the Creative Commons Attribution 4.0 License.
the Creative Commons Attribution 4.0 License.
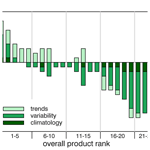
Benchmarking of snow water equivalent (SWE) products based on outcomes of the SnowPEx+ Intercomparison Project
Colleen Mortimer
Chris Derksen
Aleksandra Elias Chereque
Paul Kushner
We assess and rank 23 gridded snow water equivalent (SWE) products by implementing a novel evaluation strategy using a new suite of reference data from two cross-validated sources and a series of product intercomparisons. The new reference data combine in situ measurements from both snow courses and airborne gamma measurements. Compared to previous evaluations of gridded products, we have substantially increased the spatial coverage and sample size across North America, and we are able to evaluate product performance across both mountainous and nonmountainous regions. The evaluation strategy we use ranks overall relative product performance while still accounting for individual differences in the ability to represent SWE climatology, variability, and trends. Assessing these gridded products fills an important gap in the literature, since individual gridded products are frequently chosen without prior justification as the basis for evaluating land surface and climate model outputs, along with other climate applications. The top-performing product across the range of tests performed is ERA5-Land, followed by the Crocus snow model. Our evaluation indicates that the accurate representation of hemispheric SWE varies tremendously across the range of products. While most products are able to represent SWE reasonably well across Northern Hemisphere (NH) nonmountainous regions, the ability to accurately represent SWE in mountain regions and to accurately represent historical trends is much more variable. Finally, we demonstrate that, for the ensemble of products evaluated here, attempts to assimilate surface snow observations and/or satellite measurements lead to a deleterious influence on regional snow mass trends, which is an important consideration for how such gridded products are produced and applied in the future.
- Article
(6382 KB) - Full-text XML
-
Supplement
(2077 KB) - BibTeX
- EndNote
Historical gridded snow water equivalent (SWE) products are temporally continuous and spatially complete datasets required across many disciplines spanning climate, hydrology, and ecology (Clark et al., 2011; Dutra et al., 2011; Jones et al., 2011; Liston, 1999; Lundquist and Dettinger, 2005; Orsolini et al., 2013; Simpson et al., 2022). Numerous such products exist based on a range of techniques: output from coupled reanalysis systems, offline simulations of snow models driven by historical meteorological forcing, and satellite-based retrievals, all of which may also assimilate snow observations from surface networks or remotely sensed data. These gridded products aim to represent various aspects of historical snow conditions (e.g., areal coverage, surface snow amount, snow temperatures) and because of this are frequently used to evaluate terrestrial snow output from land surface and Earth system models (for example, Collier et al., 2018). However, the historical gridded products themselves require validation with in situ observations.
For surface snow amounts, this validation is challenging for several reasons. In situ point snow depth measurements are the most readily available and plentiful reference data; however, some gridded products already assimilate these data in the course of their production, thereby negating their use as independent reference data. Even when not incorporated into the production of a gridded product, in situ snow depths require assumptions about snow density in order to evaluate SWE and are nonideal for evaluating the spatial scale on which gridded products represent snow, which can range from roughly 102–104 km2. In place of point measurements, the use of snow courses/transects (WMO, 2018) is more appropriate. These provide information on both snow density and depth to better constrain SWE, and they also represent the snowpack on a spatial scale of roughly 0.1–1 km2, which is closer to the resolutions of the gridded products. Mortimer et al. (2020) previously used such data to evaluate a range of gridded products, but the analysis excluded complex terrain and had poor coverage across portions of North America. Along with snow transects, airborne gamma measurements can also be used to derive SWE estimates that are representative on similar spatial scales to those from snow transects (Carroll, 2001). These SWE estimates are derived by comparing the attenuation of gamma radiation due to the presence of snow with corresponding measurements conducted under snow-free conditions while accounting for changes in background soil moisture. Cho et al. (2020) used historical data of this type available over the United States to evaluate a small selection of gridded products.
Recently, Mortimer et al. (2024) cross-validated snow transect and airborne gamma SWE measurements over North America. They demonstrated broad consistency in the corresponding SWE values from the two types of measurements and consistency in the relative performance of gridded SWE products as assessed using either source of reference data. These results support combining snow course and airborne gamma measurements into a single reference dataset. The result is a new suite of reference data with an expanded spatial coverage and volume of measurements, thus greatly improving the validation domain across North America compared to prior studies.
We make extensive use of this new reference dataset, along with additional approaches to dataset intercomparisons, to produce what we consider to be the most robust and comprehensive evaluation of gridded SWE products performed to date. We evaluate 23 gridded SWE products on their ability to represent aspects of SWE climatology, variability, and trends across three segments of the snow season (snow onset, seasonal peak, and snow melt) and across regions spanning the Northern Hemisphere (NH). The breadth of evaluation criteria permits us to make recommendations on which gridded datasets are appropriate for a variety of uses.
The type of evaluation employed here shares philosophical connections to those employed by other projects, such as the International Land Model Benchmarking (ILAMB) system (Collier et al., 2018) and the Automated Model Benchmarking R (AMBER) package (Seiler et al., 2021), that aim to evaluate historical estimates of a range of land surface variables. However, ILAMB and AMBER are concerned with multiple outputs from land surface models that are evaluated using gridded data or fiducial reference measurements (which are spatially less representative of land surface model output). Our analysis is a detailed evaluation of a single variable (SWE) using both comparisons with in situ data and gridded product intercomparisons, thereby helping to inform the reference products employed in ILAMB and AMBER. By improving the temporal continuity and spatial coverage of our analysis, our ultimate goal is to provide a validation framework that would facilitate automated evaluation of forthcoming gridded SWE datasets.
The remainder of this paper is organized as follows. Section 2 provides the list of gridded SWE products we evaluate, outlines our overall evaluation strategy, and describes the specific evaluation metrics and range of reference data used in the evaluation. We illustrate product-specific performance over a range of tests in Sect. 3. In Sect. 4 we provide the overall product rankings as well as recommendations for which products may be used in what capacity and where their shortcomings exist (e.g., accurately captures spatial distribution of SWE, accurately captures seasonal snow mass trends), along with additional discussion points. We present our conclusions in Sect. 5.
2.1 Evaluated gridded SWE products
We evaluate the suite of 23 gridded SWE products listed in Table 1; the products are organized into families and described in more detail below. While some of these products are now deprecated and have been superseded by updated versions, we include them in our evaluation as they provide a baseline to indicate the improvement or deterioration of performance with subsequent versions. Additionally, by including these older product versions, our evaluation may be useful for the interpretation of previously published analyses that used such datasets.
Table 1List of all gridded SWE products evaluated.
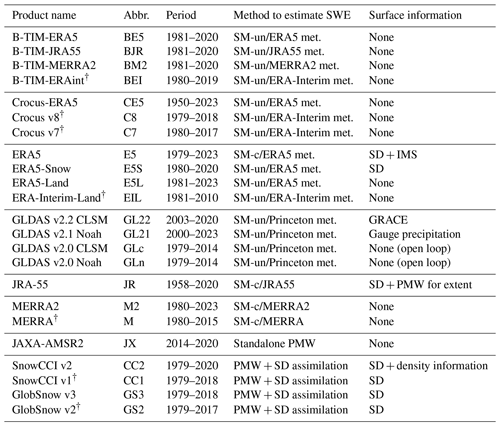
The † symbol denotes products that are deprecated or superseded by updated versions. Product availability is specified in the Data availability section. PMW refers to SWE estimated from satellite observations of passive microwave brightness temperatures. IMS refers to data from the 1 km resolution snow cover product (U.S. National Ice Center, 2008). SD refers to point snow depth information assimilated (data may vary by product, but available sources are similar overall). SM-c refers to coupled snow models driven by meteorological forcing as specified. SM-un refers to uncoupled (offline) snow models driven by meteorological forcing as specified.
The Brown Temperature Index Model (B-TIM) family of products all consist of a simple temperature index snow scheme (Brown et al., 2003; Elias Chereque et al., 2024) driven by historical estimates of temperature, precipitation, and snowfall. At present, four versions of this product exist, each driven by output from a different reanalysis. The strength of these products is that they are simple to produce, require a minimal selection of driving variables, and contain no land surface assimilation so that differences among the product versions reflect differences among the driving data. This will be a key factor that we exploit in order to analyze differences in the magnitude and seasonality of regional snow mass trends among all products (Sect. 4).
The Crocus family of products are all derived from a complex snow scheme embedded in the ISBA land model (Brun et al., 2013). The most recent version is driven by ERA5 analysis fields (temperature, precipitation, humidity, winds, etc). Two previous versions driven by fields from the now-discontinued ERA-Interim analysis are also evaluated. These two versions have similar anomalies, but differences in their parametrizations yield moderate differences in their climatologies, which affects their relative performance.
