the sea ice sampled in the AOI. In order to provide insight into the driving mechanisms of the observed SIT variability, a thermodynamic model is applied along the determined sea ice trajectories to reconstruct the AOI-sampled SIT. In addition we will compare the SIT changes in the AOI to long-term observations gathered during regular Russian cruises from Franz Josef Land to the North Pole. This additional comparison is conducted to discuss whether the observed changes are limited to the AOI or induced during ice formation and transit through the Arctic Ocean. Finally, we will use the unique opportunity to compare the long-term SIT time series to IceBird and GEM measurements conducted within the framework of the Multidisciplinary drifting Observatory for the Study of Arctic Climate (MOSAiC). At 85∘ N and 136∘ E the German icebreaker RV Polarstern (Alfred Wegener Institute, 2017) moored to a 2.8 × 3.8 km sized ice floe in October 2019 (Krumpen et al., 2020). After about 9 months of drifting through the Arctic Ocean, RV Polarstern and the MOSAiC floe reached the selected AOI in summer 2020. This allows us to consider the MOSAiC floe in the context of the long-term time series and determine whether the SIT of the MOSAiC floe in 2020 was exceptional or in agreement with historical observations.
2.1 EM sea ice thickness measurements
Electromagnetic induction (EM) SIT measurement systems take advantage of contrasting electrical conductivities between sea ice and seawater to determine the distance between the ice–water interface and the EM device (Kovacs and Morey, 1991; Haas et al., 1997). In 2001, measurements were conducted using a Geonics EM31 ground-based EM instrument (GEM). The GEM was pulled over the ice on a sledge and obtained the distance to the ice–water interface (Haas, 2004). GEM measurements included SIT values over melt ponds and pressure ridges; however, open water and thin ice were not adequately represented in the data sets due to the practical limitations of sampling those areas on foot (Haas, 2004). The 2020 GEM measurements on the MOSAiC floe were taken with the Geophex GEM-2, a broadband EM sensor used for advanced thickness observations (Hunkeler et al., 2016). After 2001, SIT was obtained using the airborne EM system (AEM), EM Bird, that was towed by a helicopter (in 2004) and the research aircraft Polar 5 and 6 (from 2010 onwards). The EM Bird was operated between 12 and 20 m above the ice surface (Krumpen et al., 2016). Following Pfaffling et al. (2007), SIT was calculated as the difference between the EM-derived distance to the ice–water interface and the laser altimeter-recorded distance between the EM device and the air–snow interface. EM measurement accuracy is within 0.1 m to drill-hole measurements over level sea ice, while water inclusions within pressure ridges lead to a general underestimation of ridges by as much as 40 % to 50 % (Pfaffling et al., 2007; Haas et al., 2009).
Thickness measurements using the ground-based and airborne EM methods always represent the total combined sea ice and snow thickness (Haas et al., 1997). Given the study period from mid-July to mid-August and following climatological values of snow depth (Warren et al., 1999; Renner et al., 2014; Krumpen et al., 2016), we assume a 0.1 m snow or weathered layer thickness, which is negligible for the EM measurements. More snow may still have been present during episodic precipitation events, but likely melted within a few days.
In order to ensure comparability of the available EM-based measurements from 2001 to 2020, only data taken between 80.5 to 86∘ N and 30∘ W to 20∘ E (AOI, Fig. 1a) were selected for the analysis. Following Krumpen et al. (2016), the AOI was also selected to be north of Fram Strait to concentrate the analysis on sea ice that was shaped along its pathways through the Arctic rather than by local melt phenomena in Fram Strait. Finally, the selected AOI allows for a more reliable analysis of the trajectories of the sampled sea ice since low-resolution sea ice motion products used for Lagrangian tracking are highly uncertain in Fram Strait (Krumpen et al., 2019). Expedition logistics and the prevailing weather conditions prevented us from acquiring continuous and overlapping measurements over the full AOI each year. However, following Rabenstein et al. (2010) the lengths of the conducted EM profiles were adequate to consider the data to be sufficiently homogeneous in time and space and representative for the sampled region and time of year. Table 1 provides an overview of all relevant field campaigns, duration, profile lengths, basic statistics, and references for the measurements from within the selected AOI.
The analysis of trends and interannual variability of summer SIT in the AOI is based on temporal and spatial averages and the most frequently occurring EM SIT – the mode of the distribution. Modal SIT is a representation for the thickness of thermodynamically grown level ice, while mean SIT includes thermodynamically and dynamically grown sea ice and therefore is an indication for the general variability of SIT (Haas, 2017). Prior to the calculation of summer mean and modal SIT values from all available data points within the predefined AOI (Fig. 1), SIT values <0.1 m, including open water values, were excluded to avoid biases due to different fractions of open water areas in the data sets.
2.2 Sea ice pathways and source regions
In order to determine the pathways and source regions of the ice that was sampled in the selected AOI, we utilized the Lagrangian ice tracking tool, ICETrack (Krumpen, 2018). The starting points for the backward tracking of AOI-sampled sea ice were derived based on the positions of the EM measurements. EM SIT data were gridded to a 25 × 25 km equal-area scalable Earth grid. For a minimum of 2000 AEM SIT values within a single grid cell, the respective grid point was selected to be a starting point for the backward tracking with ICETrack. Due to the limited number of measurements available from the short GEM surveys, ICETrack starting points for the year 2001 were also calculated for fewer than 2000 SIT values per grid cell. The MOSAiC floe trajectory is based on position records from RV Polarstern and backward tracking of the floe from the MOSAiC starting point at 85∘ N and 136∘ E in October 2019 (Krumpen et al., 2020).
Ice parcels were tracked backward in time on a daily basis. Termination criteria for the tracking were met either when the ice reached a coastline or when sea ice concentration (SIC) dropped to 25 % or less. When SIC reaches 25 % or less, ICETrack assumes that ice is formed. The applied SIC product is provided by the Center for Satellite Exploitation and Research (CERSAT) and is based on 85 GHz SSM/I brightness temperatures, using the ARTIST Sea Ice algorithm (Ezraty et al., 2007). The number of days from the first day of tracking until ice formation provided the age of the sea ice sampled in the AOI. The tracking was based on a weighted approach to determine the most appropriate of the three available low-resolution sea ice motion products (Krumpen et al., 2019): (i) motion estimates from scatterometer and radiometer data from CERSAT (Girard-Ardhuin and Ezraty, 2012), (ii) the OSI-405-c motion product produced by the Ocean and Sea Ice Satellite Application Facility (OSI SAF) (Lavergne, 2016), and (iii) Polar Pathfinder Daily Motion Vectors from the National Snow and Ice Data Center (NSIDC) (Tschudi et al., 2019). CERSAT was prioritized as it provides the most consistent time series of motion vectors (from 1991 onwards). However, when CERSAT data were missing (especially during summer months), OSI SAF data were used. Prior to 2012 or when OSI SAF data were not available, NSIDC data were utilized (Krumpen et al., 2019). A detailed description of the three motion products is given by Sumata et al. (2014). In addition to sea ice trajectories, ICETrack provided information about satellite-derived SIT and SIC as well as atmospheric parameters, like surface air temperature, 10 m wind speed, and surface pressure in daily increments along the trajectories (Krumpen, 2018). Due to this comprehensive approach to analyse sea ice along its trajectories through the Arctic and its accuracy (Krumpen et al., 2019), ICETrack has been widely used in previous studies, e.g. Damm et al. (2018) and Peeken et al. (2018).
2.3 Thermodynamic sea ice model
In order to investigate the driving mechanisms of interannual variability in modal SIT in the AOI, ICETrack was combined with a simple one-dimensional thermodynamic sea ice model developed by Thorndike (1992). Parallel to retrieving SIC, SIT, atmospheric parameters, and sea ice motion from ICETrack, the model calculated daily sea ice growth and melt along the determined sea ice trajectories. Latent heat of melting–freezing, ocean heat flux, and conductive heat loss are balanced to model ice growth at the bottom, (Thorndike, 1992; Pfirman et al., 2004):
The model used along-track snow depth, Hsnow, computed daily from the Warren climatology (Warren et al., 1999) as well as the NCEP/NCAR re-analysis sea surface temperature (Tsurf) data (Kalnay et al., 1996) that were extracted along the trajectories by ICETrack (Krumpen et al., 2019). T0 is the temperature at the ice–water interface (−1.9 ∘C), and k is the thermal conductivity (kice=2 W m−1 K−1, ksnow=0.33 W m−1 K−1). Latent heat of fusion, L, was constant at 3 × 108 Jm−3. Δt equals 86 400 s for daily increments of ice growth. Ocean heat flux, F, was assumed to be constant at 2 W m−2. Based on these input parameters the model computed daily changes in SIT along the trajectories. When melt occurred (negative growth for any given day) the model reduces the thickness by an additional 0.005 m for that day to parametrize surface melt (Thorndike, 1992; Pfirman et al., 2004). Modelled SIT values at the end of each track were used to calculate AOI summer mean thermodynamic SIT for each year. This modelled value provides SIT excluding the snow layer that is inherently included in the EM SIT values. We therefore added the Warren snow depth value from the position and time of the relevant EM SIT measurements to the final model SIT averages for the comparison to EM SIT. Like modal EM SIT, the modelled SIT is a representation of thermodynamically grown level sea ice.
Snow depth is an important parameter in modelling sea ice growth and due to the limitations of the Warren snow depth climatology (Warren et al., 1999) a major source for uncertainty (Merkouriadi et al., 2020) in the modelled SIT values calculated for each trajectory ending in the AOI. Following Laxon et al. (2013) and Ricker et al. (2014), we reduced Warren snow depth by 50 % over FYI and also based on comparisons of the Warren snow depth with snow buoy data over SYI. This step accounts for the fact that the Warren snow depth is based on observations during a period where Arctic sea ice was dominated by MYI with thicker snow. Root-mean-square errors of Warren snow depth values (Warren et al., 1999) were utilized to calculate the model SIT equivalent of the snow depth errors and provide an estimate of uncertainty of the modelled SIT as a result of the applied Warren snow climatology.
