the Creative Commons Attribution 4.0 License.
the Creative Commons Attribution 4.0 License.
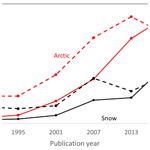
Exploring the decision-making process in model development: focus on the Arctic snowpack
Sirpa Rasmus
Ioanna Merkouriadi
Gianpaolo Balsamo
Annett Bartsch
Chris Derksen
Florent Domine
Marie Dumont
Dorothee Ehrich
Richard Essery
Bruce C. Forbes
Gerhard Krinner
David Lawrence
Glen Liston
Heidrun Matthes
Nick Rutter
Melody Sandells
Martin Schneebeli
Sari Stark
The Arctic poses many challenges for Earth system and snow physics models, which are commonly unable to simulate crucial Arctic snowpack processes,such as vapour gradients and rain-on-snow-induced ice layers. These limitations raise concerns about the current understanding of Arctic warming and its impact on biodiversity, livelihoods, permafrost, and the global carbon budget. Recognizing that models are shaped by human choices, 18 Arctic researchers were interviewed to delve into the decision-making process behind model construction. Although data availability, issues of scale, internal model consistency, and historical and numerical model legacies were cited as obstacles to developing an Arctic snowpack model, no opinion was unanimous. Divergences were not merely scientific disagreements about the Arctic snowpack but reflected the broader research context. Inadequate and insufficient resources, partly driven by short-term priorities dominating research landscapes, impeded progress. Nevertheless, modellers were found to be both adaptable to shifting strategic research priorities – an adaptability demonstrated by the fact that interdisciplinary collaborations were the key motivation for model development – and anchored in the past. This anchoring and non-epistemic values led to diverging opinions about whether existing models were “good enough” and whether investing time and effort to build a new model was a useful strategy when addressing pressing research challenges. Moving forward, we recommend that both stakeholders and modellers be involved in future snow model intercomparison projects in order to drive developments that address snow model limitations currently impeding progress in various disciplines. We also argue for more transparency about the contextual factors that shape research decisions. Otherwise, the reality of our scientific process will remain hidden, limiting the changes necessary to our research practice.
- Article
(1129 KB) - Full-text XML
-
Supplement
(1944 KB) - BibTeX
- EndNote
If the number of mentions in Intergovernmental Panel on Climate Change Assessment Reports (IPCC AR) can be used as a proxy to quantify the importance of a component in the climate system, then our understanding of the key role played by the cryosphere can be dated to the mid-2000s. Cryosphere processes and feedback covered just 5 pages in the IPCC Working Group I (WG1) AR3 (IPCC, 2001) but a 48-page dedicated chapter in the IPCC WG1 AR4 (IPCC, 2007). By the Sixth Assessment Cycle, an IPCC Special Report focused on the role of changing oceans and cryosphere under a changing climate (IPCC, 2019). The average number of mentions per page of the words “Arctic” and “snow” in 31 years of IPCC WG1 AR tripled (Fig. 1). Meanwhile, the Arctic as a whole has warmed at twice, with some regions almost 4 times, the global rate (e.g. Serreze et al., 2000; ACIA, 2005; Walsh, 2014; Rantanen et al., 2022).
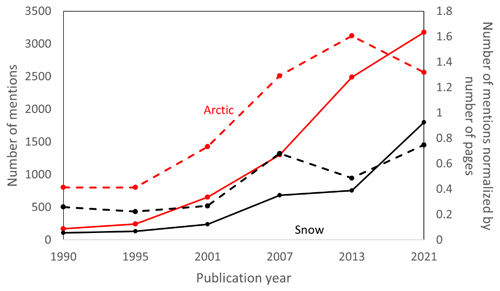
Figure 1Number of mentions of the words “Arctic” (red) and “snow” (black) in each IPCC WG1 AR (IPCC, 1990, 1995, 2001, 2007, 2013, 2021) (solid line) and number of mentions normalized by the number of pages in each report (dashed line).
The attribution and quantification of climate change by the IPCC WG1 are partly based upon simulations provided by Earth system models (ESMs), which are lines of code, written over time by multiple scientists, that describe processes relevant to life on Earth. Other types of models are dedicated to investigating specific components of the Earth system, e.g snow physics models. In both types of models, the “real world” must be translated into a numerical language, requiring modellers to make decisions at every stage of the model development. Given limited computing capabilities, modellers must decide which processes matter enough to be represented, which parameterization of the chosen processes best suits the purpose of their model, which language to use, how to select or tune parameter values, how to solve the equations, which input data are used, which decisions to leave to users, and which metrics to evaluate their model against; the list of “the choreography of coded procedures” (Gramelsberger, 2011) goes on.