The ERA5 family of products are based on the current ECMWF reanalysis (Hersbach et al., 2020; de Rosnay et al., 2022). ERA5 denotes the standard coupled reanalysis SWE output. It uses the ERA5 land surface model (HTESSEL) forced by the ERA5 meteorological analysis fields with assimilation of in situ snow depth data as available over the entire output period and snow cover extent data from the Interactive Multisensor Snow and Ice Mapping System (IMS) at 1 km resolution (U.S. National Ice Center, 2008) from mid-2004 onwards. The assimilation of IMS data is known to produce a discontinuity in the climatological SWE field (Mortimer et al., 2020). To try and correct for this, ECMWF produced a second set of SWE output (denoted ERA5-Snow) using the same land surface model and forcing as the standard ERA5 product but without assimilation of IMS snow cover extent data (assimilation of snow depth data only). ERA5-Land denotes the standard uncoupled configuration of the ERA5 analysis (Muñoz-Sabater et al., 2021), which does not assimilate any snow-related surface data. ERA-Interim-Land is the uncoupled configuration of the previous generation of the ECMWF reanalysis (Balsamo et al., 2015) and is included as a baseline product.
The GLDAS products are uncoupled configurations of the NASA Global Land Data Assimilation System Version 2. Both GLDAS-2.0 versions (Li et al., 2018; Beaudoing and Rodell, 2019) are forced by the Princeton meteorological forcing input data but use two different land surface models, Catchment and Noah 3.6. The GLDAS v2.1 product (Beaudoing and Rodell, 2020) alters the precipitation input to the Noah land surface model by incorporating information from gauge precipitation data. The GLDAS-2.2 product (Li et al., 2020) uses the CLSM land surface model and includes data assimilation of GRACE data.
The JRA-55 (JMA, 2013), MERRA2 (GMAO, 2015), and MERRA SWE products are standard coupled output from each reanalysis center.
We also assess five gridded products that incorporate information from passive microwave brightness temperatures in order to fully or partially constrain surface SWE. The JAXA-AMSR2 product is a standalone passive microwave product that estimates SWE using a retrieval algorithm based only on time-varying microwave brightness temperatures and other time-invariant ancillary data (Kelly et al., 2019). The remaining four Earth Observation (EO) products (GlobSnow v2 and v3 and SnowCCI v1 and v2) are related, with a shared development history stemming from the original GlobSnow algorithm (Takala et al., 2011), and their SWE outputs have strong similarities to one another (hereafter we refer to them collectively as GS/CCI products). All GS/CCI products use a weighted combination of passive microwave brightness temperatures and in situ snow depth measurements to constrain SWE (Luojus et al., 2021); differences among them are detailed in (Mortimer et al., 2022).
2.2 Overall evaluation strategy
We evaluate the 23 gridded SWE products (Table 1) on their ability to represent aspects of SWE climatology, variability, and trends across 14 combinations of regions and seasons as summarized in Table 2. The choices of regions and seasons that we test are controlled in part by the reference data, as we detail in Sect. 2.3. While ideally we would use a single reference dataset applied in the same manner for all tests, the characteristics of our primary reference data (referred to in Table 2 as “combined snow course + gamma SWE”) limit the types of evaluations for which they are most appropriate. Therefore, in order to facilitate the comparison of product performance among all tests, we implement a relative point system as our overall evaluation strategy. For each combination of region and season listed in Table 2, the products that perform best on a given test are rewarded and the ones that perform the worst are penalized. Results from this reward/penalty system are tallied over all 14 evaluations, allowing us to provide total relative rankings (Sect. 4) that indicate a product's overall performance compared to the entire suite of products.
Table 2Summary of evaluations performed by region, evaluation method, and reference data used.
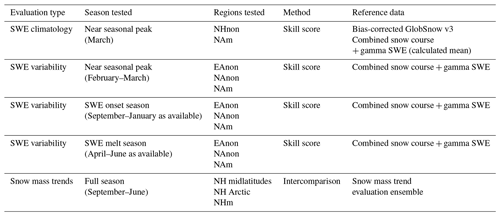
Regional abbreviations: Northern Hemisphere nonmountainous (NHnon), Northern Hemisphere mountainous (NHm), Eurasia nonmountainous (EAnon), North America nonmountainous (NAnon), and North America mountainous (NAm).
For as many tests as possible, the particular reward/penalty applied to the products is determined using a two-component skill score (the skill score itself is described in Sect. 2.4). For each product, its similarity to the specified reference data is measured in terms of this skill score, and the distribution of scores among all products on the given test is used to determine the rewards and penalties. Any products performing above the 90th percentile are awarded +1 point; any performing below the 50th percentile are penalized −1 point.
For the trend evaluations, a modified approach is required due to limited spatial coverage of in situ data with sufficiently long records. Instead, regional snow mass trends from individual products are compared to the spread among a subset of products with consistent trends (termed the “evaluation ensemble” and described in Sect. 2.3 along with the other reference data). Individual product trends for a region that generally fall within the spread of the evaluation ensemble are awarded +1 point. Products with substantial differences from the ensemble (e.g., their trends fall outside the ensemble spread throughout the entire season) receive penalties of −1 point for that region. In cases where the differences are judged to be marginal, the product is neither awarded a point nor penalized.
2.3 Reference data
2.3.1 Combined snow course and airborne gamma SWE datasets
The primary reference dataset we use for evaluation combines snow course and airborne gamma attenuation measurements as listed in the Data availability section. The data are available over the 1979–2020 period with broad spatial coverage over both North America and Eurasia (Fig. 1). While only snow course measurements are available over Eurasia, the broad coverage across North America results from the complementary availability of the two types of measurements. While the two measurement types have been used to independently evaluate gridded products locally, they have not been combined before. Mortimer et al. (2024) conducted a cross-validation of the two types of measurements. The authors demonstrated that, across North American nonmountainous terrain, both measurement types yield consistent errors when used to evaluate gridded products where overlapping measurement types are available. However, in mountainous terrain, the evaluated product errors differ according to the reference measurement type, primarily because the snow course measurements sample a larger range of SWE magnitudes and the product errors are larger for larger SWE magnitudes. Despite the differences in error magnitudes, the choice of reference data type was shown to have little impact on the relative assessment of product performance (i.e., product rankings). It is therefore possible to obtain robust relative performance measures across both mountainous and nonmountainous terrain of North America. This characteristic of the primary reference data in mountain regions is one of the reasons we implement a relative ranking system as part of our overall strategy.
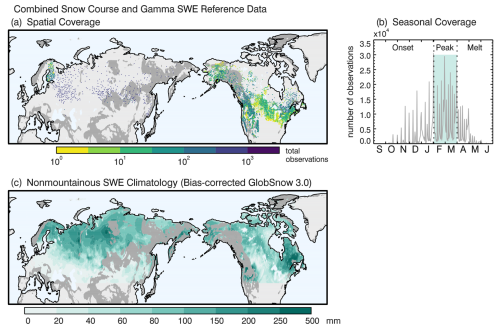
Figure 1(a) Spatial coverage of combined snow course and gamma SWE (colors show total observations available at that location over the 1979–2020 period). (b) Seasonal coverage of combined snow course and gamma reference SWE measurements. (c) March SWE climatology from bias-corrected GlobSnow version 3 (used to assess NH nonmountainous SWE climatology).
To further account for these differences in assessed errors between mountainous and nonmountainous regions, for the tests of SWE variability, we segregate the products into three distinct regions of the Northern Hemisphere: nonmountainous Eurasia (hereafter EAnon), nonmountainous North America (hereafter NAnon), and mountainous North America (hereafter NAm). While the Eurasian region contains substantial mountainous terrain, the majority of reference sites are situated in nonmountainous locations, so the evaluation results will principally reflect those characteristics. Because the temporal coverage of the data peaks during February–March (Fig. 1b), we pool all data as available during these months into a single season. Pooled data available prior to February are considered a distinct “onset” season, while pooled data available from April onwards are considered to belong to the “melt” season. For a given season, this selection of pooled data results in a sequence of SWE values that combines aspects of spatial variability (the reference data locations are at specific locations across the region), interannual variability constrained by data availability (some years will be missing at given locations), and seasonal SWE evolution (when reference data is available at multiple times within the subseason of interest).
Evaluations of mountainous climatological SWE (limited to NA) also use the combined snow course and gamma SWE. For this test, data from all years at locations with 3 or more years of data are averaged and skill scores are calculated using the resulting reference climatological values in the same manner as for the time-varying results (Sect. 2.4).
2.3.2 Bias-corrected GlobSnow v3 data
Climatological snow course and gamma SWE values at available nonmountainous locations could be used to assess gridded products similar to the way mountainous locations are used. However, bias-corrected GlobSnow v3 data (Luojus et al., 2021) represent a spatially and temporally continuous reference product that can be used to assess the gridded products across the entire nonmountainous NH (Fig. 1c). This reference product is based on the monthly mean climatology of the GlobSnow v3 product (Luojus et al., 2021) that has been bias-corrected using a subset of the snow course data discussed in Sect. 2.3.1 (NB only snow course data were used for the bias-correction; gamma attenuation SWE data were not used). Because the hemispheric coverage and sampling frequency of snow course data used to bias-correct the product are optimal during March, we limit our analysis to that month.