Another major source of uncertainty of the modelled SIT is the selected ocean heat flux value. However, due to the simplicity of the selected sea ice model (Thorndike, 1992) and the lack of long-term and Arctic-wide data, for this current study the input of a constant ocean heat flux value was required. We followed previous studies (Maykut and Untersteiner, 1971; Pfirman et al., 2004; Peeken et al., 2018; Krumpen et al., 2019) and selected a constant ocean heat flux value of 2 W m−2. This value was applied to the sea ice growth model along each trajectory from ice formation to the AOI.
2.4 Shipborne sea ice thickness observations
In general, ship-based observations of SIT benefit from the increasing number of regular ship transits through the Arctic Ocean. SIT data used here were observed visually (uncertainty of ±0.1 m; Frolov et al., 2007; AARI, 2011) either by a group of Arctic and Antarctic Research Institute (AARI) sea ice scientists using the traditional unified methodological principles in accordance with the requirements of the regulatory guidance (AARI, 2011; Alekseeva et al., 2019) or by the so-called shipborne television complex (STK). The STK consists of a high-resolution telecamera, a computer for camera control and processing, and a GPS recorder. The system records images of overturning sea ice floes in the vicinity of the moving ship as well as GPS time and coordinates. After manual selection of appropriate images, the software is able to measure the detailed geometry of single ice blocks from the ice camera feed and retrieve ice and snow thickness data. The STK system provides SIT with an uncertainty of approximately 4 % of the thickness of each floe (Frolov et al., 2007; AARI, 2011). The purpose of this system is to provide navigation data for following ships and reliable SIT data for the validation of satellite- and model-derived SIT. Over the last decades the AARI has conducted visual and STK observations regularly during summer (June–August) tourist cruises from Franz Josef Land to the North Pole (Table 2). The SIT data used here are based on STK (2006 to 2011) and visual observations (1977 to 1996 and 2012 to 2019) in July on a transect from 86 to 90∘ N along the 50∘ E meridian. Sea ice was categorized as FYI or MYI (including SYI), and mean SIT was calculated for both. Depending on the fractional occurrence of FYI and MYI along the transect, a mean summer SIT value was calculated.
Table 2Summary of used ships, number of cruises, measurement periods, observation types, and mean sea ice thicknesses (SIT) observed during Russian cruises along the 86–90∘ N, 50∘ E transect (black line Fig. 1a).
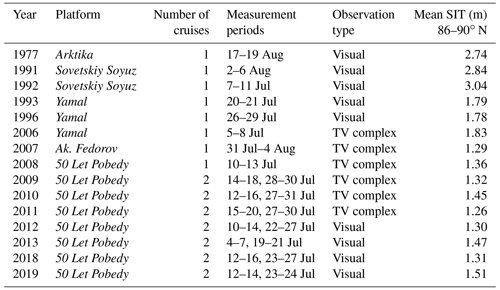
3.1 Processes driving interannual SIT variability between 2001 and 2018
3.1.1 IceBird surveys: sea ice thickness, origin, and age
Figure 1b provides an overview of the interannual variability and changes of summer SIT in the AOI from 2001 to the MOSAiC year 2020. Modal summer SIT, which is a measure for the fraction of sea ice that grew thermodynamically, decreased by approximately 24 % from 2001 (approximately 2 m) to 2018 (approximately 1.5 m). The decrease in modal SIT was not gradual but showed an 11 % drop after 2004 and an absolute minimum of approximately 1 m in 2016. The change in modal SIT was accompanied by a change in the fractional occurrences of FYI, SYI, and MYI. This categorization of different ice ages is based on the ICETrack-derived lengths of the sea ice trajectories. While the sampled sea ice almost exclusively consisted of MYI in 2001 and 2004, the fractions of SYI and even FYI increased notably between 2010 and 2018 (Fig. 1c). In 2012 and especially 2016, sea ice in the AOI was dominated by SYI.
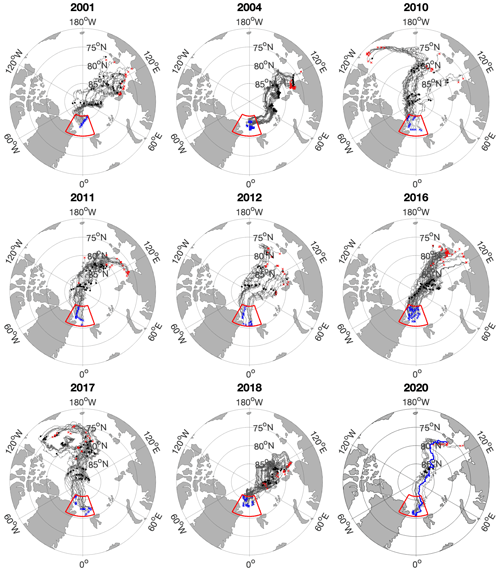
Figure 2Drift trajectories of sea ice sampled in the AOI (enclosed area). Starting points of the backtracking (blue crosses) correspond to the positions where EM SIT measurements were conducted during summer (July–August–September) from 2001 to 2020. Backward tracking ended when sea ice concentration dropped to 25 % or less, which is defined as the time and location of ice formation (red crosses). Black dots present sea ice position on 21 September of each year, when ice particles are considered to have survived the summer, becoming second-year or multi-year ice, respectively. The blue trajectory in 2020 represents the MOSAiC floe trajectory. The MOSAiC trajectory consists of GPS positions recorded during the drift (from 4 October 2019 until 21 July 2020) and backward tracked position (ICETrack) from the MOSAiC starting point (4 October 2019) to the position of ice formation (Krumpen et al., 2020).
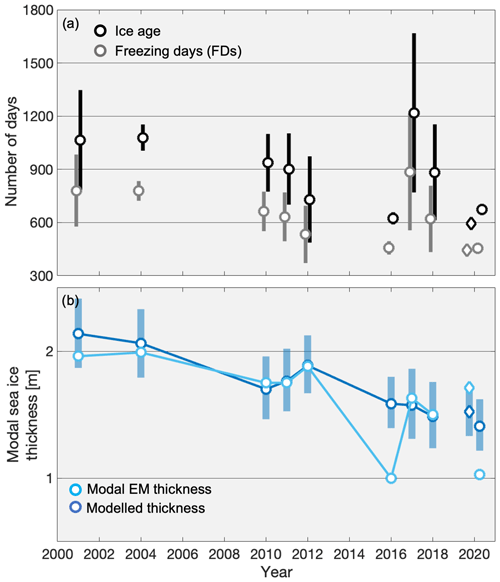
Figure 3(a) Mean sea ice age (black) and mean number of days with surface temperatures below −1.9 ∘C (grey, FDs) of AOI-sampled sea ice. Error bars indicate 1 standard deviation of age and FDs for each year. (b) Modal EM SIT (light blue; see Fig. 1b) and reconstructed modal SIT from the Thorndike (1992) thermodynamic sea ice model. Error bars show maximum modelled SIT error resulting from root-mean-square errors of Warren et al. (1999) snow values. Circles indicate larger-area measurements, while diamonds show values referring to the MOSAiC floe.
Mean SIT decreased by a similar percentage (23 %) from 2001 (approximately 2.6 m) to 2018 (approximately 2 m). The difference between mean and modal SIT was on the order of 0.5 m in 2001, 2004, 2012, and 2018 and showed a decrease to values of 0.2 to 0.3 m in 2010/2011. During the modal minimum in 2016 the mean–mode difference peaked at about 1 m and showed its minimum of 0.1 m in the following year 2017. The differences between the time series of modal and mean SIT are likely caused by the interannual variability in ridged sea ice (see SIT distributions in Fig. S1 in the Supplement and the mean of the upper 10 % of the EM SIT distribution in Table 1).
To some degree the interannual modal thickness variability can be explained by the variability in source regions of the ice (Fig. 2), its age, and the number of days with surface temperatures below freezing (−1.9 ∘C, FDs) the ice experienced during the transit through the Arctic Ocean (Fig. 3a). Figure 2 indicates that about 65 % of all analysed tracks of the ice surveyed in the AOI originated in the Laptev Sea (70 to 81.5∘ N, 100 to 145∘ E). Even though most of the surveyed ice can be linked to the Laptev Sea, the actual places of ice formation differed within this shallow shelf sea. While ice surveyed in 2001, 2004, 2011, and 2018 was formed close to the coast in the western Laptev Sea, the ice sampled in 2012 and 2016 was formed in the northern and eastern Laptev Sea, respectively. The sea ice surveyed in the AOI in 2001 and 2004 took, on average, approximately 3 years from its origin in the Laptev Sea to the AOI. In the following years, ice that originated in similar regions of the Laptev Sea took less time to reach the AOI (2011 and 2018). The decrease in sea ice age is linked to the observed increase in sea ice drift speed after 2004 (Spreen et al., 2011). With an average age of about 1.7 years, the ice sampled in the AOI in 2016, which showed the minimum modal SIT, was by far the youngest during the period from 2001 to 2018. The on average oldest ice was sampled in the AOI in 2017 and originated mostly from the Beaufort Sea. It has to be noted that ice of strongly varying ages was sampled in the AOI in 2017. These variations in age and FDs in 2017 are likely the reason why maximum modal and mean SIT values do not coincide with the on average oldest ice in 2017. Apart from the year 2017, variations in modal SIT of the ice sampled in the AOI can be largely explained by the variations in age and number of FDs. Older ice showed larger modal SIT values in the AOI, while younger ice was thinner. However, the unknown magnitude of melt during days with surface air temperatures above freezing also contributes to the observed variability.
It has to be noted that the varying number of EM surveys each year and the variation in areal coverage within the AOI of the different surveys makes the analysis of SIT trends challenging. However, large-scale and year-to-year overlapping surveys as well as sampling during the same season each year strengthen the assessment that sea ice sampled in the AOI is changing in thickness and age.
