the Creative Commons Attribution 4.0 License.
the Creative Commons Attribution 4.0 License.
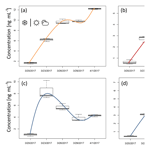
Brief communication: Analysis of organic matter in surface snow by PTR-MS – implications for dry deposition dynamics in the Alps
Dušan Materić
Elke Ludewig
Kangming Xu
Thomas Röckmann
Rupert Holzinger
The exchange of organic matter (OM) between the atmosphere and snow is poorly understood due to the complex nature of OM and the convoluted processes of deposition, re-volatilisation, and chemical and biological processing. OM that is finally retained in glaciers potentially holds a valuable historical record of past atmospheric conditions; however, our understanding of the processes involved is insufficient to translate the measurements into an interpretation of the past atmosphere. This study examines the dynamic processes of post-precipitation OM change at the alpine snow surface with the goal of interpreting the processes involved in surface snow OM.
- Article
(2375 KB) - Full-text XML
- BibTeX
- EndNote
Organic matter (OM) in the cryosphere originates from different sources (e.g. oxidation products of anthropogenic, biogenic, and biomass burning volatile organic compounds – VOCs), is transported from short and long distances, and is deposited via dry or wet deposition (Antony et al., 2014). From the moment of the emission, OM undergoes atmospheric chemistry processes, which profoundly alter the chemical composition of OM, resulting in numerous chemical species that are finally deposited on the snow/ice surface (Legrand et al., 2013; Müller-Tautges et al., 2016). Fingerprints of OM stored in the snow and ice therefore potentially hold a rich historical record of atmospheric chemistry processes and the transport pathways in the atmosphere (Fu et al., 2016; Giorio et al., 2018; Grannas et al., 2006; Pokhrel et al., 2016).
The vast diversity of OM, which is found in snow and ice samples, is impossible to characterise by one single method. The most used methods so far in snow/ice OM research are based on gas chromatography (GC) and liquid chromatography mass spectrometry (LC-MS) (Giorio et al., 2018; Gröllert et al., 1997). Novel high-resolution mass-spectrometry-based analytical methods, such as Fourier-transform ion cyclotron resonance mass spectrometry (FT-ICR-MS), Orbitrap mass spectrometry, and thermal desorption–proton transfer reaction–mass spectrometry (TD-PTR-MS), have recently been developed and can be used to characterise OM in the cryosphere with high mass resolution (Hawkes et al., 2016; Kujawinski et al., 2002; Marsh et al., 2013; Materić et al., 2017). Therefore, numerous new proxies are now potentially available to interpret the rich composition of OM in the cryosphere.
Reconstructing past atmospheric conditions from measurements of OM in the cryosphere is analytically challenging because of (1) low concentrations of target organics in the sample and (2) chemical changes that (might) happen after OM deposition. A recently developed method using TD-PTR-MS has partly solved the first issue, enabling the detection of low-molecular-weight OM ranging from 28 to 500 amu (Materić et al., 2017). However, chemical changes (e.g. photochemical, biological) and re-emission from the snow/ice surface still remain challenging to quantify, especially in the context of the diversity of OM species, both high- and low-molecular-weight OM.
The low-molecular-weight fraction represents an important part of OM in the cryosphere and the group includes VOCs and semi-volatile organic compounds (sVOCs) that deposit directly from the gas phase or as part of secondary organic aerosols (SOAs). Low-molecular-weight OM has been extensively studied in an atmospheric context by real-time or off-line PTR-MS techniques (Gkatzelis et al., 2018; Holzinger et al., 2010b, 2013; Materić et al., 2015; Timkovsky et al., 2015), however, not so in the context of deposited (e.g. dissolved) OM in the cryosphere. In this work, we applied a novel TD-PTR-MS method to measure concentrations of OM present in alpine snow. The first application of this new technique is investigation of snow–atmosphere interaction of OM during a dry weather period.
2.1 Sampling site
The snow samples were taken at 3106 m in altitude at Hoher Sonnblick, Austria, close to the research station Sonnblick Observatory. The sample site was next to the southern precipitation-measuring platform, which is about 50 m south-east of the observatory. The sampling location was carefully chosen to be least affected by potential contamination coming from the observatory. Average temperature of the site is about 1.1 ∘C in summer and −12.2 ∘C in winter considering the meteorological data being gathered since 1886.