2.3.3 Evaluation ensemble for snow mass trends
As previously stated, because the combined reference data have a limited number of locations of sufficient length to estimate local trend values, our ability to evaluate gridded product trends with that data is also limited. Instead, we compare the consistency in the seasonal evolution of regional snow mass trends among the gridded products. By examining trends of regional snow mass (local SWE amounts summed over a given area), we effectively average out some of the small-scale differences in long-term variability and draw out the largest differences among the product trends. We focus on three non-overlapping regions previously analyzed in Mudryk et al. (2015): mountainous NH terrain, nonmountainous NH terrain south of 60° N (“midlatitudes”), and nonmountainous NH terrain north of 60° N (“Arctic”). We consider mountainous terrain separately because product performance can frequently be worse in such regions (Mortimer et al., 2024 and this study), while the separation of northern and southern regions accounts for different expectations in the historical snow response – differences in both the strength and seasonality of snow mass trends are expected between more southern and northern locations. Gridded product trends over these three regions are compared to an “evaluation ensemble” constructed from seven of the gridded reanalysis-type products: ERA5-Land, Crocus-ERA5, B-TIM-ERA5, B-TIM-JRA55, MERRA, B-TIM-ERAint, and Crocus7. While these seven products represent seven different estimates of historical SWE, they are based on only four different estimates of historical meteorological conditions: those from ERA5, ERA-Interim, MERRA, and JRA-55. Our ansatz for constructing this ensemble is that, while different snow models may alter the background SWE climatology, in the absence of land surface assimilation, it is the forcing meteorology, principally the historical temperature and precipitation estimates, that controls the interannual SWE variability and thereby the seasonal evolution of trends (see Fig. 12 in Mudryk et al., 2015, for evidence consistent with this assumption). Therefore, to construct the evaluation ensemble, we average together any products that are based on the same historical meteorological conditions. Doing so averages the three products that use ERA5 forcings (ERA5-Land, Crocus-ERA5, B-TIM-ERA5) into a single anomaly field and the two products that use ERA-Interim forcings (B-TIM-ERAint and Crocus7) into a second anomaly field. These two anomaly fields, together with those from B-TIM-JRA55 and MERRA, produce four estimates of historical SWE anomalies distinguished by choice of forcing data. We compute regional snow mass trends for each of these four anomaly fields and use the spread among the four members to determine consistency with snow mass trends from other gridded products in Sect. 3.3. We note that, while the seven products chosen may initially seem subjective, we are able to retrospectively justify the choices using the comparisons presented in Sect. 3.3.
2.3.4 Independence of reference data and evaluated gridded products
While the majority of the gridded products evaluated here are completely independent from all the reference data discussed above, we discuss a few exceptions here. Firstly, it is evident that the standard GlobSnow v3 product is not independent of the bias-corrected version used to assess product climatologies across NHnon. Furthermore, given that the four GS/CCI products have a shared development history with strong similarities to one another, in the evaluation of NHnon climatological SWE, we do not rank these four products but only use them to guide the interpretation of how well the remaining products perform. We also point out that, while the GS/CCI products, along with ERA5 and ERA5-Snow, assimilate available weather station snow depths across both NH continents, these assimilated measurements differ in both measurement frequency (sampled approximately daily versus once or twice monthly) and representative scale (being point measurements versus transects) from the snow course SWE measurements in the combined reference data. Therefore, the aforementioned gridded products are explicitly independent of the reference data. SnowCCI v2 is an exception to this statement as, in addition to in situ snow depth measurements, it also incorporates extrapolated snow-course-derived snow density information (Venäläinen et al., 2021) within the SWE retrievals. Thus, it is not completely independent of the combined reference dataset.
2.4 Skill scores and target diagrams
We use skill target diagrams, adapted from Jolliff et al. (2009), in order to rank the similarity of the gridded products to the reference data using a normalized two-component distance measure,
The first component, Spattern, measures the product's ability to match the pattern of the reference data, and the second, Sbias, measures its bias relative to the reference data. When added in quadrature, the two components describe the total distance from the reference data. Akin to the bulls-eye of a shooting target, the closer the squared distance of the independent error measures is to zero, the lower the total error.
The calculation of these two components requires three independent statistics: the product bias b (mean difference from the reference data), the product correlation with the reference data R, and the ratio of product standard deviation (sometimes referred to as the amplitude) to that of the reference data . Note that the latter two statistics are related to one another through the normalized unbiased root-mean-square error, uRMSE∗, as
Equation (2) is the standard relationship used to relate σ∗ and R on a Taylor diagram (Taylor, 2001) measuring the unbiased RMSE in units of the reference data standard deviation. Skill target diagrams provide improved rankings compared to Taylor diagrams in two ways. Firstly, they account for product errors in bias which are not represented on a Taylor diagram. Secondly, they use a skill score that more appropriately weights the pattern correlation and amplitude compared to uRMSE, which otherwise preferentially ranks low-amplitude patterns above high-amplitude patterns given comparable correlations.
The first component of Eq. (1) combines the product's errors in amplitude and correlation as
The bracketed part of this formula is a standardly employed skill score ranging from 0 to 1 that can be used in place of uRMSE to better weight errors in amplitude and correlation (see, e.g., Taylor, 2001). As in Jolliff et al. (2009), values approaching zero indicate superior skill (a reversal of the typical convention, used here so that the score measures distance from the origin). The scaling factor, , is the ratio of the maximum uRMSE value among the gridded products on the test in question to the maximum uRMSE value among all tests. This factor is applied only to make it easier to compare how the gridded product performance varies from one test to another. The second component of Eq. (1) measures the errors in bias as
where uRMSEmax represents the maximum uRMSE among the ensemble of products evaluated (in absolute rather than normalized units). Our formulation differs from Jolliff et al. (2009), who use , where bmax is the maximum bias in the product ensemble. We argue that scaling by bmax can overweight the contribution of bias to the total skill distance, Stotal, whereas normalizing by a measure of the ensemble uRMSE accounts for the proportion of the total RMSE contributed by the bias, since and RMSE2 = uRMSE2 + b2. If bmax ∼ uRMSEmax, then Spattern and Sbias will contribute equally to Stotal, since there will be an ensemble member for which Sbias ∼ Spattern. However, if bmax ≪ uRMSEmax, the total skill distance should be determined principally by Spattern, which is the case as formulated here but not as formulated in Jolliff et al. (2009). The same scaling factor, f, is also applied to Sbias to better compare performance among all tests. Because the factor is applied to both skill score components of all products, it does not influence the relative rankings on a given test, only the perceived performance on the given test relative to the other tests.
Computing the combined skill distance described above requires three input statistics: bias, correlation, and standard deviation. These were calculated for each product as follows. For the tests of SWE variability (all regions/terrain/seasons) and SWE climatology (mountainous NA), the combined snow course and gamma reference data are matched up in time and space at the native resolution of each product for mountainous and nonmountainous locations separately, as detailed in Mortimer et al. (2024). In brief, the reference data for a specific terrain type are first averaged at the resolution of each product, thereby obtaining paired reference-product SWE values, and then the paired values are averaged within a search radius of 100 km. The first step limits the weight given to specific grid cells having multiple coincident observations on the same date compared to those with only one observation. The second step limits sampling differences related to gridded product resolution. For the climatological test, the final sequence of pairs is only for March and varies only by location; for the time-varying tests, the sequence varies by both date and location according to when and where reference data exist over the 1979–2020 period. For the nonmountainous climatology test, the reference data themselves are gridded, so we obtain paired values by regridding both the reference product and test products to a common 0.5° × 0.5° regular grid and weight the values by the cosine of latitude. All the procedures detailed above result in a sequence of N paired SWE samples (reference data samples are denoted as ri, and product data samples are denoted as xi) from which we calculate
3.1 Climatological SWE evaluations
Before presenting the performance of individual gridded products on the series of tests described in Sect. 2.2, we first illustrate how the spread in climatological snow mass across both mountainous and nonmountainous regions of the NH varies among the products. To do this, we sort the products into four groups. The first group we consider consists of five previous generation reanalysis-derived products (now deprecated): ERA-Interim, B-TIM-ERAint, Crocus7, Crocus8, and MERRA (denoted “Reanalysis Group 1” in Fig. 2). For comparison, in the second group (“Reanalysis Group 2”), we consider gridded SWE products based on the current generation of reanalyses: ERA5, ERA5-Snow, ERA5-Land, Crocus-ERA5, MERRA2, B-TIM-ERA5, B-TIM-MERRA2, and B-TIM-JRA55. The third group contains the GS/CCI (EO) products and the JAXA EO product (shown separately in Fig. 2). The four GLDAS products are also plotted individually, as they have large biases as illustrated in the figure and also as analyzed in the subsequent tests below.