3.1.2 Reconstruction of observed SIT using a thermodynamic model
To further investigate the processes driving interannual variability of modal SIT, we compare observed AOI values with modelled SIT values of thermodynamically grown sea ice. Sea ice growth along the ICETrack sea ice trajectories was calculated using the thermodynamic sea ice model by Thorndike (1992). The modelled SIT values at the end of the trajectories provide the AOI-mean modelled SIT for each year. Figure 3b indicates that the modelled SIT time series shows similar values compared to the modal EM SIT time series. Except in 2016, all July–August EM SIT values are within the estimated uncertainty of the modelled SIT, that is based on the root-mean-square error of snow depth provided by Warren et al. (1999). The value in 2016 is significantly smaller than the modelled value compared to the other years of the time series. While the modelled SIT slightly overestimates modal EM SIT values in 2001 and 2004, it is similar to the observed values from 2010 to 2012 and from 2017 to 2018 and underestimates EM SIT in 2020. In 2016 the model overestimates the AOI modal EM SIT by almost 0.6 m.
The general agreement between modal EM and modelled SIT supports the hypothesis that sea ice age and freezing-degree days govern the modal SIT in the AOI. However, it is evident that the exceptional modal SIT observed in 2016 can not be explained by the model, i.e. atmospheric processes alone, indicating that additional factors contributed to this minimum in modal SIT in the AOI.
3.2 Possible impact of Atlantification on SIT in 2016
The major sources for uncertainty of the reconstructed SIT time series are snow depth and the assumptions of constant ocean heat flux and surface melt. While modal SIT values are within the range of the reconstructed SIT values ± snow depth error in most years, the model significantly overestimates the modal SIT in 2016. The sea ice sampled in the AOI in 2016 was about 0.6 m thinner than the modelled SIT, which suggests an additional heat source, unaccounted for by the model, that reduced ice growth along the trajectories. Following Eq. (1), bottom sea ice growth and melt are the result of the heat fluxes from either the atmosphere or the ocean. Considering that the variability of the atmospheric component of the model was accounted for along the trajectories, the other parameter potentially responsible for the overestimation of modal SIT is the insufficient assumption of constant ocean heat flux along the trajectories of the AOI-sampled ice in 2016.
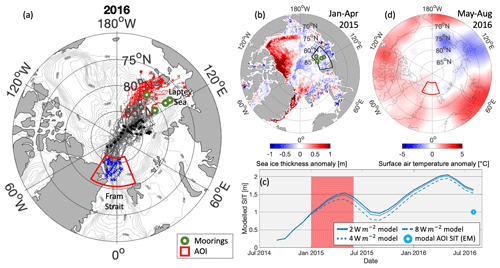
Figure 4(a) Drift trajectories of AOI-sampled (enclosed area) sea ice in 2016. Starting points for the backtracking (blue crosses) correspond to the positions where EM SIT measurements were conducted during July and August 2016. Tracking ended when sea ice concentration dropped to 25 % or less, which is defined as time and position of ice formation (red crosses). Black dots present sea ice position on 21 September, when ice particles are considered to have survived the summer, becoming second-year and multi-year ice, respectively. The sections of the trajectories coloured in red indicate sea ice position between January and through May 2015. Green markers indicate the positions of oceanographic moorings operated between summer 2013 and summer 2015 (Polyakov et al., 2017). (b) January to April 2015 SIT anomaly (compared to the January to April mean from 2003 to 2020) from the ESA CCI-2 SIT climate data record (Hendricks et al., 2018a, b). (c) Mean SIT modelled along the drift trajectories of the sea ice sampled in the AOI in 2016. Solid blue line indicates the mean modelled thickness over all trajectories using a constant ocean heat flux value of 2 W m−2. Dotted (dashed) blue line shows average modelled SIT using 2 W m−2 ocean heat flux along the black parts of the trajectories (Fig. 3a) and 4 W m−2 (8 W m−2) along the red parts of the trajectories (shaded red area). Light blue marker indicates the observed modal EM SIT in the AOI in 2016. (d) The 2016 May to August surface air temperature anomaly in comparison to the 2000 to 2019 long-term mean (data source http://www.esrl.noaa.gov/psd/products, last access: 8 July 2020).
Ocean heat flux is the main source of bottom melting (Lin and Zhao, 2019); it is a parameter that is widely debated and still being investigated. It is the sum of heat that enters the surface mixed layer from the deep ocean and heat that enters the surface mixed layer through leads and openings in the ice cover (Zhang et al., 2000; McPhee et al., 2003; Perovich et al., 2011; Wang et al., 2016). Multiple existing studies have shown that Arctic ocean heat flux is highly variable in time and space (Maykut, 1982; Maykut and McPhee, 1995; McPhee et al., 2003; Krishfield and Perovich, 2005; Lin and Zhao, 2019). Nevertheless, the assumption of a constant average ocean heat flux value seemed sufficient for thermodynamic sea ice modelling in the past (Peeken et al., 2018; Krumpen et al., 2020) and is confirmed in this study by the agreement between modelled and modal SIT in all years except 2016.
The studies by Polyakov et al. (2017, 2020) showed that the observed decline in sea ice extent and increased open water area enable increased ocean ventilation and weakening of the upper ocean stratification in the eastern marginal ice zones. The resulting change in stratification, warming of the upper pycnocline, and shoaling of the Atlantic Water (AW) layer result in enhanced upward AW heat flux in winter, which leads to further thinning of the overlaying ice cover. This process of so-called Atlantification is considered to be a positive feedback mechanism (Polyakov et al., 2020) and was mainly observed at the inflow gates of AW into the Arctic Ocean in the Barents Sea (Smedsrud et al., 2013) and north of Svalbard (Ivanov et al., 2012; Onarheim et al., 2014). However, based on mooring and buoy data, Polyakov et al. (2017, 2020) showed that Atlantification is progressing eastward, impacting ocean stratification and sea ice growth even in the main regions of Arctic sea ice formation in and around the Laptev Sea.
Figure 4 summarizes the relevant parameters and conditions for the observed modal SIT minimum in the AOI in 2016 and the potential linkage to intensified AW heat flux events along the Russian shelf. Polyakov et al. (2017) observed a significant Atlantification event with increased upward transport of AW heat at multiple mooring sites in the northern and eastern Laptev Sea (Fig. 4a) between January and May 2015 (Fig. 3A in Polyakov et al., 2017). These moorings were part of a larger network and measured ocean properties between autumn 2013 and autumn 2015. The red coloured sections of the sea ice drift trajectories in Fig. 4a indicate that the ice sampled in the AOI in 2016 passed the region around the Laptev Sea moorings during the exact period of the observed Atlantification event, exposing the ice to increased ocean heat from below. As a result of the observed upward ocean heat flux, Polyakov et al. (2017) suggested reduced sea ice growth of approximately 0.4 m for the 2015 winter period. Satellite-derived mean SIT from the ESA Climate Change Initiative Phase 2 (CCI-2) climate data record confirms that a negative SIT anomaly existed in the Laptev Sea during this Atlantification event in 2015 (Fig. 4b). This anomaly is likely a result of multiple factors, including increased upward ocean heat fluxes due to Atlantification, but also the observed increase in ice export from the Russian shelves (Krumpen et al., 2013; Itkin and Krumpen, 2017) and ocean heat as a result of increased solar energy input during ice-free periods (Perovich et al., 2011; Wang et al., 2016).
In order to quantify the impact of increased upward ocean heat flux on sea ice in the Laptev Sea and in the AOI, we adjusted the thermodynamic model to provide a constantly higher ocean heat flux value along the 2016 sea ice trajectories during the Atlantification event observed by Polyakov et al. (2017) in winter 2015 (Fig. 3c). Conservative estimates of ocean heat flux during the Atlantification event between January and May 2015 (shaded red area) vary between averages of 4 W m−2 from moorings closer to the Laptev Sea shelf (Polyakov et al., 2020) and averages of 8 W m−2 estimated for the moorings further north (Polyakov et al., 2017). Based on these estimates we adjusted the model to provide both values along the red parts of the sea ice trajectories (Fig. 4a), which resulted in a mean reduction of SIT of 0.06 (4 W m−2 run) and 0.20 m (8 W m−2 run) at the end of the winter (May 2015, Fig. 4c). These values are significantly lower than the 0.4 m reduced sea ice growth suggested by Polyakov et al. (2017), which indicates that our adjusted heat fluxes might still be too low. However, the model confirms that increased ocean heat flux reduces sea ice growth in the Laptev Sea. In the AOI, modelled SIT reduced by 0.03 (4 W m−2 run) and 0.10 m (8 W m−2 run) in July 2016 (Fig. 4c). Although the adjusted model runs show that SIT anomalies induced by increased upward-directed ocean heat fluxes at the beginning of the drift trajectories persist, they are not able to fully overcome the overestimation of observed SIT in the AOI. The adjusted model assumptions about ocean heat fluxes are still too crude and also neglect the influence of ocean heat resulting from solar energy input through open water areas. It is clear that a more realistic representation of ocean heat fluxes along sea ice trajectories is required. Additionally, more data are needed to determine the spatial extent to which Atlantification and ocean heat fluxes in general affect sea ice growth. Nevertheless, the presented downstream EM measurements of SIT and our model analyses suggest that the winter 2015 SIT anomaly in the Laptev Sea persisted into the central Arctic Ocean and was ultimately recorded in the AOI as late as summer 2016. In general, atmospheric influences are able to induce SIT anomalies similar to the one measured in the AOI in 2016. However, there is no indication that increased air temperatures at the sea surface between May and August 2016 (Fig. 4d), or along the trajectories of the ice sampled in the AOI in 2016 (Fig. S2), resulted in the measured AOI modal SIT minimum. We therefore consider the ice conditions measured in the AOI in 2016 to be partially the result of extreme events of intensified upward ocean heat fluxes that occurred between ice formation in autumn 2014 and sampling in July–August 2016. Our adjusted upward-directed ocean heat fluxes were not able to explain the entire offset between modelled and measured modal SIT in the AOI, which is likely the result of conservative estimates, insufficient temporal and spatial representation of upward-directed AW heat fluxes, and the negligence of ocean heat resulting from solar energy input during open water periods in the model. Additionally, snow and surface melt processes altering SIT are likely responsible for a considerable fraction of the observed difference between modelled and observed SIT in the AOI in 2016 as well. Both snow depth (Merkouriadi et al., 2017, 2020) and surface melt (Perovich et al., 2014) can vary substantially from year to year and also from the assumptions applied for the presented model. Especially a thick snowpack, like has been observed during the 2015 N-ICE campaign (Granskog et al., 2016; Merkouriadi et al., 2017), could significantly limit sea ice bottom growth through thermal insulation (Merkouriadi et al., 2020). However, with limited observations of long-term snow depth and surface melt processes along the investigated sea ice trajectories, we resorted to available climatologies and parameterizations.