The representation of snow in ESMs and snow physics models (hereafter, when combined, referred to as “snow models”) can take on various levels of complexity (here meaning incorporating an increasing number of processes) (see e.g. Slater et al., 2001; Largeron et al., 2020). The simplest representation is a soil–snow composite layer in which the top soil layer “becomes” snow by adopting some of its attributes when present, e.g. albedo and thermal conductivity. The next complexity level represents a single snow layer where bulk snowpack properties e.g., snow water equivalent (SWE), depth, and density, are simulated. Finally, multi-layer snow models usually allow a pre-determined maximum number of snow layers, although some models add snow layers corresponding to each snowfall, with their specific thickness, density, and other attributes.
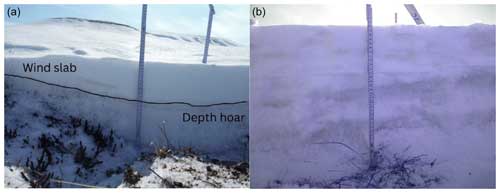
Figure 2High Arctic snowpack with wind slab over depth hoar, taken on Bylot island on 18 May 2015 by Florent Dominé, (a) and a near-infrared picture showing a 2 mm ice layer at 26 cm on 16 March 2018 (b). The ice layer on the right was the result of rain on snow on 15 January. Taken at Trail Valley Creek, Canada, by Nick Rutter.
Most multi-layer snow models use a densification model first developed by Anderson (1976), itself based on measurements made by Kojima (1967) in Sapporo and Moshiri, Hokkaido, Japan (hereafter the Anderson–Kojima scheme). The model parameters account for compaction due to the weight of the overlying snow, as well as destructive, constructive, and melt metamorphism; as such, each layer increases in density with depth. This snow profile broadly resembles the properties associated with montane forest and maritime snow (Sturm and Liston, 2021) but is not appropriate to simulate wind-packed snow and depth hoar, i.e. what Arctic tundra snowpacks are often almost entirely composed of (Fig. 2). Some snow physics models attempt to simulate Arctic-specific snowpack processes: the vapour diffusion that leads to depth hoar formation, the internal snowpack ice layers that commonly occur after rain-on-snow events, and the thick ice crust that forms at the surface of the snowpack following freezing rain (e.g. SNOWPACK in Wever et al., 2016 and Jafari et al., 2020; SnowModel in Liston et al., 2020; Crocus in Quéno et al., 2018, Touzeau et al., 2018 and Royer et al., 2021). However, no ESM, i.e. none of the state-of-the-art models that are used by researchers and policymakers globally to understand the complex interactions in the Earth's climate system, so far has simulated Arctic-specific snowpack processes. This is despite many in the climate change scientific community considering these processes to be critical for understanding changes in Arctic biodiversity, livelihood, permafrost, and the global carbon budget (e.g. Zhang et al., 1996; Rennert et al., 2009; Descamps et al., 2016; Domine et al., 2019; Serreze et al., 2021).
The aim of this study is, therefore, to understand why decisions made by the snow modelling community over the past decades have led to little or no progress in the representation of Arctic snowpack processes, i.e. in the part of the planet that warms faster than anywhere else. While a systematic literature review would provide some answers, this study takes a different approach borrowed from science and technology studies (STS), an interdisciplinary field wherein the scientists themselves are part of the investigation into understanding science in the making. Although the type of decisions needed throughout the different stages of model construction has been well documented by epistemologists and philosophers of climate science (e.g. Winsberg, 1999; Gramelsberger, 2011; Gramelsberger and Mansnerus, 2012; Parker and Winsberg, 2018; Morrison, 2021), what leads to these decisions remains “mostly hidden from view” (Winsberg, 2012). Therefore, to address our aim, we will investigate the construction of snow models by employing qualitative research methodologies, i.e. by interviewing the individuals who shape the content of snow models in order to uncover the factors that influence their decisions. The underlying premise of this aim is rooted in the belief that comprehending the cause of a problem – if indeed the absence of an Arctic snowpack is one – provides a foundation for addressing it and recommending ways to move forward, which we will do in the section “Discussion and moving forward”.
