the Creative Commons Attribution 4.0 License.
the Creative Commons Attribution 4.0 License.
Climate warming over the past half century has led to thermal degradation of permafrost on the Qinghai–Tibet Plateau
Youhua Ran
Guodong Cheng
Air temperature increases thermally degrade permafrost, which has widespread impacts on engineering design, resource development, and environmental protection in cold regions. This study evaluates the potential thermal degradation of permafrost over the Qinghai–Tibet Plateau (QTP) from the 1960s to the 2000s using estimated decadal mean annual air temperatures (MAATs) by integrating remote-sensing-based estimates of mean annual land surface temperatures (MASTs), leaf area index (LAI) and fractional snow cover values, and decadal mean MAAT date from 152 weather stations with a geographically weighted regression (GWR). The results reflect a continuous rise of approximately 0.04 ∘C a−1 in the decadal mean MAAT values over the past half century. A thermal-condition classification matrix is used to convert modelled MAATs to permafrost thermal type. Results show that the climate warming has led to a thermal degradation of permafrost in the past half century. The total area of thermally degraded permafrost is approximately 153.76×104 km2, which corresponds to 88 % of the permafrost area in the 1960s. The thermal condition of 75.2 % of the very cold permafrost, 89.6 % of the cold permafrost, 90.3 % of the cool permafrost, 92.3 % of the warm permafrost, and 32.8 % of the very warm permafrost has been degraded to lower levels of thermal condition. Approximately 49.4 % of the very warm permafrost and 96 % of the likely thawing permafrost has degraded to seasonally frozen ground. The mean elevations of the very cold, cold, cool, warm, very warm, and likely thawing permafrost areas increased by 88, 97, 155, 185, 161, and 250 m, respectively. The degradation mainly occurred from the 1960s to the 1970s and from the 1990s to the 2000s. This degradation may lead to increased risks to infrastructure, reductions in ecosystem resilience, increased flood risks, and positive climate feedback effects. It therefore affects the well-being of millions of people and sustainable development at the Third Pole.
- Article
(15793 KB) - Full-text XML
- BibTeX
- EndNote
Permafrost is defined as earth materials, including ice or organic material, that remain at or below 0 ∘C for at least 2 years (Permafrost Subcommittee, National Research Council of Canada, 1988; Williams and Smith, 1989). An increase in air temperatures often thermally degrades permafrost, which has widespread impacts on engineering design, construction, resource development, carbon and water cycles, and ecological protection in cold regions (Collett, 2002; Cheng and Wu, 2007; Tarnocai et al., 2009; Schuur et al., 2009; Schaefer et al., 2011; Hinzman et al., 2013; Mu et al., 2015; Zhu et al., 2016). In terms of middle- and high-elevation permafrost regions, the area of permafrost in the Qinghai–Tibet Plateau (QTP) is the largest in the world. The permafrost in the QTP experiences higher temperatures than those observed in Siberia and the Arctic, which are more sensitive to global climate warming and human activity (Wu et al., 2002; Haeberli and Hohmann, 2008; Li et al., 2008; Ran et al., 2012; Ran and Li, 2016).
Monitoring and simulation show that substantial permafrost degradation is occurring on the QTP. For example, the mean annual air temperature (MAAT) increased by approximately 0.2–0.4 ∘C from the 1970s to the late 1990s (Wang et al., 2000). From 1962 to 2010, the decadal average MAAT rose by 1.3 ∘C, with an average rate of increase of 0.03 ∘C a−1 (Jin et al., 2011; Ran and Li, 2016). From 1996 to 2001, the thickness of the active layer increased by 0.15–0.50 m, and the mean annual ground temperature (MAGT) rose by 0.1–0.5 ∘C in the past 30 years (Yang et al., 2010). At Xidatan, near the city of Golmud (Fig. 1) at the northern boundary of permafrost adjacent to the Qinghai–Tibet Railway (QTR), the lower elevation limit of permafrost moved upward ∼25 m from 1975 to 2002 (Nan et al., 2003). On the northern and southern slopes of the Bayan Har Mountains (Fig. 1), the lower elevation limits of discontinuous permafrost have moved upward ∼90 and ∼100 m, respectively, from 1991 to 2010 (Luo et al., 2013). On the southern side of the Tanggula Mountains (Fig. 1), climate change and infrastructure development have resulted in permafrost degradation; from 2006 to 2012, permafrost temperatures at 10 m depth have increased by 0.03∘C in undisturbed areas, and 0.06∘C beneath an embankment, and respective active layers have deepened by 0.29 and 0.41 m (Sun et al., 2014). Cheng et al. (2012) reported on the decadal changes in permafrost distribution on the QTP over the past 50 years (1960–2009) and demonstrated that the rate of permafrost loss had accelerated since the 1980s, and about one-fifth of the total area of permafrost that existed in the 1960s has degraded.
However, many of these studies focus on either the local or in situ scales, and few studies have focused on the regional scale. Although the decadal changes in the permafrost distribution over the QTP were simulated by Cheng et al. (2012), this study emphasized the migration of permafrost “boundaries”. However, such boundaries are continuous, inexact representations of the permafrost distribution and permafrost degradation (Yang et al., 2010). As an alternative, Ran and Li (2016) used spatially distributed near-surface air temperature reanalysis data to assess spatial variation in the thermal degradation of permafrost in China over a 30-year period, but the dataset has a low resolution and large uncertainties. These studies are not comprehensive and do not adequately reflect changes in the thermal state of the permafrost, especially in the interior of permafrost zones. Therefore, the thermal state of permafrost should be evaluated with a higher resolution over a longer period than previous studies. However, the evaluation is general limited by the sparse data. Of the most commonly used indices (i.e., MAAT and MAGT), MAGT is the most direct indicator of the thermal state of permafrost (Cheng, 1984). However, long-term measurements of MAGT are almost non-existent due to the high cost of drilling boreholes. The potential problems of using MAAT to predict permafrost degradation are well known, such as the neglecting the influences of thermal inertia of deep soil layers or geothermal heat flux (Smith and Riseborough, 2002; Jin et al., 2006; J. Wu et al., 2010). However, MAAT is easy to measure and has high spatial representativeness. Importantly, long-term in situ measurements of MAATs are available. However, MAAT measurement stations are sparse on the QTP, especially in the western region. In previous studies MAAT measurements were interpolated onto grids based on digital elevation models (DEMs), but the uncertainty of the gridded MAAT is substantial because of the locations of weather stations and heterogeneity of the surface characteristics, including snow cover and vegetation (Vancutsem et al., 2010).
Fortunately, the remote sensing era has led to changes in this situation. Thermal infrared remote sensing provides direct observations of land surface temperatures (LSTs) at high spatial and temporal resolutions, from which mean annual land surface temperature (MAST) can be determined. For example, the Moderate Resolution Imaging Spectroradiometer (MODIS) LST product is freely available and has been validated over large areas via a series of field campaigns. Its accuracy is better than 1∘C (0.5∘C in most cases) (Wan et al., 2002, 2004, 2008). Remote-sensing-based estimates of LSTs provide a key high-resolution temperature pattern of the land surface that can potentially be used in monitoring permafrost degradation. However, criteria for using LST estimates to distinguish permafrost types are not traditionally available, and the relatively short-term series of LST data does not meet the needs of long-term permafrost monitoring.