We argue that the analysis of the 2016 IceBird SIT data allows for a first estimation of the impact of Atlantification on sea ice, its thickness, and the timescale on which these signals can persist. However, it has to be noted that the upward AW heat fluxes vary in strength from year to year, but Atlantification is a process that continuously increases in the eastern marginal ice zone. In fact, Polyakov et al. (2020) showed that the influence of AW heat flux on the ice in the Laptev Sea showed a dramatic increase during the last decade. The upward-directed AW heat fluxes in the Laptev Sea showed an increase during winter periods between 2007/2008 and 2018 that resulted in a more than 2-fold reduction of winter ice growth in the last decade (Polyakov et al., 2020).
The example of the 2016 minimum in modal SIT in the AOI is a first indication that the increasing influence of Atlantification potentially persists far beyond the eastern Arctic shelf regions due to its preconditioning effect on SIT. However, to further confirm this discovery, it is vital to build continuous long-term SIT time series in the Laptev Sea as well as in the vicinity of the Fram Strait. The current IceBird SIT time series in the AOI is not sufficiently continuous to fully confirm that the AW heat flux preconditioning of sea ice in the Laptev Sea is still measurable in Fram Strait. The measurement gap between 2012 and 2016 prevented us from potentially discovering the influence of Atlantification on SIT in the AOI earlier. The ice sampled in the AOI in 2017 originated largely from the Beaufort Sea and accordingly did not show the signature of Atlantification. The 2018 ice was formed in the Laptev Sea in autumn 2015. However, it passed the shelf region where Atlantification was prominent in previous years in winter 2016 when the stratification was strong, and upward AW heat flux was anomalously weak for the new regime discovered by Polyakov et al. (2020). According to Polyakov et al. (2020) the strongest recorded upward AW heat flux, so far, occurred in winter 2018. The averages of AOI sea ice age indicate that the ice influenced by this event likely reached the AOI in 2019. Unfortunately, no IceBird campaign was conducted in the AOI in summer 2019. These missing measurements only confirm the importance of yearly IceBird campaigns in the AOI to further investigate the strength of the preconditioning of sea ice, as well as the persistence of SIT anomalies due to oceanic influences.
3.3 Interpretation of sea ice surveys from the MOSAiC year
The continuation of the IceBird SIT time series in the MOSAiC year 2020 was aggravated by the COVID-19 pandemic which only allowed for survey flights over the AOI from Longyearbyen (Svalbard) and after the usual sampling period from mid-July to mid-August (Table 1). Mean and modal SIT values were obtained over the AOI in September 2020 and are shown in Fig. 1b. The pathway analysis (Fig. 2) confirms the trend that ice reaching the AOI in summer is increasingly dominated by SYI (Fig. 1c). Although the modal SIT is similar to the 2016 value, it has to be noted that measurements were conducted considerably later in the melt season, which makes a direct comparison difficult and shows that summer melt has a considerable impact on SIT in the AOI.
Due to the late IceBird MOSAiC campaign in 2020 and the ensuing limitations for the comparability to the existing IceBird time series, we turn to the only other available SIT data set that was obtained in the AOI during the relevant period between mid-July and mid-August of 2020 – GEM SIT measurements from the MOSAiC floe. Compared to the areal coverage achievable with the AEM, GEM SIT values provide point measurements that are only partly representative for a larger area. Nevertheless, these floe-scale measurements provide the means for an important first estimation of whether the MOSAiC floe is thicker or thinner compared to the sea ice that was sampled in the AOI in the years prior to 2020.
The MOSAiC Central Observatory (CO) and the ice in its immediate vicinity (radius of approximately 40 km), which accommodated the Distributed Network (DN) of various autonomous measurement stations (Krumpen and Sokolov, 2020), was formed during a polynya event north of the New Siberian Islands (Fig. 2) in early December 2018 (Krumpen et al., 2020). The ice originated in shelf waters less than 10 m deep, drifted eastward along the shallow shelf, and ultimately reached deeper waters in February 2019. By the time the German icebreaker RV Polarstern (Alfred Wegener Institute, 2017) moored to the floe in October 2019 (beginning of the drift at 85∘ N and 136∘ E; see Fig. S3), the CO and DN regions (DNR) were surrounded by thicker residual ice that was formed in early November 2018 (Krumpen et al., 2020). Due to the comparably fast drift along the Transpolar Drift (the floe was only about 1.65 years old when it was sampled in the AOI, Fig. 3a) the DNR reached the southernmost border of our selected AOI already in the second half of July 2020 (Figs. 2 and S3). Along its trajectory through the Arctic Ocean DNR, SIT was continuously measured using ground-based and airborne EM devices. Unfortunately, technical problems and unfavourable weather conditions limited the availability of SIT measurements covering larger areas in the vicinity of the floe in the second half of July 2020. However, regular GEM measurements were conducted on the remainders of the CO. The GEM thickness results shown here are based on the rapid-release quick-view thickness data, have undergone initial quality control, and have been calibrated against manual observations. In order to ensure the best possible comparability to the IceBird SIT time series, we only consider GEM measurements that were conducted while the floe was in the AOI and during the sampling period of the previous measurements. The resulting preliminary AOI SIT values are based on a total of four surveys obtained between 16 and 21 July. Although GEM measurements were only conducted on the central, more stable part of the MOSAiC floe, large-scale AEM measurements conducted over the DNR in April 2020 indicate that modal and mean SIT values measured on the extended MOSAiC floe were representative for the DNR (Figs. S3 and S4). Additional AEM measurements conducted in the beginning of July 2020 confirm that the modal SIT derived from the GEM surveys is in fact reliable and representative for the wider area (Fig. S3 and S5).
On the basis of AEM surveys conducted over the DNR in April and early July 2020 and the already existing IceBird time series, we argue that the modal SIT of 1.71 m measured on the MOSAiC floe is not just representative for the wider area around the floe but also in line with measurements from previous years (Fig. 1b). The modal thickness of the MOSAiC floe is within 1 standard deviation of the long-term average over all modal SIT values derived for the AOI. This agreement indicates that the MOSAiC floe and its wider vicinity are not exceptional in terms of modal thickness compared to the long-term time series. The comparison of the MOSAiC floe modal SIT with SIT values reconstructed by the thermodynamic model from Thorndike (1992) confirms that the MOSAiC floe was not exceptionally thin. In fact, Fig. 3b shows an underestimation of modal SIT by the model. Despite the fact that when the MOSAiC floe reached the AOI it was of a similar age as the ice in 2016, modal SIT was considerably thicker. This indicates that the MOSAiC floe might have been less impacted by oceanic heat, among other factors, than the ice that reached the AOI in 2016.
Nevertheless, it is important to note that these results are preliminary. Detailed studies of the ice thickness development of the MOSAiC floe along its drift path through the Arctic and its surroundings are the basis for future studies.
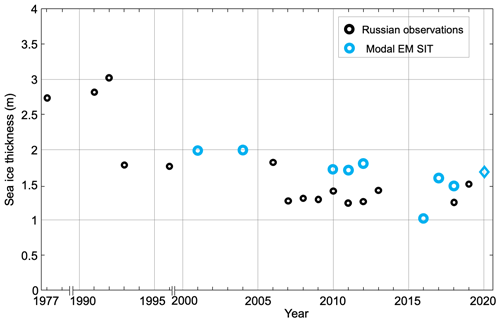
Figure 5Sea ice thickness observations conducted on regular tourist cruises from Franz Josef Land to the North Pole in July–August. SIT was determined using visual observations from AARI ice specialists or TV-complex calculations and averaged over a transect from 86 to 90∘ N, along the 50∘ E meridian (see black line Fig. 1a). Light blue markers indicate the time series of modal AOI EM SIT (Fig. 1b).