This study originated from discussions between the first three authors of this paper (Cecile B. Menard, Sirpa Rasmus, and Ioanna Merkouriadi) during which the representations, shortfalls, and progress in snowpack modelling were debated. Our understanding was that current snow models fell short of representing all the Arctic snowpack processes needed by our project collaborators on the interdisciplinary project CHARTER, which aims to enhance the adaptive capacity of Arctic communities to climatic and biodiversity changes (CHARTER, 2023). For example, for reindeer husbandry and investigations into the Arctic food web, CHARTER partners required accurate snowpack density profiles and information on the spatial distribution and hardness of ice layers formed by rain-on-snow events (see e.g. Laptander et al., 2024, for details). Recognizing that we had had these types of conversations with other colleagues over the years, we concluded that a different approach was needed to understand why any Arctic snowpack processes were yet to be included in most snow models. We opted to use qualitative research methodologies because they “place emphasis on seeking understanding of the meanings of human actions and experiences, and on generating accounts of their meaning from the viewpoints of those involved” (Fossey et al., 2002). As such and in accordance with qualitative research participant selection methodology, we compiled a shortlist of participants, both within and outside CHARTER, “who [could] best inform the research questions and enhance understanding of the phenomenon under study” (Sargeant, 2012). The shortlist was split into five so-called “expert” groups.
-
Snow modeller collaborators (SMCs) included participants with research expertise in Arctic fauna and flora biodiversity.
-
Field scientists (FSs) included participants whose field campaigns focus on snow-related processes and whose fieldwork supports the development of remote sensing and snow physics models.
-
Remote sensing scientists (RSSs) included participants involved in the development of satellite products or of remote sensing models for snow.
-
Snow physics modellers (SPMs) included participants who have developed and/or who are involved in the development a snow physics model.
-
Large-scale modellers (LSMs) included participants with expertise in ESMs, in the land surface component of ESMs, and/or in numerical weather prediction (NWP).
The shortlist initially included three participants in each of the five so-called expert groups. Potential participants were emailed with a request for participation that included a participant information sheet and consent form (see the Supplement); all those contacted accepted the invitation to participate. The groups were broadly split between stakeholders (SMC, FS, and RSS), i.e. users of snow models whose needs may influence the development priorities in snow model, and snow modellers (SPM and LSM), here meaning those who make the decisions about which developments are prioritized in the snow models they are involved in. The expertise classification was somewhat artificial, and, as we discovered during some interviews, distinctions between groups were sometimes negligible. For example, all but the LSM had extensive field experience, one FS had expertise in Arctic biodiversity, one RSS had been involved in the development of a snow physics model, one SPM had contributed to the development of a land surface model, and so on. These overlaps prompted the addition of four more participants to the shortlist to ensure comprehensive representation of expertise within some of the groups.
In total, 19 one-to-one interviews lasting between 40 and 65 min took place on Microsoft Teams or Zoom between August 2022 and January 2023. One SMC withdrew from the study shortly after the interview and their data are not used. All interviews, which were conducted by Cecile B. Menard, were individual in-depth semi-structured interviews, a qualitative data collection method in which a set of pre-determined open-ended questions, as well as themes emerging from the dialogue between interviewer and participants, are discussed (DiCicco-Bloom and Crabtree, 2006).
The description of Arctic snowpack processes and of their effects on various aspects of the Earth system was kept intentionally short in the Introduction section of this paper. Implicit within the rationale for this study is the assumption that opinions about the importance of including Arctic snowpack characteristics in snow models differ; otherwise, it would not be a topic for debate within the Arctic snow community (here meaning all disciplines where Arctic snow is significant, thus encompassing all of this study's participants). As all participants were asked to explain the significance of snowpack structure in their research and to articulate their understanding of the importance of representing Arctic snowpacks in snow models, the implications of Arctic snowpack processes not being represented are presented, throughout the paper, in the participants' own words.
Some questions asked by Cecile B. Menard differed between groups to reflect the expertise of the participants. SMC, FS, and RSS were interviewed to understand the diverse applications of Arctic snow (e.g. snow as a habitat, snow as an insulating medium, snow as a water resource, snow as a complex microstructure) and to evaluate whether limitations in snow models constrained their research. Interviews with individual group members followed in sequence (i.e. group 3 after 2 after 1, etc.) so that SMC, FS, and RSS could suggest questions to SPM and LSM. SPM and LSM were then asked about their decision-making process; e.g. how do they prioritize model developments? What are the limitations of their model, and how do they affect our understanding of Arctic snow processes?