Figure 2 illustrates that snow mass across nonmountainous terrain has, on average, increased in the current generation of reanalysis-based products from the versions analyzed in Mudryk et al. (2015). The updated products agree better both with one another and with nonmountainous snow mass aggregated from the bias-corrected GlobSnow version 3 SWE reference data (Sect. 2.3.2). Non-bias-corrected GS/CCI products have lower snow mass on average during March than the current generation of reanalysis-derived products do. Across mountain regions, the spread and mean values have increased among the newer reanalysis-type products. These increases are due to deeper SWE conditions in the Crocus-ERA5 and ERA5-Land products specifically, whereas the remaining Group 2 products have a similar range of snow mass estimates to the Group 1 products (not shown). JAXA is the only EO product that attempts to estimate SWE in mountain regions but estimates unrealistically low snow mass compared to that found in any of the reanalysis-type products other than the GLDAS products. Figure 2 also illustrates climatological snow mass from the four GLDAS products. GLDAS v2.0 output from either land model (Noah or CLSM) has unreasonably low snow mass across both nonmountainous and mountainous regions. Even if data assimilation is used as for the GLDAS v2.2 output using CLSM, the nonmountainous snow mass remains unreasonably low. However, GLDAS v2.1 using Noah (Fig. 2, dark-green cross), which replaces the Princeton precipitation forcing used for all other versions with the gauge-based GPCP v1.3 precipitation product, has snow mass that is much more consistent with the other products.
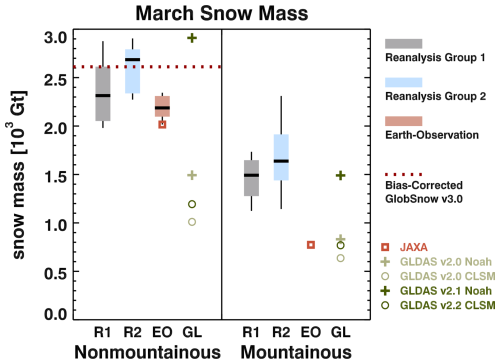
Figure 2Nonmountainous and mountainous March snow mass for various groupings of products. Heavy black lines show the median snow mass within the group, shading shows the interquartile range, and vertical lines show the entire range of snow mass for the group. JAXA and GLDAS products are considered separately, as denoted by the symbols.
In Fig. 3 we examine the relative ability of products to capture the correct spatial distribution of climatological SWE across both nonmountainous and mountainous terrain. Products are evaluated using skill target diagrams (after Jolliff et al., 2009; see Sect. 2 for details) with Taylor diagrams also shown for reference. Figure 3 illustrates that, when assessed using a Taylor diagram, roughly half the products have minimal spread in their skill at reproducing the correct spatial distribution of climatological SWE in nonmountainous regions and perform nearly as well as the GS/CCI products (red squares), which are shown on the plot but are not ranked due to their similarity to the bias-corrected GlobSnow version 3 reference data (see Sect. 2.3.4). More discernment among the products is apparent on the target diagram, which illustrates that ERA-Interim-Land, JAXA, JRA55, and three of the four GLDAS products are in the lower half of the product distribution and that, among the remaining products, there is a range of positive and negative biases. Note that using the total skill distance (target diagram) yields different rankings from when uRMSE errors are used (Taylor diagram). This difference is especially important in mountainous regions where the products' ability to capture the variance in the climatological SWE distribution varies dramatically. As highlighted in Sect. 2.4, the fact that essentially all products underestimate the spatial variability in climatological SWE compared to the reference data affects the uRMSE-based rankings. In particular, despite having both modestly improved correlation and substantially improved spatial variability compared to the reference data, both Crocus-ERA5 and ERA5-Land have higher uRMSE values in mountainous regions than several of the other products (Fig. 3, upper right, where they are ranked third and seventh, respectively). When ranked by their total skill distances instead (Fig. 3, lower right), these are the two best-performing products in mountain regions, performing above the 90th percentile among the range of products. We also note that mean bias forms a larger fraction of the total mean error in mountainous regions compared to nonmountainous regions (they contribute roughly equally in mountain regions, whereas, in nonmountainous regions, bias is typically less than half the value of uRMSE). For these reasons, we use only the skill target diagrams in the subsequent analysis and the combined skill score to rank the products.
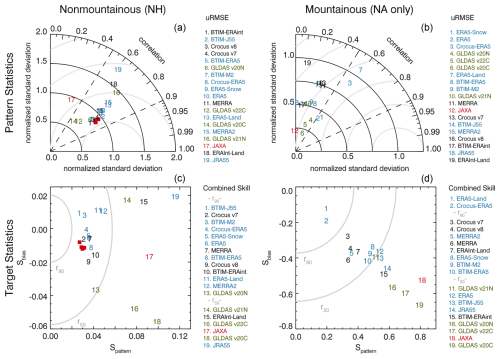
Figure 3Taylor plots (a, b) illustrate performance ranked by uRMSE (distance from reference data measured in units of the standard deviation and shown by the concentric gray circles) in nonmountainous (a, c) and mountainous (b, d) regions. Target plots (c, d) illustrate performance ranked by total skill distance (skill scores of zero represent no difference from the reference data in terms of pattern statistics or mean bias). Gray curves indicate the 90th and 50th percentiles. Red squares denote the performance of the GS/CCI products which are considered “close” to the reference data in nonmountainous regions. Colors reflect the groupings from Fig. 1.
3.2 Time-varying SWE evaluations
The next series of tests evaluates the gridded products on their ability to capture time-varying SWE during three portions of the seasonal cycle. We initially examine performance near the seasonal peak (February–March). Before presenting the overall skill rankings for this evaluation, we first examine separate rankings of uRMSE, correlation, and bias to provide a sense of how they relate to one another. Figure 4 illustrates performance across nonmountainous terrain in North America compared to nonmountainous terrain in Eurasia. In general, products have poorer performance over North America than over Eurasia. This may occur because the range of reference SWE sampled is higher in North America, and this is a strong control on product bias and RMSE (see Mortimer et al., 2024). Product performances evaluated by either uRMSE or correlation are similar to one another: product rankings 1–6, 7–14, 15–20, and 21–23 all contain the same subsets of products when evaluated using uRMSE or correlation. In contrast, bias is a poor discriminant of product performance in nonmountainous terrain. Products may have low bias but high uRMSE and low correlation due to poor representation of SWE anomalies (JAXA, JRA55).
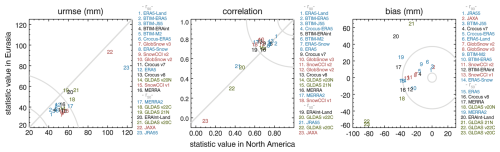
Figure 4Product-wise performance for peak SWE in North America versus Eurasia evaluated over nonmountainous regions. Products are ranked based on their North American and Eurasian statistics added in quadrature. Gray curves denote the 90th and 50th percentiles of the product distributions; these two percentiles are listed among the ranked products where they occur.
For this reason, in Fig. 5, we employ the same target plots as presented for climatological snow mass and which account for combined errors of bias, uRMSE, and correlation. Consistent with Figs. 4 and S1, the latter of which shows results for uRMSE, bias, and correlation metrics over mountainous terrain, the combined skill distance in Fig. 5 illustrates that product performance is generally best over nonmountainous Eurasia, worse over North American nonmountainous terrain, and worse again over North American mountainous terrain. Across Eurasia, no product substantially outperforms another (none are above the 90th percentile), although most of the worst-performing products also fall below the 50th percentile across all three combinations of continent and terrain (JAXA, JRA55, and two of the four GLDAS product versions). ERA5-Land and Crocus-ERA5 display the greatest skill in North American mountainous terrain and have good to excellent performance in nonmountainous regions of Eurasia and North America, as well. While the B-TIM suite of products are typically top performers in nonmountainous North America, they perform more modestly across North American mountainous regions. The GS/CCI products have good performance across Eurasia, but their performance is poorer across North America. As seen for climatological SWE (Fig. 3), in mountainous terrain, product bias is more strongly associated with overall performance than in nonmountainous terrain (see also Fig. S1).
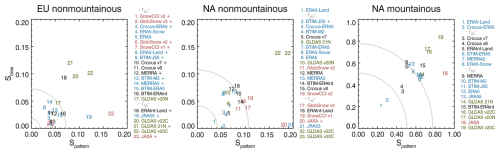
Figure 5Target plots based on statistics for peak SWE from temporally and spatially varying data for available continents and regions. Products with positive biases have a “+” symbol appended to their label, and negative biases are unmarked. Products are ranked based on total skill distance (skill scores of zero represent no difference from the reference data in terms of pattern statistics or mean bias). Gray curves denote the 90th and 50th percentiles of the product distributions; these two percentiles are listed among the ranked products where they occur.