Several studies have demonstrated a high correlation between satellite-based LST observations and near-surface air temperature, and thus the potential of satellite-based methods in estimating near-surface air temperatures (Hachem et al., 2009, 2012; Vancutsem et al., 2010; Yao and Zhang, 2013; Ran et al., 2015). Variation and uncertainty in estimated near-surface air temperatures are mainly related to surface conditions such as snow cover and vegetation, the amount of solar radiation, and cloud cover (Henderson-Sellers and Hughes, 1982; Zhang, 2005; Vancutsem et al., 2010; Lawrence et al., 2011; Hachem et al., 2012; Ran et al., 2015). Highly accurate remote-sensing-based snow cover and vegetation products are now available to reduce some of the uncertainty, and solar radiation and cloud cover can be accounted for. Therefore we propose that high-resolution remote-sensing-based LST and the long-term in situ MAAT measurement can be integrated to monitor the thermal state of permafrost. The objective of this study is to evaluate potential thermal degradation of permafrost, including temporal changes, spatial changes in the map plane, and spatial changes with elevation over the QTP from 1960 to 2010, by integrating multi-criterion remote sensing observations and an air temperature observation network.
In this study, the potential thermal degradation of permafrost is evaluated based on a MAAT model and the improved MAAT data over the QTP in the past half century. The MAAT in situ measurement data at 152 sites over the QTP and remote sensing data with six independent variables were combined using a geographically weighted regression (GWR) model to estimate the MAAT with a 1 km resolution over the QTP during the past five decades.
2.1 Permafrost thermal condition classification system
In this study, a permafrost thermal condition classification system is used. That is defined according to the high-altitude permafrost zonation proposed by Cheng (1984). In Cheng's system, permafrost is classified into extremely stable, stable, sub-stable, transitional, unstable, and extremely unstable types. The system is a high-level summary of the relation between MAGT measurements and the three-dimensional zonation of high-altitude permafrost (elevation, latitude, and aridity). Cheng's (1984) system relates air temperature to permafrost conditions according to the statistical relations between MAGT, elevation, and in situ MAAT measurements. The extremely unstable type in this system refers to regions that include cave ice and frozen gravel below the lower limit of permafrost, which is a very scattered distribution. However, the thermal stability of permafrost is a function of surficial materials and ground-ice content as much as temperature. From a strictly thermal perspective, the notion that cold permafrost is more “thermally stable” than warm permafrost typically does not hold true. From a changing stability perspective, for a given increase in MAAT, MAGT in cold permafrost often responds more quickly than warm permafrost because of relatively low latent heat effects in the former versus the latter. In the extreme case, thawing permafrost that is nearly 0∘C may appear thermally stable, because heat is used for phase change rather than temperature change. This process intertwined with the changing geothermal flux makes it difficult to reflect the thermal stability change using MAAT index as a thermal condition. Therefore, we rename the permafrost stability types into thermal condition types include very cold, cold, cool, warm, very warm, and likely thawing types in this paper, as shown in Table 1. This system is more useful to describe permafrost degradation from a spatially distributed perspective, rather than a boundary perspective. On the QTP, a MAAT of −2∘C has typically been used to distinguish permafrost from seasonally frozen ground (Cheng, 1984; Ran and Li, 2016). Here we use a MAAT of −1 ∘C to distinguish likely thawing permafrost from seasonally frozen ground based on experienced judgement for the region of cave ice and frozen gravel distribution area.
2.2 Simulation of MAAT using geographically weighted regression
In this study, GWR is used to simulate MAATs. Local parameters are employed in the GWR model to estimate MAATs while considering the spatial locations of meteorological stations (Brunsdon et al., 1998; Kumar et al., 2012). The weighting is a function of the distance between the location of each regression point and the sites where observations are available. The GWR model used in the present study is shown below in Eq. (1):
where yi is the MAAT at pixel i, xik is the kth explanatory factor at pixel i, β0(μi,υi) and βk(μi,υi) represent the intercept and slope for the kth explanatory factor, m is the number of explanatory factors, and εi is the residual term.
The quantities β0(μi,υi) and βk(μi,υi) are estimated using Eq. (2):
where is an unbiased estimation of the regression coefficients. It is a vector that includes an intercept and m regression coefficients associated with m explanatory factors, i.e., the six independent variables selected by a stepwise linear regression analysis (see below). X is a matrix of explanatory factors (n×m); W(μi,υi) is the spatial weight matrix, which is a diagonal matrix; Y is a vector (n×1) for the dependent variables, i.e., the decadal mean MAAT in the 1960s, 1970s, 1980s, 1990s, and 2000s; and n is the number of MAAT observation stations for each year.
In this study, the Gaussian function is used as a spatial weighting function, as shown in Eq. (3):
where di is the distance between the ith observation station and the point to be estimated, and r is the bandwidth parameter. To accommodate different station densities, the corrected Akaike information criterion (AIC) is used to determine the optimal bandwidth parameters.
A stepwise linear regression analysis is used to select the independent variables for the GWR model. The variance inflation factor (VIF) is used to assess the multicollinearity of the model. The GWR is implemented in System for Automated Geoscientific Analyses (SAGA) (Conrad et al., 2015), using the GWR for multiple predictor grids, as a geoprocessing tool with a Gaussian weighting function, and a global search range.
Due to the unavailability of vegetation, snow cover, and LST datasets during the 1960s to 2000s, the effects of the dynamics of vegetation, snow cover, and LST on estimating MAAT during this period are unknown, and will inevitably cause some errors in the estimation of MAAT. Recent studies of the QTP show overall that vegetation increased during the past 30 years and snow cover decreased during the past 15 years (Wang et al., 2016; Huang et al., 2017). The effect of vegetation and snow cover change on MAAT and related feedback processes are highly complex. For example, the vegetation–snow interaction effect on MAAT is related to humidity (Zhong et al., 2010; T. Wang et al., 2013; Wu et al., 2015; Yuan et al., 2017). However, we believe that such effects mainly occur at the local level in vegetation dominated areas (Wang et al., 2016; Huang et al., 2017), and they can be partially compensated by in situ time-series MAAT measurements over the QTP for the past 50 years.
2.3 Evaluation of the rate of permafrost thermal degradation
A linear regression model is used to evaluate the rates of MAAT change in the QTP over the past 50 years, and the statistical significance of the regression model is evaluated using Student's t test. Thirteen elevation ranges (< 3600, 3600–3800, 3800–4000, 4000–4200, 4200–4400, 4400–4600, 4600–4800, 4800–5000, 5000–5200, 5200–5400, 5400–5600, 5600–5800 m, and > 5800 m) are used to evaluate the elevation dependence of the warming rate.
The degradation of permafrost thermal condition is assessed from two perspectives, the change in area of the different permafrost types and the spatial heterogeneity of the change. For the area change, we determined the total area of each permafrost type during the past five decades and calculated the rate of change (i.e., the degradation rate) using the linear regression model. The spatial pattern of the degradation of permafrost thermal condition is assessed at two levels. At the pixel level the spatial distribution of the degradation is evaluated, and at the level of the permafrost types a transfer matrix is used to evaluate the conversions among the types (Stehman, 1997). We also analyse the changes in the elevation histograms for each permafrost typed over the past 50 years.
