the Creative Commons Attribution 4.0 License.
the Creative Commons Attribution 4.0 License.
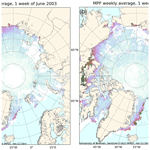
Updated Arctic melt pond fraction dataset and trends 2002–2023 using ENVISAT and Sentinel-3 remote sensing data
Hannah Niehaus
Gunnar Spreen
Melt ponds on Arctic sea ice affect the radiative balance of the region as they introduce darkening of the sea ice during the Arctic summer. The temporal extent and spatial extent of the ponding, as well as its amplitude, reflect the state of Arctic sea ice and are important for our understanding of Arctic sea ice change. Remote sensing retrievals of melt pond fraction (MPF) provide information on both the present state of the melt pond development and its change throughout the years, which is valuable information in the context of climate change and Arctic amplification.
In this work, we transfer the earlier published Melt Pond Detector (MPD) remote sensing retrieval to the Ocean and Land Colour Instrument (OLCI) data on board the Sentinel-3 satellite and so complement the existing Medium Resolution Imaging Spectrometer (MERIS) MPF dataset (2002–2011) from Environmental Satellite (ENVISAT) with recent data (2017–present). To evaluate the bias of the MPF product, comparisons to Sentinel-2 MultiSpectral Instrument (MSI) high-resolution satellite imagery are presented, in addition to earlier published validation studies. Both MERIS and OLCI MPD tend to overestimate the small MPFs (ranging from 0 to 0.2), which can be attributed to the presence of water-saturated snow and sea ice before onset of ponding. Good agreement for the middle-range MPF (0.2–0.8) is observed, and the areas of exceptionally high MPF = 100 % are recognized as well.
The earlier published MERIS MPFs (2002–2011) were reprocessed using an improved cloud clearing routine and together with recent Sentinel-3 data provide an internally consistent dataset, which allows the MPF development in the past 20 years to be analyzed. Although the total summer hemispheric MPF trend is moderate, at +0.75 % per decade, the regional weekly MPF trends display a pronounced dynamic and range from −10 % to as high as +20 % per decade, depending on the region. We conclude the following effects:
-
The global Arctic melt onset shifted towards spring by at least 2 weeks, with the melt onset happening in late May in recent years as compared to early June to mid-June in the beginning of the dataset.
-
There has been a change in the pond onset regime in recent years, with the East Siberian and Laptev Sea dominating the melt onset and not the Beaufort Gyre region as before.
-
The central Arctic, north Greenland and the Canadian Arctic Archipelago (CAA) have shown signs of increasing first-year ice (FYI) fraction in recent years.
The daily gridded MPF averages are available on the web page of the Institute of Environmental Physics, University of Bremen, as a historic dataset for the ENVISAT data and as ongoing operational processing for the Sentinel-3 data.
- Article
(10225 KB) - Full-text XML
- BibTeX
- EndNote
The last 9 years, 2016–2023, has been the warmest period in history (WMO, 2023), and summer 2023 was the hottest on record (C3S, 2023). The Arctic is warming 3 to 4 times faster than the rest of the world (Rantanen et al., 2022). The summer sea ice of today has reduced to half of its average extent of the 1980s (Perovich et al., 2020), and it has become younger (Tschudi et al., 2020, Stroeve and Notz, 2018). The floe residency time and ice thickness have been reducing as well (Haas et al., 2008; Sumata et al., 2023). Due to the open ocean being darker than sea ice, both lateral sea ice melt and surface sea ice melt decrease the albedo of the Arctic Ocean during summer, affecting the energy budget and contributing to direct and indirect surface albedo feedbacks within the Arctic amplification mechanisms (Wendisch et al., 2023).
According to the Global Climate Observing System (GCOS), sea ice is one of the Essential Climate Variables (ECVs). The primary parameters of the sea ice ECV are ice concentration, area and extent, ice type, motion, deformation, age, thickness, and volume (GCOS-200, 2016). While sea ice albedo has been included recently (GCOS-244, 2022), sea ice surface melt, which is a main contributor to the decreasing albedo and increasing transmittance of the sea ice in summer (Perovich et al., 2002; Nicolaus et al., 2012; Light et al., 2022), is not yet considered a part of sea ice ECV. At the same time, the melt pond fraction is not directly linked to the sea ice albedo, as the same fraction of melt pond can have very different albedo depending on the pond type, and vice versa.
Satellite remote sensing has been used to produce many of the sea ice ECV datasets to obtain pan-Arctic coverage (Sandven et al., 2023). However, the passive microwave (PM) sea ice concentration (SIC), which is used to produce the sea ice area and extent, is compromised by summer sea ice surface melt due to open water being indistinguishable from melt ponds at these frequencies (Ivanova et al., 2015; Kern et al., 2019, 2020, 2022). PM L-band and altimeter sea ice thickness datasets do not provide their products in the presence of melt ponds (Huntemann et al., 2014; Paţilea et al., 2019; Ricker et al., 2017), with altimeter retrievals having difficulties to retrieve sea ice freeboard in the presence of melt ponds. Only recently has an altimeter-based sea ice thickness retrieval coupled with a machine learning approach been presented (Landy et al., 2022). A PM-based sea ice drift product is affected during the melt season and is complemented with a parametric model during summertime (Lavergne and Down, 2023). Not only does the most drastic sea ice change happen during the Arctic summer, but also it is the most challenging season for the Arctic remote sensing retrievals.
Global climate models (GCMs) have difficulty simulating melt ponds as well as they do not include sea ice topography, the major factor determining the melt pond fraction (MPF). At the time of writing, sea ice melt ponding has been included in GCMs via parameterizations (Flocco et al., 2010; Hunke et al., 2013; Schroeder et al., 2014; Zhang et al., 2018). However, agreement in terms of GCM melt pond representation is yet to be reached, and the lack thereof might explain parts of the existing discrepancies between long-term GCM sea ice forecasts (Stroeve et al., 2012).
Although melt ponds have been observed in situ for many decades (Yackel et al., 2000; Perovich et al., 2001, 2002; Eicken et al., 2004; Polashenski et al., 2012; Webster et al., 2022; Light et al., 2022), the spatial and temporal coverage of these observations is sporadic. There is a need for a high-quality remote sensing MPF dataset that can be assimilated into the GCM and be potentially included in the sea ice ECV.
The currently published satellite MPF datasets can be put in the following main groups: optical, passive microwave (PM) and synthetic aperture radar (SAR). Among the optical MPF datasets, the spatial resolution defines the algorithm approaches depending on whether the melt ponds can still be detected separately or are already subpixel. The following sensor and algorithm groups can be distinguished: very high resolution (0.3–10 m, WorldView, Pleiades, commercial sensors, Wright and Polashenski, 2018; Webster et al., 2015), high-resolution (10–60 m, e.g., Sentinel-2 MultiSpectral Instrument (MSI), Landsat-7 and Landsat-8, Rösel and Kaleschke, 2011; Wang et al., 2020; Li et al., 2020; Qin et al., 2021; Niehaus et al., 2023) and moderate-resolution (250–1000 m, e.g., Moderate Resolution Imaging Spectroradiometer (MODIS) (Tschudi et al., 2008; Rösel et al., 2012; Ding et al., 2020; Lee et al., 2020; Feng et al., 2020; Peng et al., 2022) and Medium Resolution Imaging Spectrometer (MERIS) (Zege et al., 2015; Istomina et al., 2015a)). In terms of swath width and revisit time, out of all optical sensors, those of moderate resolution have the most potential to obtain daily pan-Arctic coverage. Sensors of higher resolution, although providing potential for high-quality retrievals as the melt ponds are no longer subpixel, have limited spatial coverage and can be used as comparison datasets to evaluate the moderate-resolution retrievals. However, it must be noted that optical observations are hindered by clouds.
The PM-sensor-based MPF retrievals (AMSR-E, AMSR-2 (Tanaka et al., 2016; Tanaka and Scharien, 2022), SMOS (Mäkynen et al., 2020)) do not have this disadvantage as they are only partly sensitive to the atmospheric influence at higher frequencies (89 GHz, spatial resolution 3 km). The coarse spatial resolution of lower frequencies (37–1.4 GHz, 10–40 km) renders the vast majority of data a subpixel mixture of lots of Arctic summer surface types. At microwave frequencies, the imaginary part of the complex permittivity of water differs by orders of magnitude from that of sea ice or snow; therefore the penetration depth in snow and sea ice reduces drastically (from decimeters to submillimeters) in the presence of surface wetness and melt. Low penetration depth means that the underlying sea ice in the pond (“pond bottom”) cannot be recognized (that is, melt pond cannot be distinguished from open water); therefore PM MPF retrievals can only be used for dry, cold sea ice surfaces with open melt ponds at 100 % ice concentration. When applied globally during Arctic summer, the resulting MPF will be biased as PM MPF retrievals cannot distinguish between open water, water-saturated surfaces and melt ponds.
The available Synthetic Aperture Radar (SAR) MPF retrievals (Scharien et al., 2018; Han et al., 2016; Fors et al., 2017; Li et al., 2017; Ramjan et al., 2018; Howell et al., 2020), in addition to limitations on the spatial coverage, are also affected by the inability to distinguish melt ponds and open water due to their equally low backscatter signal in calm conditions or equally high backscatter signal in windy conditions. In general case, an unknown sea ice surface roughness has to be resolved from an unknown water/melt pond surface roughness, and the angular backscatter dependency delivers additional challenges in terms of signal-to-noise ratio. In some SAR scenes of higher resolution, however, single melt ponds can be detected by their shape.
Among optical/IR spectroradiometers, MODIS is the one with the longest time series of data available (Arctic data from MODIS Terra since 2000 and MODIS Aqua since 2002, Terra and Aqua being satellite platforms with MODIS sensors). MODIS data are being comprehensively utilized to provide a variety of higher-level products in addition to the Level-1B top-of-atmosphere (TOA) spectral reflectance. Important for MPF retrievals are the composite daily and 8 d cloud-free surface reflectance products. After neural network approaches (Rösel et al., 2012) applying the initial threshold (Tschudi et al., 2008) and fixed surface classes, neural network MPF retrievals that use high-resolution training data have followed (Ding et al., 2020; Lee and et al., 2020; Feng et al., 2020; Peng et al., 2022). It is important to note the issues of the MODIS sensors such as the saturation over bright surfaces (Madhavan et al. 2012) and striping issues (Lee et al., 2020), which disturb the TOA reflectance and might affect the MPF retrievals. In addition, the great variability of the sea ice and melt pond inherent scattering properties stems from the past and potentially future changes in the Arctic sea ice type and other properties. Therefore, an adequate and versatile summer sea ice representation is required; therefore limited training datasets used in the neural network approaches might not always suffice.
In this work, we present a MPF dataset based on an inversion of a physical forward model of snow-covered and bare sea ice with melt ponds. This dataset is based on the algorithms described (Zege et al., 2015, Malinka et al., 2016, Malinka et al., 2018) and consists of an improved version of an earlier published historic MERIS dataset (Istomina et al., 2015a) and a new operational Ocean and Land Colour Instrument (OLCI) MPF dataset. As the MPD algorithm takes nine spectral channels, we do not use MODIS due to the saturation issue mentioned above and use MERIS (ENVISAT) and OLCI (Sentinel-3) instead. We perform comparisons to high-resolution MPF data, investigate the internal consistency of the combined MERIS and OLCI dataset, and present Arctic-wide MPF trends for 2002–2023 as an update to MPF trends 2002–2011 (Istomina et al., 2015b). The structure of the paper is presented as a flowchart in Fig. 1.