In Fig. 6, we examine if the product-wise performance analyzed in Figs. 4 and 5 near seasonal peak SWE (February–March) remains consistent during the onset and melt seasons. Figure 6 illustrates that the product accuracy tends to worsen as the snow season progresses: on average, both the bias and pattern skill decrease, corresponding to increasing uRMSE, decreasing correlation, and increasing magnitude of bias. However, the products that have better performance when evaluated near seasonal peak SWE (when the most reference data are available, thereby yielding more accurate statistics) tend to have better performance during the onset and melt seasons. In particular, the pattern skill component assessed during peak season is also a reasonable indicator of performance during both onset and melt. In contrast, the evolution of seasonal bias can change substantially among the products, especially in nonmountainous regions.
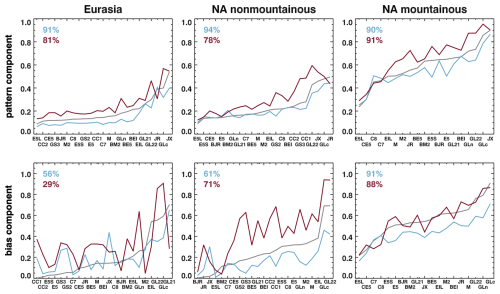
Figure 6Seasonal evolution of skill components by continent and region. Products ranked by February–March performance (gray, x-axis labels) with corresponding performance shown during onset (blue, September–January as available) and melt (red, April–June as available) seasons. Numbers displayed in corners show the percentage of onset and melt performance explained by corresponding performance during February–March.
3.3 Trend evaluations
Finally, we evaluate differences in product trends using the quantitative intercomparison approach described in Sect. 2.2. All results are summarized in Fig. 7. The first four rows are separated according to the forcing meteorology used to create the reanalysis-type products; the EO products are shown in the last row.
The first row demonstrates one of our key results, that the assimilation of surface snow or satellite information can often have a deleterious effect on product trends. It illustrates the different seasonal evolution of snow mass trends from JRA55 and B-TIM-JRA55. The two products use the same forcing meteorology but differ in their snow schemes and in whether or not they assimilate in situ snow depth measurements and passive-microwave-derived information on snow presence: B-TIM-JRA55 does not, but JRA55 does (Kobayashi et al., 2015). We argue it is unlikely that the differences in trend magnitudes and timing shown are due to differences in the snow scheme employed, nor can they be due to differences in meteorological forcing. This suggests that fluctuations in the availability of in situ snow depth measurements and/or regional and seasonal variability in the ability to detect snow presence using passive microwave information could be causing the anomalous trends, particularly in mountain and midlatitude regions. JRA55 trends in Arctic regions still have anomalous signals in their seasonal evolution; however, we assess the agreement in that region as marginal (resulting in a score of 0 instead of −1).
Next, we demonstrate the same existence of spurious trends in products related to ERA5 that assimilate surface information (second row of Fig. 7). We first note an absence of spurious trend signals in the ERA5-Land, Crocus-ERA5, and B-TIM-ERA5 products, which do not assimilate land surface information and whose average is one of the components in the evaluation ensemble. By contrast, the standard ERA5 SWE output is known to contain an abrupt drop in climatological SWE coincident with its assimilation of IMS data from 2004 onwards (Mortimer et al., 2020; Ochi et al., 2023). This discontinuity results in trend variability that is seasonally coherent with the other products but at a much more negative background trend magnitude across all three regions. ERA5-Snow is an “offline” product which was forced by ERA5 analysis fields in an uncoupled configuration. It was produced to allow the assimilation of weather station snow depth information but to avoid the abrupt incorporation of IMS information from 2004 onwards. While ERA5-Snow trends have better agreement with the evaluation ensemble than ERA5, they are still more strongly negative over Arctic regions. We assess this level of disagreement as marginal in comparison to that shown by ERA5 trends in all three regions.
The third row of Fig. 7 compares snow mass trends from the original MERRA reanalysis output with those from the updated MERRA2 product and the B-TIM snow scheme forced by MERRA2 temperature and snowfall. None of the products assimilate surface snow or satellite information, so the differences illustrated result from other factors. B-TIM-MERRA2 and MERRA2 trends have similar timing and magnitudes, but, across midlatitude and mountainous regions, their magnitude is much weaker than those from the evaluation ensemble. The fact that B-TIM-MERRA2, which is driven by the same temperature and precipitation forcing as MERRA2, has similar snow mass trends to those from the MERRA2 reanalysis output suggests that the temperature or precipitation forcing or both are inconsistent with the meteorological forcing used by the other products in the evaluation ensemble across mountainous and midlatitude regions (but consistent over the Arctic).
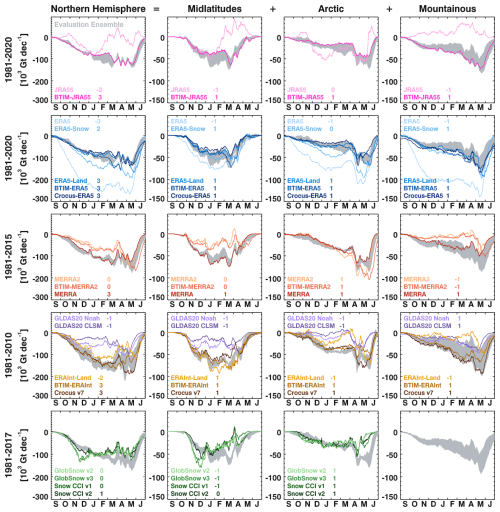
Figure 7Evaluation of snow mass trends grouped by region/terrain (columns) and the meteorological forcing data used to create them (rows), with the EO products in the bottom row. Gray shading shows the spread across the evaluation ensemble (see Sect. 2.3.3). For each row, trends are calculated over the period denoted on the left, chosen based on the period during which the plotted products are available; therefore the gray shading denoting the evaluation ensemble spread differs somewhat among the rows. Numbers denote trend scores for each region (columns 2–4) and cumulative totals for the NH (column 1) based on arguments presented in the text.
The fourth row of Fig. 7 compares trends from two separate forcing groups, the two GLDAS 2.0 products, and the three ERA-Interim-forced products (ERA-Interim-Land, Crocus7, and B-TIM-ERAint). The ERA-Interim products are consistent over midlatitude regions, but ERA-Interim-Land has inconsistencies over Arctic and mountainous regions, where its trends are weaker than those of the evaluation ensemble. The two GLDAS products are marginally consistent with the evaluation ensemble over mountainous regions but have overly weak trends in midlatitude and Arctic regions.
Finally, the bottom row of Fig. 7 compares the evaluation ensemble with trends from the four GS/CCI products (the JAXA product does not have enough years available to calculate trends). Overall, the GS/CCI products are consistent with the evaluation ensemble across the Arctic but inconsistent over midlatitude regions. Because the GS/CCI products do not provide SWE estimates in mountainous regions, we use mean anomalies from the evaluation ensemble in those regions to determine total NH trends, which allows us to observe how differences in the midlatitudes and Arctic regions combine hemispherically. The weak Arctic trends apparent in the GS/CCI products during May and June are likely related to the reduced availability of weather station snow depths during this time of the year (assimilated as part of the satellite product retrieval algorithms) combined with reduced satellite algorithm performance once the snowpack begins to melt (Mortimer et al., 2022). A similar weakening of trends is also apparent over midlatitude regions from March onwards. The three earlier GS/CCI product versions also show stronger midlatitude trends than the evaluation ensemble during snow onset (most prominent in November and December). This difference has been reduced in the most recent SnowCCIv2 product. In Fig. S3, we connect this difference across the midlatitude region to temporal discontinuities in the early and late parts of the record that have been improved but not eliminated in the most recent product. We also note that additional improvements to the snow masking (Zschenderlein et al., 2023) feeding into successor versions of SnowCCI (e.g., the forthcoming version 3 SWE product) further improve the agreement with the evaluation ensemble not only across midlatitudes but also over Arctic regions during snow onset (November to January; Kari Luojus, personal communication, 2024)
Figure 8 shows the complete list of hemispheric products organized by overall performance. The overall product rank is determined by a product's cumulative score on all tests divided by the number of tests on which it was evaluated. This allows the assessment to be agnostic about products whose performance in a particular test was unable to be evaluated. For example, JAXA, GLDAS v2.1, and GLDAS v2.2 did not have enough available years of data to calculate trends, while the GS/CCI products are not available across mountainous regions and so are untested there. For comparison, we also provide a second set of rankings that only reflects the tests that use skill scores (and thereby excludes the trend intercomparison assessment). The products with the best and worst performance are ranked similarly in these two sets of rankings; however, the positions of products with average performance (ranks 4–16) are influenced by the trend intercomparison. While we believe the trend intercomparison provides additional information by which these products can be compared, we leave it to readers to determine for themselves how they wish to consider this additional information.
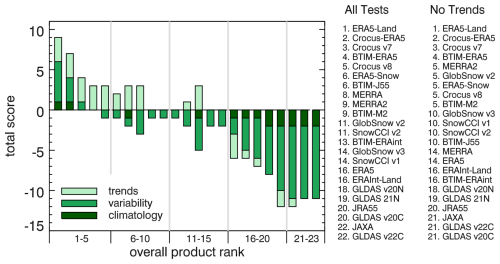
Figure 8Ranked overall performance based on all tests (x axis) broken down by category: climatology (dark), variability (medium), and trends (light). The first ranked list is based on all test categories; the second ranked list excludes the trend evaluation.