2.4 Datasets
2.4.1 Mean annual land surface temperature
MODIS Terra/Aqua daytime and nighttime LST products (MOD11A1 and MYD11A1, version 5) with a spatial resolution of 1 km and covering 2006 to 2010 were acquired from the Distributed Active Archive Center (DAAC) operated by the U.S. National Aeronautics and Space Administration (NASA). These data are used in this study to estimate MASTs. A pragmatic approach proposed by Ran et al. (2015) is employed to estimate the MASTs using the four daily MODIS LST products. This approach assumes that the arithmetic average of the daytime and nighttime LSTs represent the daily mean LST with acceptable accuracy, and that the daily amplitude of LST is more homogeneous than the LST itself (Liu et al., 2006; Kogan et al., 2011; Ran et al., 2015). This approach allows the full use of every value at any time in any pixel of the MODIS LST products through the use of the temporally and spatially complete LST daily amplitude, which is interpolated using a gap-filling algorithm (Garcia, 2010). This algorithm employs a penalized least squares regression based on discrete cosine transforms that explicitly utilize information from a time series to predict the missing values. The penalized least squares regression is a thin-plate spline smoother for a generally one-dimensional data array, and it can trade off fidelity to the data versus the roughness of the mean function (Garcia, 2010; Wang et al., 2012). This approach is easy to implement and independent of other observations. Validation shows that the scheme is effective in restoring the missing values in MODIS instantaneous LST observations and produces a spatially and temporally continuous daily average LST dataset that displays good agreement with observations made at the ground surface. The errors in the results originate mainly from the original instantaneous LST MODIS products. A more detailed description of this scheme can be found in Ran et al. (2015). The temporally and spatially continuous daily mean LSTs from 1 January 2006 to 31 December 2010 and the corresponding MASTs used in this study are produced using the above approach.
2.4.2 Fractional snow cover
Arithmetic mean values of daily cloudless FSC products from 2006 to 2010 are used in this study. This product is derived from the daily MODIS 500 m snow cover product (MOD10A1) using a gap filling process based on a cubic spline interpolation algorithm. A comparison with reference fractional snow cover (FSC) data obtained from Landsat Enhanced Thematic Mapper Plus (ETM+) shows the high accuracy with which this product reflects snow cover information over the QTP (Tang et al., 2013). The cloudless FSC products were acquired from the Cold and Arid Regions Science Data Center, Lanzhou, China (http://westdc.westgis.ac.cn).
2.4.3 Leaf area index
Annual mean leaf area index (LAI) values obtained from the Global Land Surface Satellite (GLASS), which make up a high-quality LAI product with an 8-day temporal resolution and a 1 km spatial resolution and cover the period from 2006 to 2010, are used in this study. The GLASS LAI product is derived from the fused MODIS and CYCLOPES LAI products, and the remaining effects of cloud contamination have been removed using MODIS time series surface reflectance data and general regression neural networks (Xiao et al., 2014). The results of validation show that the GLASS LAI product has a lower uncertainty than the MODIS and CYCLOPES LAI products (Xiang et al., 2014). The GLASS LAI product was acquired from the GLASS project website (http://glass-product.bnu.edu.cn).
2.4.4 In situ MAAT observations
The MAAT measurements, which were collected at 131 stations for the 1960s and 1970s, 133 stations for the 1980s, 144 stations for the 1990s, and 152 stations for the 2000s within the QTP and the surrounding area, were acquired from the data centre of the China Meteorological Administration (http://cdc.nmic.cn). The distribution of the 152 stations for the 2000s is shown in Fig. 1. The density of stations in the eastern QTP is higher than in earlier years. The decadal mean MAAT values over the past five decades are used in this study.
2.4.5 Validation data
Validation of the long-term permafrost thermal condition is difficult due to the limited amounts of reference data that are available. In this study, we evaluate the results by comparing the estimated permafrost distribution in the 2000s with previous regional-scale permafrost maps and borehole measurements at individual sites. The permafrost maps that cover the QTP from Li and Cheng (1996), Nan et al. (2002), and Zou et al. (2017) are used at the regional scale. In particular, the map of Zou et al. (2017) integrates the MODIS 8-day LST product within the framework of the temperature at the top of the permafrost (TTOP) model (Smith and Riseborough, 1996), and careful validation of this map has been performed using MAGT data. At the site scale, the MAGT values used in this study were collected from 142 boreholes presented in the existing literature (Yu et al., 2008; Q. F. Wang et al., 2013; Luo et al., 2013) and the International Permafrost Association's International Polar Year Thermal State of Permafrost Snapshot Borehole Inventory, downloaded from the National Snow and Ice Data Center (NSIDC) (http://nsidc.org) (International Permafrost Association, 2010). The distribution of these boreholes is shown in Fig. 1.
2.4.6 Ancillary data
The distribution of water bodies in the MODIS land cover product (MOD12Q1) and the map showing the distribution of glacier ice from the second Chinese glacier inventory are used to support the permafrost area statistics. The MOD12Q1 product is used for consistency with the other remote sensing products employed in this study. Conversely, the glacier extents from the second Chinese glacier inventory are compiled based on Landsat Thematic Mapper (TM) or ETM+ images acquired from 2004 to 2011, as well as other ancillary data, such as DEMs. The robust band ratio segmentation method is first used to delineate the glacier outlines, and intensive manual improvements are then performed to improve its accuracy. An error assessment shows that the area error for all of the glaciers in China is approximately 3.2 % (Guo et al., 2015).
Stepwise linear regression analysis shows that the use of the MAST, the leaf area index (LAI), FSC, elevation, latitude, and longitude as independent variables results in the highest degree of explanatory power for the past five decades, and the significance level is less than 0.0001 (Model 6 in Table 2). The VIF value less than 1.5 shows that the degree of tolerance is high, and the multicollinearity of the model is thus acceptable. The performance of the GWR model in the 2010s is shown in Table 2. Then decadal mean MAAT estimates with a 1 km resolution over the QTP in the past 50 years are produced using the model 6. The permafrost stability map in the past five decades is then produced based on the simulated MAAT and the permafrost types defined in Table 1.
3.1 Change of MAAT over the QTP in the past 50 years
The MAATs over the QTP have risen continuously in the past 50 years. The mean MAAT values for the 1960s, 1970s, 1980s, 1990s, and 2000s are −2.38, −1.85, −1.78, −1.32, and −0.58∘C, respectively. These values reflect a continuous rise at a rate of approximately 0.04 ∘C a−1. This value is higher than the global average warming rate, as well as the estimated warming rates for the QTP reported by Cheng et al. (2012) and Ran and Li (2016) that are based on interpolated elevation-based air temperature data or surface air temperature reanalysis data. The warming rate in the western part of the QTP is higher than that in the eastern part and depends on elevation, as shown in Figs. 2 and 3. The warming rate increases with increasing elevation from approximately 0.33∘C per decade at 3600 m to 0.49∘C per decade at 5200 m. This finding is similar to that of previous studies (Liu and Chen, 2000; Qin et al., 2009). The physical mechanisms of this phenomenon may be related to the combined effects of the cloud-radiation and snow-albedo feedback (Giorgi et al., 1997; Liu et al., 2009; Pepin et al., 2015). These elevated warming rates may have a substantial impact on the thermal condition of the permafrost.
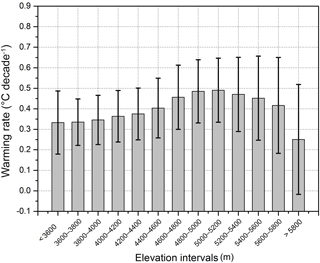
Figure 3Warming rates with increasing elevation. These rates are derived from MAATs estimated by integrating remote-sensing-based mean annual land surface temperatures (MASTs), leaf area index (LAI) and fractional snow cover values, and decadal mean MAAT date from 152 weather stations with a geographically weighted regression. Error bars display the standard deviations.
3.2 Potential thermal degradation
Based on the map of permafrost types covering the past five decades (Fig. 4a–e), we analyse the degradation from three perspectives, including temporal changes, spatial changes in the map plane, and spatial changes with elevation.