The sample period spans the days from 20 March to 1 April 2017. During this time period the Sonnblick Observatory experienced an average day length of 12.5 h, an average temperature of −4 ∘C, 78 % relative humidity, an average wind speed of 7.3 m s−1, and a pressure of 696 hPa. There was no significant precipitation observed but these days were mostly foggy in the morning with the exception of 27 and 28 March 2017, which were nearly clear-sky days, followed by less cloudy days till 1 April 2017. The measured air temperatures (2 m above the surface) at the site were below zero for the whole time, with the exception of three brief instances when the temperature was recorded at 0.1 ∘C for 10 min (Fig. 1a). However, hourly temperature averages for these events were also <0 ∘C. If we use a positive degree-day (PDD) model to assess the melting possibility of those single 10 min periods, we calculated the depth of the meltwater to be 1.4–5.5 µm (using the snow melt factor of 2–8 ) (Singh et al., 2000). Thus, we conclude that no significant melting and runoff happened for the entire sampling period.
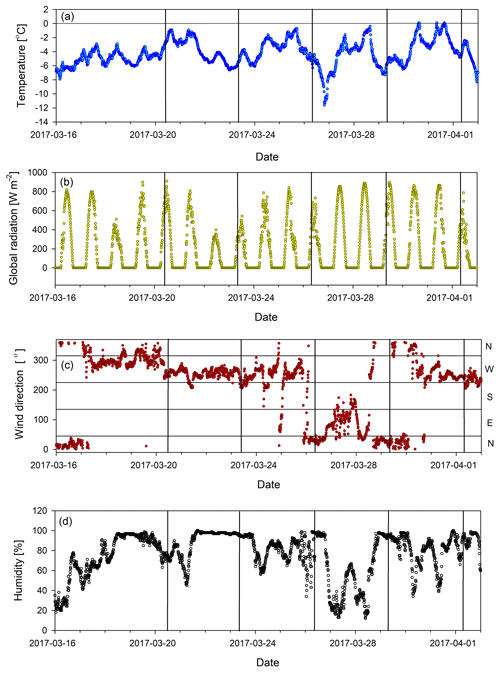
Figure 1Meteorological data measured at Sonnblick station during the sampling: (a) temperature, (b) global radiation, (c) wind direction, (d) relative humidity. Vertical lines represent the sampling time. Note the wind direction change in the period preceding the sampling on 29 March 2017.
More information on the meteorological conditions can be found in Figs. 1 and A1.
2.2 Sampling
Snow samples were taken every third day from the surface snow (<2 cm), scooping the snow directly into clean 50 mL polypropylene vials. We also took field blanks (ultrapure water) to assure that our blanks were exposed to the same impurities as the snow samples. The samples were stored in a freezer at −20 ∘C until the end of the sampling campaign and then shipped on dry ice to the analysis lab, where they were kept frozen until the analysis.
2.3 Analysis
Prior to the analysis, the samples (and blanks) were melted at room temperature and filtered through a 0.2 µm PTFE filter. We loaded 1 mL of each sample into clean 10 mL glass vials that had been prebaked at 250 ∘C overnight. The samples together with the field blanks were dehydrated using a low-pressure evaporation–sublimation system and analysed by TD-PTR-MS (PTR-TOF 8000, IONICON Analytik), following the method described before (Materić et al., 2017). The samples (triplicates) were run randomly and the blanks (four replicates) were run in between covering the entire period of the experiment. PTR conditions included a drift-tube pressure of 295 Pa, drift-tube temperature of 120 ∘C, and drift-tube voltage of 603 V, yielding E ∕ N 122 Td. The thermal desorption procedure was optimised for snow-sample analysis and has the following temperature sequence: (1) 1.5 min incubation at 35 ∘C, (2) ramp to 250 ∘C at a rate of 40 , (3) 5 min at 250 ∘C, and (4) cooling down to <35 ∘C. The method is fast (<15 min per run), sensitive (e.g. limit of detection (LoD) <0.17 ng mL−1 for pinonic acid, LoD <0.26 ng mL−1 for levoglucosan), requires a small sample size (<2 mL of water), and provides reasonably high-mass-resolution data (>4500, full width at half maximum, FWHM).
For the data analysis, we used the custom-made software package PTRwid for peak integration and identification and R scripts for statistical analyses (linear regression, fitting, etc.) (Holzinger, 2015). We used 3σ of the field blanks for estimating the LoD, so only ions that are above this value were taken into account for the scientific interpretation (Armbruster and Pry, 2008). We evaluated the impurities in the field blanks by comparing them with the system blanks (clean vials) and discovered that the average impurity level of a field blank was reasonably low (7.0 ng mL−1), which mostly (60 %) originated from the ion m∕z 81.035 (C5H4OH+). The impurities here might originate from the polypropylene vials we used; however, the levels are much lower compared to the methods used for measuring total and dissolved OM (Giorio et al., 2018). The impurities were taken into account by means of field blank subtraction and LoD filtering (Materić et al., 2017).