3.4 Comparison to Russian shipborne SIT observations
Due to the position of the selected AOI just north of the Fram Strait, the presented SIT time series provides the possibility to investigate interannual variability of the time-integrated signal of Arctic-wide SIT changes. Nevertheless, the selected AOI is a highly variable and small excerpt of the Arctic Ocean. Additionally, the presented time series is interrupted and still too short to provide insight into SIT changes on climatological scales. For example, the transition from a MYI-dominated towards a FYI/SYI-dominated Transpolar Drift (Kwok, 2018) that was accompanied by a drastic reduction in Arctic SIT and sea ice volume (Kwok, 2018; Spreen et al., 2020) and accelerated drift speeds along the Transpolar Drift (Spreen et al., 2011; Krumpen et al., 2019) between 2005 and 2007 is hardly recognizable in the presented IceBird time series. In order to determine whether the reduction in SIT in the AOI is also observed upstream in the central Arctic, we turn to the only continuous long-term SIT observational data set available close to the North Pole. Visual and STK July SIT observations obtained during Russian tourist cruises from Franz Josef Land to the North Pole (86 to 90∘ N along the 50∘ E meridian; see black line in Fig. 1a) confirm a step-wise decrease in SIT between 2005 and 2007 (Fig. 5), which is hardly visible in the AOI time series. The Russian observations in fact indicate that a similar regime shift already occurred between 1992 and 1993. Although SIT shows interannual variability, no trends are observed during each of the three regimes (prior to 1993, between 1993 and 2006, and from 2006 onwards). In years where both time series provide mean SIT values (2010 to 2012 and 2018) Russian observations tend to show thinner sea ice compared to IceBird measurements. Considering that the Russian observations were conducted during the same season as the IceBird measurements and upstream of the AOI, one would expect those values to be larger than the downstream AOI values. However, we attribute the lower estimates of SIT from the Russian observations to the inherent differences between the observation techniques. While visual and STK SIT observations are largely dependent on the ships' route and the avoidance of thicker ice patches for faster navigation through the ice, AEM measurements provide SIT distributions on larger spatial scales. We would therefore assume a bias towards, on average, thinner sea ice for the Russian observations compared to AEM measurements. Nevertheless, the Russian observations provide a much longer time series than the AOI measurements, which allows us to confirm general changes in the overall SIT regime in the central Arctic (Kwok, 2018). While the AOI time series indicate further thinning of sea ice between 2010 and 2020, Russian observations show no trend at all.
The length of the Russian observational time series and its ability to show the previously observed regime shift in SIT indicates how valuable consistent long-term time series are. However, the deviations from the AOI measurements also show its limitations and the importance of joint observations for a better understanding of differences and ultimately a better basis for the interpretation of past, present, and future ship-based observations from Russian sea ice experts.
This study provides an important extension of the long-term EM-derived summer SIT time series at the end of the Transpolar Drift presented by Krumpen et al. (2016). We combine these large-scale summer SIT measurements conducted within the framework of the IceBird and previous campaigns with Lagrangian ice tracking and a reconstructed SIT time series from a thermodynamic sea ice growth model (Thorndike, 1992). With this comprehensive approach we explain the observed interannual SIT variability within our selected area of interest (AOI, 80.5–86∘ N and 30∘ W–20∘ E) and investigate the driving mechanisms and source regions of this variability. Based on preliminary results from SIT measurements gathered during the MOSAiC drift experiment, we also put the MOSAiC floe into a historical context in terms of its thickness.
The analysis of pathways and sea ice origin with the Lagrangian ice tracking tool ICETrack reveals that approximately 65 % of the ice sampled in the AOI originated in the Laptev Sea. Sea ice reaching the end of the Transpolar Drift is thinning, which has also been shown by Hansen et al. (2013) and Renner et al. (2014) for sea ice reaching Fram Strait further south. AOI mean and modal SIT values decreased by about 20 % from 2001 to 2020. Most of the observed interannual variability in modal SIT is explained by the increase in drift speeds along the Transpolar Drift and the ensuing decrease in sea ice age. The fact that ice has less time to grow is also represented in the increasing fractions of FYI and especially SYI observed in the AOI. SIT measurements conducted on the MOSAiC floe, when it reached the southern border of the AOI in July 2020, show that the MOSAiC modal SIT is consistent with IceBird measurements from previous years.
The absolute modal SIT minimum that was measured in the AOI in 2016 is partly attributed to the influence of intensified upward Atlantic Water (AW) heat flux during a strong Atlantification event in the Laptev Sea in winter 2015. Using ICETrack we were able to detect that the ice sampled in the AOI in 2016 formed in autumn 2014 and passed the region where Polyakov et al. (2017, 2020) observed strong Atlantification between January and May 2015. Increased upward-directed ocean heat flux reduced ice growth in the Laptev Sea during this period. Based on the analysis with the thermodynamic sea ice growth model and the AOI EM SIT time series, we are able to show how persistent in time and space the impact of Atlantification on Arctic sea ice potentially is. It seems that, due to the fast drift across the Arctic Ocean, winter ice growth was not able to compensate for the low initial ice thickness after the Atlantification event. With a tendency towards even faster ice drift along the Transpolar Drift in the future, the impact of Atlantification on sea ice in the eastern marginal ice zone will increasingly be felt in other parts of the sea-ice-covered Arctic. The presented model analyses also revealed that the assumption of a constant and also our adjusted upward-directed ocean heat fluxes along the sea ice trajectories are insufficient to fully explain the observed modal SIT minimum in 2016. However, it is evident that the influence of oceanic heat, both upward-directed from AW at depth (Polyakov et al., 2017, 2020) and as a result of solar heating of the upper ocean during open water periods (McPhee et al., 2003; Wang et al., 2016), on sea ice is drastically increasing. Sea ice models require improved representations of spatial and temporal variability of ocean heat fluxes but also of melt processes and snow cover to reliably predict SIT changes along the Transpolar Drift.
Further investigations and measurements are required to monitor the development of Atlantification in the eastern marginal ice zones. But in order to strengthen our conclusion that Atlantification is able to precondition sea ice and that this preconditioning persists far beyond the eastern Arctic, additional uninterrupted SIT time series are vital along the pathways and at the exit gates of Arctic sea ice. The presented summer SIT time series at the end of the Transpolar Drift is an important effort to establish long-term and large-scale measurements of SIT, especially during the melt season. Airborne EM measurements of SIT during IceBird campaigns provide the necessary accuracy and areal coverage that is unmatched by any other non-satellite SIT measurement approach. Russian shipborne SIT measurements show significant differences to EM-based measurements, but their regularity and spatial consistency enable the depiction of regime shifts in SIT that are hardly resolved by the presented EM SIT time series. Obtaining SIT distributions over large areas and developing and continuing long-term SIT time series will provide unique input data for modelling efforts and ultimately will improve predictions of Arctic sea ice and its thickness in the future. Continuing regular IceBird measurement campaigns in the vicinity of Fram Strait and combining the results with reliable models, ice tracking tools, and additional upstream and downstream SIT data sets, like the Russian shipborne observations and the Fram Strait ULS time series (Hansen et al., 2013; Renner et al., 2014; Spreen et al., 2020), will prove indispensable for monitoring the complex and radical change of sea ice in the Transpolar Drift system and on an Arctic-wide scale.
IceBird EM data are available on request from Thomas Krumpen and H. Jakob Belter (tkrumpen@awi.de, jbelter@awi.de).
Electromagnetic induction (EM Bird) raw data from the IceBird MOSAiC summer campaign 2020 are available on PANGAEA (https://doi.org/10.1594/PANGAEA.924916, Belter et al., 2020b).
All MOSAiC-related data are archived in the MOSAiC Central Storage (MCS) and will be available on PANGAEA after finalization of the respective datasets according to the MOSAiC data policy.
Sea ice concentration data utilized by ICETrack are provided by CERSAT and available here: ftp://ftp.ifremer.fr/ifremer/cersat/products/gridded/psi-concentration/data/ (last access: 17 June 2020; Ezraty et al., 2007).
The EUMETSAT Ocean and Sea Ice Satellite Application Facility (OSI SAF) provided the global sea ice concentration (interim, 2016 onwards) climate data record 1979–2015 (OSI-450 and OSI-430-b, http://osisaf.met.no/p/ice/, last access: 26 June 2020; Lavergne et al., 2019).
ESA Sea Ice Climate Change Initiative (Sea_Ice_cci): Northern Hemisphere sea ice thickness from ENVISAT satellite (Hendricks et al., 2018b) and from CryoSat-2 satellite (Hendricks et al., 2018a) on a monthly grid (L3C) v2.0 are available from the Centre for Environmental Data Analysis database.
Surface air temperature reanalysis data are provided by NOAA/OAR/ESRL PSL, Boulder, Colorado, USA, and available from https://www.psl.noaa.gov/data/gridded/data.ncep.reanalysis.surface.html (last access: 8 July 2020; Kalnay et al., 1996).
The supplement related to this article is available online at: https://doi.org/10.5194/tc-15-2575-2021-supplement.
HJB and TK analysed the sea ice thickness data and conducted the backward-tracking and model simulations for the sea ice sampled north of Fram Strait. HJB prepared the manuscript. All authors contributed to the discussion and provided input during the writing process. HJB, TK, and CH participated in the different expeditions during which the EM sea ice thickness data were gathered. AH was vital for the planning and organization of multiple IceBird campaigns including the one in 2020. IP provided ocean heat flux estimates, calculations for the model analysis, and valuable input to the manuscript. LvA, GB, IR, MW, and SH planned and conducted the GEM/EM surveys during Leg 4 of MOSAiC and processed the preliminary SIT data. RR provided preliminary airborne EM SIT data from Leg 3 of MOSAiC. TA, SVF, and SSS gathered and analysed the observational data from the Russian cruises to the North Pole.
All authors declare that they have no conflict of interest.
The 2020 AEM and GEM data used in this manuscript were produced as part of the international Multidisciplinary drifting Observatory for the Study of the Arctic Climate (MOSAiC20192020) during IceBird MOSAiC summer (P6-222_IceBird_MOSAiC_2020) and the RV Polarstern Legs 3 (AWI_PS122_03) and 4 (AWI_PS122_04).
We want to thank the AWI logistics department, the crews of the research aircraft Polar 5 and 6, the crews of Station North in Greenland, the pilots and crews on RV Polarstern (Alfred Wegener Institute, 2017), and the observers on the Russian icebreakers for their tireless efforts during the various expeditions. This unique collection of data from different expeditions would not exist without you!
Thank you to the two anonymous reviewers for their valuable and constructive comments and suggestions during the review process and to editor John Yackel and the team at The Cryosphere for their support and processing of our paper.
Finally, we would like to express our deepest gratitude and respect to Sergei V. Frolov, a leader of the Ice Navigation Laboratory in the Arctic and Antarctic Research Institute between 2000 and 2021. Sergei V. Frolov passed away in March 2021. He was a unique specialist in sea ice observations and hydrometeorological support of ice navigation and participant in numerous Arctic expeditions. His idea to use the TV complex to measure sea ice thickness has been successfully used during scientific expeditions since 2004.