The objective of this work is to continue the historic ENVISAT MPF dataset published earlier (Istomina et al., 2015b) using the validated MPD method (Zege et al., 2015; Istomina et al., 2015a) and Sentinel-3 OLCI data. As the optical sensors OLCI and Sea and Land Surface Temperature Radiometer (SLSTR) on board Sentinel-3 are built to be direct successors of MERIS and Along Track Scanning Radiometer (AATSR) on board ENVISAT, the earlier published MERIS MPD retrieval can be applied. For this, we improve the earlier published cloud screening (Istomina et al., 2020) for the updated MERIS dataset and also use MERIS-consistent pre- and postprocessing routines for the Sentinel-3 OLCI data.
Table 1Moderate-resolution spectroradiometers used in this work; spectral bands used in MPD retrieval are shown in bold italic.
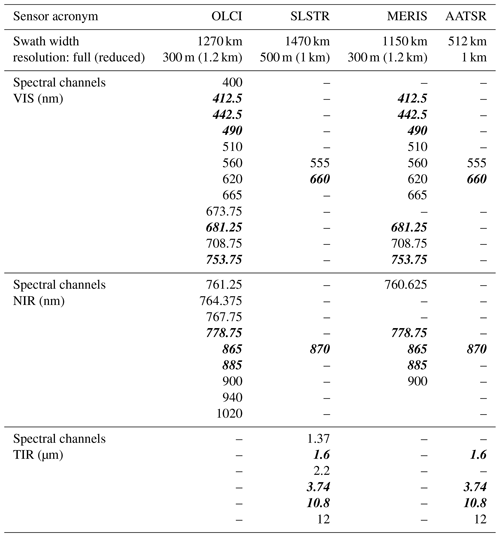
2.1 Data used
The following remote sensing data have been utilized for the MPD retrieval (their summary is given in Table 1):
-
ENVISAT (2002–2012) – Arctic data available 2002–2011, sensors MERIS (MPD MPF retrieval) and AATSR (training of the Bayesian cloud screening method MEris Cloudscreening Over Snow and Ice (MECOSI)),
-
Sentinel-3A and 3B (since 2016 till present) – Arctic data available since 2017, sensors OLCI (MPD MPF retrieval) and SLSTR (in synergy with OLCI – a cloud screening routine used as reference for MECOSI).
An overview of the spectral and spatial resolution characteristics of these sensors is given in Table 1.
To evaluate the quality of the obtained MPF dataset, we use Sentinel-2 MSI data for the OLCI dataset (Table 2); see Sect. 3 for details.
2.2 MPD retrieval
The MPD retrieval has been developed by Zege et al. (2015). The MPD algorithm takes Level-1B top of atmosphere reflectances at nine spectral channels as input (Table 1) and inverts the forward physical model of melting sea ice (Malinka et al., 2016) with melt ponds (Malinka et al., 2018) to obtain the fraction of melt ponds in a given pixel as well as its black-sky spectral albedo at 400, 500, 600, 700, 800 and 900 nm, which is then converted into broadband albedo according to Pohl et al. (2020). The sea ice is modeled as a stochastic medium and can represent various inclusions such as air bubbles, brine and sediment. The parameters controlling the sea ice scattering properties are optical thickness of the ice τice, effective grain size of the scattering layer or snow cover Aeff, and the absorption coefficient of inclusions αinc. The melt pond is represented as a Lambertian melt pond bottom of varying optical thickness τpond with the ice transport scattering coefficient σice and a freshwater layer of varying depth τmeltwater on top. The atmospheric correction is performed using a fast radiative transfer model (Tynes et al., 2001) for typical Arctic aerosol conditions (Tomasi et al., 2007). The constraints on the sea ice and melt pond model parameters are obtained from ∼ 200 field spectra of sea ice and melt ponds measured by Istomina et al. (2013) during August 2012 in the Central Arctic. This dataset contains spectra of bare ice of various grain sizes, snow, dark and light melt ponds with or without the ice lid, and blue ice without the scattering layer, for a range of ice thickness from 30 cm to 2.5 m within the melt ponds. To perform the model inversion, we use the Newton–Raphson method (Press et al., 1992). The resulting MPF is defined as the fraction of melt ponds divided by the fraction of ponded and not ponded sea ice. In the case of open water being present in the pixel, the resulting MPF deviates from this definition; see Sect. 3.2 for details.
The MPD has been validated against in situ, ship-based and airborne data (Istomina et al. (2015a). Case studies and trends for the MERIS dataset have been presented by Istomina et al. (2015b).
MPD does not perform cloud/surface classification of the input TOA reflectances and therefore relies on external cloud screening and sea ice and snow flagging. The details of this procedure can be found in Sect. 2.3.
2.3 Cloud screening
A cloud screening routine of high quality is essential for the MPD retrieval as unscreened clouds contaminate the MPF product. Cloud screening over snow and ice is a challenging task as both clouds and the surface are bright and white in the visible spectral range. Near-infrared (NIR) and thermal infrared (TIR) spectral bands have been proven to be more effective for the task (e.g., Ackerman et al., 1998). As both MERIS and OLCI sensing range is limited to 900 and 1020 nm, respectively, we use synergy with, respectively, AATSR and SLSTR for TIR channels (Table 1).
For the MERIS part of the dataset, we use the Bayesian cloud screening method MECOSI (Istomina et al., 2020). In MECOSI, a set of spectral and spatial features is utilized, using a VIS, NIR and TIR-based AATSR cloud mask (Istomina et al., 2010, 2011) as a reference dataset. This approach is based on selecting the spectral behavior of snow and ice surfaces and screening out all other surfaces. This means that clouds and cloud shadows are screened out as they do not show the spectral signature of snow and ice. In this work, we improve the reference dataset by omitting Eq. (3) in Istomina et al. (2010), as this snow flag correctly screens out higher MPFs, which must be preserved for this work. We also apply a threshold of 0.05 to the reflectance component of the 3.7 µm brightness temperature (BT) channel as described in Istomina et al. (2011) to help separate snow and ice from clouds. Then, the Bayesian approach is used to expand the reference AATSR cloud mask to the entire MERIS swath, as the AATSR swath only covers one-third of the MERIS swath. The resulting swath-wise cloud mask is applied to the MERIS swaths, and the pixels which are cloud-free during at least one overpass are included (as opposed to areas consistently cloud-free throughout the entire day in Istomina et al., 2020).
For the OLCI part of the dataset, as the SLSTR swath covers the OLCI swath completely, we use the MECOSI reference mask routine directly on the SLSTR and OLCI data. As SLSTR is the AATSR successor, no adaptation is needed.
During the SLSTR sensor recalibration, the TIR channels are either unavailable or of degraded quality (e.g., 300 K over snow and ice in the Arctic); therefore the TIR part of the cloud screening cannot be used. In these cases, we alternate between the Sentinel 3A and 3B platforms for a given day.
2.4 Daily gridded product
The adaptation of the MPD retrieval to the OLCI data comprises accounting for the data format differences, producing synergy with the SLSTR data and establishing the operational processing. We use the C Foreign Function Interface (CFFI) Python package to write the wrapper on the MPD retrieval, which is written in the C programming language. OLCI data preprocessing is done with the GPT tool of the Brockmann Consult Java-based SNAP software.
Per default, Sentinel-3A data are used due to its longer dataset starting in 2017 as compared to 2018 for Sentinel-3B. In cases that SLSTR or OLCI data discontinuity occurs for the given platform, Sentinel-3B data for that entire day are used, to produce possibly consistent daily averages of the MPF. Typically, 13–15 OLCI swaths per day are processed, with about five SLSTR granules corresponding to each OLCI swath subset.
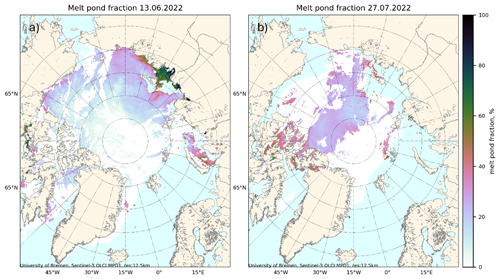
Figure 2Example of the daily gridded MPD product for (a) 13 June 2022 and (b) 27 July 2022. Note the variable MPF on 13 June 2022 during the melt onset and the uniform MPF during the evolution of melt on 27 July 2022. The white areas depict the cloud-covered sea ice where no MPF retrieval is possible. Sea ice coverage is given by the ARTIST Sea Ice (ASI) PM sea ice concentration product (Spreen et al., 2008).
The cloud-screened MERIS and OLCI swaths are gridded into a 12.5 km polar stereographic grid and stored as NetCDF files available for download. The OLCI and SLSTR files used for a given swath or a given daily average are stored in the corresponding NetCDF files as metadata for future reference. The minimal number of cloud-free OLCI pixels to form a valid 12.5 km grid cell is Nvalid_OLCI > 50. As we exclude the dark pixels with the RTOA_412.5 nm < 0.3 already earlier during the swath data processing, by limiting the number of valid OLCI pixels during gridding, we exclude residual pixels of darker ice just above the threshold, e.g., in an otherwise ice-free area. In addition, we remove an edge of 2 pixels on the cloud-free areas in the swath data before gridding into the daily average. In this way, we preserve larger areas of continuous coverage but remove single pixels or data with pixel-wise gaps, as we expect these situations to occur in the areas where MPD cannot be applied (broken ice, slush ice, ice edge with lower SIC, cloud shadows around cloudy areas). Examples of the daily product for the melt onset (13 June 2022, Fig. 2a) and for the height of the melt season (Fig. 2b, 27 July 2022) are shown in Fig. 2.
The MPD algorithm has been designed for use in areas of high SIC with bare or snow-covered dry sea ice covered with blue melt ponds (Zege et al., 2015; Istomina et al., 2015a). When applying the algorithm to global data, deviations from this scenario are possible, and potential bias needs to be investigated. In Sect. 3.1, we evaluate the quality of the MPD product for a range of MPF values with the help of the Sentinel-2 comparison dataset.
3.1 Evaluation of the full-resolution swath MPF dataset
The MPD algorithm is based on the physical forward model of sea ice and melt ponds with boundary values for the parameters of air bubbles, brine inclusions and pollutants derived from field data. Its potential to account for the geophysical variability of the Arctic sea ice has been confirmed by in situ data (Malinka et al., 2016, 2018). However, a correct interpretation of the sea ice scattering parameters from TOA reflectances of subpixel sea ice combined with melt ponds is not a trivial task. While there are satellite retrievals of, e.g., effective grain size Aeff of snow and sea ice (e.g., Wiebe et al., 2013), the knowledge of sea ice inherent scattering properties (e.g., spectral extinction coefficient or better the transport scattering coefficient σice as used in MPD) is limited (Perovich, 1996).
Comparisons of the MERIS MPD algorithm to the point measurements on in situ, airborne and shipborne data were performed by Istomina et al. (2015a), and comparisons to very high resolution (VHR; 1 m pixel size) satellite data were presented by Marks (2015). When applying the MPD to the moderate-resolution optical data like that of MERIS or OLCI, subpixel mixtures of many surface types occur, and high-resolution (HR) satellite imagery can aid in correct upscaling of the ground truth to the global scale. As the spatial resolution of HR data is still lower than that of in situ aerial photographs or VHR data, a classification and retrieval routine of its own is necessary to obtain the comparison dataset.
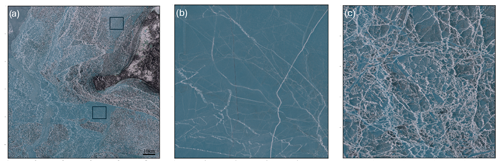
Figure 3(a) RGB of the first full-resolution comparison case on 1 June 2021, MSI tile T54XVG, 03 h 05 min 49 s, relative orbit = R075, at landfast ice in the Laptev Sea. (b) RGB subset for the bottom square in (a). (c) RGB subset for the top square in (a).