The top-performing SWE product is ERA5-Land, followed by two versions of the Crocus model (versions using forcing data from both the previous ECMWF ERA-Interim reanalysis and the updated ERA5 reanalysis). These products benefit from a comparatively high horizontal resolution in the case of ERA-Land (10 km) or from a high vertical resolution in the case of the Crocus snow model (up to 50 layers of snow can be modeled, allowing complex stratigraphy). This may be a reason for their strong performance, especially in the highly variable SWE of the North American mountain regions. These products also benefit from the absence of surface snow assimilation which negatively impacts snow mass trends of other products.
The B-TIM suite of products, which are based on a simple temperature index scheme, generally have good performance in nonmountainous regions, where they are consistently in the top half of the rankings, indicating that these simple products have value across nonmountainous areas (Figs. 3 and 5). Furthermore, the trend intercomparison (Fig. 7) suggests that they are also a valuable tool for detecting anomalous SWE trends in other products, at least on a regionally aggregated basis.
In general, the GLDAS products perform poorly when evaluated hemispherically (Fig. 5) due in part to large biases (Figs. 2 and 4). However, GLDAS v2.1, which uses different precipitation forcing than the other three versions, performs better when evaluated over nonmountainous North America (Fig. 5). Thus, while it is tempting to extrapolate regional performance, this product provides a good counter-example where doing so is particularly detrimental.
The GS/CCI products have better performance over Eurasia than North America (Fig. 5). This is a well-documented result (Luojus et al., 2021; Mortimer et al., 2020, 2022). Part of the explanation may be that the nonmountainous reference SWE has higher median values over North America (approx. 15 mm higher), which could alter the performance of the GS/CCI products, since their algorithms' SWE retrievals tend to saturate above 150 mm. However, previous analysis that restricted the reference data to under ∼ 150 to 200 mm (Luojus et al., 2021; Mortimer et al., 2020, 2022) still reported comparatively larger errors in North America. The retrieval performance in these products is also known to decrease with distance from the nearest assimilated snow depth measurement (Fig. 8 in Luojus et al., 2021). Hence it is also possible that, compared to North America, Eurasia may have more commonality in how the locations and overall coverage of the reference data align with weather station snow depth measurements assimilated by the products (see, for example, Fig. 2 in Mortimer et al., 2022). The latter is essential information for the GS/CCI algorithms to perform accurately. If locations of reference data across North American tend to be further from locations with assimilated data compared to Eurasia, this would also lower product performance. Because of these considerations, we suggest the evaluated accuracy of GS/CCI products over North America is more reflective of their true performance.
Finally, the trend analysis indicates that, for the ensemble of products evaluated here, all attempts to assimilate snow information from surface and/or satellite measurements lead to a deleterious influence on snow mass trends (e.g., ERA5 and JRA-55). The influence of the assimilation techniques employed on snow mass trends is not minor or localized but leaves clear signals even in the trends of regionally or hemispherically aggregated snow mass. While the assimilation of surface information may improve instantaneous local measures of the overall performance of a reanalysis system, it reinforces that reanalysis centers should provide multiple product streams: not only those that provide the best instantaneous estimates as needed for prediction applications but also temporally consistent historical estimates, which are needed for climate applications. In some ways, the series of GLDAS products provides a good model for this sort of treatment, with an open-loop suite of output without assimilation and another assimilated product. Unfortunately, at present, the forcing data used for the multiple GLDAS product streams differ, and there is insufficient overlap of the analysis periods to permit the attribution of differences in trends between the products to the presence or absence of data assimilation.
Finally, we point out that the relative rankings shown in Fig. 8 are meant to function as a guideline only. We stand by our results to the degree that the coverage of reference data permits such generalizations. However, for localized regions, the product performance may differ from the rankings in Fig. 8. GLDAS v2.1 provides a specific example where its performance over nonmountainous North America does not reflect its much poorer performance outside that region. Likewise, our results do not account for any idiosyncrasies in product performance in regions not covered by our reference data. The absence of reference data from mountainous regions of Europe and western Asia is one such gap. And so, while our assessment of North American mountain regions likely captures some aspects of product performance over mountainous terrain in general, we will not have captured any deficiencies that are particular to those unevaluated regions. We also acknowledge that, for some tests, the dividing line between the top and bottom 50th percentile of performance fell among closely grouped products instead of at a well-separated gap. However, the number and breadth of tests presented should help ensure that our conclusions on which products are superior performers are robust.
An expanded reference dataset (Fig. 1) consisting of snow course and airborne gamma measurements (Mortimer et al., 2024), combined with a novel evaluation strategy, allowed a comprehensive assessment of 23 gridded SWE products. The general strategy we present is easily modified to include additional products or to limit the evaluation to specific regions of interest, provided reference data are available. We adapted skill target diagrams (Jolliff et al., 2009) to rank products according to their ability to represent SWE climatology (Fig. 3), variability (Fig. 5), and trends (Fig. 7). Most products evaluated can reasonably represent the climatology and variability of nonmountainous SWE but have substantially lower skill in mountain regions (Figs. 3 and 5). The relatively poorer performance in mountain regions is consistent with previous studies (Fang et al., 2022; Kim et al., 2021; Liu et al., 2022; Snauffer et al., 2016; Terzago et al., 2017; Wrzesien et al., 2019) and points to a need for targeted mountain SWE products. For the ensemble of products evaluated, the assimilation of snow surface and/or satellite measurements has a deleterious influence on regional snow mass trends (Fig. 7). This result illustrates that products that accurately represent SWE climatology and variability may not be appropriate for trend analysis and vice versa, and it reinforces that user needs and objectives must guide product selection.
Combined reference data are available at https://doi.org/10.5281/zenodo.10287093 (Mortimer and Vionnet, 2024). The bias-corrected GlobSnow version 3 product is available at https://www.globsnow.info/swe/archive_v3.0/ (last access: 19 July 2021; DOI: https://doi.org/10.1594/PANGAEA.911944, Luojus et al., 2020b). Gridded SWE products from Table 1 are available as specified in Appendix A.
The supplement related to this article is available online at: https://doi.org/10.5194/tc-19-201-2025-supplement.
LM and CM developed the general evaluation strategy and code to calculate statistics and performed the analysis. LM, CD, PK, and AEC developed the trend intercomparison strategy. LM prepared the article with contributions from all co-authors.
At least one of the (co-)authors is a member of the editorial board of The Cryosphere. The peer-review process was guided by an independent editor, and the authors also have no other competing interests to declare.
Publisher’s note: Copernicus Publications remains neutral with regard to jurisdictional claims made in the text, published maps, institutional affiliations, or any other geographical representation in this paper. While Copernicus Publications makes every effort to include appropriate place names, the final responsibility lies with the authors.
This research has been supported by the European Space Agency (grant no. 4000111278/14/I-LG-SnowPEx CCN-2).
This paper was edited by Ruth Mottram and reviewed by Kate Hale and one anonymous referee.
Balsamo, G., Albergel, C., Beljaars, A., Boussetta, S., Brun, E., Cloke, H., Dee, D., Dutra, E., Muñoz-Sabater, J., Pappenberger, F., de Rosnay, P., Stockdale, T., and Vitart, F.: ERA-Interim/Land: a global land surface reanalysis data set, Hydrol. Earth Syst. Sci., 19, 389–407, https://doi.org/10.5194/hess-19-389-2015, 2015.
Beaudoing, H. and Rodell, M.: GLDAS Noah Land Surface Model L4 3 hourly 0.25 x 0.25 degree V2.0, Greenbelt, Maryland, USA, Goddard Earth Sciences Data and Information Services Center (GES DISC) [data set], https://doi.org/10.5067/342OHQM9AK6Q, 2019.
Beaudoing, H. and Rodell, M.: GLDAS Noah Land Surface Model L4 3 hourly 0.25 x 0.25 degree V2.1, Greenbelt, Maryland, USA, Goddard Earth Sciences Data and Information Services Center (GES DISC) [data set], https://doi.org/10.5067/E7TYRXPJKWOQ, 2020.
Brown, R. D., Brasnett, B., and Robinson, D.: Gridded North American monthly snow depth and snow water equivalent for GCM evaluation, Atmos. Ocean, 41, 1–14, https://doi.org/10.3137/ao.410101, 2003.
Brun, E., Vionnet, V., Boone, A., Decharme, B., Peings, Y., Valette, R., Karbou, F., and Morin, S.: Simulation of Northern Eurasian Local Snow Depth, Mass, and Density Using a Detailed Snowpack Model and Meteorological Reanalyses, J. Hydrometeorol., 14, 203–219, https://doi.org/10.1175/JHM-D-12-012.1, 2013.