This project was carried out in the framework of the BMBF-funded Russian-German cooperation QUARCCS (grant no. 03F0777A). The processing of visual and STK data by Tatiana A. Alekseeva, Sergei V. Frolov, and Sergei S. Serovetnikov was funded by the Russian Foundation for Basic Research (RFBR) under the research project number 18-05-60048.
The article processing charges for this open-access publication were covered by the Alfred Wegener Institute, Helmholtz Centre for Polar and Marine Research (AWI).
This paper was edited by John Yackel and reviewed by two anonymous referees.
AARI: Guidance to Special Shipborne Ice Observations, Arctic and Antarctic Research Institute, St. Petersburg, 2011. a, b, c
Alekseeva, T., Tikhonov, V., Frolov, S., Repina, I., Raev, M., Sokolova, J., Sharkov, E., Afanasieva, E., and Serovetnikov, S.: Comparison of Arctic Sea Ice Concentrations from the NASA Team, ASI, and VASIA2 Algorithms with Summer and Winter Ship Data, Remote Sensing, 11, 2481, https://doi.org/10.3390/rs11212481, 2019. a
Alfred Wegener Institute: Polar Research and Supply Vessel POLARSTERN Operated by the Alfred Wegener Institute Helmholtz Centre for Polar and Marine Research, Journal of Large-Scale Research Facilities, 3, A119, https://doi.org/10.17815/jlsrf-3-163, 2017. a, b, c
Assmy, P., Fernández-Méndez, M., Duarte, P., Meyer, A., Randelhoff, A., Mundy, C. J., Olsen, L. M., Kauko, H. M., Bailey, A., Chierici, M., Cohen, L., Doulgeris, A. P., Ehn, J. K., Fransson, A., Gerland, S., Hop, H., Hudson, S. R., Hughes, N., Itkin, P., Johnsen, G., King, J. A., Koch, B. P., Koenig, Z., Kwasniewski, S., Laney, S. R., Nicolaus, M., Pavlov, A. K., Polashenski, C. M., Provost, C., Rösel, A., Sandbu, M., Spreen, G., Smedsrud, L. H., Sundfjord, A., Taskjelle, T., Tatarek, A., Wiktor, J., Wagner, P. M., Wold, A., Steen, H., and Granskog, M. A.: Leads in Arctic pack ice enable early phytoplankton blooms below snow-covered sea ice, Sci. Rep., 7, 40850, https://doi.org/10.1038/srep40850, 2017. a
Belter, H. J., Krumpen, T., Hendricks, S., Hoelemann, J., Janout, M. A., Ricker, R., and Haas, C.: Satellite-based sea ice thickness changes in the Laptev Sea from 2002 to 2017: comparison to mooring observations, The Cryosphere, 14, 2189–2203, https://doi.org/10.5194/tc-14-2189-2020, 2020a. a
Belter, H. J., Krumpen, T., and Herber, A.: Electromagnetic induction raw data (EM Bird) of POLAR 6 during 2020 IceBird MOSAiC Summer campaign, PANGAEA [Dataset], https://doi.org/10.1594/PANGAEA.924916, 2020b. a
Cavalieri, D. J. and Parkinson, C. L.: Arctic sea ice variability and trends, 1979–2010, The Cryosphere, 6, 881–889, https://doi.org/10.5194/tc-6-881-2012, 2012. a
Damm, E., Bauch, D., Krumpen, T., Rabe, B., Korhonen, M., Vinogradova, E., and Uhlig, C.: The Transpolar Drift conveys methane from the Siberian Shelf to the central Arctic Ocean, Sci. Rep., 8, 4515, https://doi.org/10.1038/s41598-018-22801-z, 2018. a
Ezraty, R., Girard-Ardhuin, F., Piolle, J.-F., Kaleschke, L., and Heygster, G.: Arctic and Antarctic Sea Ice Concentration and Arctic Sea Ice Drift Estimated from Special Sensor Microwave Data, Spatial and Physical Oceanography Laboratory IFREMER, Brest, France and University of Bremen, Germany, version 2.1 Edn., 2007. a
Frolov, S. V., Klein, A. E., and Tretyakov, V. Y.: Results of using a digital TV complex for ice thickness measurements in the Arctic Basin in 2004-02005, Arct. Antarct. Res., 75, 123–127, 2007. a, b
Girard-Ardhuin, F. and Ezraty, R.: Enhanced Arctic Sea Ice Drift Estimation Merging Radiometer and Scatterometer Data, IEEE T. Geosci. Remote, 50, 2639–2648, https://doi.org/10.1109/TGRS.2012.2184124, 2012. a
Granskog, M., Assmy, P., Gerland, S., Spreen, G., Steen, H., and Smedsrud, L. H.: Arctic research on thin ice – Consequences of Arctic sea ice loss, Eos Trans. AGU, 97, 22–26, https://doi.org/10.1029/2016EO044097, 2016. a
Haas, C.: Late-summer sea ice thickness variability in the Arctic Transpolar Drift 1991–2001 derived from ground-based electromagnetic sounding, Geophys. Res. Lett., 31, 9, https://doi.org/10.1029/2003GL019394, 2004. a, b, c, d
Haas, C.: Sea ice thickness distribution, in: Sea ice, edited by: Thomas, D. N., chap. 2, 3rd Edn., John Wiley and Sons, Ltd, 42–64, https://doi.org/10.1002/9781118778371, 2017. a
Haas, C., Gerland, S., Eicken, H., and Miller, H.: Comparison of sea-ice thickness measurements under summer and winter conditions in the Arctic using a small electromagnetic induction device, Geophysics, 62, 749–757, https://doi.org/10013/epic.11729, 1997. a, b
Haas, C., Pfaffling, A., Hendricks, S., Rabenstein, L., Etienne, J.-L., and Rigor, I. G.: Reduced ice thickness in Arctic Transpolar Drift favors rapid ice retreat, Geophys. Res. Lett., 35, 17, https://doi.org/10.1029/2008GL034457, 2008. a, b, c
Haas, C., Lobach, J., Hendricks, S., Rabenstein, L., and Pfaffling, A.: Helicopter-borne measurements of sea ice thickness, using a small and lightweight, digital EM system, J. Appl. Geophys., 67, 234–241, https://doi.org/10.1016/j.jappgeo.2008.05.005, 2009. a
Haas, C., Hendricks, S., Eicken, H., and Herber, A.: Synoptic airborne thickness surveys reveal state of Arctic sea ice cover, Geophys. Res. Lett., 37, 9, https://doi.org/10.1029/2010GL042652, 2010. a, b
Hansen, E., Gerland, S., Granskog, M. A., Pavlova, O., Renner, A. H. H., Haapala, J., Loyning, T. B., and Tschudi, M.: Thinning of Arctic sea ice observed on Fram Strait: 1990–2011, J. Geophys. Res.-Oceans, 118, 5202–5221, https://doi.org/10.1002/jgrc.20393, 2013. a, b, c, d, e
Hendricks, S. and Ricker, R.: C3S_312b_Lot3_CLS_2018SC2 – Product User Guide and Specification, Copernicus Climate Change Service, available at: http://datastore.copernicus-climate.eu/documents/satellite-sea-ice/thickness/D3.SIT.1-v1.1_PUGS_of_v1_Sea_Ice_Thickness_Products_v1.3_APPROVED_Ver1.pdf (last access: 2 July 2020), 2019. a
Hendricks, S., Paul, S., and Rinne, E.: ESA Sea Ice Climate Change Initiative (Sea_Ice_cci): Northern hemisphere sea ice thickness from the CryoSat-2 satellite on a monthly grid (L3C), v2.0 (Oct 2020), DATA SET at Centre for Environmental Data Analysis: https://doi.org/10.5285/ff79d140824f42dd92b204b4f1e9e7c2, 2018a. a, b
Hendricks, S., Paul, S., and Rinne, E.: ESA Sea Ice Climate Change Initiative (Sea_Ice_cci): Northern hemisphere sea ice thickness from the Envisat satellite on a monthly grid (L3C), v2.0 (Oct 2020), DATA SET at Centre for Environmental Data Analysis: https://doi.org/10.5285/f4c34f4f0f1d4d0da06d771f6972f180, 2018b. a, b
Holland, M. M., Bitz, C. M., and Tremblay, B.: Future abrupt reductions in the summer Arctic sea ice, Geophys. Res. Lett., 33, 23, https://doi.org/10.1029/2006GL028024, 2006. a
Horvat, C., Jones, D. R., Iams, S., Schroeder, D., Flocco, D., and Feltham, D.: The frequency and extent of sub-ice phytoplankton blooms in the Arctic Ocean, Sci. Adv., 3, 3, https://doi.org/10.1126/sciadv.1601191, 2017. a
Hunkeler, P., Hendricks, S., Hoppmann, M., Farquharson, C., Kalscheuer, T., Grab, M., Kaufmann, M. S., Rabenstein, L., and Gerdes, R.: Improved 1D inversions for sea ice thickness and conductivity from electromagnetic induction data: Inclusion of nonlinearities caused by passive bucking, Geophysics, 81, WA45–WA58, https://doi.org/10.1190/GEO2015-0130.1, 2016. a
Itkin, P. and Krumpen, T.: Winter sea ice export from the Laptev Sea preconditions the local summer sea ice cover and fast ice decay, The Cryosphere, 11, 2383–2391, https://doi.org/10.5194/tc-11-2383-2017, 2017. a
Ivanov, V. V., Alexeev, V. A., Repina, I., Koldunov, N. V., and Smirnov, A.: Tracing Atlantic Water Signature in the Arctic Sea Ice Cover East of Svalbard, Adv. Meteorol., 2012, 201818, https://doi.org/10.1155/2012/201818, 2012. a