In this work, we perform OLCI MPD comparison to 10 m resolution Level-1C orthorectified TOA reflectances of Sentinel-2 MSI. For the evaluation of the MPF from the Sentinel-2 MSI imagery, a classification algorithm by Niehaus et al. (2023) is used. In this algorithm, the difference between the spectral bands of wavelengths 490 and 842 nm within ice surface types is exploited (Grenfell and Maykut, 1977) as an adaptation of the LinearPolar algorithm (Wang et al., 2020) for larger areas. Here the main feature of the melting ice as opposed to white ice, namely the decreased near infrared reflectance, is exploited, thus making it possible to only use a ratio of two channels to, in the first approximation, separate melting and non-melting sea ice. The resulting MPF is assigned under the assumption of linear mix between fixed sea ice and melt pond principal axes. To ensure geospatial variability of the validation data, we use a comprehensive MPF dataset presented by Niehaus et al. (2023), with an addition of exceptionally high MPF on the landfast ice around Tiksi Bay in Laptev Sea, which is also presented as a case study below. In total, 50 scenes from June–August 2017–2023 are used for the evaluation (Table 2). The resulting Sentinel-2 MSI MPF is downsampled to 300 m for comparison to the full-resolution Sentinel-3 OLCI MPF and to 12.5 km for comparison to the daily averaged OLCI MPF. Sentinel-2 MPF with the spatial resolution of 10 m offers a drastic increase in the validation dataset quality compared to the validation effort presented earlier (Istomina et al., 2015a) and thus allows us to significantly improve our understanding on the MPD algorithm performance as compared to the previously published validation (Istomina et al., 2015a). Please note that the MPD algorithm presented here was not modified and is the same as that published by Istomina et al. (2015a), with the exception of a uniform cloud screening applied to both MERIS and OLCI parts of the dataset. Therefore, the data comparison and the spectral mixing clarification presented in Sect. 3 can be seen as performance assessment of the original 2-surface MPD retrieval, e.g., when the long-term MPF dataset is used as input to climate models.
For comparisons at full resolution, we have selected two MSI tiles both observed on the 1 June 2021: T54XVG and T53XMD, which are relative orbits R075 and R004, respectively. The selection of these two cases stems from the need to illustrate the MPD performance on the entire span of MPF range, from low to very high MPFs of 100 %, possibly showing the spectral ambiguity issue without the open-water influence. The effect of the open water on the MPD retrieval is discussed further along in the text (Fig. 7) and in Niehaus et al. (2024).
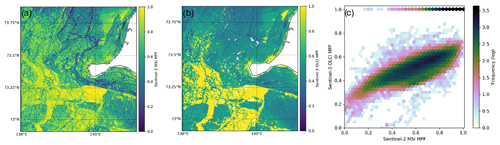
Figure 4(a) MSI MPF for the case in Fig. 3a. (b) Corresponding OLCI MPF, OLCI granule from 1 June 2021, 01 h 31 min 48 s, cycle number 072, relative orbit 231, 300 m resolution. (c) Density plot of OLCI MPF correspondence to MSI MPF. Note the bimodal OLCI MPF for MSI MPF = 1.
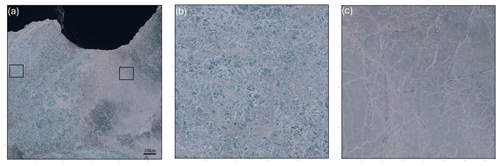
Figure 5(a) RGB of the second full-resolution comparison case on 1 June 2021, MSI tile T53XMD, 03 h 35 min 39 s, relative orbit = R004. (b) RGB subset for the left square in (a). (c) RGB subset for the right square in (a).
In the first comparison case, an exceptionally high melt pond occurrence on the landfast ice took place (MSI tile T54XVG, orbit R075, Fig. 3a). The typical MPF values on first-year ice (FYI) are assumed to be ∼ 20 %–40 % during the height of melt season and up to 80 % during the pond onset peak, where lateral meltwater transport is responsible for the high MPF (Eicken et al., 2004; Polashenski et al., 2012). An example of this process is the presented case where Sentinel-2 MSI detects a continuous field of MPF = 100 % stretching at least over 80 km (Fig. 4a). The Sentinel-3 OLCI MPF also detects the area of 100 % MPF, showing good agreement (Fig. 4b) to MSI MPF. MSI MPF values in the range 0.6–0.7 are slightly underestimated by the OLCI MPD retrieval (Fig. 4c), e.g., for the case shown in Fig. 3c. The two retrievals agree, with a correlation coefficient (R) = 0.778 and a root mean square deviation (RMSD) = 0.147. Bimodal OLCI MPF behavior (OLCI MPF = 1 and MPF = 0.65) in the high MSI MPF is visible (Fig. 4c; see upper MSI MPF range ∼ 0.9). As the subpixel mixture of melting sea ice and melt ponds is spectrally ambiguous, the MPD retrieval finds two different solution families equally fitting to these similar conditions, with the retrieval changing the ice and melt pond optical parameters without changing their fractions, as long as the boundary conditions allow, so that one set of measured TOA reflectances is being represented by a variety of surface-type mixtures equally well. The jump towards MPF = 1 happens when the boundary condition on the transport scattering coefficient of ice under the melt pond σice = 5 is reached. Figure 3a shows MSI RGB for the area of MPF = 1, where MPD MPF and MSI MPF agree well (Fig. 3a, lower square, also see Fig. 4a and b). Here we see a continuous field of uniform blue ice with meltwater on top. The area where higher MSI MPF values of 0.8–0.9 have been underestimated by OLCI MPD (MPF = 0.7) is shown in Fig. 3c and in Fig. 3a, marked by the top square. Here, the sea ice surface is not as smooth: a high fraction of ridges with accumulated snow and bright features on top of the melt ponds can be observed. In the case of fresh snow (Aeff ∼ 50 µm), the NIR (> 700 nm) feature of the snow grain size causes an increase in the TOA reflectance as compared to larger grain sizes (e.g., Burkhart et al., 2017), mimicking the increased subpixel fraction of melting sea ice (Aeff ∼ 500 µm and greater) and causing the MPF underestimation. The effective grain size (mean photon path length in a stochastic medium as modeled by MPD) at the retrieval convergence for the misclassified MPF (top square) is Aeff = 1600 µm and Aeff = 2300 µm for the correct MPF (bottom square), with corresponding optical thicknesses of white ice τice = 53 and 10. This confirms the assumption of the fresh snow presence under an assumption of otherwise equal sea ice properties. Subpixel fresh snow on partially frozen-over melt ponds would likely not be visible in MSI MPF but can potentially be detected within MPD. Additionally, the inclined topography of the ridges might also bias the MPD retrieval as it distorts the directional reflectance properties of the (flat) sea ice surface assumed within MPD.
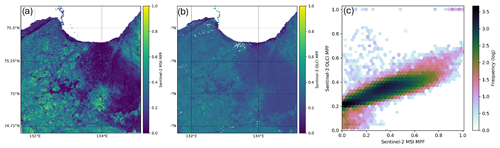
Figure 6(a) MSI MPF for the case in Fig. 5a. (b) Corresponding OLCI MPF, OLCI granule from 1 June 2021, 01 h 31 min 48 s, cycle number 072, relative orbit 231, 300 m resolution. (c) Density plot of the OLCI MPF correspondence to MSI MPF. Note the bimodal OLCI MPF for MSI MPF < 0.1.
The second comparison case (MSI tile T53XMD, orbit R004, Fig. 5a) shows moderate MPF ∼ 0.4, which agrees well with OLCI MPF, with areas of low MPF between 0 and 0.1 being overestimated by OLCI MPD (Fig. 6a and b). The good agreement at mid-range MPF changes into OLCI MPF displaying bimodal behavior for the lower MSI MPF (Fig. 6c). Analysis of the MSI RGB for the area of good correspondence (Fig. 5b or left square on Fig. 5a) shows white ice with light-blue melt ponds, the conditions for which the MPD retrieval has been designed. The area of MPD misclassification, where lower MSI MPFs were overestimated by OLCI, is shown in Fig. 5c and in Fig. 5a with the right square. Here, MSI MPF is less than 0.1, whereas OLCI MPF is 0.2. There are no visible melt ponds on top of the sea ice in Fig. 5c but a darker water-saturated sea ice without thick snow cover or scattering layer or subnivean ponds with meltwater already gathering on top of the sea ice but still beneath the snow cover but also possibly thin sea ice. The spectral ambiguity presented in Fig. 8 prevents us from confident determination of the surface type at hand. The spectral reflectance of sea ice surface just before melt differs only in amplitude but not so much in spectral shape from that of melt ponds (Istomina et al., 2013); therefore misclassifications can occur due to the spectral ambiguity of the ice–pond mixture. The MPD grain size for this misclassification case (right square) is Aeff = 500 µm, whereas the correctly retrieved case (left square) shows Aeff = 1500 µm (white ice with light-blue melt ponds). The boundary σice = 5 is reached for the left square but not the right (σice = 3.29). τice is 25 for the correct classification and lower (10) for the misclassified case. The MPD retrieval appears to alternate between fine snow grains of fresh snow and high absorption of the water-saturated sea ice underneath, which supports the assumption of a translucent scattering layer or snow on top of this blue ice, with both surfaces influencing the OLCI TOA reflectance. The two retrievals agree with R = 0.85 and RMSD = 0.132, and as in the previous case shown in Fig. 3c, the spectral ambiguity of the different sea ice surfaces causes two solution families to be present in this MPD run and causes bimodal distribution of the OLCI MPF in the high MSI MPF range. Investigations to improve this behavior of the MPD retrieval with respect of investigating the boundary conditions (Malinka et al., 2016) within the Newton–Raphson inversion routine (Zege et al., 2015) led to including the third surface class and are shown in Niehaus et al. (2024).
The presented comparisons of the OLCI MPF against MSI MPF (Figs. 3c and 5c) resemble the comparisons of the MERIS MPF to Global Fiducials Library (GFL) imagery (Marks, 2015), with good agreement of the values in the middle range but overestimation of the lower MPF range and underestimation of the higher MPF by MPD. As in the case of GFL comparison, this can be explained by the ambiguity of the sea ice–melt pond mixture, where inherent scattering properties of sea ice and melt pond are rather being varied to reproduce the TOA reflectance without changing the corresponding sea ice and melt pond fractions far enough. A new feature of the presented comparison, namely the good agreement for MPF = 1, was not analyzed for MERIS MPD for the absence of corresponding HR satellite data for the MERIS dataset. The exceptionally high MPF occurrence is, however, also present in the OLCI and MERIS MPF for other years (e.g., for 2022 in Fig. 2a), with an increasing tendency in recent years (see Sect. 4 for corresponding MPF trends).
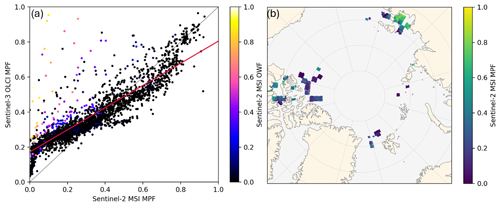
Figure 7Evaluation of the daily gridded OLCI MPF dataset. (a) The comparison of the MSI MPF to OLCI MPF in relation to the fraction of open water as seen by MSI, R = 0.86, N = 3152, RMSD = 0.13, intercept = 0.17 and slope = 0.63. (b) The spatial distribution of the MSI validation data used in (a), remote sensing time 2017–2023; see Table 2 for details on the MSI dataset used.