3.2.1 Temporal dynamics of thermal degradation
Permafrost has thermally degraded continuously over the past 50 years. The area occupied by the colder types has decreased continuously, and the area occupied by the very warm types has increased continuously (Table 3). The areas occupied by the very cold, cold, cool, and warm types display net decreases of approximately 8.99×104 km2 (72.79 %), 27.06×104 km2 (70.12 %), 9.30×104 km2 (27.24 %), and 1.18×104 km2 (4.77 %) from the 1960s to the 2000s, respectively. In particular, the cold type displays the most serious degradation, and its rate of loss is approximately 6.15×104 km2 (15.94 %) per decade. Moreover, the area occupied by the very warm type has increased by approximately 3.99×104 km2 (9.02 %) at a rate of 1.06×104 km2 (2.4 %) per decade. Specifically, this degradation mainly occurred during the 1960s to 1970s and the 1980s to 1990s for the very cold type, the 1960s to the 1970s and the 1990s to the 2000s for the cold type, and the 1980s to the 2000s for the cool type. The area occupied by the likely thawing type has not changed substantially. If glaciers and the likely thawing type are included, the total permafrost area has decreased significantly from 174.76×104 km2 in the 1960s to 133.1×104 km2 in the 2000s at a rate of approximately 9.52×104 km2 (5.45 %) per decade, and this loss of area occurred mainly during the 1960s to the 1970s and the 1990s to the 2000s (Table 3).
3.2.2 Spatial variation of thermal degradation
Degradation of permafrost thermal condition has likely occurred over a broad region of permafrost on the QTP within the past 50 years, especially during the 1960s to the 1970s and the 1990s to the 2000s. The degradation of permafrost condition in the western QTP was substantial during the 1960s to the 1970s. In the subsequent 40 years, the degradation of permafrost condition in the QTP was relatively homogeneous (Fig. 4f–i). Specifically, the extent of the very cold, cold, and cool types retreated from the south to the north (Fig. 4a–e). The extents of the warm, very warm, and likely thawing types extended northward correspondingly. Approximately 42.30 % of the very cold type, 42.09 % of the cold type, and 39.83 % of the cool type have degraded to the cold, cool, and warm types from the 1960s to the 1970s, respectively. At the same time, approximately 57.26 % of the warm type, 29.34 % of the very warm type, and 59.47 % of the likely thawing type have degraded to the very warm type, likely thawing type, and seasonally frozen ground, respectively. Overall, approximately 75.24 % of the very cold type, 89.56 % of the cold type, 90.3 % of the cool type, 92.31 % of the warm type, and 32.8 % of the very warm type have degraded to lower levels of thermal condition in the past 50 years (Table 4). The potential reduction in the area of permafrost is mainly due to the degradation of the very warm and likely thawing types. Approximately 49.4 % of the very warm type and 95.95 % of the likely thawing type has degraded to seasonally frozen ground (Table 4). The total degraded area is approximately 153.76×104 km2, which accounts for 87.98 % of the area occupied by the permafrost region in the 1960s (Fig. 4j). Permafrost thermal condition did not change over a 21×104 km2 area (12.02 %) located primarily in the central part of the plateau, which contains extremely high mountains, and it is dominated by the very cold type.
Notably, the thermal condition of a specific permafrost area of approximately 1.63×104 km2 has likely improved. This area is located primarily east of Lhasa in the southeastern part of QTP where there are numerous marine glaciers and substantial snow cover (Fig. 4j). The improved permafrost thermal condition in this area may relate to large uncertainties as the MAAT trend is estimated using regression parameters that are appropriate for low-elevation areas. This relates to the lack of long-term MAAT measurements in the high mountain regions where glaciers and snow are prevalent. The effects of snow or glacier cover on permafrost may be more important than those of MAAT due to the former having greater latent heat than atmosphere. Recent study also shows that there is no evidence of widespread decline of snow cover in QTP (Wang et al., 2017). Although records of long-term snow cover and glacier changes in the past 50 years are not available in this study, the sensitivity of glacier and snow cover in a warming climate is dependent on the climate zone. Low snow–climate sensitivities have been found in continental interior climates with relatively cold and dry winters (Brown and Mote, 2009). Larger glaciers have lower climate sensitivities (Ding and Haeberli, 1996; Ye et al., 2001).
Additionally, the complex process and limited knowledge of permafrost–glacier interactions may enhance the uncertainty (Haeberli, 2005; Otto and Keuschnig, 2014). Therefore, we believe the permafrost thermal condition in this area has not changed substantially in the past 50 years, based on this low climate sensitivity, but this notion requires further investigation.
3.2.3 Relation of variation in thermal degradation to elevation
The elevation statistics of the permafrost type distribution over the QTP in the past five decades indicate that the mean elevation occupied by each permafrost type in the QTP has likely increased continuously (Table 5). For the very cold type, the mean elevation of the distribution decreased from 5240 to 5161 m from the 1960s to the 1970s and then rose continuously at a rate of approximately 56.4 m per decade. The reduction in elevation during 1960s to 1970s for very cold permafrost is mainly due to the degradation of the very cold permafrost type in the Kailas Mountains. The degradation leads to a decrease in the proportion of the very cold permafrost type in high elevation areas and a decrease in the mean elevation for the very cold permafrost type in 1970s relative to the 1960s. This fluctuation of the mean elevation for very cold permafrost during the 1960s to 1980s reduced the statistical significance (low R2 in Table 5) of the increasing rate of mean elevation over the past 50 years. Overall, in the past 50 years, the mean rate of increase of the very cold type has been approximately 24.7 m per decade. Moreover, the mean elevation of the cold, cool, warm, very warm, and likely thawing types have risen at a rate of 23.6, 36.3, 43, 36.5, and 56.2 m, respectively. Overall, the mean elevation of the very cold, cold, cool, warm, very warm, and likely thawing types increased by 88, 97, 155, 185, 161, and 250 m, respectively, over the past 50 years. As in the last section, the degradation mainly occurred from the 1960s to the 1970s and from the 1990s to the 2000s.
4.1 Cross validation and uncertainty analysis
We validate the permafrost extent only in the 2000s because long-term records of permafrost thermal condition and extent are not available for earlier periods, as mentioned in Sect. 2.4.5. Comparison of the estimated permafrost extent in the 2000s with the permafrost map provided by Zou et al. (2017) shows that the difference is small. Within permafrost areas, the likely thawing permafrost type mainly refers to cave ice and frozen gravel, which are distributed below the lower limit of permafrost (Cheng, 1984). This kind of permafrost is usually not counted in the total area of permafrost. Therefore, the permafrost area in the 2000s is approximately 107.19×104 km2, if glaciers and lakes are neglected. This result is similar to that of Zou et al. (2017), who showed that the permafrost area in the 2000s was approximately 106.47×104 km2. The permafrost distribution is also very similar (consistency is 92 %; kappa coefficient is 0.82) to Zou et al. (2017) (Fig. 5b). At the site scale, 89 % of the 142 locations are consistent with the borehole survey, whereas this proportion is only 74, 28, and 86 % for the maps of Li and Cheng (1996), Nan et al. (2002), and Zou et al. (2017), respectively. These proportions indicate that the accuracy of the permafrost extent identified in this study is at least comparable with that of Zou et al. (2017).