From the mass spectra, identified peaks were integrated over 8 min starting when the temperature in the TD system reached 50 ∘C. Extracted peaks were quantified by PTRwid and the concentration was expressed in nanograms per millilitre of sample. We calculated the molar concentration of C, H, O, and N for each sample, from which atomic ratios (O∕C, H∕C, N∕C), mean carbon number (nC), and mean carbon oxidation state (OSC) are calculated as described earlier (Holzinger et al., 2013; Materić et al., 2017). For the elemental composition calculation, we excluded ions as these are dominated by thermal dissociation products of non-volatile high-molecular-weight compounds. Taking into account these fragments of bigger molecules would substantially alter elemental composition and atomic ratios.
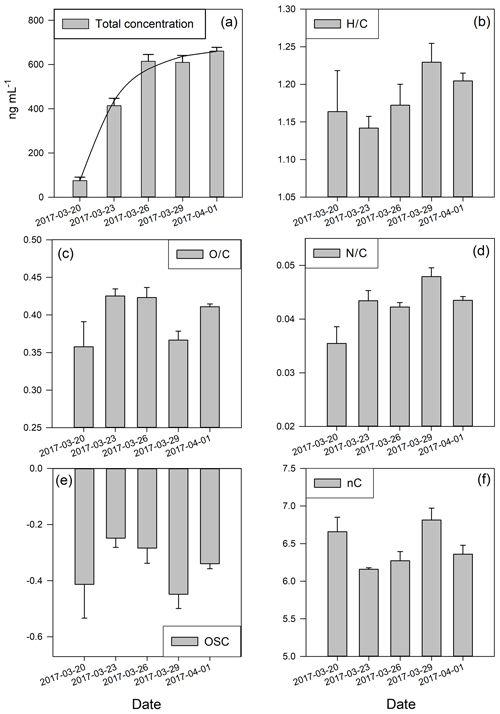
Figure 2Total concentration of organic ions and cumulative metrics of atomic ratio distribution. (a) Total concentration in nanograms per millilitre; the line represents the fit from the simple deposition model explained in the text (Eq. 1); (b) H∕C ratio; (c) O∕C ratio; (d) N∕C ratio; (e) oxidative state of carbon; (f) mean numbers of carbon. The error bars represent the standard deviation of three replicates.
3.1 Total ion concentration and simple mass balance model
During our sampling period, the total concentration of organics increases in general over the time that the snow was exposed to the atmosphere (Fig. 2a). The concentration of organics in the snow surface reflects a dynamic balance between two opposing processes that work independently: deposition as source and loss. If we consider just dry deposition (it was a period without precipitation), the retained (actual) concentration of the organics in the snow can be described as
where m is the concentration of organics remaining in the snow, D is the total dry deposition rate, and L is the overall loss rate due to re-volatilisation, photochemical reactions, biological processes, etc. As our samples generally show an increase in the ion concentrations (Figs. 2 and 3), the loss rate by re-volatilisation, photochemical reaction, and biological decay is lower than the total deposition rate (D>L). A negative mass balance, i.e. D<L, can happen, for example, in periods of extensive photochemical reactions together with snow exposure to an air mass with a low concentration of OM.
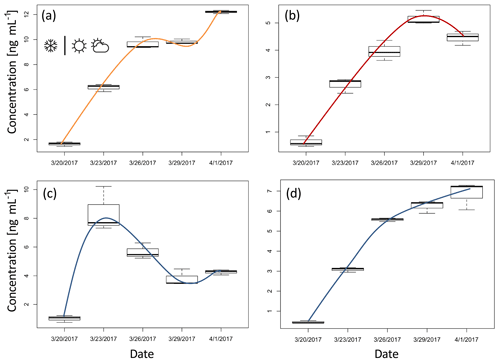
Figure 3Box plots of concentration for ions representing four distinctive groups (tick line of a box represents the median and upper and lower line maximum and minimum values): (a) ion m∕z 115.070 – pinonic acid, (b) ion m∕z 85.029 – levoglucosan, (c) ion m∕z 99.008, and (d) ion m∕z 159.065. The lines illustrate the change in the concentration over the time that is typical for each group. The first sample is taken just after the precipitation (snow symbol), followed by a non-precipitation period for the rest of the experiment (other weather symbols).