3.2 Evaluation of the daily gridded MPF dataset
In order to assess the MPD quality of the daily gridded product before analyzing the spatial trends in Sect. 4, the 50 MSI scenes were downsampled to the 12.5 km grid and compared to the daily OLCI MPFs (Fig. 2). Unfortunately, due to the Sentinel-2 MSI only observing coastal areas, the central Arctic and typical pack multiyear ice (MYI) areas, which are rougher than the FYI, meaning this increase in sea ice relief would lead to a decrease in the maximal possible MPF for this ice type, are not represented. Nevertheless, the entire MPF range is present. The comparison scatter plot and the corresponding Sentinel-2 MSI data distribution are shown in (Fig. 7). Also, see Table 2 for details on the used MSI data. In this dataset the sea ice type is not exclusively landfast; therefore the open-water fraction (OWF; OWF = 1 −SIC) might affect the OLCI MPD retrieval. This is observed in Fig. 7a, where the majority of the MPD strong overestimation in the lower MPF range can be explained by a subpixel OWF (colored points). The grid cells with OWF = 0 display similar behavior to that presented in the case study above, with a characteristic overestimation of small MSI MPF < 0.1 and underestimation around MSI MPF > 0.7. Overall, the two datasets show good agreement, with R = 0.86, sample size N = 3152, RMSD = 0.13, intercept = 0.17 and slope = 0.63. The more detailed comparison of the MSI MPF dataset to MPD MPF aimed to improve the MPD retrieval and to include the open-water class in the inversion is presented in Niehaus et al. (2024) in the original OLCI resolution for each pixel.
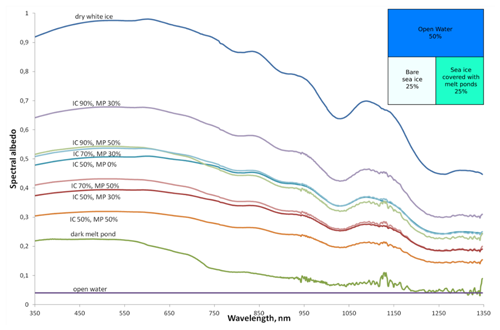
Figure 8Linear mixture of in situ-measured spectra of white ice and dark melt pond as the most frequent Arctic scenario. Moderate-resolution spectroradiometers with optical and NIR bands will not be able to distinguish the influence of MPF from the influence of open water (OW) for ice concentrations (ICs) < 90 %. Spectral data for dark melt pond and dry white ice surfaces are taken from Istomina et al. (2016); open water is assumed to have a constant spectral albedo of 0.04.
It is important to note the offset of the OLCI MPF for the lower range of MSI MPF < 0.1. Although this dynamic is also persistent throughout the entire validation effort for MERIS (Zege et al., 2015; Istomina et al., 2015a; Marks, 2015), the pan-Arctic maps show MPF values in the range 0.01–0.05 regularly (e.g., Figs. 2 and 10a). A possible reason for the observed discrepancy in the absence of open water is the misclassification of the water-saturated sea ice for melt ponds surrounded by a surface with fine Aeff (Fig. 6), discussed above, as these surface classes are spectrally ambiguous in a subpixel situation. This spectral ambiguity also occurs for open water and is illustrated in Fig. 8.
Here, we mimic the Arctic conditions and mix various fractions of the three surface types: open water, bare white ice and dark melt pond, representing the frequent ice and melt pond types (spectra taken from Istomina et al., 2016). We mix them linearly with various fractions. The resulting spectra are shown in Fig. 8. The scenarios with SIC 50 %–90 % and MPF 0 %–50 % (green and blue lines in Fig. 8), as well as MPF of 30 %–50 % with SIC 50 %–70 % (red lines in Fig. 8), are challenging to distinguish correctly given the coarse spectral resolution of the moderate-resolution spectroradiometers like MERIS and OLCI but also MODIS and VIIRS. Figure 8 presents only one spectrum for each sea ice and melt pond type for the sake of clarity; given the great in situ surface-type variability (Istomina et al., 2013, 2016), sea ice and melt pond are each represented by partly overlapping families of spectra, with an addition of surface types such as blue ice, drained melt pond, young ice, etc. Therefore, in the absence of additional information, the influence of the subpixel open water on the retrieved MPF is virtually impossible to resolve and would cause mutual misclassification of open water and melt ponds and vice versa. The results are falsely interdependent melt pond and open-water classes, as shown for the case of the neural network MODIS MPF retrieval (Rösel et al., 2012) by Marks (2015, Sect. 4.4 and Fig. 4.27 therein). It is important to note that this misclassification can potentially occur also in the areas of SIC = 100 %, thus biasing even this favorable MPF situation of two surface classes only. The reason for this being the ambiguity of the spectral TOA reflectance measured by moderate-resolution spectroradiometers like MERIS, MODIS and OLCI (Fig. 8), the three-surface MPF retrieval will not be able to distinguish whether all three surfaces are present and, if so, which of the surfaces are present. This is due to the fact that a multitude of surface combinations and fractions give the same TOA reflectance, making the inverse retrieval from this TOA reflectance to derive the subpixel surface fractions inaccurate. This means that, given no additional external information, the three-surface MPF retrieval will be always able to find a suitable combination of three surfaces, even when only two surfaces are present, as the spectral TOA reflectance it obtains from the satellite data does not constrain the surface mixture confidently. Which of this many combinations it then mostly finds depends on the training and calibration of the algorithm, but since the limited training data present limited surface conditions, there will always be conditions for which the three-surface MPF retrieval without additional data is not able to retrieve data correctly. Niehaus et al. (2024) present the three-surface retrieval with additional data and address this issue in detail.
To avoid this and to preserve the quality of the MPD MPF in the areas of 100 % SIC, we refrain from separating the observed RTOA into three surface classes and therefore expect some MPF overestimation in the areas with lower SIC, as is shown in Fig. 7a. Here, the SIC is shown as color-coding of the data points. It can be seen that low SIC < 50 % causes a strong overestimation of the MPD MPF, especially for the cases of low MSI MPF and bright sea ice surfaces. This confirms the issue of the spectral ambiguity presented in Fig. 8 (light blue and green lines). On the other hand, cases with higher SIC > 70 % are within +0.05 MPF corridor from the regression line, reaching +0.2 MPF for SIC up to 50 %.
From this we can conclude that the effect of open water on the MPD MPF is not linear and depends on whether or not the spectral ambiguity of the ice/water mixture can still be accommodated by changing the inherent ice or melt pond scattering properties during the MPD algorithm iterations: in cases of higher SIC, i.e., lower OWF, subpixel open water like, e.g., leads can be accounted for using darker sea ice with larger grains; therefore the resulting MPF is not affected, whereas in cases of higher OWF, the MPF has to be increased as well, as the boundary conditions do not allow for even darker sea ice. It has to be noted that, although the earlier versions (Zege et al., 2015; Istomina et al., 2015a, b) as well as the current MPD versions give the MPF of the pixel for the OLCI–MERIS swath data (Figs. 4 and 6) as the fraction of open water cannot be accounted for, the daily gridded product (Fig. 2) can be considered MPF of the ice fraction of the grid cell as the open water and low SICs have been removed during gridding, and only the relative MPF, i.e., MPF as a fraction of sea ice, is delivered. Marks (2015) has confirmed this by comparing it to the product by Rösel et al. (2012) and showing good correspondence in the case of the relative MODIS MPFs (Fig. 4.21 in Marks, 2015). The new three-surface class MPD version presented by Niehaus et al. (2024) shows the implementation of these considerations to advance the MPD retrieval towards an accurate open-water effect estimation using external temperature history data.
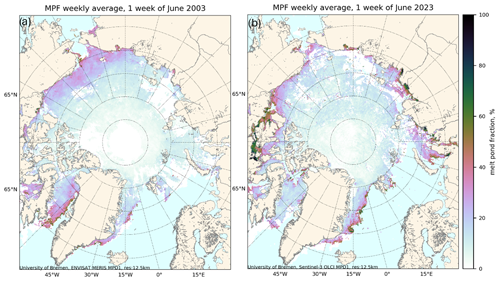
Figure 9Weekly averages for (a) the first week of June 2003, MERIS MPD, and (b) the first week of June 2023, OLCI MPD. Note the MPF differences in the Canadian Arctic Archipelago and Kara and Laptev Sea.
As moderate-resolution optical data alone are not sufficient to retrieve both SIC and MPF simultaneously (Fig. 8), we recommend using an independent SIC product for the SIC evaluation of a given grid cell. It might stem from higher-resolution optical data, meaning the open water and melting sea ice are no longer subpixel, or, depending on the required date range, PM SIC. Although PM SIC products are compromised in summer in the presence of surface melt, a recent study by Rostosky and Spreen (2023) suggests that SIC by the National Snow and Ice Data Center (NSIDC) (Meier et al., 2021) performs best, even in the presence of surface metamorphosis associated with warm-air intrusions. We therefore expect NSIDC SIC to be less affected by the water-saturated sea ice right before ponding and therefore be potentially applicable up to the pond onset. In this work, however, we preserve the earlier published procedure (Istomina et al., 2015a) and do not account for the SIC of the grid cells. We thus expect MPF overestimation connected to the open-water influence to be present and to play an increased role at the end of the melting season (depending on latitude, August–September), when cases of SIC < 70 % become spatially more frequent. Conversely, as the MPD MPF is not so much affected by SIC between 70 %–100 %, we expect good performance of the MPD MPF product in the first half of the melting season (June–July). This gradual decrease in the MPD performance throughout the melting season is also confirmed by the temporal comparison by Niehaus et al. (2024; e.g., Figs. A1–A4 therein).
To produce the MPF trends, we averaged the daily gridded MPF into a 7 d average for each pixel of the NSIDC polar stereographic grid and analyzed the resulting pan-Arctic maps for 2002–2023 (data for 2012–2016 are not available). An example of a weekly average is shown in Fig. 9 for the first week of June 2003 and 2023. In 2003, higher MPF was observed in the western Beaufort and Chukchi Sea in the beginning of the melting season, whereas in 2023 higher MPF can be seen in the Kara Sea, Laptev Sea, eastern Beaufort Sea and Canadian Arctic Archipelago (CAA). Note the very low MPF values in the high Arctic. This good performance of the MPD retrieval is observed throughout the entire dataset before the melt-associated surface darkening occurs (Fig. 10a).
The internal consistency of the combined dataset can be seen from the absence of offset, similar MPF minimum and maximum values, and similar MPF distributions between the MERIS and OLCI (Fig. 10). Here, MPF weekly averages for the 3 example weeks are shown: 1 week of May, June and July. August and September data tend to have limited spatial coverage due to higher cloud fraction during this time and are not shown. Note the very low MPF values for the first week of May, with the mean MPF < 0.1. The MPF values of 0.05 occur in the gridded product due to the misclassification of leads, as can be observed on the daily maps (Fig. 2a). The MPF data distribution for the first week of June (Fig. 10b and c) shows increasing MPF, with a stable MPF range from 0 to 1 and uniform MPF histogram. The first week of July presents further MPF increase, with the majority of the data being greater than 0.2 and reaching an MPF of 0.5 in the OLCI part of the dataset.
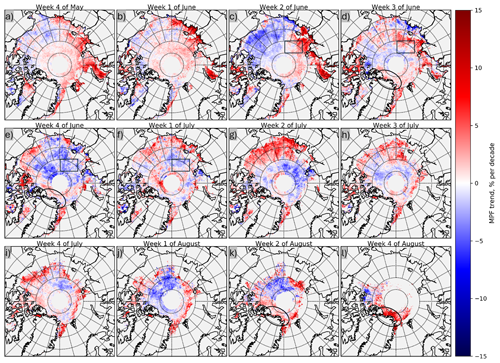
Figure 11Weekly MPF trends of the combined MERIS and OLCI dataset 2002–2023 (data 2012–2016 not available).