The uncertainty of the results may stem from the MAAT model, insufficient resolution, inaccuracies in the surface station data, or the sparseness of these stations, the latter of which being a particular problem in high mountain areas. Beyond these uncertainties, the response time and the depth to which permafrost is affected by climate warming depend on the extent, duration, amplitude, and rate of climate warming and are closely related to soil type, surface coverage, ice content, groundwater occurrence, geothermal anomalies, and human activity (Stieglitz et al., 2003; Zhang, 2005; Lawrence et al., 2008; Cheng and Jin, 2013; Westermann et al., 2016). For example, the low heat conductivity of soil leads to lag between increases in surface temperatures and the subsequent increase in permafrost temperature or reduction in permafrost thickness (Li et al., 1996). The delay time is longer for permafrost thickness than temperature and varies with the thermal condition (Li et al., 1996; J. Wu et al., 2010). For the cold permafrost, the thermal degradation may be delayed by thermal offset and seasonal offset effects in the permafrost table due to the negative heat budget; i.e., the amount of heat released from the active layer during winter is greater than the amount of heat absorbed in summer (Smith and Riseborough, 2002; J. Wu et al., 2010). For the warm permafrost, a positive heat budget appears in the upper soil layer that leads to a greater spatial degradation rate than that seen in cold permafrost, since the thickness of the warm type is less than that of the cold type (Li et al., 1996; J. Wu et al., 2010). However, the complex physical mechanisms of the interactions between climate change and permafrost are currently poorly understood (Jin et al., 2011), and a large degree of uncertainty may exist in previous evaluations as well as the permafrost area change over the past 50 years in this study. To summarize, despite current warming, large permafrost areas may persist due to the effective thermal inertia of permafrost (Cheng et al., 2012). A final consideration is that the geothermal heat flux leads to thawing of the base of the permafrost (Jin et al., 2006; J. Wu et al., 2010). However, the MAAT model cannot reflect the change of geothermal flux from the crustal interior. Additionally, the geothermal flux data are generally limited or unavailable. The missing geothermal heat flux may lead to a delay in permafrost degradation, especially for the cold permafrost, because the geothermal flux is independent of air temperature. Thirdly, although the resolution of the simulation has been significantly improved to 1 km, it is still coarse relative to the degradation rate of mountain permafrost. The degradation of mountain permafrost is presented in terms of the increase in the elevation of the lower limit of the permafrost, which is generally ∼100 m. A 1 km change in the horizontal extent change may correspond to a change in elevation of hundreds of metres. Finally, the lack of long-term MAAT measurements in the glacier- and snow-dominated high mountain regions may lead to errors in the estimated MAATs.
Overall, the accelerated degradation effect of the MAAT model may be partly counteracted by the delayed degradation effect of the missing geothermal heat flux component thermal inertia of permafrost. However, long-term observation shows that the mean increasing rate of ground temperature at 10–20 m depth in the QTP is approximately 0.024∘C (Zhao et al., 2010; Q. B. Wu et al., 2010; Jin et al., 2011), which is comparable with the warming rate of air temperature. This shows that the evaluation results of permafrost thermal degradation using the MAAT model are generally acceptable at the overall QTP scale.
4.2 The implications of the degradation of thermal condition
The degradation of the permafrost thermal condition in the QTP has important impacts on the safety of infrastructure in permafrost regions, water quality, ecosystem health, and the feedback for regional and global climates. Firstly, as the permafrost thermally degrades, the risk of deterioration and damage to engineered structures in permafrost zones will likely increase. This suggests that the measures used to prevent permafrost degradation may need to be enhanced for new structures. For example, permafrost accounted for 90.1 % of a 10 km long segment of the QTR from Golmud to Lhasa in the 1960s, and these permafrost areas were dominated by the cool type; however, after 50 years (i.e., in the 2000s), these permafrost areas accounted for only 67.77 % and were dominated by the very warm type. For the very warm permafrost, an enhanced measure to prevent permafrost degradation, i.e., the proactive roadbed cooling approach, has been successfully applied in the construction of the QTR (Cheng, 2004, 2005; Cheng et al., 2008). Secondly, the degradation of permafrost in the QTP may affect the hydrologic cycle in the Third Pole region, which includes the QTP and the surrounding arid regions. Permafrost controls the distribution, recharge, flow paths, discharge, dynamics, and hydrochemistry of groundwater (Cheng and Jin, 2013). The degradation of permafrost affects the interactions among the surface water, subsoil water, and groundwater by changing the hydraulic conductivity and hydraulic connectivity of the soil. The degradation of the ice-rich permafrost itself makes important contributions to surface runoff and the development of thermokarst lakes in the inner Tibetan Plateau (Zhang et al., 2013). The enhanced drainage may lead to increases in flood risk (Larsen et al., 2008) and reductions in ecosystem resilience via seasonal shifts in stream flow and groundwater abundance, because the decrease in permafrost water storage capacity in the QTP will lead to a reduction in dry-season water availability. All of these changes will affect the well-being of millions of people and sustainable development at the Third Pole, which contains the headwater areas of several of the major rivers in Southeast Asia, such as the Yellow, Yangtze, Mekong, Yarlung Zangbo and Shiquan rivers. The Third Pole also includes many inland rivers, such as the Shiyang, Heihe, Shule, and Tarim Rivers in northwestern China. Finally, the permafrost region in the QTP contains approximately 160 Pg of organic carbon (Mu et al., 2015), many thermokarst lakes, and wetlands (Jorgenson et al., 2010; Niu et al., 2011; Luo et al., 2015). Thawing of the permafrost may lead to the drainage or growth of thermokarst lakes (Smith et al., 2005), which may affect greenhouse gas emissions and influence on climate change (Tarnocai et al., 2009; Schuur et al., 2009; Schaefer et al., 2011; McCalley et al., 2014). Additionally, changes in thermokarst lakes may both accelerate and delay permafrost thawing (Westermann et al., 2016; You et al., 2017).
This study evaluates likely permafrost thermal degradation over the QTP from the 1960s to the 2000s based on the improved decadal means of the MAATs data over the QTP in the past 50 years obtained by integrating remote-sensing-based MASTs, LAI, and fractional snow cover values, as well as decadal mean MAATs measured at 152 weather stations using a GWR model. Cross validation shows that the accuracy of the estimated permafrost extent is greater than that of previous maps.
The decadal mean MAATs reflect a continuous rise at a rate of approximately 0.04 ∘C a−1 during the past half century. The warming rate increases with increasing elevation from approximately 0.33∘C per decade at 3600 m to 0.49∘C per decade at 5200 m and then decreases as elevation increases further. Climate warming has led to the thermal degradation of permafrost in the past half century. The area occupied by the cold permafrost types has continuously decreased, and the area occupied by the very warm types has continuously increased. The total degraded area is approximately 153.76×104 km2, which accounts for 87.98 % of the permafrost area in the 1960s. The thermal condition for all permafrost types has degraded to lower levels. The extent of the very cold, cold, and cool types retreated from the south to the north, whereas the extent of the warm, very warm, and likely thawing types extended northward. The mean elevations of the very cold, cold, cool, warm, very warm, and likely thawing types increased by 88, 97, 155, 185, 161, and 250 m, respectively. The degradation mainly occurred during the 1960s to the 1970s and the 1990s to the 2000s. The thermal degradation of permafrost in the QTP has important impacts on the safety of infrastructure, flood risks, ecosystem resilience, and climate feedback, as well as the well-being of millions of people and sustainable development at the Third Pole.
The uncertainties inherent in this analysis cannot be discounted. These uncertainties are due to asynchronous changes in near-surface air temperatures and deep soil layer temperatures, the missing geothermal flux, insufficient resolution, or the inaccuracies and sparseness of the surface station data employed. In order to reduce these uncertainties, a deep layer soil map, surficial geology, and ground ice map are required. All of this will benefit from the accumulation of field data in the future, especially from boreholes.