Our total concentration data (as well as many individual ion groups; see below) indicate a relaxation towards a source–sink equilibrium. Mathematically, the simplest model that has these characteristics is a system with quasi-constant deposition rate D (i.e. changes in deposition are much slower than changes in the loss rate) and a first-order loss rate ( in Eq. 1), which can be integrated to yield
where m0 is the initial concentration of m, k is the first-order loss rate coefficient, and t is time. In our experiment, we measured m with a time step t of 3 days and consider m0 to be our measurement of the fresh snow in the beginning of the analysis period. Equation (2) can then be fit to the data and the best fit for the total concentration of semi-volatile organic traces (R2>0.9899 and rRMSE<3.5 %) was found for k=0.31 day−1 and D=206 . When the fit is applied to the mass of carbon in the detected organics, the best-fit values for the two parameters are k=0.30 day−1 and D=114 , respectively. Considering reported average organic aerosol (OA) concentration we assume the winter air concentration (C) to be at most 2 µg C m−3 (Guillaume et al., 2008; Holzinger et al., 2010a; Strader et al., 1999). Further taking an average sampling depth of 2 cm and a snow density of 250 mg mL−1 we calculated a deposition velocity of 0.33 cm s−1 according to Eq. (3):
where D is the measured deposition rate, C is concentration (2 µg C m−3), and A is the area that was typically sampled (combining sampling depth and snow density relates 1 mL of the sample to an area of 2 cm2). Assuming slightly higher (3 µg C m−3) or lower (1 µg C m−3) OA concentration in air and sampling variation between 1.5 and 2 cm in depth we calculated a positive error of a factor of 2 and a negative error of a factor of 2−1. Thus, a deposition velocity for OAs of 0.17–0.66 cm s−1 would be required to be consistent with the observations. However, the deposition velocities for OA were previously estimated to be 0.034±0.014 and 0.021±0.005 cm s−1 for particles in the 0.15–0.3 and 0.5–1.0 µm size ranges (Duan et al., 1988; Gallagher et al., 2002). The required deposition velocities are approximately an order of magnitude higher than the previously reported estimates even if we use the upper limit of expected OA concentration (2 µg C m−3). Therefore, we conclude that the dominating contribution to OM in the snow is from gas-phase sVOCs. As direct measurements of bulk sVOCs do not exist, we estimated the required average loads of sVOCs in the air passing the sampling location to explain the observations. Using the deposition rate calculated from our measurements (Eq. 2), the concentration-weighted average molecular mass of measured compounds, and deposition velocities of 1 cm s−1 (assuming that sVOC deposition velocities are similar to that of formic acid) (Nguyen et al., 2015), we calculated an average gas-phase sVOC burden of 883 ng m−3 of air which is equivalent to 247 ppt. Assuming slightly higher or lower deposition velocities (±0.2 cm s−1) yields errors of +221 and −148 ng m−3, or +62 and −41 ppt. Our calculated value of average sVOC concentration agrees with previous estimates of 600 ng m−3 (Zhao et al., 2014). Thus, our data suggest that dynamic processes of dissolved organic matter (DOM) on the surface snow are dominated by deposition and re-volatilisation of gas-phase sVOCs. This has important implications for our understanding of the snow surface processes. Our analysis suggests that air masses with different sVOC composition can leave different OM fingerprints in the snow (discussed in the sections below).
The D∕k ratio quantifies the equilibrium point (asymptote) for the model described in Eq. (2). This represents a point at which the equilibrium is established between deposition and losses. The derived time constant for loss of about 3 days implies that 90 % equilibrium is established for the total ion concentration in only 6 days. This value, however, represents an average equilibrium time for total measured DOM, and it is reasonable to assume that this equilibration timescale differs among different compounds. In particular, it is estimated to be established much faster for the gas-phase sVOCs compared to SOA.
Similar mass balance calculations will be carried out in the following section for individual ion groups.
3.2 Grouping of ions with similar time evolution
In the data analysis of TD-PTR-MS spectra, we found 270 organic ions above the detection limit present in the samples. Compounds that have the same origin (similar sources or atmospheric chemistry processes) should feature similar time evolution, if the lifetime is not so short that such a common time evolution is lost. Based on the pattern of concentration change over time (using a linear regression model) we identified four groups of ions with a similar time evolution (Fig. 3, Table A1). In groups 1, 2, 3, and 4 we assigned 25, 33, 9, and 21 ions, respectively (88 ions in total 33 %), and 175 ions did not fall into any of these groups. Ions which we did not assign to any group either showed different time evolution or had concentrations close to the detection limit causing poor correlation. On average, the total concentration levels of the ions within the four groups were 30, 56, 16, and 57 ng mL−1 and 315 ng mL−1 for ions which did not fall into any of the described groups. Specific information can be found in Materić (2019). These levels of OM retrieved by PTR-MS agree with previous measurements at the site, although different methods have been used (Gröllert et al., 1997).