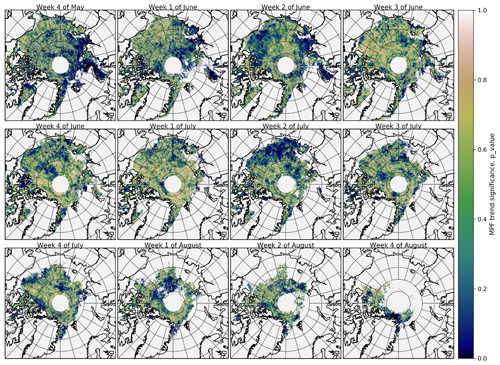
Figure 12Trend significance for the weekly MPF trends of the combined MERIS and OLCI dataset in Fig. 11.
Out of 17 years of data, we took at least 11 valid points to produce a valid trend point. As weekly MPF averages do not have complete pan-Arctic coverage with arbitrary cloud gaps equally present in both parts of the dataset, we employed this trade-off to obtain pan-Arctic coverage of the trend maps. Weekly trends from the fourth week of May till the fourth week of August (Fig. 11) were then produced via linear regression. Each week was then analyzed separately, so that seasonality was eliminated. For a given week and grid cell, the MPF distribution was assumed to be near-normal throughout the dataset. As the grid cells were processed independently of each other, spatial continuity can be used to evaluate the quality of the trend, whereas the p value (Fig. 12) is to be taken with caution given the small sample size and geophysical variability of the MPF.
4.1 Arctic MPF trend maps
The mean and maximum MPF on sea ice depends not only on the air temperature, but also on its roughness and other parameters (Polashenski et al., 2012). The Arctic sea ice consists of two main types: the FYI, featuring uniform surface with larger maximum MPFs up to 80 % being possible, and MYI, where the rougher surface prevents high MPFs, with maximum values being only up to 30 % (Untersteiner, 1986; Perovich et al., 2002; Eicken et al., 2004). These different ice types display different temporal behavior during the melt season. The FYI is going through (1) melt onset followed by onset of ponding, (2) melt maximum of up to 80 % MPF, (3) drainage, and (4) evolution of melt with the lower second MPF peak and disintegration. MYI experiences a single wide MPF peak in the middle of the melting season with little to no drainage and MPF up to 30 % (Eicken et al., 2002; Istomina et al., 2015a, and Fig. 1 therein). This dependence of MPF on the sea ice type and its surface roughness needs to be considered while analyzing the MPF trends. Similarly, with the recent change influencing the sea ice thickness (Sumata et al., 2023), the ability of sea ice to hold a certain MPF before drainage (Polashenski et al., 2017) may have been affected as well, and changes of precipitation affecting snow depth and available meltwater (Webster et al., 2014) cannot be excluded either. In the following trend discussion, all MPF trends are given in percent per decade. The optical data from OLCI or MERIS (or MODIS) are not sufficient to perform an accurate retrieval of the melt phases as described above, so we use weekly trends as in the previously published work (Istomina et al., 2015a), which also enables a comparison of these trends to each other.
A strong significant positive MPF trend reaching +20 % in the Kara and Laptev Sea can be observed, starting in the fourth week of May to the second week of June (Figs. 11a–c and 12), followed by a spatially inhomogeneous negative trend up to −10 % in the third and fourth weeks of June (Fig. 11d–e), which then turns into a positive trend of +10 % during the first and second week of July (Fig. 11f–g). We interpret this as a shift of the four FYI melt phases towards spring. The negative MPF trend occurs when the pond drainage occurs already in the week of the melt onset peak. Similarly, a melt phase temporal shift by at least 2 weeks towards spring is observed in the central Arctic, marked with the rectangle in Fig. 11c–g.
The negative trend around −6 % in the Beaufort and Chukchi Sea in the second week of June (Fig. 11c) is preceded and followed by the spatially inhomogeneous trends of +2 % (Fig. 11b and d); therefore, here a temporal shift of melt phases can also play a role. Note also the statistical significance of this trend (Fig. 12). However, as the amplitudes of these trends do not match, an actual decrease in MPF in this area is possible, e.g., due to an increase in sea ice roughness or due to lower meltwater availability via a decreased snow depth in the western Arctic (Webster et al., 2014). These factors cause the MPF to increase gradually instead of a strong melt onset peak; therefore higher MPFs do not happen till the third week of June. This assumption is supported by a spatially matching positive MPF trend of +6 % in Fig. 11g, which corresponds to the peak of the gradual MPF evolution.
The south CAA shows the temporal shift of the melt onset from the fourth week of June and the first week of July (Fig. 11e and f) to the first–third weeks of June (Fig. 11b–d), with a positive trend of +12 %. The positive MPF trend of +2 % to +6 % during the height of the melt season during the third week of July through to the second week of August (Fig. 11h–k) corresponds to the ice-type shift towards FYI in this landfast ice area. Interesting to note is that the observed MPFs are higher than the typical pack FYI MPF. The MPF evolution in 2023 shows an initial melt onset on 14 June with MPF ∼ 50 %, an MPF decrease to 30 % on 8 July, and a higher second peak of MPF ∼ 65 % on 29 July 2023 and till the ice disintegration on 15 August. For comparison, in 2018, the MPF is ∼ 40 % throughout the entire season without much variation (Istomina, 2023b).
The MYI area of north Greenland (marked with an oval, Figs. 11d, e and 11k, l) displays an inhomogeneous MPF trend of up to +6 % throughout the melt season with an increase at the end of August, which is also statistically significant (Fig. 11). This increase might indicate the ice-type shift towards FYI but also open-water influence on the MPF, meaning a complementary negative SIC trend. Both sea ice-type shift and potential negative SIC trend are indicators of younger sea ice that is more prone to break up (Maslanik et al., 2007; Gregory et al., 2022) in this typical MYI region. The MPD retrieval and MERIS–OLCI data presented in this work do not give us the possibility to retrieve sea ice type in summer due to the issues described in the previous sections' therefore future investigations are required to further clarify the observed MPF trend behavior.
The negative MPF trend between −1 % and −4 % in the central Arctic in the height of the melting season for the second–fourth weeks of July (Fig. 11g–i) can be interpreted as the ice-type shift towards FYI, where the MYI melt peak is being replaced with the FYI melt evolution phase. The FYI onset peak in the fourth week of May–third week of June with the trend +3 % (Fig. 11a–d) and the FYI drainage phase in the fourth week of June (Fig. 11e) seen as the negative MPF trend confirm this assumption. The subsequent negative MPF trend −5 % in August (Fig. 11j–k) suggests an increased role of pond drainage connected to the decreased sea ice thickness; therefore the average MPF is not as high as in the earlier years of the dataset.
It has to be noted that the displayed MPF trends are only valid under an assumption of absent cloud cover trend; i.e., irregularities of the Arctic cloud coverage throughout the combined dataset years will influence the MPF trend due to irregular representation of, e.g., different melt stages. Similarly, we attribute at least some of the positive MPF trends to the presumably decreasing summer sea ice concentration trend, as the thinner, younger Arctic sea ice would be prone to sea ice motion and lead formation (Maslanik et al., 2007; Gregory et al., 2022).
4.2 Hemispheric averaged MPF trends
The weekly hemispheric MPF trends for the combined MERIS and OLCI dataset are shown in Fig. 13, and the corresponding values of trend in percent per decade and trend baseline and the p values are given in Table 3.
Table 3Weekly hemispheric MPF trends of the combined MERIS and OLCI dataset 2002–2023 (data 2012–2016 not available).
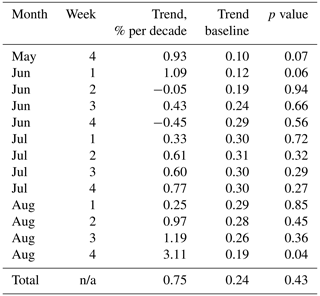
n/a: not applicable.
Despite pronounced regional MPF variability, the weekly hemispheric MPF trends are moderate in the range of +0.15 % to +3 %, except for the second and fourth weeks of June, where the negative trend can be attributed to the increased melt in the eastern Arctic and melting season shifting towards spring (Sect. 4.1). A significant hemispheric MPF trend of +1 % is observed at the end of May (fourth week) and beginning of June (first week). The last 3 weeks of July displays a consistent trend of +0.6 % to +0.7 %, which can be explained by higher MPFs during the melt evolution stage on flatter, younger ice in recent years of the dataset, as opposed to lower MPFs on rough MYI in the beginning of the dataset. The positive trends of +1 % to +3 % seen in the last 3 weeks of August are potentially connected to the change of the ice type toward FYI as well but are likely enhanced by the negative SIC trends associated with thinner sea ice being more prone to breakup. As can be seen from the regional dynamic (Fig. 11), this also stands for the MYI region of north Greenland and is statistically significant. The total hemispheric MPF trend for the entire melting season from the fourth week of May till the fourth week of August is moderate, at +0.75 % per decade.
The weekly averaged hemispheric MPF displays positive dynamic for the summer 2023 as compared to the previous years, with MPF2023 being in the top 20th percentile for 8 out of 13 weeks shown in Table 3 and displaying the highest MPF of the combined dataset for 6 weeks (third and fourth week of May and July and second and fourth week of August).
The earlier published MERIS dataset displayed positive MPF trends in the CAA and north Greenland MYI region in 2002–2011 (Istomina et al., 2015b), which are potentially caused by the loss of older, thicker sea ice (Maslanik et al., 2007; Sumata et al., 2023) after 2007. The additional OLCI data 2017–2023 suggest the sea-ice-type change towards FYI for this and other regions, in addition to an earlier melt onset where the east Arctic predominates. That is, the thinning of the Arctic sea ice as shown by Sumata et al. (2023) and Haas et al. (2008) can potentially contribute to the negative sea ice concentration trend, which in turn might affect the MPF trends presented here. Further investigations, also concerning the potential change of atmospheric and ocean circulations, which can play a role, e.g., via the Arctic Oscillation (e.g., Lim et al., 2022), are needed to further clarify the observed MPF trend variability.
Melt ponds play a key role in the energy balance of the sea ice covered Arctic Ocean during summer. In order for the summer sea ice melt to be included in the climate models, long-term remote sensing datasets are needed. In this work, we present a combined remote sensing melt pond fraction dataset produced from ENVISAT MERIS and Sentinel-3 OLCI sensors based on a physical forward model of sea ice and melt ponds. The resulting dataset starts from 2002 and is ongoing within daily operational processing. We apply the earlier published dataset for the MERIS MPD algorithm on OLCI data and update the cloud screening routine to ensure internal consistency of the combined dataset. We perform quality evaluation of the new OLCI dataset against high-resolution Sentinel-2 MSI MPFs and analyze the MPF trends from 2002–2023, omitting 2012–2016 due to no available data.
Intercomparison studies between OLCI and Sentinel-2 MSI MPF show good correspondence for the middle MPF range with an overestimation in the lower MPF range, which is connected to the presence of water-saturated snow and sea ice. Good correspondence for the very high MPF = 100 % is observed. The mean correlation coefficient from the full-resolution and daily gridded comparisons to Sentinel-2 MSI is R = 0.84, and the mean RMSD is 0.137.