The data used in this paper are available upon request from the corresponding author.
The authors declare that they have no conflict of interest.
This study was jointly supported by the Strategic Priority Research Program
of the Chinese Academy of Sciences (Grant No. XDA19070204), National
Natural Science Foundation of China projects (Grant No. 41471359), and the Youth Innovation Promotion Association of the Chinese
Academy of Sciences (Grant No. 2016375). The authors thank the associate
editor and three anonymous reviewers for their insightful comments and
suggestions that helped improve this paper.
Edited by: Peter Morse
Reviewed by: three anonymous referees
Brown, R. D. and Mote, P. W.: The response of Northern Hemisphere snow cover to a changing climate, J. Climate, 22, 2124–2145, 2009.
Brunsdon, C., Fotheringham, S., and Charlton, M.: Geographically weighted regression, J. Roy. Stat. Soc. D, 47, 431–443, 1998.
Cheng, G. D.: Problems on zonation of high-altitude permafrost, ACTA Geographica Sinica, 39, 185–193, 1984 (in Chinese).
Cheng, G.: Influences of local factors on permafrost occurrence and their implications for Qinghai-Xizang Railway design, Sci. China D, 47, 704–709, 2004.
Cheng, G.: A roadbed cooling approach for the construction of Qinghai–Tibet Railway, Cold Reg. Sci. Technol., 42, 169–176, 2005.
Cheng, G. D. and Jin, H. J.: Permafrost and groundwater on the Qinghai-Tibet Plateau and in northeast China, Hydrogeol. J., 21, 5–23, 2013.
Cheng, G. D. and Wu, T. H.: Responses of permafrost to climate change and their environmental significance, Qinghai-Tibet Plateau, J. Geophys. Res.-Earth Surf., 112, F02S03, https://doi.org/10.1029/2006JF000631, 2007.
Cheng, G., Sun, Z., and Niu, F.: Application of the roadbed cooling approach in Qinghai-Tibet railway engineering, Cold Reg. Sci. Technol., 53, 241–258, 2008.
Cheng, W. M., Zhao, S. M., Zhou, C. H., and Chen, X.: Simulation of the Decadal Permafrost Distribution on the Qinghai-Tibet Plateau (China) over the Past 50 Years, Permafrost Periglac., 23, 292–300, 2012.
Collett, T. S.: Energy resource potential of natural gas hydrates, AAPG bulletin, 86, 1971-1992, 2002.
Conrad, O., Bechtel, B., Bock, M., Dietrich, H., Fischer, E., Gerlitz, L., Wehberg, J., Wichmann, V., and Böhner, J.: System for Automated Geoscientific Analyses (SAGA) v. 2.1.4, Geosci. Model Dev., 8, 1991–2007, https://doi.org/10.5194/gmd-8-1991-2015, 2015.
Ding, Y. and Haeberli, W.: Compilation of long-term glacier-fluctuation data in China and a comparison with corresponding records from Switzerland, J. Glaciol., 42, 389–400, 1996.
Garcia, D.: Robust smoothing of gridded data in one and higher dimensions with missing values, Comput. Stat. Data Anal., 54, 1167–1178, 2010.
Giorgi, F., Hurrell, J. W., Marinucci, M. R., and Beniston, M.: Elevation dependency of the surface climate change signal: a model study, J. Climate, 10, 288–296, 1997.
Guo, W., Liu, S., Xu, J., Wu, L., Shangguan, D., Yao, X., Wei, J., Bao, W., Yu, P., Liu, Q., and Jiang, Z.: The second Chinese glacier inventory: data, methods and results, J. Glaciol., 61, 357–372, 2015.
Hachem, S., Allard, M., and Duguay, C.: Using the MODIS land surface temperature product for mapping permafrost: an application to Northern Quebec and Labrador, Canada, Permafrost Periglac., 20, 407–416, 2009.
Hachem, S., Duguay, C. R., and Allard, M.: Comparison of MODIS-derived land surface temperatures with ground surface and air temperature measurements in continuous permafrost terrain, The Cryosphere, 6, 51–69, https://doi.org/10.5194/tc-6-51-2012, 2012.
Haeberli, W.: Investigating glacier – permafrost relationships in high-mountain areas: historical background, selected examples and research needs, in: Cryospheric Systems – Glaciers and Permafrost, edited by: Harris, C. and Murton, J. B., Geological Society Special Publication 242, London, 29–37, 2005.
Haeberli, W. and Hohmann, R.: Climate, glaciers and permafrost in the Swiss Alps 2050: scenarios, consequences and recommendations, in: Proceedings Ninth International Conference on Permafrost, 1, 607–612, 2008.
Henderson-Sellers, A. and Hughes, N. A.: Albedo and its importance in climate theory, Prog. Phys. Geogr., 6, 1–44, 1982.
Hinzman, L. D., Deal, C. J., McGuire, A. D., Mernild, S. H., Polyakov, I. V., and Walsh, J. E.: Trajectory of the Arctic as an integrated system, Ecol. Appl., 23, 1837–1868, 2013.
Huang, X., Deng, J., Wang, W., Feng, Q., and Liang, T.: Impact of climate and elevation on snow cover using integrated remote sensing snow products in Tibetan Plateau, Remote Sens. Environ., 190, 274–288, 2017.
International Permafrost Association (IPA): IPA-IPY Thermal State of Permafrost (TSP) Snapshot Borehole Inventory, Version 1. Boulder, Colorado USA. NSIDC: National Snow and Ice Data Center, https://doi.org/10.7265/N57D2S25, 2010.
Jin, H., Zhao, L., Wang, S., and Jin, R.: Thermal regimes and degradation modes of permafrost along the Qinghai-Tibet Highway, Sci. China D, 49, 1170–1183, 2006.
Jin, H., Luo, D., Wang, S., Lü, L., and Wu, J.: Spatiotemporal variability of permafrost degradation on the Qinghai-Tibet Plateau, Sci. Cold Arid Reg., 3, 281–305, 2011.
Jorgenson, M. T., Romanovsky, V., Harden, J., Shur, Y., O'Donnell, J., Schuur, E. A., Kanevskiy, M., and Marchenko, S.: Resilience and vulnerability of permafrost to climate change, Can. J. Forest Res., 40, 1219–1236, 2010.
Kogan, F., Powell, A., and Fedorov, O.: Use of Satellite and In-Situ Data to Improve Sustainability, Springer, 2011.
Kumar, S., Lal, R., and Liu, D.: A geographically weighted regression kriging approach for mapping soil organic carbon stock, Geoderma, 189, 627–634, 2012.
Larsen, P. H., Goldsmith, S., Smith, O., Wilson, M. L., Strzepek, K., Chinowsky, P., and Saylor, B.: Estimating future costs for Alaska public infrastructure at risk from climate change, Global Environ. Change, 18, 442–457, 2008.
Lawrence, D. M., Slater, A. G., Romanovsky, V. E., and Nicolsky, D. J.: Sensitivity of a model projection of near-surface permafrost degradation to soil column depth and representation of soil organic matter, J. Geophys. Res.-Earth Surf., 113, F02011, https://doi.org/10.1029/2007JF000883, 2008.
Lawrence, D. M., Oleson, K. W., Flanner, M. G., Thornton, P. E., Swenson, S. C., Lawrence, P. J., Zeng, X. B., Yang, Z. L., Levis, S., Sakaguchi, K., Bonan, G. B., and Slater, A. G.: Parameterization improvements and functional and structural advances in version 4 of the Community Land Model, J. Adv. Model. Earth Syst., 3, M03001, https://doi.org/10.1029/2011MS000045, 2011.