All interviews were video-recorded and transcribed. The data (i.e. the interview transcripts) were analysed by conducting a thematic analysis (Braun and Clark, 2008; Rapley, 2010). This qualitative analytical approach consists of identifying codes, i.e. semantic content or latent features in interviews, and then collating them into overarching themes. In our study, one code or multiple codes were attributed by Cecile B. Menard to each statement in the transcripts. Iterative coding was conducted in NVivo, qualitative data analysis software that facilitates the classification and visualization of unstructured data. Three iterations were necessary to identify all codes and to classify codes into themes. Codes had to be identified in multiple conversations in order to be included in the final themes. Each theme is analysed separately in the section “Findings: separating the content from context” and provides the heading of each subsection (i.e. 3.x.x.). The quotes that best illustrated the themes are the ones included in the paper and are used throughout the paper. For readability (1) speech dysfluency in quotes was edited, and (2) the group of the participant who is quoted is indicated before or after the quote, generally between square brackets.
Qualitative researchers must declare “the position they adopt about a research task and its social and political context” (Holmes, 2020) because it influences how research is conducted and evaluated (Rowe, 2014). “Positionality” statements are necessary in qualitative research because one of the purposes they serve is to establish whether the researchers undertaking the study are “insiders” or “outsiders” to the culture under investigation (Holmes, 2020). As qualitative methods were employed to comprehend decision-making processes within a quantitative field, the positions of Cecile B. Menard, Sirpa Rasmus, and Ioanna Merkouriadi as either insiders or outsiders in relation to the expertise of the participants are presented here: Cecile B. Menard has been a model developer on snow physics and large-scale models. Sirpa Rasmus and Ioanna Merkouriadi have been users of snow physics models. All have conducted winter and summer fieldwork in the Arctic. All have collaborated or are currently collaborating closely with all groups represented.
Finally, as was stated on the consent form signed by the participants before each interview, all participants were invited to be co-authors of this paper. This practice is becoming increasingly customary in qualitative research because it recognizes that participants are joint contributors to the findings of a research project (Given, 2008; Pope, 2020). All but two accepted the invitation.
By opting for the semi-structured interview format, our aim was to use a medium, the conversation, in which using “I” was natural. The working title of this study on the participant information sheet was “A multi-perspective approach to snow model developments”, thus implicitly alluding to the fact that, by approaching a single issue from multiple angles, this study sought to elicit diverse responses. This certainly turned out to be the case. All participants provided important information related to their field – information that is presented in Sect. 3.1 – but they also ventured where few scientists do, at least in their publications: they offered opinions. No opinion was unanimous; in fact, every statement made by each participant was contradicted by a statement made by another participant. As such, none of the quotes are endorsed by all authors, and, by extension, it is expected that readers will also inevitably disagree with some quotes.
Some opinions were offered cautiously and reflected the participants' professional expertise. Others were more personal: “I'm sick of modellers who think the world is a computer screen”; “the scientific community is very conservative, so as soon as you try to change the paradigm, you have outcry and everyone hits each other”; “the[se] models spend so much time doing things that aren't very important that for lots of applications, they're kind of worthless”; “other groups have said we're going to start over, and that is also totally fraught”. Such open and candid comments do not (usually) make it into publications, but we argue that such statements are a manifestation of the participants' research identity, a concept extensively examined in education studies (e.g. Välimaa, 1998; Clegg, 2008; Fitzmaurice, 2013; Borlaug et al. 2023), defined by McCune (2019) as “the dynamic interplay over time of personal narratives, values and processes of identification with diverse groups and communities”. These processes of identification are clear in the participants' choice of words which echo McCune's definition: the participant who qualifies the scientific “community” as conservative distances themselves from this community, as does the other one from “groups” whose strategy they reject.
The participants' research identity also manifested itself in their interpretation of the Arctic under discussion. There are many definitions of Arctic, some of which are based on the Arctic circle, treeline, climate, permafrost, and so on (ACIA, 2005). CM began each interview by describing Arctic snowpack processes absent in existing models but did not define “Arctic” beyond land snow processes, causing varied interpretations. SMC, FS, and RSS, all of whom had extensive field experience, generally defined the type of Arctic they meant when describing a process, even if their description was at times itself open to interpretation: “proper Arctic”, “entire Arctic”, “high Arctic”, “Canadian Arctic”, “tundra”, “sub-Arctic and low Arctic”, “Scandinavian Arctic”, “polar snowpack”, “Finnish snowpack but not high Arctic”, and “pan-Arctic”. Only two SPMs and one LSM (out of four in each group) specified what Arctic they meant. No retrospective definition is provided because, despite these different interpretations, all participants knew of processes that snow models could not represent in “their” Arctic. Examples include rain-on-snow-induced ice layers, which predominantly occur in Fennoscandian oroarctic tundra, and internal snowpack thermal gradients and vapour fluxes, which are more relevant in the high Arctic.