As moderate-resolution VIS–NIR data alone are not sufficient for simultaneous MPF and SIC retrieval due to the spectral ambiguity between subpixel melting sea ice and open water, the open water is not accounted for in a purely optical MPF retrieval. Within MPD, it introduces an overestimation of +0.05 MPF for SIC > 70 % and up to +0.2 MPD MPF for SIC ∼ 50 %. Threshold-based and morphological filters are applied to remove lower SIC in the daily gridded product; therefore a high-quality MPF is expected for the first half of the melting season in June–July before the sea ice disintegration phase in August.
The internal conformity analysis between MERIS and OLCI datasets showed good consistency with no systematic differences in the lower and higher MPF range as well as the MPF distribution shapes. Despite the known effects of the water-saturated sea ice and leads, both of which will cause MPF overestimation, low MPF values MPF < 0.1 are consistently seen for both datasets for the exemplary first weeks of May and June of the dataset.
Analysis of the weekly MPF trend maps showed pronounced regional variability, with peak trend values between −10 % and +20 % per decade. Depending on the region, moderate weekly MPF trends are also observed in the range between −5 % and +5 % per decade.
The significant positive trend around 15 % in the Laptev and Kara Sea combined with the spatially extended negative MPF trend of −6 % in the Beaufort Gyre region in the beginning of the melting season lets us assume the melt onset regime shift in recent years, where the eastern Arctic dominates the melt onset and not the western Arctic, as in the earlier years of the dataset. The exceptionally high MPF in the Laptev Sea is confirmed with the Sentinel-2 MSI MPF for June 2021 and is also visible in the other years of the combined MPF dataset.
The observed melt onset shifted at least 2 weeks towards spring, and signs of sea-ice-type change from MYI towards FYI are observed in the central Arctic, the CAA and north Greenland. The observed regional dynamics of the MPF trend suggest that, in addition to the ice relief determining the MPF, additional parameters like sea ice permeability and thickness, precipitation, and meltwater availability need to be analyzed to fully clarify the observed regional MPF trend dynamics.
Hemispheric averaged MPF trends display positive trends +0.15 % to +3 % per decade for all weeks except for the negative trends in the second and fourth week of June, which can be partly attributed to melt stages shifting towards spring. This trend behavior is likely connected to the increased role of thinner, younger sea ice on the pan-Arctic scale in recent years.
We conclude that despite pronounced interannual variability, there is a moderate long-term increase in the Arctic MPF, with a hemispheric MPF trend of +0.75 % per decade, with the summer of 2023 advancing the positive MPF trend.
Additional studies are also needed to evaluate the effect of potential atmospheric and SIC trends on the observed MPF trends.
The OLCI MPFs for 2017 until present are available at https://seaice.uni-bremen.de/data/olci/ (Istomina, 2023b). The historic MERIS MPF dataset is available at https://data.seaice.uni-bremen.de/meris/mecosi/ (Istomina, 2023a). The Sentinel-2 melt pond fraction product is available via PANGAEA: https://doi.org/10.1594/PANGAEA.950885 (Niehaus and Spreen, 2022).
LI adapted the MPD retrieval and cloud screening routine to Sentinel-3 data, performed the comparison to the classified Sentinel-2 MSI MPF data, established Sentinel-3 operational processing, performed the new training of the cloud screening MECOSI, reprocessed the MERIS dataset, produced the updated MPF trends, and outlined the manuscript. HN performed the MPF retrieval on the Sentinel-2 MSI data. GS provided the computational infrastructure and participated in discussions of the results. All authors provided critical feedback on the manuscript and contributed to the text.
The contact author has declared that none of the authors has any competing interests.
Publisher's note: Copernicus Publications remains neutral with regard to jurisdictional claims made in the text, published maps, institutional affiliations, or any other geographical representation in this paper. While Copernicus Publications makes every effort to include appropriate place names, the final responsibility lies with the authors.
The authors express gratitude to ESA and the EU for providing ENVISAT, Sentinel-2 and Sentinel-3 data and to Brockmann Consult for providing the software packages BEAM and SNAP.
This research has been supported by the Deutsche Forschungsgemeinschaft (grant nos. 424326801 and 268020496) and EU Horizon 2020 (grant no. 640161).
The article processing charges for this open-access publication were covered by the University of Bremen.
This paper was edited by Stephen Howell and reviewed by Randall Scharien and one anonymous referee.
Ackerman, S. A., Strabala, K. I., Menzel, W. P., Frey, R. A., Moeller, C. C., and Gumley, L. E.: Discriminating clear sky from clouds with MODIS, J. Geophys. Res., 103, 32141–32157, https://doi.org/10.1029/1998JD200032, 1998.
Burkhart, J. F., Kylling, A., Schaaf, C. B., Wang, Z., Bogren, W., Storvold, R., Solbø, S., Pedersen, C. A., and Gerland, S.: Unmanned aerial system nadir reflectance and MODIS nadir BRDF-adjusted surface reflectances intercompared over Greenland, The Cryosphere, 11, 1575–1589, https://doi.org/10.5194/tc-11-1575-2017, 2017.
C3S: Summer 2023: the hottest on record, Copernicus Climate Change Services press release, 5 September 2023, Copernicus Climate Change Service, European Commission, https://climate.copernicus.eu/summer-2023-hottest-record (last access: 7 January 2025), 2023.
Ding, Y., Cheng, X., Liu, J., Hui, F., Wang, Z., and Chen S.: Retrieval of Melt Pond Fraction over Arctic Sea Ice during 2000–2019 Using an Ensemble-Based Deep Neural Network, Remote Sens.-Basel, 12, 2746, https://doi.org/10.3390/rs12172746, 2020.
Eicken, H., Krouse, H. R., Kadko, D., and Perovich, D. K.: Tracer studies of pathways and rates of meltwater transport through Arctic summer sea ice, J. Geophys. Res., 107, 8046, https://doi.org/10.1029/2000JC000583, 2002.
Eicken, H., Grenfell, T. C., Perovich, D. K., Richter-Menge, J. A., and Frey, K.: Hydraulic controls of summer Arctic pack ice albedo, J. Geophys. Res., 109, C08007, https://doi.org/10.1029/2003JC001989, 2004.
Feng, J., Zhang, Y., Cheng, Q., Wong, K., Li, Y., and Tsou, J. Y.: Effect of melt ponds fraction on sea ice anomalies in the Arctic Ocean, Int. J. Appl.Earth Obs., 98, 102297, https://doi.org/10.1016/j.jag.2021.102297, 2020.
Flocco, D., Feltham, D. L., and Turner, A. K.: Incorporation of a physically based melt pond scheme into the sea ice component of a climate model, J. Geophys. Res., 115, C08023, https://doi.org/10.1029/2009JC005569, 2010.
Fors, A. S., Divine, D. V., Doulgeris, A. P., Renner, A. H. H., and Gerland, S.: Signature of Arctic first-year ice melt pond fraction in X-band SAR imagery, The Cryosphere, 11, 755–771, https://doi.org/10.5194/tc-11-755-2017, 2017.
GCOS-200: The global observing system for climate: implementation needs, World Meteorological Organization, https://library.wmo.int/records/item/55469-the-global-observing-system-for-climate (last access: 7 January 2025), 2016.
GCOS-244: The 2022 GCOS Implementation Plan, World Meteorological Organization, https://library.wmo.int/records/item/58104-the-2022-gcos-implementation-plan-gcos-244 (last access: 7 January 2025), 2022.
Gregory, W., Stroeve, J., and Tsamados, M.: Network connectivity between the winter Arctic Oscillation and summer sea ice in CMIP6 models and observations, The Cryosphere, 16, 1653–1673, https://doi.org/10.5194/tc-16-1653-2022, 2022.
Grenfell, T. C. and Maykut, G. A.: The Optical Properties of Ice and Snow in the Arctic Basin, J. Glaciol., 18, 445–463, https://doi.org/10.1017/S002214300002112, 1977.
Haas, C., Pfaffling, A., Hendricks, S., Rabenstein, L., Etienne, J.-L., and Rigor, I.: Reduced ice thickness in Arctic Transpolar Drift favors rapid ice retreat, Geophys. Res. Lett., 35, L17501, https://doi.org/10.1029/2008GL034457, 2008.
Han, H., Im, J., Kim, M., Sim, S., Kim, J., Kim, D., and Kang, S.: Retrieval of Melt Ponds on Arctic Multiyear Sea Ice in Summer from TerraSAR-X Dual-Polarization Data Using Machine Learning Approaches: A Case Study in the Chukchi Sea with Mid-Incidence Angle Data, Remote Sens.-Basel, 8, 57, https://doi.org/10.3390/rs8010057, 2016.
Howell, S. E. L., Scharien, R. K., Landy, J., and Brady, M.: Spring melt pond fraction in the Canadian Arctic Archipelago predicted from RADARSAT-2, The Cryosphere, 14, 4675–4686, https://doi.org/10.5194/tc-14-4675-2020, 2020.
Hunke, E. C., Herbert, D. A., and Lecomte, O.: Level-ice melt ponds in the Los Alamos Sea Model, CICE, Ocean Model., 71, 26–42, https://doi.org/10.1016/j.ocemod.2012.11.008, 2013.
Huntemann, M., Heygster, G., Kaleschke, L., Krumpen, T., Mäkynen, M., and Drusch, M.: Empirical sea ice thickness retrieval during the freeze-up period from SMOS high incident angle observations, The Cryosphere, 8, 439–451, https://doi.org/10.5194/tc-8-439-2014, 2014.
Istomina, L.: Reprocessed MECOSI cloud screened MPD MPFs, Institute for Environmental Physics, University of Bremen, Bremen [data set], https://seaice.uni-bremen.de/data/meris/mecosi/ (last access: 20 August 2023), 2023a.
Istomina, L.: The OLCI MPD MPF dataset, Institute for Environmental Physics, University of Bremen, Bremen [data set], https://seaice.uni-bremen.de/data/olci/ (last access: 30 August 2023), 2023b.
Istomina, L., Nicolaus, M., and Perovich, D.: Spectral albedo of sea ice and melt ponds measured during POLARSTERN cruise ARK XXII/3 (IceArc) in 2012, PANGAEA dataset, Institute for Environmental Physics, University of Bremen, Bremen, https://doi.org/10.1594/PANGAEA.815111, 2013.
Istomina, L., Heygster, G., Huntemann, M., Schwarz, P., Birnbaum, G., Scharien, R., Polashenski, C., Perovich, D., Zege, E., Malinka, A., Prikhach, A., and Katsev, I.: Melt pond fraction and spectral sea ice albedo retrieval from MERIS data – Part 1: Validation against in situ, aerial, and ship cruise data, The Cryosphere, 9, 1551–1566, https://doi.org/10.5194/tc-9-1551-2015, 2015a.
Istomina, L., Heygster, G., Huntemann, M., Marks, H., Melsheimer, C., Zege, E., Malinka, A., Prikhach, A., and Katsev, I.: Melt pond fraction and spectral sea ice albedo retrieval from MERIS data – Part 2: Case studies and trends of sea ice albedo and melt ponds in the Arctic for years 2002–2011, The Cryosphere, 9, 1567–1578, https://doi.org/10.5194/tc-9-1567-2015, 2015b.
Istomina, L., Nicolaus, M., and Perovich, D. K.: Surface spectral albedo complementary to ROV transmittance measurements at 6 ice stations during POLARSTERN cruise ARK XXVII/3 (IceArc) in 2012, Institut für Umweltphysik, Universität Bremen, Bremen, https://doi.org/10.1594/PANGAEA.867292, 2016.
Istomina, L., Marks, H., Huntemann, M., Heygster, G., and Spreen, G.: Improved cloud detection over sea ice and snow during Arctic summer using MERIS data, Atmos. Meas. Tech., 13, 6459–6472, https://doi.org/10.5194/amt-13-6459-2020, 2020.