In the first two groups (Fig. 3a and b), among the numerous ions we identified masses that we tentatively attribute to pinonic acid (m∕z 115.07 fragment) and levoglucosan (e.g. m∕z 85.03 and 97.03 fragments) (Salvador et al., 2016). Pinonic acid is an oxidation product of monoterpenes and the main source is expected to be emissions from surrounding alpine conifer forests; thus group 1 ions indicate air masses that were originally rich in biogenic VOCs, which have been processed during transport. Levoglucosan is a clear indicator of biomass burning and the most likely source during this period is domestic wood combustion. Therefore, we associate group 2 ions with the anthropogenic wood combustion sources and their products in complex atmospheric processing.
The compounds that fall in group 3 show, after an initial increase in the concentration on 23 March 2017, a decreasing trend (Fig. 3c; see also Table A1). The change in the concentration of the compounds constituting this group may point to a one-time significant pollution event which happened between 20 and 23 March. The total concentration of ions in this group was measured to be 34 ng mL−1 (8.2 % of the total organics) on 23 March 2017. This deposition event could have come from a single source; however higher-time-resolution measurements are needed to further characterise the potential source. As total concentration of ions in this group drops in 6 days below 20 ng mL−1 (3.1 % of the total organics), this group is also an example of how contaminated snow equilibrates with the cleaner atmosphere on timescales similar to those we derived from the simple box model.
As for total concentration, most of the ions and ion groups show an increase in the ion concentrations throughout the sampling period. Group 4 (Fig. 3d, Table A1) represents the compound group for which the concentration seems steadily increasing towards an equilibrium. This indicates that the simple mass balance model may be applicable, i.e. the assumption of a (close to) constant deposition and first-order loss rate. Therefore we also applied the simple mass balance model (see Sect. 3.1) to the individual ions in group 4 to investigate whether individual organic compounds have different k values. This is expected due to the different chemical and physical properties (such as volatility, susceptibility to photolysis, etc.) as well as different nutrition adequacy for potential biodegradation. For the sum of organic ions in group 4 (Fig. 3d, Table A1), k=0.20 day−1. Generally, the lower k value of this group compared to the total sVOC could be related to the fact that most of the ions here are heavier (thus less volatile). However, within this group k values of individual ions were found to be independent of the molecular weight and also independent of the composition, i.e. O∕C, H∕C, OSC, and nC (R2<0.12). As the volatility of sVOC is expected to depend on molecular weight and functional groups (longer sVOCs are in general less volatile, unless additional functional groups are involved), this suggests that volatility might not play the only role in the loss processes of this group.
A deviation in the general concentration trend in individual ions (from the expected growth, Sect. 3.1) was observed on 29 March 2017, particularly in groups 1 and 2 represented by pinonic acid and levoglucosan (Fig. 3a and b). Elevated levoglucosan and lower pinonic acid levels observed on 29 March are temporally related to a change in wind direction. On 29 March, the air masses originate from the north-east direction, rather than the north-west direction seen for other samples (Fig. 1c), so this event is attributed to the meteorological situation and possibly a more pronounced source of biomass burning following the transport regime at the time.
Presence of such distinctive patterns of concentration change over time, ion grouping, and their relation with the meteorological data indicate that meteorology and deposition of sVOCs after fresh precipitation strongly affect the organic composition in snow, which questions the most straightforward approach of interpreting OM signals in terms of OA in the air.
3.3 Elemental composition
We further investigate the processing of OM in snow during the study period by calculating cumulative metrics of the OM composition from the PTR-MS data, namely the elemental ratios O∕C and H∕C, nC, and OSC of the organic carbon, in order to further characterise the processes behind the observed changes. The fresh snow sample (20 April 2017) has the lowest total concentration of all measured organics, low OSC, the lowest O∕C and N∕C values, and high H∕C and nC values (Fig. 2), which all indicate “fresh” OM in the air (Kroll et al., 2011), which was captured in the snow.
An interesting signature in the metrics is observed on 29 March 2017 when the prevailing air flow regime was interrupted (wind direction change, Fig. 1c). This sample showed the highest value of nC, the lowest OSC, and elevated H∕C and low O∕C ratios (Fig. 2). This all indicates photochemically younger (fresher) emissions of VOCs and semi-volatiles, originating from air masses rich in biomass burning aerosols (Fig. 3b), which is in agreement with previous results linking low OSC and high nC to biomass burning aerosols (Kroll et al., 2011). However, on 29 March we also observed lower average total OM concentration in the sample compared to the previous period, which clearly indicates a net loss of OM. Potential processes that could explain such a loss of OM involve photolysis-induced re-volatilisation, OM runoff (e.g. snow melting), or oxidation. The photolysis-induced volatilisation should be higher for this sample as the previous days (27 and 28 March) had the highest global radiation values (33 % higher than the average for the sampling period) and the longest sunshine duration (>12 h) (Figs. 1b and A1). Conversely, no significant temperature increase has been measured to support increased melting and OM runoff. Loss by oxidation (referring to “dark” oxidation that is uncoupled from photooxidation) is also unlikely as a main process since the O∕C ratio did not increase for 29 March (Fig. 3c). Thus, the most likely cause of the lower total OM concentration observed on 29 March is re-volatilisation, possibly enhanced by photolysis, which would indicate that the air contained a lower burden of SVOCs. In addition, new OM material with different characteristics was deposited before that sample was collected. Combining all metrics (Fig. 2) and meteorological data available (Fig. 1), we can conclude that the air passing the site prior to 29 March 2017 was cleaner and photochemically younger and contained higher molecular weight compounds that might have originated from anthropogenic emissions such as biomass burning (high levels of levoglucosan, Fig. 3).