In Sect. 3.1, we will outline the scientific reasons given by the participants for the lack of development of an Arctic snowpack based on the content of the interviews. In Sect. 3.2 we will examine the statements that deal with the context in which the participants' research is undertaken. By content we refer to the actual information being communicated, while context refers to the circumstances that help interpret that content.
3.1 Content
This section presents the participants' reflections on the scientific reasons why few snow model developments have accounted for properties relevant to Arctic snow.
3.1.1 Scale, heterogeneity, and internal consistency
The most often cited challenges impeding the implementation of an Arctic snowpack in large-scale models were related to scale, sub-grid heterogeneity, and the interplay of processes within the models. The difficulty in reconciling this triad when prioritizing model developments was captured by one participant: “[large-scale models] try to represent all land processes that are relevant to all around the world for all different problems and snow, of course, is just one of however many processes that we need to be considering” [LSM]. Therefore, “by necessity, you have to make some trade-offs” [FS].
These “trade-offs” vary in nature. One trade-off is to rank errors according to the perceived importance of the missing process as per this example: “the spatial variability of snow depth is so high that with respect to the energy exchange with the soil below, the error that you make if you get your snow depths wrong by a few centimetres is much larger than if you miss an ice layer” [SPM]. Another trade-off aims to maintain internal consistency in terms of complexity between the modelled processes: “Why would I have the perfect snow model and, at the same time, I would simplify clouds? (…) I want the model to be of the same degree of complexity in all its domains” [LSM]. Related to this is the opinion that “it is undesirable in global models to have regionally specific parameterizations” [SPM], as the inclusion of Arctic-specific processes was seen to be by some participants. This argument was countered by others who argued that, in models, solving the Arctic snowpack was not a geographical issue but a physical one: “the physics doesn't care where it is. [Getting the physics right] should make the model work wherever” [FS]. Finally, the last identified trade-off, which all LSMs mentioned, is error compensation. Sometimes modellers know that a parameter “is completely wrong, but it helps compensate an error [in another process. So] you have that resistance against improving a parameterization because you know that you have the error compensation” [LSM]. For instance, for this LSM, “in the final stages of model tuning for CMIP, I realized that error compensations had been broken away by improving the snow albedo. (…) So we backtracked and decided not to simulate snow albedo over the Antarctic. We set it to, 0.77 full stop; it's completely wrong but it helped compensate an error in the downwelling longwave”.
Issues of scale are further complicated by the fact that some models are being repurposed and operate at scales that they were not intended to. Examples include models initially developed for context-specific usage now being applied globally (“a lot of snow models are being used now in land surface schemes as broadly applicable snow models for all snow climate classes. But, I mean Crocus, it's an avalanche model, right?” [RSS]) and large-scale models increasing their resolution even though “the physics may not be anymore realistic. It's just a little sexier to be able to say you can run an Earth system model globally at 25 kilometres compared to what you used to run so” [RSS]. Although increasing resolution means that “processes that were before negligible are not so much so now” [LSM], LSM ranked improving the representation of albedo or of sub-grid heterogeneity due to shading and orography as higher on the priority list than e.g. vapour fluxes.
3.1.2 Data availability
Model developments are supported by and evaluated against observations: “Everything always starts at field site level in terms of testing a new model parameterization” [LSM]. Participants from all groups (which is not to say all participants) mentioned that more data were needed to understand the processes typical of an Arctic snowpack formation before being able to implement them in a model: “we need to be out there when it's really happening” and “we have very few sites across the Arctic”, so “it's not easy with the available data. We're looking to the observations people to provide the information on the Arctic snow” [SPM, RSS, SPM].