Istomina, L. G., von Hoyningen-Huene, W., Kokhanovsky, A. A., and Burrows, J. P.: The detection of cloud-free snow-covered areas using AATSR measurements, Atmos. Meas. Tech., 3, 1005–1017, https://doi.org/10.5194/amt-3-1005-2010, 2010.
Istomina, L. G., von Hoyningen-Huene, W., Kokhanovsky, A. A., Schultz, E., and Burrows, J. P.: Remote sensing of aerosols over snow using infrared AATSR observations, Atmos. Meas. Tech., 4, 1133–1145, https://doi.org/10.5194/amt-4-1133-2011, 2011.
Ivanova, N., Pedersen, L. T., Tonboe, R. T., Kern, S., Heygster, G., Lavergne, T., Sørensen, A., Saldo, R., Dybkjær, G., Brucker, L., and Shokr, M.: Inter-comparison and evaluation of sea ice algorithms: towards further identification of challenges and optimal approach using passive microwave observations, The Cryosphere, 9, 1797–1817, https://doi.org/10.5194/tc-9-1797-2015, 2015.
Kern, S., Lavergne, T., Notz, D., Pedersen, L. T., Tonboe, R. T., Saldo, R., and Sørensen, A. M.: Satellite passive microwave sea-ice concentration data set intercomparison: closed ice and ship-based observations, The Cryosphere, 13, 3261–3307, https://doi.org/10.5194/tc-13-3261-2019, 2019.
Kern, S., Lavergne, T., Notz, D., Pedersen, L. T., and Tonboe, R.: Satellite passive microwave sea-ice concentration data set inter-comparison for Arctic summer conditions, The Cryosphere, 14, 2469–2493, https://doi.org/10.5194/tc-14-2469-2020, 2020.
Kern, S., Lavergne, T., Pedersen, L. T., Tonboe, R. T., Bell, L., Meyer, M., and Zeigermann, L.: Satellite passive microwave sea-ice concentration data set intercomparison using Landsat data, The Cryosphere, 16, 349–378, https://doi.org/10.5194/tc-16-349-2022, 2022.
Landy, J. C., Dawson, G. J., Tsamados, M., Bushuk, M., Stroeve, J. C., Howell, S. E., Krumpen, T., Babb, D. G., Komarov, A. S., Heorton, H. D. B. S., Belter, H. J., and Aksenov, Y.: A year-round satellite sea-ice thickness record from CryoSat-2, Nature, 609, 517–522, https://doi.org/10.1038/s41586-022-05058-5, 2022.
Lavergne, T. and Down, E.: A climate data record of year-round global sea-ice drift from the EUMETSAT Ocean and Sea Ice Satellite Application Facility (OSI SAF), Earth Syst. Sci. Data, 15, 5807–5834, https://doi.org/10.5194/essd-15-5807-2023, 2023.
Lee, S., Stroeve, J., Tsamados, M., and Khan, A. L.: Machine learning approaches to retrieve pan-Arctic melt ponds from visible satellite imagery, Remote Sens. Environ., 247, 0034–4257, https://doi.org/10.1016/j.rse.2020.111919, 2020.
Li, H., Perrie, W., Li, Q., and Hou, Y.: Estimation of melt pond fractions on first year sea ice using compact polarization SAR, J. Geophys. Res.-Oceans, 122, 8145–8166, https://doi.org/10.1002/2017JC013248, 2017.
Li, Q., Zhou, C., Zheng, L., Liu, T., and Yang, X.: Monitoring evolution of melt ponds on first-year and multiyear sea ice in the Canadian Arctic Archipelago with optical satellite data, Ann. Glaciol., 61, 154–163. https://doi.org/10.1017/aog.2020.24, 2020.
Light, B., Smith, M., Perovich, D., Webster, M., Holland, M., Linhardt, F., Raphael, I., Clemens-Sewall, D., Macfarlane, A., Anhaus, P., and Bailey, D.: Arctic sea ice albedo: Spectral composition, spatial heterogeneity, and temporal evolution observed during the MOSAiC drift, Elementa: Science of the Anthropocene, 10, 000103, https://doi.org/10.1525/elementa.2021.000103, 2022.
Lim, Y.-K., Wu, D. L., Kim, K.-M., and Lee, J. N.: Impact of the Arctic oscillation from March on summertime sea ice, Environmental Research: Climate, 1, 021001, https://doi.org/10.1088/2752-5295/ac91e8, 2022.
Mäkynen, M., Haapala, J., Aulicino, G.; Balan-Sarojini, B., Balmaseda, M., Gegiuc, A., Girard-Ardhuin, F., Hendricks, S., Heygster, G., Istomina, L., Kaleschke, L., Karvonen, J., Krumpen, T., Lensu, M., Mayer, M., Parmiggiani, F., Ricker, R., Rinne, E., Schmitt, A., Similä, M., Tietsche, S., Tonboe, R., Wadhams, P., Winstrup, M., and Zuo, H.: Satellite Observations for Detecting and Forecasting Sea-Ice Conditions: A Summary of Advances Made in the SPICES Project by the EU's Horizon 2020 Programme, Remote Sens.-Basel, 12, 1214, https://doi.org/10.3390/rs12071214, 2020.
Madhavan, S., Angal, A., Dodd, J., Sun, J., and Xiong, X.: Analog and digital saturation in the MODIS reflective solar bands, Proc. SPIE, 8510, Earth Observing Systems XVII, 85101N, https://doi.org/10.1117/12.929998, 2012.
Malinka, A., Zege, E., Heygster, G., and Istomina, L.: Reflective properties of white sea ice and snow, The Cryosphere, 10, 2541–2557, https://doi.org/10.5194/tc-10-2541-2016, 2016.
Malinka, A., Zege, E., Istomina, L., Heygster, G., Spreen, G., Perovich, D., and Polashenski, C.: Reflective properties of melt ponds on sea ice, The Cryosphere, 12, 1921–1937, https://doi.org/10.5194/tc-12-1921-2018, 2018.
Marks, H.: Investigation of Algorithms to Retrieve Melt Pond Fraction on Arctic Sea Ice from Optical Satellite Observations, Masters thesis, Universität Tübingen, Tübingen, Germany, https://www.iup.uni-bremen.de/iuppage/psa/documents/2015_thesis_marks.pdf (last access: 7 January 2025), 2015.
Maslanik, J. A., Fowler, C., Stroeve, J., Drobot, S., Zwally, J., Yi, D., and Emery, W. A.: Younger, thinner Arctic ice cover: increased potential for rapid, extensive sea-ice loss, Geophys. Res. Lett., 34, L2450, https://doi.org/10.1029/2007GL032043, 2007.
Meier, W. N., Fetterer, F., Windnagel, A. K., and Stewart, S.: NOAA/NSIDC Climate Data Record of Passive Microwave Sea Ice Concentration, Version 4, NSIDC: National Snow and Ice Data Center, Boulder, Colorado, USA, [data set], https://doi.org/10.7265/efmz-2t65, 2021.
Nicolaus, M., Katlein, C., Maslanik, J., and Hendricks, S.: Changes in Arctic sea ice result in increasing light transmittance and absorption, Geophys. Res. Lett., 39, L24501, https://doi.org/10.1029/2012GL053738, 2012.
Niehaus, H. and Spreen, G.: Melt pond fraction on Arctic sea-ice from Sentinel-2 satellite optical imagery (2017–2021), PANGAEA [data set], https://doi.org/10.1594/PANGAEA.950885, 2022.
Niehaus, H. Spreen, G., Birnbaum, G., Istomina, L., Jäkel, E., Linhardt, F., Neckel, N., Fuchs, N., Nicolaus, M., Sperzel, T., Tao, R., Webster, M., and Wright, N.: Sea Ice Melt Pond Fraction Derived From Sentinel-2 Data: Along the MOSAiC Drift and Arctic-Wide, Geophys. Res. Lett., 50, e2022GL102102, https://doi.org/10.1029/2022GL102102, 2023.
Niehaus, H., Istomina, L., Nicolaus, M., Tao, R., Malinka, A., Zege, E., and Spreen, G.: Melt pond fractions on Arctic summer sea ice retrieved from Sentinel-3 satellite data with a constrained physical forward model, The Cryosphere, 18, 933–956, https://doi.org/10.5194/tc-18-933-2024, 2024.
Paţilea, C., Heygster, G., Huntemann, M., and Spreen, G.: Combined SMAP–SMOS thin sea ice thickness retrieval, The Cryosphere, 13, 675–691, https://doi.org/10.5194/tc-13-675-2019, 2019.
Peng, Z., Ding, Y., Qu, Y., Wang, M., and Li, X.: Generating a long-term spatiotemporally continuous melt pond fraction dataset for Arctic sea ice using an artificial neural network and a statistical-based temporal filter, Remote Sens.-Basel, 14, 4538, https://doi.org/10.3390/rs14184538, 2022.
Perovich, D., Meier, W., Tschudi, M., Hendricks, S., Petty, A. A., Divine, D., Farrell, S., Gerland, S., Haas, C., Kaleschke, L., Pavlova, O., Ricker, R., Tian-Kunze, X., Webster, M., and Wood, K.: Sea ice, in: Arctic report card 2020, NOAA, https://doi.org/10.25923/n170-9h57, 2020.
Perovich, D. K.: The optical properties of sea ice, CRREL Monogr 96–1, 25 pp., Hanover, N.H.: US Army Corps of Engineers, 1996.
Perovich, D. K., Richter-Menge, J. A., and Tucker, W. B.: Seasonal changes in Arctic sea-ice morphology, Ann. Glaciol., 33, 171–176, 2001.
Perovich, D. K., Grenfell, T. C., Light, B., and Hobbs, P. V.: Seasonal evolution of the albedo of multiyear Arctic sea ice, J. Geophys. Res., 107, 8044, https://doi.org/10.1029/2000JC000438, 2002.
Pohl, C., Istomina, L., Tietsche, S., Jäkel, E., Stapf, J., Spreen, G., and Heygster, G.: Broadband albedo of Arctic sea ice from MERIS optical data, The Cryosphere, 14, 165–182, https://doi.org/10.5194/tc-14-165-2020, 2020.
Polashenski, C., Perovich, D. K., and Courville, Z.: The mechanisms of sea ice melt pond formation and evolution, J. Geophys. Res., 117, C01001, https://doi.org/10.1029/2011JC007231, 2012.
Polashenski, C., Golden, K. M., Perovich, D. K., Skyllingstad, E., Arnsten, A., Stwertka, C., and Wright, N.: Percolation blockage: A process that enables melt pond formation on first year Arctic sea ice, J. Geophys. Res.-Oceans, 122, 1, 413–440, https://doi.org/10.1002/2016JC011994, 2017.
Press, W. H., Teukolsky, S. A., Vetterling, W. T., and Flannery, B. P.: Numerical recipes in C. The art of scientific computing, 2nd edn., Cambridge University Press, Cambridge, ISBN-10 0521431085, 1992.
Qin, Y., Su, J., and Wang, M.: Melt Pond Retrieval Based on the LinearPolar Algorithm Using Landsat Data, Remote Sens.-Basel, 13, 4674, https://doi.org/10.3390/rs13224674, 2021.
Ramjan, S., Geldsetzer, T., Scharien, R., and Yackel, J.: Predicting Melt Pond Fraction on Landfast Snow Covered First Year 1595 Sea Ice from Winter C-Band SAR Backscatter Utilizing Linear, Polarimetric and Texture Parameters, Remote Sens.-Basel, 10, 1603, https://doi.org/10.3390/rs10101603, 2018.
Rantanen, M., Karpechko, A. Y., Lipponen, A., Nordling, K., Hyvärinen, O., Ruosteenoja, K., Vihma T., and Laaksonen, A.: The Arctic has warmed nearly four times faster than the globe since 1979, Commun. Earth Environ., 3, 168, https://doi.org/10.1038/s43247-022-00498-3, 2022.