Carroll, T. R.: Airborne Gamma Radiation Snow Survey Program: A user's guide, Version 5.0, National Operational Hydrologic Remote Sensing Center (NOHRSC), Chanhassen, https://www.nohrsc.noaa.gov/technology/pdf/tom_gamma50.pdf (last access: 13 January 2025), 2001.
Cho, E., Jacobs, J. M., and Vuyovich, C. M.: The Value of Long-Term (40 years) Airborne Gamma Radiation SWE Record for Evaluating Three Observation-Based Gridded SWE Data Sets by Seasonal Snow and Land Cover Classifications, Water Resour. Res., 56, e2019WR025813, https://doi.org/10.1029/2019WR025813, 2020.
Clark, M. P., Hendrikx, J., Slater, A. G., Kavetski, D., Anderson, B., Cullen, N. J., Kerr, T., Örn Hreinsson, E., and Woods, R. A.: Representing spatial variability of snow water equivalent in hydrologic and land-surface models: A review, Water Resour. Res., 47, W07539, https://doi.org/10.1029/2011WR010745, 2011.
Collier, N., Hoffman, F. M., Lawrence, D. M., Keppel-Aleks, G., Koven, C. D., Riley, W. J., Mu, M., and Randerson, J. T.: The International Land Model Benchmarking (ILAMB) System: Design, Theory, and Implementation, J. Adv. Model. Earth Sy., 10, 2731–2754, https://doi.org/10.1029/2018MS001354, 2018.
Decharme, B.: Crocus-ERA-Interim daily snow product over the Northern Hemisphere at 0.5° resolution, Zenodo [data set], https://doi.org/10.5281/zenodo.10911538, 2024.
Decharme, B. and Barbu, A.: Crocus-ERA5 daily snow product over the Northern Hemisphere at 0.25° resolution (Version 2023), Zenodo [data set], https://doi.org/10.5281/zenodo.10943718, 2024.
de Rosnay, P., Browne, P., de Boisséson, E., Fairbairn, D., Hirahara, Y., Ochi, K., Schepers, D., Weston, P., Zuo, H., Alonso-Balmaseda, M., Balsamo, G., Bonavita, M., Borman, N., Brown, A., Chrust, M., Dahoui, M., Chiara, G., English, S., Geer, A., Healy, S., Hersbach, H., Laloyaux, P., Magnusson, L., Massart, S., McNally, A., Pappenberger, F., and Rabier, F.: Coupled data assimilation at ECMWF: current status, challenges and future developments, Q. J. Roy. Meteor. Soc., 148, 2672–2702, https://doi.org/10.1002/qj.4330, 2022.
Dutra, E., Schär, C., Viterbo, P., and Miranda, P. M. A.: Land-atmosphere coupling associated with snow cover, Geophys. Res. Lett., 38, L15707, https://doi.org/10.1029/2011GL048435, 2011.
Elias Chereque, A.: B-TIM snow for ERA5, V1, Borealis [data set], https://doi.org/10.5683/SP3/HHIRBU, 2024a.
Elias Chereque, A.: B-TIM snow for JRA55, V1, Borealis [data set], https://doi.org/10.5683/SP3/X5QJ3P, 2024b.
Elias Chereque, A.: B-TIM snow for MERRA2, V1, Borealis [data set], https://doi.org/10.5683/SP3/C5I5HN, 2024c.
Elias Chereque, A., Kushner, P. J., Mudryk, L., Derksen, C., and Mortimer, C.: A simple snow temperature index model exposes discrepancies between reanalysis snow water equivalent products, The Cryosphere, 18, 4955–4969, https://doi.org/10.5194/tc-18-4955-2024, 2024.
Fang, Y., Liu, Y., and Margulis, S. A.: A western United States snow reanalysis dataset over the Landsat era from water years 1985 to 2021, Sci. Data, 9, 677, https://doi.org/10.1038/s41597-022-01768-7, 2022.
Global Modeling and Assimilation Office (GMAO): MERRA-2 tavg1_2d_lnd_Nx: 2d,1-Hourly,Time-Averaged,Single-Level,Assimilation,Land Surface Diagnostics V5.12.4, Goddard Earth Sciences Data and Information Services Center (GES DISC) [data set], https://doi.org/10.5067/RKPHT8KC1Y1T, 2015.
Hersbach, H., Bell, B., Berrisford, P., Hirahara, S., Horányi, A., Muñoz-Sabater, J., Nicolas, J., Peubey, C., Radu, R., Schepers, D., Simmons, A., Soci, C., Abdalla, S., Abellan, X., Balsamo, G., Bechtold, P., Biavati, G., Bidlot, J., Bonavita, M., De Chiara, G., Dahlgren, P., Dee, D., Diamantakis, M., Dragani, R., Flemming, J., Forbes, R., Fuentes, M., Geer, A., Haimberger, L., Healy, S., Hogan, R. J., Hólm, E., Janisková, M., Keeley, S., Laloyaux, P., Lopez, P., Lupu, C., Radnoti, G., De Rosnay, P., Rozum, I., Vamborg, F., Villaume, S., and Thépaut, J.-N.: The ERA5 global reanalysis, Q. J. Roy. Meteor. Soc., 146, 1999–2049, https://doi.org/10.1002/qj.3803, 2020.
Hersbach, H., Bell, B., Berrisford, P., Biavati, G., Horányi, A., Muñoz Sabater, J., Nicolas, J., Peubey, C., Radu, R., Rozum, I., Schepers, D., Simmons, A., Soci, C., Dee, D., and Thépaut, J.-N.: ERA5 hourly data on single levels from 1940 to present, Copernicus Climate Change Service (C3S) Climate Data Store (CDS) [data set], https://doi.org/10.24381/cds.adbb2d47, 2023.
Japan Meteorological Agency (JMA): JRA-55: Japanese 55-year Reanalysis, Daily 3-Hourly and 6-Hourly Data, Research Data Archive at the National Center for Atmospheric Research, Computational and Information Systems Laboratory [data set], https://doi.org/10.5065/D6HH6H41, 2013.
Jolliff, J. K., Kindle, J. C., Shulman, I., Penta, B., Friedrichs, M. A. M., Helber, R., and Arnone, R. A.: Summary diagrams for coupled hydrodynamic-ecosystem model skill assessment, J. Marine Syst., 76, 64–82, https://doi.org/10.1016/j.jmarsys.2008.05.014, 2009.
Jones, H. G., Pomeroy, J. W., Walker, D. A., and Hoham, R. W.: Snow Ecology: An Interdisciplinary Examination of Snow-Covered Ecosystems, Cambridge University Press, ISBN 9780521188890, 2011.
Kelly, R., Li, Q., and N. Saberi: 'The AMSR2 Satellite-Based Microwave Snow Algorithm (SMSA): A New Algorithm for Estimating Global Snow Accumulation, in: IGARSS 2019–2019 IEEE International Geoscience and Remote Sensing Symposium, Yokohama, Japan, 28 July–2 August 2019, 5606–5609, https://doi.org/10.1109/IGARSS.2019.8898525, 2019.
Kim, R. S., Kumar, S., Vuyovich, C., Houser, P., Lundquist, J., Mudryk, L., Durand, M., Barros, A., Kim, E. J., Forman, B. A., Gutmann, E. D., Wrzesien, M. L., Garnaud, C., Sandells, M., Marshall, H.-P., Cristea, N., Pflug, J. M., Johnston, J., Cao, Y., Mocko, D., and Wang, S.: Snow Ensemble Uncertainty Project (SEUP): quantification of snow water equivalent uncertainty across North America via ensemble land surface modeling, The Cryosphere, 15, 771–791, https://doi.org/10.5194/tc-15-771-2021, 2021.
Kobayashi, S., Ota, Y., Harada, Y., Ebita, A., Moriya, M., Onoda, H., Onogi, K., Kamahori, H., Kobayashi, C., Endo, H., Miyaoka, K., and Takahashi, K.: The JRA-55 Reanalysis: General Specifications and Basic Characteristics, J. Meteorol. Soc. Jpn. Ser. II, 93, 5–48, https://doi.org/10.2151/jmsj.2015-001, 2015.
Li, B., Beaudoing, H., and Rodell, M.: GLDAS Catchment Land Surface Model L4 daily 0.25 x 0.25 degree V2.0, Greenbelt, Maryland, USA, Goddard Earth Sciences Data and Information Services Center (GES DISC) [data set], https://doi.org/10.5067/LYHA9088MFWQ, 2018.
Li, B., Beaudoing, H., and Rodell, M.: GLDAS Catchment Land Surface Model L4 daily 0.25 x 0.25 degree GRACE-DA1 V2.2, Greenbelt, Maryland, USA, Goddard Earth Sciences Data and Information Services Center (GES DISC) [data set], https://doi.org/10.5067/TXBMLX370XX8, 2020.
Liston, G. E.: Interrelationships among Snow Distribution, Snowmelt, and Snow Cover Depletion: Implications for Atmospheric, Hydrologic, and Ecologic Modeling, J. Appl. Meteorol., 38, 1474–1487, https://doi.org/10.1175/1520-0450(1999)038<1474:IASDSA>2.0.CO;2, 1999.