In this work, we analysed the concentrations of low-molecular-weight organic matter (20–500 amu) in alpine snow samples during a 12-day no-precipitation period, 20 March–1 April 2017. We noticed four distinctive groups of ions with a similar concentration trend over that time (R2>0.9), suggesting common sources, chemistry processes, or transport pathways. The largest two groups of ions came from (a) surrounding forests (e.g. pinonic acid – associated with monoterpene oxidation) and (b) residential fires (levoglucosan – common biomass burning marker). The snow sample taken on 29 March showed a change in the general concentration trend, consistent with a shift in wind direction, indicating different air mass origin. This is also in agreement with a change in atomic ratio metrics (O∕C, H∕C, OSC, and nC), which also indicated that re-volatilisation is the most important pathway of OM loss here, suggesting that the advected air was cleaner during this period. Dry deposition can be approximated by a mass balance model with a roughly constant deposition rate of D=206 and a first-order loss rate constant k=0.31 day−1. Calculated deposition velocities were inconsistent with the idea that OAs contribute the bulk of deposited OM; instead we suggest a dominant contribution of gas-phase sVOCs over the OA in the total bulk organic matter. This all indicates that, at least for this site and location, snow–atmosphere DOM exchange processes are mostly driven by gas-phase sVOCs, for which equilibration with air is fast. This has implications for the reconstruction of recent atmospheric conditions by analysis of organics in the snow.
Data are available in Materić (2019).
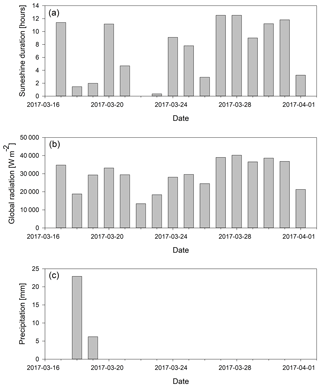
Figure A1Light conditions and precipitation during the sampling period. (a) Global radiation (W m−2) integrated for each day; (b) total daily sunshine duration in hours; (c) precipitation for the sampling period.
DM and RH designed the experiments and DM carried them out. EL provided the samples and meteorological data. DM prepared the paper with contributions from all co-authors.
The authors declare that they have no conflict of interest.
This work is supported by the Netherlands Earth System Science Centre (NESSC)
research network and by the Dutch NWO Earth and Life Science (ALW), project 824.14.002.
We thank the operators at the Sonnblick Observatory for taking
the samples.
Edited by: Martin Schneebeli
Reviewed by: two anonymous referees
Antony, R., Grannas, A. M., Willoughby, A. S., Sleighter, R. L., Thamban, M., and Hatcher, P. G.: Origin and sources of dissolved organic matter in snow on the East Antarctic ice sheet, Environ. Sci. Technol., 48, 6151–6159, https://doi.org/10.1021/es405246a, 2014.
Armbruster, D. A. and Pry, T.: Limit of Blank, Limit of Detection and Limit of Quantitation, Clin. Biochem. Rev., 29, S49–S52, 2008.
Duan, B., Fairall, C. W., and Thomson, D. W.: Eddy Correlation Measurements of the Dry Deposition of Particles in Wintertime, J. Appl. Meteorol., 27, 642–652, https://doi.org/10.1175/1520-0450(1988)027<0642:ECMOTD>2.0.CO;2, 1988.
Fu, P., Kawamura, K., Seki, O., Izawa, Y., Shiraiwa, T., and Ashworth, K.: Historical Trends of Biogenic SOA Tracers in an Ice Core from Kamchatka Peninsula, Environ. Sci. Technol. Lett., 3, 351–358, https://doi.org/10.1021/acs.estlett.6b00275, 2016.