Ricker, R., Hendricks, S., Kaleschke, L., Tian-Kunze, X., King, J., and Haas, C.: A weekly Arctic sea-ice thickness data record from merged CryoSat-2 and SMOS satellite data, The Cryosphere, 11, 1607–1623, https://doi.org/10.5194/tc-11-1607-2017, 2017.
Rösel, A. and Kaleschke, L.: Comparison of different retrieval techniques for melt ponds on Arctic sea ice from Landsat and MODIS satellite data, Ann. Glaciol., 52, 185–191, https://doi.org/10.3189/172756411795931606, 2011.
Rösel, A., Kaleschke, L., and Birnbaum, G.: Melt ponds on Arctic sea ice determined from MODIS satellite data using an artificial neural network, The Cryosphere, 6, 431–446, https://doi.org/10.5194/tc-6-431-2012, 2012.
Rostosky, P. and Spreen, G.: Relevance of warm air intrusions for Arctic satellite sea ice concentration time series, The Cryosphere, 17, 3867–3881, https://doi.org/10.5194/tc-17-3867-2023, 2023.
Sandven, S., Spreen, G., Heygster, G., Girard-Ardhuin, F., Farrell, S., Dierking, W., and Allard, R.: Sea Ice Remote Sensing – Recent Developments in Methods and Climate Data Sets, Surv. Geophys., 44, 1653–1689, https://doi.org/10.1007/s10712-023-09781-0, 2023.
Scharien, R., Segal, R., Yackel, J., Howell, S., and Nasonova, S.: Linking winter and spring thermodynamic sea-ice states at critical scales using an object-based image analysis of Sentinel-1, Ann. Glaciol., 59, 148–162. https://doi.org/10.1017/aog.2017.43, 2018.
Schröder, D., Feltham, D. L., Flocco, D., and Tsamados, M.: September Arctic sea-ice minimum predicted by spring melt-pond fraction, Nat. Clim. Change, 4, 353–357, https://doi.org/10.1038/nclimate2203, 2014.
Spreen, G., Kaleschke, L., and Heygster, G.: Sea ice remote sensing using AMSR-E 89-GHz channels, J. Geophys. Res., 113, C02S03, https://doi.org/10.1029/2005JC003384, 2008.
Stroeve, J. and Notz, D.: Changing state of Arctic sea ice across all seasons, Environ. Res. Lett., 13, 103001, https://doi.org/10.1088/1748-9326/aade56, 2018.
Stroeve, J. C., Serreze, M. C., Holland, M. M., Kay, J. E., Malanik, J., and Barrett, A. P.: The Arctic's rapidly shrinking sea ice cover: A research synthesis, Climatic Change, 110, 1005–1027, https://doi.org/10.1007/s10584-011-0101-1, 2012.
Sumata, H., de Steur, L., Divine, D. V., Granskog, M., and Gerland, S.: Regime shift in Arctic Ocean sea ice thickness, Nature, 615, 443–449, https://doi.org/10.1038/s41586-022-05686-x, 2023.
Tanaka, Y. and Scharien, R. K.: Potential of Melt Pond Fraction Retrieval From High Spatial Resolution AMSR-E/2 Channels, IEEE Geosci. Remote S., 19, 1501405, https://doi.org/10.1109/LGRS.2020.3038888, 2022.
Tanaka, Y., Tateyama, K., Kameda, T., and Hutchings, J. K.: Estimation of melt ponds fraction over high-concentration Arctic sea ice using AMSR-E passive microwave data, J. Geophys. Res.-Oceans, 121, 7056–7072, https://doi.org/10.1002/2016JC011876, 2016.
Tomasi, C., Vitale, V., Lupi, A., Di Carmine, C., Campanelli, M., Herber, A., Treffeisen, R., Stone, R. S., Andrews, E., Sharma, S., Radionov, V., von Hoyningen-Huene, W., Stebel, K., Hansen, G. H., Myhre, C. L., Wehrli, C., Aaltonen, V., Lihavainen, H., Virkkula, A., Hillamo, R., Strom, J., Toledano, C., Cachorro, V. E., Ortiz, P., de Frutos, A. M., Blindheim, S., Frioud, M., Gausa, M., Zielinski, T., Petelski, T., and Yamanouchi, T.: Aerosols in polar regions: A historical overview based on optical depth and in situ observations, J. Geophys. Res., 112, D16205, https://doi.org/10.1029/2007JD008432, 2007.
Tschudi, M. A., Maslanik, J. A., and Perovich, D. K.: Derivation of melt pond coverage on Arctic sea ice using MODIS observations, Remote Sens. Environ., 112, 2605–2614, https://doi.org/10.1016/j.rse.2007.12.009, 2008.
Tschudi, M. A., Meier, W. N., and Stewart, J. S.: An enhancement to sea ice motion and age products at the National Snow and Ice Data Center (NSIDC), The Cryosphere, 14, 1519–1536, https://doi.org/10.5194/tc-14-1519-2020, 2020.
Tynes, H., Kattawar, G. W., Zege, E. P., Katsev, I. L., Prikhach, A. S., and Chaikovskaya, L. I.: Monte Carlo and multi-component approximation methods for vector radiative transfer by use of effective Mueller matrix calculations, Appl. Optics, 40, 400–412, 2001.
Untersteiner, N.: The Geophysics of Sea Ice, Plenum Press, NY, ISBN 0-306-42465-7, Polar Rec., 23, 729–730, https://doi.org/10.1017/S0032247400008482, 1986.
Wang, M., Su, J., Landy, J. Leppäranta, M., and Guan., L.: A new algorithm for sea ice melt pond fraction estimation from high-resolution optical satellite imagery, J. Geophys. Res.-Oceans, 125, e2019JC015716, https://doi.org/10.1029/2019JC015716, 2020.
Webster, M. A., Rigor, I. G., Nghiem, S. V., Kurtz, N. T., Farrell, S. L., Perovich, D. K., and Sturm, M.: Interdecadal changes in snow depth on Arctic sea ice, J. Geophys. Res.-Oceans, 119, 5395–5406, https://doi.org/10.1002/2014JC009985, 2014.
Webster, M. A., Rigor, I. G., Perovich, D. K., Richter-Menge, J. A., Polashenski, C. M., and Light, B.: Seasonal evolution of melt ponds on Arctic sea ice, J. Geophys. Res.-Oceans, 120, 5968–5982, https://doi.org/10.1002/2015JC011030, 2015.
Webster, M. A., Holland, M. M., Wright, N. C., Hendricks, S., Hutter, N., Itkin, P., Light, B., Linhardt, F., Perovich, D. K., Raphael, I. A., Smith, M. M., von Albedyll, L., and Zhang, J.: Spatiotemporal evolution of melt ponds in the Arctic: MOSAiC observations and model results, Elementa: Science of the Anthropocene, 10, https://doi.org/10.1525/elementa.2021.000072, 2022.
Wendisch, M., Brückner, M., Crewell, S., Ehrlich, A., Notholt, J., Lüpkes, C., Macke, A., Burrows, J. P., Rinke, A., Quaas, J., Maturilli, M., Schemann, V., Shupe, M. D., Akansu, E. F., Barrientos-Velasco, C., Bärfuss, K., Blechschmidt, A.-M., Block, K., Bougoudis, I., Bozem, H., Böckmann, C., Bracher, A., Bresson, H., Bretschneider, L., Buschmann, M., Chechin, D. G., Chylik, J., Dahlke, S., Deneke, H., Dethloff, K., Donth, T., Dorn, W., Dupuy, R., Ebell, K., Egerer, U., Engelmann, R., Eppers, O., Gerdes, R., Gierens, R., Gorodetskaya, I. V., Gottschalk, M., Griesche, H., Gryanik, V. M., Handorf, D., Harm-Altstädter, B., Hartmann, J., Hartmann, M., Heinold, B., Herber, A., Herrmann, H., Heygster, G., Höschel, I., Hofmann, Z., Hölemann, J., Hünerbein, A., Jafariserajehlou, S., Jäkel, E., Jacobi, C., Janout, M., Jansen, F., Jourdan, O., Jurányi, Z., Kalesse-Los, H., Kanzow, T., Käthner, R., Kliesch, L. L., Klingebiel, M., Knudsen, E. M., Kovács, T., Körtke, W., Krampe, D., Kretzschmar, J., Kreyling, D., Kulla, B., Kunkel, D., Lampert, A., Lauer, M., Lelli, L., von Lerber, A., Linke, O., Löhnert, U., Lonardi, M., Losa, S. N., Losch, M., Maahn, M., Mech, M., Mei, L., Mertes, S., Metzner, E., Mewes, D., Michaelis, J., Mioche, G., Moser, M., Nakoudi, K., Neggers, R., Neuber, R., Nomokonova, T., Oelker, J., Papakonstantinou-Presvelou, I., Pätzold, F., Pefanis, V., Pohl, C., van Pinxteren, M., Radovan, A., Rhein, M., Rex, M., Richter, A., Risse, N., Ritter, C., Rostosky, P., Rozanov, V. V., Ruiz Donoso, E., Saavedra-Garfias, P., Salzmann, M., Schacht, J., Schäfer, M., Schneider, J., Schnierstein, N., Seifert, P., Seo, S., Siebert, H., Soppa, M. A., Spreen, G., Stachlewska, I. S., Stapf, J., Stratmann, F., Tegen, I., Viceto, C., Voigt, C., Vountas, M., Walbröl, A., Walter, M., Wehner, B., Wex, H., Willmes, S., Zanatta, M., and Zeppenfeld, S.: Atmospheric and Surface Processes, and Feedback Mechanisms Determining Arctic Amplification: A Review of First Results and Prospects of the (AC)3 Project, B. Am. Meteorol. Soc., 104, E208–E242, https://doi.org/10.1175/BAMS-D-21-0218.1, 2023.
Wiebe, H., Heygster, G., Zege, E., Aoki, T., and Hori, M.: Snow grain size retrieval SGSP from optical satellite data: Validation with ground measurements and detection of snow fall events, Remote Sens. Environ., 128, 11–20, https://doi.org/10.1016/j.rse.2012.09.007, 2013.
WMO: State of the Global Climate 2022, World Meteorological Organization, Geneva, Switzerland, WMO-No. 1316, ISBN 978-92-63-11316-0, 2023.
Wright, N. C. and Polashenski, C. M.: Open-source algorithm for detecting sea ice surface features in high-resolution optical imagery, The Cryosphere, 12, 1307–1329, https://doi.org/10.5194/tc-12-1307-2018, 2018.
Yackel, J. J., Barber, D. G., and Hanesiak, J. M.: Melt ponds on sea ice in the Canadian Archipelago: 1. Variability in morphological and radiative properties, J. Geophys. Res., 105, 22049–22060, 2000.
Zege, E. P., Malinka, A. V., Katsev, I. L., Prikhach, A. S., Heygster, G., Istomina, L. G., Birnbaum, G., and Schwarz, P.: Algorithm to retrieve the melt pond fraction and the spectral albedo of Arctic summer ice from satellite data, Remote Sens. Environ., 163, 153–164, https://doi.org/10.1016/j.rse.2015.03.012, 2015.
Zhang., J., Schweiger, A., Webster, M., Light, B., Steele, M., Ashjian, C., Campbell, R., and Spitz, Y.: Melt Pond Conditions on Declining Arctic Sea Ice Over 1979–2016: Model Development, Validation, and Results, J. Geophys. Res.-Oceans, 123, 7983–8003, https://doi.org/10.1029/2018JC014298, 2018.