Johannessen, O. M., Bengtsson, L., Miles, M. W., Kuzmina, S. I., Semenov, V. A., Alekseev, G. V., Nagurnyi, A. P., Zakharov, V. F., Bobylev, L. P., Pettersson, L. H., Hasselmann, K., and Cattle, H. P.: Arctic climate change: observed and modelled temperature and sea-ice variability, Tellus A, 56, 328–341, https://doi.org/10.3402/tellusa.v56i4.14418, 2004. a
Kalnay, E., Kanamitsu, M., Kistler, R., Collins, W., Deaven, D., Gandin, L., Iredell, M., Saha, S., White, G., Woollen, J., Zhu, Y., Chelliah, M., Ebisuzaki, W., Higgins, W., Janowiak, J., Mo, K. C., Ropelewski, C., Wang, J., Leetmaa, A., Reynolds, R., Jenne, R., and Joseph, D.: The NCEP/NCAR 40-Year Reanalysis Project, B. Am. Meteorol. Soc., 77, 437–472, https://doi.org/10.1175/1520-0477(1996)077<0437:TNYRP>2.0.CO;2, 1996. a
Katlein, C., Arndt, S., Belter, H. J., Castellani, G., and Nicolaus, M.: Seasonal Evolution of Light Transmission Distributions Through Arctic Sea Ice, J. Geophys. Res.-Oceans, 124, 5418–5435, https://doi.org/10.1029/2018JC014833, 2019. a
Kern, S., Khvorostovsky, K., and Skourup, H.: D4.1 Product Validation and Intercomparison Report (PVIR-SIT) – SICCI-PVIR-SIT, Tech. rep., European Space Agency Sea Ice Climate Change Initiative, 2018. a
Kovacs, A. and Morey, R. M.: Sounding sea ice thickness using a portable electromagnetic induction instrument, Geophysics, 56, 1992–1998, https://doi.org/10.1190/1.1443011, 1991. a
Krishfield, R. A. and Perovich, D. K.: Spatial and temporal variability of oceanic heat flux to the Arctic ice pack, J. Geophys. Res., 110, C7, https://doi.org/10.1029/2004JC002293, 2005. a
Krumpen, T.: TIFAX 2012 Summer Campaign – Sea ice thickness measurements with Polar 5 from Station Nord and Svalbard, [Miscellaneous], https://doi.org/10013/epic.6e9bb068-dd33-49ff-8b7b-6cc02ec201e7, 2012. a
Krumpen, T.: AWI ICETrack: Antarctic and Arctic Sea Ice Monitoring and Tracking Tool, Vers. 1.3, https://doi.org/10013/epic.9ee550b6-5966-4db6-a042-f4256810ec3f, 2018. a, b, c
Krumpen, T. and Sellmann, M.: Campaign Report TIFAX 2016: Sea ice thickness measurements from Station Nord, Greenland, [Miscellaneous], https://doi.org/10013/epic.51537, 2016. a
Krumpen, T. and Sokolov, V.: The Expedition AF122/1: Setting up the MOSAiC Distributed Network in October 2019 with Research Vessel AKADEMIK FEDOROV, Berichte zur Polar-und Meeresforschung, Alfred Wegener Institute for Polar and Marine Research, 744, 119, https://doi.org/10013/epic.16bc0ab7-fe46-4fb5-ae69-3edc93a72356, 2020. a
Krumpen, T., Gerdes, R., and Herber, A.: TIFAX 2011 Summer Campaign – Sea ice thickness measurements with Polar 5 from Station Nord and Svalbard, [Miscellaneous], https://doi.org/10013/epic.2165dad3-b55c-48c1-9c6d-ca9969391809, 2011. a
Krumpen, T., Janout, M., Hodges, K. I., Gerdes, R., Girard-Ardhuin, F., Hölemann, J. A., and Willmes, S.: Variability and trends in Laptev Sea ice outflow between 1992–2011, The Cryosphere, 7, 349–363, https://doi.org/10.5194/tc-7-349-2013, 2013. a
Krumpen, T., Gerdes, R., Haas, C., Hendricks, S., Herber, A., Selyuzhenok, V., Smedsrud, L., and Spreen, G.: Recent summer sea ice thickness surveys in Fram Strait and associated ice volume fluxes, The Cryosphere, 10, 523–534, https://doi.org/10.5194/tc-10-523-2016, 2016. a, b, c, d, e
Krumpen, T., Sellmann, M., and Rohde, J.: TIFAX 2017 Campaign Report: Sea ice thickness measurements with Polar 6 from Station Nord and Alert, [Miscellaneous], https://doi.org/10013/epic.51536, 2017. a
Krumpen, T., Goessling, H., and Sellmann, M.: IceBird 2018 summer Campaign - Sea ice thickness measurements with Polar 6 from Station Nord and Alert, [Miscellaneous], https://doi.org/10013/epic.96923a78-d232-4bd8-82f4-337799d2fa07, 2018. a
Krumpen, T., Belter, H. J., Boetius, A., Damm, E., Haas, C., Hendricks, S., Nicolaus, M., Noethig, E.-M., Paul, S., Peeken, I., Ricker, R., and Stein, R.: Arctic Warming interrupts the Transpolar Drift and affects long-range transport of sea ice and ice-rafted matter, Nature Scientific Reports, 9, 5459, https://doi.org/10.1038/s41598-019-41456-y, 2019. a, b, c, d, e, f, g, h, i
Krumpen, T., Birrien, F., Kauker, F., Rackow, T., von Albedyll, L., Angelopoulos, M., Belter, H. J., Bessonov, V., Damm, E., Dethloff, K., Haapala, J., Haas, C., Harris, C., Hendricks, S., Hoelemann, J., Hoppmann, M., Kaleschke, L., Karcher, M., Kolabutin, N., Lei, R., Lenz, J., Morgenstern, A., Nicolaus, M., Nixdorf, U., Petrovsky, T., Rabe, B., Rabenstein, L., Rex, M., Ricker, R., Rohde, J., Shimanchuk, E., Singha, S., Smolyanitsky, V., Sokolov, V., Stanton, T., Timofeeva, A., Tsamados, M., and Watkins, D.: The MOSAiC ice floe: sediment-laden survivor from the Siberian shelf, The Cryosphere, 14, 2173–2187, https://doi.org/10.5194/tc-14-2173-2020, 2020. a, b, c, d, e, f
Kurtz, N. T., Farrell, S. L., Studinger, M., Galin, N., Harbeck, J. P., Lindsay, R., Onana, V. D., Panzer, B., and Sonntag, J. G.: Sea ice thickness, freeboard, and snow depth products from Operation IceBridge airborne data, The Cryosphere, 7, 1035–1056, https://doi.org/10.5194/tc-7-1035-2013, 2013. a
Kwok, R.: Arctic sea ice thickness, volume, and multiyear ice coverage: losses and coupled variability (1958–2018), Environ. Res. Lett., 13, 105005, https://doi.org/10.1088/1748-9326/aae3ec, 2018. a, b, c, d, e
Lavergne, T.: Validation and Monitoring of the OSI SAF Low Resolution Sea Ice Drift Product (v5), Technical report, The EUMETSAT Network of Satellite Application Facility, https://doi.org/10.13140/RG.2.1.4155.5449, 2016. a
Lavergne, T., Sørensen, A. M., Kern, S., Tonboe, R., Notz, D., Aaboe, S., Bell, L., Dybkjær, G., Eastwood, S., Gabarro, C., Heygster, G., Killie, M. A., Brandt Kreiner, M., Lavelle, J., Saldo, R., Sandven, S., and Pedersen, L. T.: Version 2 of the EUMETSAT OSI SAF and ESA CCI sea-ice concentration climate data records, The Cryosphere, 13, 49–78, https://doi.org/10.5194/tc-13-49-2019, 2019. a
Laxon, S. W., Giles, K. A., Ridout, A. L., Wingham, D. J., Willatt, R., Cullen, R., Kwok, R., Schweiger, A., Zhang, J., Haas, C., Handricks, S., Krishfield, R., Kurz, N., Farrell, S., and Davidson, M.: CryoSat-2 estimates of Arcitc sea ice thickness and volume, Geophys. Res. Lett., 40, 732–737, https://doi.org/10.1002/grl.50193, 2013. a
Lin, L. and Zhao, J.: Estimation of Oceanic Heat Flux Under Sea Ice in the Arctic Ocean, J. Ocean U. China, 18, 605–614, https://doi.org/10.1007/s11802-019-3877-7, 2019. a, b
Maykut, G. A.: Large-scale heat exchange and ice production in the central Arctic, J. Geophys. Res., 87, 7971–7984, https://doi.org/10.1029/JC087iC10p07971, 1982. a
Maykut, G. A. and McPhee, M. G.: Solar heating of the Arctic mixed layer, J. Geophys. Res.-Oceans, 100, 24691–24703, https://doi.org/10.1029/95JC02554, 1995. a
Maykut, G. A. and Untersteiner, N.: Some Results from a Time-Dependent Thermodynamic Model of Sea Ice, J. Geophys. Res., 76, 6, https://doi.org/10.1029/JC076i006p01550, 1971. a
McPhee, M. G., Kikuchi, T., Morison, J. H., and Stanton, T. P.: Ocean-to-ice heat flux at the North Pole environmental observatory, Geophys. Res. Lett., 30, 24, https://doi.org/10.1029/2003GL018580, 2003. a, b, c
Merkouriadi, I., Cheng, B., Graham, R. M., Roesel, A., and Granskog, M. A.: Critical Role of Snow on Sea Ice Growth in the Atlantic Sector of the Arctic Ocean, Geophys. Res. Lett., 44, 10479–10485, https://doi.org/10.1002/2017GL075494, 2017. a, b
Merkouriadi, I., Cheng, B., Hudson, S. R., and Granskog, M. A.: Effect of frequent winter warming events (storms) and snow on sea-ice growth – a case from the Atlantic sector of the Arctic Ocean during the N-ICE2015 campaign, Ann. Glaciol., 61, 164–170, https://doi.org/10.1017/aog.2020.25, 2020. a, b, c