Gallagher, M. W., Nemitz, E., Dorsey, J. R., Fowler, D., Sutton, M. A., Flynn, M., and Duyzer, J.: Measurements and parameterizations of small aerosol deposition velocities to grassland, arable crops, and forest: Influence of surface roughness length on deposition, J. Geophys. Res.-Atmos., 107, AAC 8-1–AAC 8-10, https://doi.org/10.1029/2001JD000817, 2002.
Giorio, C., Kehrwald, N., Barbante, C., Kalberer, M., King, A. C. F., Thomas, E. R., Wolff, E. W., and Zennaro, P.: Prospects for reconstructing paleoenvironmental conditions from organic compounds in polar snow and ice, Quaternary Sci. Rev., 183, 1–22, https://doi.org/10.1016/j.quascirev.2018.01.007, 2018.
Gkatzelis, G. I., Tillmann, R., Hohaus, T., Müller, M., Eichler, P., Xu, K.-M., Schlag, P., Schmitt, S. H., Wegener, R., Kaminski, M., Holzinger, R., Wisthaler, A., and Kiendler-Scharr, A.: Comparison of three aerosol chemical characterization techniques utilizing PTR-ToF-MS: a study on freshly formed and aged biogenic SOA, Atmos. Meas. Tech., 11, 1481–1500, https://doi.org/10.5194/amt-11-1481-2018, 2018.
Grannas, A. M., Hockaday, W. C., Hatcher, P. G., Thompson, L. G., and Mosley-Thompson, E.: New revelations on the nature of organic matter in ice cores, J. Geophys. Res.-Atmos., 111, D04304, https://doi.org/10.1029/2005JD006251, 2006.
Gröllert, C., Kasper, A., and Puxbaum, H.: Organic Compounds in High Alpine Snow, Int. J. Environ. Anal. Chem., 67, 213–222, https://doi.org/10.1080/03067319708031405, 1997.
Guillaume, B., Liousse, C., Galy-Lacaux, C., Rosset, R., Gardrat, E., Cachier, H., Bessagnet, B., and Poisson, N.: Modeling exceptional high concentrations of carbonaceous aerosols observed at Pic du Midi in spring–summer 2003: Comparison with Sonnblick and Puy de Dôme, Atmos. Environ., 42, 5140–5149, https://doi.org/10.1016/j.atmosenv.2008.02.024, 2008.
Hawkes, J. A., Dittmar, T., Patriarca, C., Tranvik, L., and Bergquist, J.: Evaluation of the Orbitrap Mass Spectrometer for the Molecular Fingerprinting Analysis of Natural Dissolved Organic Matter, Anal. Chem., 88, 7698–7704, https://doi.org/10.1021/acs.analchem.6b01624, 2016.
Holzinger, R.: PTRwid: A new widget tool for processing PTR-TOF-MS data, Atmos. Meas. Tech., 8, 3903–3922, https://doi.org/10.5194/amt-8-3903-2015, 2015.
Holzinger, R., Kasper-Giebl, A., Staudinger, M., Schauer, G., and Röckmann, T.: Analysis of the chemical composition of organic aerosol at the Mt. Sonnblick observatory using a novel high mass resolution thermal-desorption proton-transfer-reaction mass-spectrometer (hr-TD-PTR-MS), Atmos. Chem. Phys., 10, 10111–10128, https://doi.org/10.5194/acp-10-10111-2010, 2010a.
Holzinger, R., Williams, J., Herrmann, F., Lelieveld, J., Donahue, N. M., and Röckmann, T.: Aerosol analysis using a Thermal-Desorption Proton-Transfer-Reaction Mass Spectrometer (TD-PTR-MS): a new approach to study processing of organic aerosols, Atmos. Chem. Phys., 10, 2257–2267, https://doi.org/10.5194/acp-10-2257-2010, 2010b.
Holzinger, R., Goldstein, A. H., Hayes, P. L., Jimenez, J. L., and Timkovsky, J.: Chemical evolution of organic aerosol in Los Angeles during the CalNex 2010 study, Atmos. Chem. Phys., 13, 10125–10141, https://doi.org/10.5194/acp-13-10125-2013, 2013.
Kroll, J. H., Donahue, N. M., Jimenez, J. L., Kessler, S. H., Canagaratna, M. R., Wilson, K. R., Altieri, K. E., Mazzoleni, L. R., Wozniak, A. S., Bluhm, H., Mysak, E. R., Smith, J. D., Kolb, C. E., and Worsnop, D. R.: Carbon oxidation state as a metric for describing the chemistry of atmospheric organic aerosol, Nat. Chem., 3, 133–139, https://doi.org/10.1038/nchem.948, 2011.
Kujawinski, E. B., Freitas, M. A., Zang, X., Hatcher, P. G., Green-Church, K. B., and Jones, R. B.: The application of electrospray ionization mass spectrometry (ESI MS) to the structural characterization of natural organic matter, Org. Geochem., 33, 171–180, https://doi.org/10.1016/S0146-6380(01)00149-8, 2002.