Li, S. D. and Cheng, G. D.: Map of permafrost on the Qinghai-Tibet Plateau (1:3 000 000), Gansu Culture Press, Lanzhou, 1996 (in Chinese).
Li, S., Cheng, G., and Guo, D.: The future thermal regime of numerical simulating permafrost on Qinghai-Xizang (Tibet) Plateau, China, under climate warming, Sci. China Ser. D, 39, 434–441, 1996.
Li, X., Cheng, G., Jin, H., Kang, E., Che, T., Jin, R., Wu, L., Nan, Z. T., Wang, J., and Shen, Y.: Cryospheric change in China, Global Planet. Change, 62, 210–218, 2008.
Liu, X. and Chen, B.: Climatic warming in the Tibetan Plateau during recent decades, Int. J. Climatol., 20, 1729–1742, 2000.
Liu, X. D., Yin, Z. Y., Shao, X. M., and Qin, N. S.: Temporal trends and variability of daily maximum and minimum, extreme temperature events, and growing season length over the eastern and central Tibetan Plateau during 1961–2003, J. Geophys. Res., 111, D19109, https://doi.org/10.1029/2005JD006915, 2006.
Liu, X., Cheng, Z., Yan, L., and Yin, Z. Y.: Elevation dependency of recent and future minimum surface air temperature trends in the Tibetan Plateau and its surroundings, Global Planet. Change, 68, 164–174, 2009.
Luo, D. L., Jin, H. J., Lin, L., You, Y. H., Yang, S. Z., and Wang, Y. P.: Distributive features and controlling factors of permafrost and the active layer thickness in the Bayan Har Mountains along the Qinghai-Kangding Highway on Northeastern Qinghai-Tibet Plateau, Scient Geograph. Sin., 33, 635–640, 2013 (In Chinese, English abstract).
Luo, J., Niu, F., Lin, Z., Liu, M., and Yin, G.: Thermokarst lake changes between 1969 and 2010 in the beilu river basin, qinghai – tibet plateau, China, Sci. Bull., 60, 556–564, 2015.
McCalley, C. K., Woodcroft, B. J., Hodgkins, S. B., Wehr, R. A., Kim, E. H., Mondav, R., Crill, P. M., Chanton, J. P., Rich, V. I., Tyson, G. W., and Saleska, S. R.: Methane dynamics regulated by microbial community response to permafrost thaw, Nature, 514, 478–481, 2014.
Mu, C., Zhang, T., Wu, Q., Peng, X., Cao, B., Zhang, X., Cao, B., and Cheng, G.: Editorial: Organic carbon pools in permafrost regions on the Qinghai-Xizang (Tibetan) Plateau, The Cryosphere, 9, 479–486, https://doi.org/10.5194/tc-9-479-2015, 2015.
Nan, Z. T., Li, S. X., and Liu, Y, Z.: Mean annual ground temperature distribution on the Tibetan Plateau: Permafrost distribution mapping and further application, J. Glaciol. Geocryol., 24, 142–148, 2002 (in Chinese).
Nan, Z., Gao, Z., Li, S., and Wu, T.: Permafrost changes in the northern limit of permafrost on the Qinghai-Tibet Plateau in the last 30 years, Acta Geogr. Sinica, 58, 817–823, 2003 (In Chinese, English abstract).
Niu, F., Lin, Z., Liu, H., and Lu, J.: Characteristics of thermokarst lakes and their influence on permafrost in Qinghai–Tibet Plateau, Geomorphology, 132, 222–233, 2011.
Otto, J. C. and Keuschnig, M.: Permafrost-Glacier Interaction–Process Understanding of Permafrost Reformation and Degradation. permAfrost–Austrian Permafrost Research Initiative, Final Report, Innsbruck: Institute for Interdisciplinary Mountain Research, ÖAW, 3–16, 2014.
Permafrost Subcommittee, National Research Council of Canada: Glossary of Permafrost and Related Ground-ice Terms, National Research Council of Canada Technical Memorandum, 1988.
Pepin, N., Bradley, R. S., Diaz, H. F., Baraer, M., Caceres, E. B., Forsythe, N., Fowler, H., Greenwood, G., Hashmi, M. Z., Liu, X. D., Miller, J. R., Ning, L., Ohmura, A., Palazzi, E., Rangwala, I., Schöner, W., Severskiy, I., Shahgedanova, M., Wang, M. B., Williamson, S. N., and Yang, D. Q.: Elevation-dependent warming in mountain regions of the world, Nat. Clim. Change, 5, 424–430, 2015.
Qin, J., Yang, K., Liang, S., and Guo, X.: The altitudinal dependence of recent rapid warming over the Tibetan Plateau, Clim. Change, 97, 321–327, 2009.
Ran, Y. H. and Li, X.: Evaluation of the permafrost stability degradation from 1980 to 2010 in China, Sci. Cold Arid Reg., 8, 0359–0366, available at: http://www.scar.ac.cn/fulltext/2016/5/20160501.htm (last access: 6 February 2017), 2016.
Ran, Y., Li, X., Cheng, G., Zhang, T., Wu, Q., Jin, H., and Jin, R.: Distribution of permafrost in China: an overview of existing permafrost maps, Permafrost Periglac., 23, 322–333, 2012.
Ran, Y., Li, X., Jin, R., and Guo, J.: Remote sensing of the mean annual surface temperature and surface frost number for mapping permafrost in China, Arct. Antarct. Alp. Res., 47, 255–265, 2015.
Schaefer, K., Zhang, T., Bruhwiler, L., and Barrett, A. P.: Amount and timing of permafrost carbon release in response to climate warming, Tellus B, 63, 165–180, 2011.
Schuur, E. A., Vogel, J. G., Crummer, K. G., Lee, H., Sickman, J. O., and Osterkamp, T. E.: The effect of permafrost thaw on old carbon release and net carbon exchange from tundra, Nature, 459, 556–559, 2009.
Smith, L. C., Sheng, Y., MacDonald, G. M., and Hinzman, L. D.: Disappearing arctic lakes, Science, 308, 1429–1429, 2005.
Smith, M. W. and Riseborough, D. W.: Permafrost monitoring and detection of climate change, Permafrost Periglac., 7, 301–309, 1996.
Smith, M. W. and Riseborough, D. W.: Climate and the limits of permafrost: a zonal analysis, Permafrost Periglac., 13, 1–15, 2002.
Sun, Z. Z., Wu, G. L., Yun, H. B., Liu, G. J., and Rui, P. F.: Permafrost degradation under an embankment of the Qinghai-Tibet Railway in the southern limit of permafrost, J. Glaciol. Geocryol., 36, 767–771, 2014 (in Chinese, English abstract).
Stehman, S. V.: Selecting and interpreting measures of thematic classification accuracy, Remote Sens. Environ., 62, 77–89, 1997.
Stieglitz, M., Déry, S. J., Romanovsky, V. E., and Osterkamp, T. E.: The role of snow cover in the warming of arctic permafrost, Geophys. Res. Lett., 30, 1721, https://doi.org/10.1029/2003GL017337, 2003.
Tang, Z., Wang, J., Li, H., and Yan, L.: Spatiotemporal changes of snow cover over the Tibetan plateau based on cloud-removed moderate resolution imaging spectroradiometer fractional snow cover product from 2001 to 2011, J. Appl. Remote Sens., 7, 073582–073582, 2013.
Tarnocai, C., Canadell, J. G., Schuur, E. A. G., Kuhry, P., Mazhitova, G., and Zimov, S.: Soil organic carbon pools in the northern circumpolar permafrost region, Global Biogeochem. Cy., 23, GB2023, https://doi.org/10.1029/2008GB003327, 2009.