Nicolaus, M., Katlein, C., Maslanik, J., and Hendricks, S.: Changes in Arctic sea ice result in increasing light transmittance and absorption, Geophys. Res. Lett., 39, 24, https://doi.org/10.1029/2012GL053738, 2012. a
NPI: Thickness of sea ice measured in the Fram Strait. Environmental monitoring of Svalbard and Jan Mayen (MOSJ), Norwegian Polar Institute, available at: http://www.mosj.no/en/climate/ocean/sea-ice-thickness-arctic-ocean-fram-strait.html (last access: 28 May 2020), 2018. a
Onarheim, I. H., Smedsrud, L. H., Ingvaldsen, R. B., and Nilsen, F.: Loss of sea ice during winter north of Svalbard, Tellus A, 66, 23933, https://doi.org/10.3402/tellusa.v66.23933, 2014. a
Overland, J., Dunlea, E., Box, J. E., Corell, R., Forsius, M., Kattsov, V., Olsen, M. S., Pawlak, J., Reiersen, L.-O., and Wang, M.: The urgency of Arctic change, Polar Sci., 21, 6–13, https://doi.org/10.1016/j.polar.2018.11.008, 2019. a
Overland, J. E. and Wang, M.: When will the summer Arctic be nearly sea ice free?, Geophys. Res. Lett., 40, 2097–2101, https://doi.org/10.1002/grl.50316, 2013. a
Peeken, I., Primpke, S., Beyer, B., Guetermann, J., Katlein, C., Krumpen, T., Bergmann, M., Hehemann, L., and Gerdts, G.: Arctic sea ice is an important temporal sink and means of transport for microplastic, Nat. Commun., 9, 1505, https://doi.org/10.1038/s41467-018-03825-5, 2018. a, b, c
Perovich, D., Richter-Menge, J., Polashenski, C., Elder, B., Arbetter, T., and Brennick, O.: Sea ice mass balance observations from the North Pole Environmental Observatory, Geophys. Res. Lett., 41, 2019–2025, https://doi.org/10.1002/2014GL059356, 2014. a
Perovich, D. K., Light, B., Eicken, H., Jones, K. F., Runciman, K., and Nghiem, S. V.: Increasing solar heating of the Arctic Ocean and adjacent seas, 1979–2005: Attribution and role in the ice-albedo feedback, Geophys. Res. Lett., 34, 19, https://doi.org/10.1029/2007GL031480, 2007. a
Perovich, D. K., Jones, K. F., Light, B., Eicken, H., Markus, T., Stroeve, J., and Lindsay, R.: Solar partitioning in a changing Arctic sea-ice cover, Ann. Glaciol., 52, 192–196, https://doi.org/10.3189/172756411795931543, 2011. a, b
Pfaffling, A., Haas, C., and Reid, J. E.: A direct helicopter EM sea ice thickness inversion, assessed with synthetic and field data, Geophysics, 72, 4, https://doi.org/10.1190/1.2732551, 2007. a, b
Pfirman, S., Haxby, W., Eicken, H., Jeffries, M., and Bauch, D.: Drifting Arctic sea ice archives changes in ocean surface conditions, Geophys. Res. Lett., 31, 19, https://doi.org/10.1029/2004GL020666, 2004. a, b, c
Pinker, R. T., Niu, X., and Ma, Y.: Solar heating of the Arctic Ocean in the context of ice-albedo feedback, J. Geophys. Res.-Oceans, 119, 12, https://doi.org/10.1002/2014JC010232, 2014. a
Polyakov, I. V., Pnyushkov, A. V., Alkire, M. B., Ashik, I. M., Baumann, T. M., Carmack, E. C., Goszczko, I., Guthrie, J., Ivanov, V. V., Kanzow, T., Krishfield, R., Kwok, R., Sundfjord, A., Morison, J., Rember, R., and Yulin, A.: Greater role for Atlantic inflows on sea-ice loss in the Eurasian Basin of the Arctic Ocean, Science, 356, 285–291, https://doi.org/10.1126/science.aai8204, 2017. a, b, c, d, e, f, g, h, i, j, k
Polyakov, I. V., Rippeth, T. P., Fer, I., Alkire, M. B., Baumann, T. M., Carmack, E. C., Ingvaldsen, R., Ivanov, V. V., Janout, M., Padman, S. L. L., Pnyushkov, A. V., and Rember, R.: Weakening of Cold Halocline Layer Exposes Sea Ice to Oceanic Heat in the Eastern Arctic Ocean, J. Climate, 33, 8107–8123, https://doi.org/10.1175/JCLI-D-19-0976.1, 2020. a, b, c, d, e, f, g, h, i, j
Rabenstein, L., Hendricks, S., Martin, T., Pfaffhuber, A., and Haas, C.: Thickness and surface-properties if different sea-ice regimes within the Arctic Trans Polar Drift: Data from summers 2001, 2004 and 2007, J. Geophys. Res., 115, C12, https://doi.org/10.1029/2009JC005846, 2010. a
Renner, A. H. H., Gerland, S., Haas, C., Spreen, G., Beckers, J. F., Hansen, E., Nicolaus, M., and Goodwin, H.: Evidence of Arctic sea ice thinning from direct observations, Geophys. Res. Lett., 41, 5029–5036, https://doi.org/10.1002/2014GL060369, 2014. a, b, c, d
Ricker, R., Hendricks, S., Helm, V., Skourup, H., and Davidson, M.: Sensitivity of CryoSat-2 Arctic sea-ice freeboard and thickness on radar-waveform interpretation, The Cryosphere, 8, 1607–1622, https://doi.org/10.5194/tc-8-1607-2014, 2014. a
Ricker, R., Hendricks, S., Kaleschke, L., Tian-Kunze, X., King, J., and Haas, C.: A weekly Arctic sea-ice thickness data record from merged CryoSat-2 and SMOS satellite data, The Cryosphere, 11, 1607–1623, https://doi.org/10.5194/tc-11-1607-2017, 2017. a
Smedsrud, L. H., Esau, I., Ingvaldsen, R. B., Eldevik, T., Haugan, P. M., Li, C., Lien, V. S., Olsen, A., Omar, A. M., Ottera, O. H., Risebrobakken, B., Sandø, A. B., Semenov, V. A., and Sorokina, S. A.: The role of the Barents Sea in the Arctic climate system, Rev. Geophys., 51, 415–449, https://doi.org/10.1002/rog.20017, 2013. a
Spreen, G., Kwok, R., and Menemenlis, D.: Trends in Arctic sea ice drift and role of wind forcing: 1992–2009, Geophys. Res. Lett., 38, 19, https://doi.org/10.1029/2011GL048970, 2011. a, b, c
Spreen, G., de Steur, L., Divine, D., Gerland, S., Hansen, E., and Kwok, R.: Arctic Sea Ice Volume Export Through Fram Strait From 1992 to 2014, J. Geophys. Res.-Oceans, 125, 6, https://doi.org/10.1029/2019JC016039, 2020. a, b, c, d, e
Stroeve, J. C., Kattsov, V., Barrett, A., Pavlova, T., Holland, M., and Meier, W. N.: Trends in Arctic sea ice extent from CMIP5, CMIP3 and observations, Geophys. Res. Lett., 39, 16, https://doi.org/10.1029/2012GL052676, 2012. a
Sumata, H., Lavergne, T., Girard-Ardhuin, F., Kimura, N., Tschudi, M. A., Kauker, F., Karcher, M., and Gerdes, R.: An Intercomparison of Arctic ice drift products to deduce incertainty estimates, J. Geophys. Res.-Oceans, 119, 4887–4921, https://doi.org/10.1002/2013JC009724, 2014. a
Thorndike, A. S.: A toy model linking atmospheric thermal radiation and sea ice growth, J. Geophys. Res.-Oceans, 97, 9401–9410, https://doi.org/10.1029/92JC00695, 1992. a, b, c, d, e, f, g, h
Tschudi, M., Meier, W. N., Stewart, J. S., Fowler, C., and Maslanik, J.: Polar Pathfinder Daily 25 km EASE-Grid Sea Ice Motion Vectors, Version 4, Boulder, Colorado USA, NASA National Snow and Ice Data Center Distributed Active Archive Center, https://doi.org/10.5067/INAWUWO7QH7B, 2019. a
Wang, C., Granskog, M. A., Hudson, S. R., Gerland, S., Pavlov, A. K., Perovich, D. K., and Nicolaus, M.: Atmospheric conditions in the central Arctic Ocean through the melt seasons of 2012 and 2013: impact on surface conditions and solar energy deposition into the ice-ocean system, J. Geophys. Res.-Atmos., 121, 1043–1058, https://doi.org/10.1002/2015JD023712, 2016. a, b, c
Wang, M. and Overland, J. E.: A sea ice free summer Arctic within 30 years?, Geophys. Res. Lett., 36, 7, https://doi.org/10.1029/2009GL037820, 2009. a
Warren, S. G., Rigor, I. G., and Untersteiner, N.: Snow Depth on Arctic Sea Ice, J. Climate, 12, 1814–1829, https://doi.org/10.1175/1520-0442(1999)012<1814:SDOASI>2.0.CO;2, 1999. a, b, c, d, e, f
WHOI: Upward-Looking Sonar data at BGEP Moorings from 2003 through 2013, Woods Hole Oceanographic Institution, available at: https://www.whoi.edu/page/preview.do?pid=66559 (last access: 28 May 2020), 2014. a
Zhang, J., Rothrock, D., and Steele, M.: Recent Changes in Arctic Sea Ice: the Interplay between Ice Dynamics and Thermodynamics, J. Climate, 13, 3099–3114, https://doi.org/10.1175/1520-0442(2000)013<3099:RCIASI>2.0.CO;2, 2000. a