Legrand, M., Preunkert, S., Jourdain, B., Guilhermet, J., Faïn, X., Alekhina, I., and Petit, J. R.: Water-soluble organic carbon in snow and ice deposited at Alpine, Greenland, and Antarctic sites: a critical review of available data and their atmospheric relevance, Clim. Past, 9, 2195–2211, https://doi.org/10.5194/cp-9-2195-2013, 2013.
Marsh, J. J. S., Boschi, V. L., Sleighter, R. L., Grannas, A. M., and Hatcher, P. G.: Characterization of dissolved organic matter from a Greenland ice core by nanospray ionization Fourier transform ion cyclotron resonance mass spectrometry, J. Glaciol., 59, 225–232, https://doi.org/10.3189/2013JoG12J061, 2013.
Materić, D.: Analysis of organic matter in surface snow by PTR-MS – implications for dry deposition dynamics in the Alps, Utrecht University, Utrecht, https://doi.org/10.24416/UU01-6LY8GT, 2019.
Materić, D., Bruhn, D., Turner, C., Morgan, G., Mason, N., and Gauci, V.: Methods in Plant Foliar Volatile Organic Compounds Research, Appl. Plant Sci., 3, 1500044, https://doi.org/10.3732/apps.1500044, 2015.
Materić, D., Peacock, M., Kent, M., Cook, S., Gauci, V., Röckmann, T., and Holzinger, R.: Characterisation of the semi-volatile component of Dissolved Organic Matter by Thermal Desorption – Proton Transfer Reaction – Mass Spectrometry, Sci. Rep.-UK, 7, 15936, https://doi.org/10.1038/s41598-017-16256-x, 2017.
Müller-Tautges, C., Eichler, A., Schwikowski, M., Pezzatti, G. B., Conedera, M., and Hoffmann, T.: Historic records of organic compounds from a high Alpine glacier: influences of biomass burning, anthropogenic emissions, and dust transport, Atmos. Chem. Phys., 16, 1029–1043, https://doi.org/10.5194/acp-16-1029-2016, 2016.
Nguyen, T. B., Crounse, J. D., Teng, A. P., St. Clair, J. M., Paulot, F., Wolfe, G. M., and Wennberg, P. O.: Rapid deposition of oxidized biogenic compounds to a temperate forest, P. Natl. Acad. Sci. USA, 112, E392–E401, https://doi.org/10.1073/pnas.1418702112, 2015.
Pokhrel, A., Kawamura, K., Ono, K., Seki, O., Fu, P., Matoba, S., and Shiraiwa, T.: Ice core records of monoterpene- and isoprene-SOA tracers from Aurora Peak in Alaska since 1660s: Implication for climate change variability in the North Pacific Rim, Atmos. Environ., 130, 105–112, https://doi.org/10.1016/j.atmosenv.2015.09.063, 2016.
Salvador, C. M., Ho, T.-T., Chou, C. C.-K., Chen, M.-J., Huang, W.-R., and Huang, S.-H.: Characterization of the organic matter in submicron urban aerosols using a Thermo-Desorption Proton-Transfer-Reaction Time-of-Flight Mass Spectrometer (TD-PTR-TOF-MS), Atmos. Environ., 140, 565–575, https://doi.org/10.1016/j.atmosenv.2016.06.029, 2016.
Singh, P., Kumar, N., and Arora, M.: Degree–day factors for snow and ice for Dokriani Glacier, Garhwal Himalayas, J. Hydrol., 235, 1–11, https://doi.org/10.1016/S0022-1694(00)00249-3, 2000.
Strader, R., Lurmann, F., and Pandis, S. N.: Evaluation of secondary organic aerosol formation in winter, Atmos. Environ., 33, 4849–4863, https://doi.org/10.1016/S1352-2310(99)00310-6, 1999.
Timkovsky, J., Dusek, U., Henzing, J. S., Kuipers, T. L., Röckmann, T., and Holzinger, R.: Offline thermal-desorption proton-transfer-reaction mass spectrometry to study composition of organic aerosol, J. Aerosol Sci., 79, 1–14, https://doi.org/10.1016/j.jaerosci.2014.08.010, 2015.
Zhao, Y., Hennigan, C. J., May, A. A., Tkacik, D. S., de Gouw, J. A., Gilman, J. B., Kuster, W. C., Borbon, A., and Robinson, A. L.: Intermediate-Volatility Organic Compounds: A Large Source of Secondary Organic Aerosol, Environ. Sci. Technol., 48, 13743–13750, https://doi.org/10.1021/es5035188, 2014.