Vancutsem, C., Ceccato, P., Dinku, T., and Connor, S. J.: Evaluation of MODIS land surface temperature data to estimate air temperature in different ecosystems over Africa, Remote Sens. Environ., 114, 449–465, 2010.
Wan, Z.: New refinements and validation of the MODIS land-surface temperature/emissivity products, Remote Sens. Environ., 112, 59–74, 2008.
Wan, Z., Zhang, Y., Zhang, Q., and Li, Z. L.: Validation of the land-surface temperature products retrieved from Terra Mode rate Resolution Imaging Spectroradiometer data, Remote Sens. Environ., 83, 163–180, 2002.
Wan, Z., Zhang, Y., Zhang, Q., and Li, Z.L.: Quality assessment and validation of the MODIS global land surface temperature, Int. J. Remote Sens., 25, 261–274, 2004.
Wang, S. L., Jin, H. J., Li, S. X., and Zhao, L.: Permafrost degradation on the Qinghai-Xizang (Tibet) Plateau and its environmental impacts, Permafrost Periglac., 11, 43–53, 2000.
Wang, G., Garcia, D., Liu, Y., de Jeu, R., and Dolman, A.J.: A three dimensional gap filling method for large geophysical datasets: application to global satellite soil moisture observations, Environ. Modell. Softw., 30, 139–142, 2012.
Wang, Q. F., Zhang, T. J., Wu, J. C., Peng, X. Q., Zhong, X. Y., Mou, C., Wang, K., Wu, Q. B., and Cheng, G. D.: Investigation on permafrost distribution over the upper reaches of the Heihe River in the Qilian Mountains, J. Glaciol. Geocryol., 35, 19–25, 2013 (in Chinese, English abstract).
Wang, T., Peng, S., Lin, X., and Chang, J.: Declining snow cover may affect spring phenological trend on the Tibetan Plateau, Proc. Natl. Acad. Sci., 110, E2854–E2855, 2013.
Wang, W., Feng, Q., Yu, H., Liang, T., and Guo, N.: Spatio-temporal change of vegetation on Tibetan Plateau based on AVHRR-NDVI data. In Geoscience and Remote Sensing Symposium (IGARSS), 2016 IEEE International, 1374–1377, IEEE, 2016.
Wang, X., Wu, C., Wang, H., Gonsamo, A., and Liu, Z.: No evidence of widespread decline of snow cover on the Tibetan Plateau over 2000–2015, Scientific Rep., 7, 14645, https://doi.org/10.1038/s41598-017-15208-9, 2017.
Westermann, S., Langer, M., Boike, J., Heikenfeld, M., Peter, M., Etzelmüller, B., and Krinner, G.: Simulating the thermal regime and thaw processes of ice-rich permafrost ground with the land-surface model CryoGrid 3, Geosci. Model Dev., 9, 523–546, https://doi.org/10.5194/gmd-9-523-2016, 2016.
Williams, P. J. and Smith, M.W.: The frozen earth: fundamentals of geocryology, UK, Cambridge, Cambridge University Press, 1989.
Wu, D., Zhao, X., Liang, S., Zhou, T., Huang, K., Tang, B., and Zhao, W.: Time-lag effects of global vegetation responses to climate change, Global Change Biol., 21, 3520–3531, 2015.
Wu, J., Sheng, Y., Wu, Q., and Wen, Z.: Processes and modes of permafrost degradation on the Qinghai-Tibet Plateau, Sci. China Earth Scie., 53, 150–158, 2010.
Wu, Q. B., Zhang, Z. Q., and Liu, Y. Z.: Long-term thermal effect of asphalt pavement on permafrost under embankment, Cold Reg. Sci. Technol., 60, 221–229, 2010.
Wu, Q. B., Zhu, Y. L., and Liu, Y. Z.: Evaluation model of permafrost thermal stability and thawing sensibility under engineering activity, Cold Reg. Sci. Technol., 34, 19–30, 2002.
Xiang, Y., Xiao, Z. Q., Ling, S. L., Wang, J. D., and Song, J. L.: Validation of Global LAnd Surface Satellite (GLASS) leaf area index product, J. Remote Sens., 18, 573–596, 2014.
Xiao, Z., Liang, S., Wang, J., Chen, P., Yin, X., Zhang, L., and Song, J.: Use of general regression neural networks for generating the GLASS leaf area index product from time-series MODIS surface reflectance, IEEE Trans. Geosci. Remote Sens., 52, 209–223, 2014.
Yang, M., Nelson, F. E., Shiklomanov, N. I., Guo, D., and Wan, G.: Permafrost degradation and its environmental effects on the Tibetan Plateau: A review of recent research, Earth-Sci. Rev., 103, 31–44, 2010.
Yao, Y. and Zhang, B.: MODIS-based estimation of air temperature of the Tibetan Plateau, J. Geogr. Sci., 23, 627–640, 2013.
Ye, B. S., Ding, Y. J., and Liu, C. H.: Response of Valley Glaciers in Various Size and Their Runoff to Climate Change, J. Glaciol. Geocryol., 23, 103–110, 2001 (in Chinese, English abstract).
You, Y., Yu, Q., Pan, X., Wang, X., Guo, L., and Wu, Q.: Thermal effects of lateral supra-permafrost water flow around a thermokarst lake on the Qinghai-Tibet Plateau, Hydrol. Process., 31, 2429–2437, https://doi.org/10.1002/hyp.11193, 2017.
Yuan, X., Wang, W., Cui, J., Meng, F., Kurban, A., and De Maeyer, P.: Vegetation changes and land surface feedbacks drive shifts in local temperatures over Central Asia, Scientific Reports, 7, 2017.
Yu, H., Wu, Q. B., and Liu, Y. Z.: The long-term monitoring system on permafrost regions along the Qinghai-Tibet Railway, J. Glaciol. Geocryol., 30, 475–481, 2008 (In Chinese, English abstract).
Zhang, G., Yao, T., Xie, H., Kang, S., and Lei, Y.: Increased mass over the Tibetan Plateau: from lakes or glaciers?, Geophys. Res. Lett., 40, 2125–2130, 2013.
Zhang, T.: Influence of the seasonal snow cover on the ground thermal regime: An overview, Rev. Geophys., 43, RG4002, https://doi.org/10.1029/2004RG000157, 2005.
Zhao, L., Wu, Q., Marchenko, S. S., and Sharkhuu, N.: Thermal state of permafrost and active layer in Central Asia during the International Polar Year, Permafrost Periglac., 21, 198–207, 2010.
Zhong, L., Ma, Y., Salama, M. S., and Su, Z.: Assessment of vegetation dynamics and their response to variations in precipitation and temperature in the Tibetan Plateau, Clim. Change, 103, 519–535, 2010.
Zhu, Z., Kang, G., Ma, Y., Xie, Q., Zhang, D., and Ning, J.: Temperature damage and constitutive model of frozen soil under dynamic loading, Mechan. Material., 102, 108–116, 2016.
Zou, D., Zhao, L., Sheng, Y., Chen, J., Hu, G., Wu, T., Wu, J., Xie, C., Wu, X., Pang, Q., Wang, W., Du, E., Li, W., Liu, G., Li, J., Qin, Y., Qiao, Y., Wang, Z., Shi, J., and Cheng, G.: A new map of permafrost distribution on the Tibetan Plateau, The Cryosphere, 11, 2527–2542, https://doi.org/10.5194/tc-11-2527-2017, 2017.