Liu, Y., Fang, Y., Li, D., and Margulis, S. A.: How Well do Global Snow Products Characterize Snow Storage in High Mountain Asia?, Geophys. Res. Lett., 49, e2022GL100082, https://doi.org/10.1029/2022GL100082, 2022.
Lundquist, J. D. and Dettinger, M. D.: How snowpack heterogeneity affects diurnal streamflow timing, Water Resour. Res., 41, W05007, https://doi.org/10.1029/2004WR003649, 2005.
Luojus, K., Moisander, M., Pulliainen, J., Takala, M., Lemmetyinen, J., Derksen, C., Mortimer, C., Schwaizer, G., and Nagler, T.: ESA Snow Climate Change Initiative (Snow_cci): Snow Water Equivalent (SWE) level 3C daily global climate research data package (CRDP) (1979–2018), version 1.0, Centre for Environmental Data Analysis [data set], https://doi.org/10.5285/fa20aaa2060e40cabf5fedce7a9716d0, 2020a.
Luojus, K.,Pulliainen, J., Takala, M., Lemmetyinen, J., and Moisander, M.: GlobSnow v3.0 snow water equivalent (SWE), PANGAEA [data set], https://doi.org/10.1594/PANGAEA.911944, 2020b.
Luojus, K., Pulliainen, J., Takala, M., Lemmetyinen, J., Mortimer, C., Derksen, C., Mudryk, L., Moisander, M., Hiltunen, M., Smolander, T., Ikonen, J., Cohen, J., Salminen, M., Norberg, J., Veijola, K., and Venäläinen, P.: GlobSnow v3.0 Northern Hemisphere snow water equivalent dataset, Scientific Data, 8, 163, https://doi.org/10.1038/s41597-021-00939-2, 2021.
Luojus, K., Moisander, M., Pulliainen, J., Takala, M., Lemmetyinen, J., Derksen, C., Mortimer, C., Schwaizer, G., Nagler, T., and Venäläinen, P.: ESA Snow Climate Change Initiative (Snow_cci): Snow Water Equivalent (SWE) level 3C daily global climate research data package (CRDP) (1979–2020), version 2.0. NERC EDS Centre for Environmental Data Analysis [data set], https://doi.org/10.5285/4647cc9ad3c044439d6c643208d3c494, 2022.
Mortimer, C. and Vionnet, V.: Northern Hemisphere historical in-situ Snow Water Equivalent dataset (1979–2021) (Version 1), Zenodo [data set], https://doi.org/10.5281/zenodo.10287093, 2024.
Mortimer, C., Mudryk, L., Derksen, C., Luojus, K., Brown, R., Kelly, R., and Tedesco, M.: Evaluation of long-term Northern Hemisphere snow water equivalent products, The Cryosphere, 14, 1579–1594, https://doi.org/10.5194/tc-14-1579-2020, 2020.
Mortimer, C., Mudryk, L., Derksen, C., Brady, M., Luojus, K., Venäläinen, P., Moisander, M., Lemmetyinen, J., Takala, M., Tanis, C., and Pulliainen, J.: Benchmarking algorithm changes to the Snow CCI+ snow water equivalent product, Remote Sens. Environ., 274, 112988, https://doi.org/10.1016/j.rse.2022.112988, 2022.
Mortimer, C., Mudryk, L., Cho, E., Derksen, C., Brady, M., and Vuyovich, C.: Use of multiple reference data sources to cross-validate gridded snow water equivalent products over North America, The Cryosphere, 18, 5619–5639, https://doi.org/10.5194/tc-18-5619-2024, 2024.
Mudryk, L. R., Derksen, C., Kushner, P. J., and Brown, R.: Characterization of Northern Hemisphere Snow Water Equivalent Datasets, 1981–2010, J. Climate, 28, 8037–8051, https://doi.org/10.1175/JCLI-D-15-0229.1, 2015.
Muñoz Sabater, J.: ERA5-Land hourly data from 1950 to present, Copernicus Climate Change Service (C3S) Climate Data Store (CDS) [data set], https://doi.org/10.24381/cds.e2161bac, 2019.
Muñoz-Sabater, J., Dutra, E., Agustí-Panareda, A., Albergel, C., Arduini, G., Balsamo, G., Boussetta, S., Choulga, M., Harrigan, S., Hersbach, H., Martens, B., Miralles, D. G., Piles, M., Rodríguez-Fernández, N. J., Zsoter, E., Buontempo, C., and Thépaut, J.-N.: ERA5-Land: a state-of-the-art global reanalysis dataset for land applications, Earth Syst. Sci. Data, 13, 4349–4383, https://doi.org/10.5194/essd-13-4349-2021, 2021.
Ochi, K., de Rosnay, P., and Fairbin, D.: Impact of assimilating ESA CCI Snow Cover on ECMWF Land Reanalysis, 10th EARSeL workshop on Land Ice and Snow, Bern, Switzerland, 6–8 February 2023, http://www.earsel.org/SIG/Snow-Ice/files/ws2023/Poster/1_A_Ochi_POSTER.pdf (last access: 13 January 2025), 2023.
Orsolini, Y. J., Senan, R., Balsamo, G., Doblas-Reyes, F. J., Vitart, F., Weisheimer, A., Carrasco, A., and Benestad, R. E.: Impact of snow initialization on sub-seasonal forecasts, Clim. Dynam., 41, 1969–1982, https://doi.org/10.1007/s00382-013-1782-0, 2013.
Seiler, C., Melton, J. R., Arora, V. K., and Wang, L.: CLASSIC v1.0: the open-source community successor to the Canadian Land Surface Scheme (CLASS) and the Canadian Terrestrial Ecosystem Model (CTEM) – Part 2: Global benchmarking, Geosci. Model Dev., 14, 2371–2417, https://doi.org/10.5194/gmd-14-2371-2021, 2021.
Simpson, I. R., Lawrence, D. M., Swenson, S. C., Hannay, C., McKinnon, K. A., and Truesdale, J. E.: Improvements in Wintertime Surface Temperature Variability in the Community Earth System Model Version 2 (CESM2) Related to the Representation of Snow Density, J. Adv. Model. Earth Sy., 14, e2021MS002880, https://doi.org/10.1029/2021MS002880, 2022.
Snauffer, A. M., Hsieh, W. W., and Cannon, A. J.: Comparison of gridded snow water equivalent products with in situ measurements in British Columbia, Canada, J. Hydrol., 541, 714–726, https://doi.org/10.1016/j.jhydrol.2016.07.027, 2016.
Takala, M., Luojus, K., Pulliainen, J., Derksen, C., Lemmetyinen, J., Kärnä, J.-P., Koskinen, J., and Bojkov, B.: Estimating northern hemisphere snow water equivalent for climate research through assimilation of space-borne radiometer data and ground-based measurements, Remote Sens. Environ., 115, 3517–3529, https://doi.org/10.1016/j.rse.2011.08.014, 2011.
Taylor, K. E.: Summarizing multiple aspects of model performance in a single diagram, J. Geophys. Res.-Atmos., 106, 7183–7192, https://doi.org/10.1029/2000JD900719, 2001.
Terzago, S., von Hardenberg, J., Palazzi, E., and Provenzale, A.: Snow water equivalent in the Alps as seen by gridded data sets, CMIP5 and CORDEX climate models, The Cryosphere, 11, 1625–1645, https://doi.org/10.5194/tc-11-1625-2017, 2017.
U.S. National Ice Center: IMS Daily Northern Hemisphere Snow and Ice Analysis at 1 km, 4 km, and 24 km Resolutions, G02156, Version 1, National Snow and Ice Data Center [data set], https://doi.org/10.7265/N52R3PMC, 2008.
Venäläinen, P., Luojus, K., Lemmetyinen, J., Pulliainen, J., Moisander, M., and Takala, M.: Impact of dynamic snow density on GlobSnow snow water equivalent retrieval accuracy, The Cryosphere, 15, 2969–2981, https://doi.org/10.5194/tc-15-2969-2021, 2021.
WMO: Guide to Instruments and Methods of Observation Volume II: Measurement of Cryospheric Variables, https://wgms.ch/downloads/WMO_8_II-2023_en.pdf (last access: 13 January 2025), 2018.
Wrzesien, M. L., Pavelsky, T. M., Durand, M. T., Dozier, J., and Lundquist, J. D.: Characterizing Biases in Mountain Snow Accumulation From Global Data Sets, Water Resour. Res., 55, 9873–9891, https://doi.org/10.1029/2019WR025350, 2019.
Zschenderlein, L., Luojus, K., Takala, M., Venäläinen, P., and Pulliainen, J.: Evaluation of passive microwave dry snow detection algorithms and application to SWE retrieval during seasonal snow accumulation, Remote Sens. Environ., 288, 113476, https://doi.org/10.1016/j.rse.2023.113476, 2023.