While the scale at which the models of the participants operate differed, all but one participant identified data gaps as being a limit to model developments. “If you don't have site data to attribute a process to, it is difficult to defend its implementation. For example, I'm not aware of sites that we could use to tackle wind compaction” [LSM]. Other participants highlighted the difficulty of parameterizing ice layer formation: “when you find an ice crust in the snow pit, you don't know whether it is from rain on snow or wind compaction”, so “for starters, you need the precipitation to be right” [RSS, LSM]. While some snow physics models attempt to simulate depth hoar formation (e.g. Crocus in Vionnet et al., 2012; SnowModel in Liston and Elder, 2006; SNOWPACK in Jafari et al., 2020), data against which to evaluate the thermal gradients and vapour transport that contribute to depth hoar formation are limited; to the authors' knowledge only one such dataset, which provides both driving and evaluation data, at a single site exists (Domine et al., 2021, at Bylot Island, Canada). However, “it's a pretty high bar before something changes [in large-scale models] based on a bit of experimental work. So, just because we get to show it at one site, that's not going to be good enough. You've got to show it over multiple sites, multiple regions” [FS].
However, there is one area where snow physics models were judged to be lagging behind data availability. Five participants mentioned that the micro-CT (Heggli et al., 2011), which allows measurements of the 3-D snowpack architecture, was a “step change” [RSS] in understanding internal snowpack properties. “[Models are trying] to catch up with [the available data] because they now have something which is higher-resolution and more objective than people looking through the microscopes handling lenses and trying to measure snow crystals on the grid, which was hugely subjective to compare to” [RSS].
3.1.3 The historical development of snow models
A total of 10 participants began the interview by providing some background about snow model developments, using this as a historical justification for Arctic snowpack properties not being included in snow models. For “the first 30 years, [snow physics models were] driven by climate system processes and hydrology, snow for water resources applications” or “were designed to understand and predict avalanches” [SPM, FS]. As for large-scale models “what [they] want to know about polar climate is when it influences where people live. There are people living, of course, in the high latitude, but most of the people live in the mid-latitudes” so “every parameterization in every [large -scale] model was developed for mid-latitudes. And some of them work in the Arctic and some of them don't” [LSM, LSM]. The historical legacy of model development impedes the implementation of Arctic-specific processes because the stratigraphy used in the Anderson–Kojima scheme makes it numerically challenging to adapt existing models. “[Models] are limiting the number of [snow] layers for computational stability and efficiency so they are not respecting the way in which the snowpack is actually built up, i.e. in episodic snowfall events, which will form different layers. (…) That structure couldn't represent ice layers; it would refreeze meltwater or rain on snow, but in layers that are thicker than you'd observe. With numerical diffusion, these layers would spread out so there won't be a strong density contrast” [SPM]. “Numerically, it's just messy [to simulate the formation of an ice layer] because all of a sudden you have a new layer in the middle of other layers” [SPM].
3.2 Context
This section draws on the arguments and opinions provided by the participants in Sect. 3.1, but with a focus on understanding the factors that influence them. Here, the arguments and opinions are framed within the context within which the participants evolve and which the participants either implied or explicitly mentioned, As such, this section relates more to the research environment than to the science itself.
3.2.1 The scale of needed resources
With the exception of error compensation, which is a numerical exercise, the trade-offs discussed in Sect. 3.1.1 are only necessary because developments perceived to be the most important needed to be prioritized. Prioritization itself is only necessary because human, financial, and computational resources are limited: “when I speak to large-scale modellers about rain on snow, the feedback is usually `we are aware that something needs to be done, but we have other priorities and we don't have resources for this'. It's not straightforward” [RSS].
The “few people called `academic scientists' [are but] a tiny group among the armies of people who do science” (Latour, 1979). These “armies” include stakeholders, governmental research agencies, funders, taxpayers, and others, all capable of influencing funding decisions. While participants generally accepted the competitive nature of funding stoically (“We've had trouble getting funding to do the work”, [but] “really good and important science will not always be funded because there's not enough money to go around” [SPM, SPM]), participants from all groups voiced concerns about the inadequate resources allocated to modelling centres given the high expectations placed on them: “we have two groups running two different land surface schemes within the same government department on a small budget. That makes no sense” and “that just means we're distributing our resources way too thin. Every group is tasked with doing everything – and there's a huge number of things to do in land modelling. (…) I don't think we're that far off from having a crisis situation. These models desperately need to be modernized” [RSS, LSM]. National modelling capabilities “need a lot more software engineering support to be able to rebuild these models” and to “make them sleek and flexible enough that we actually have the ability to make changes more quickly without causing bugs” [LSM].
Short-termism was also perceived to hinder progress. “It's very difficult to make [an Arctic snowpack] model and there are also very few measurements detailing the complexity of the stratigraphy. (…) It's a long-term task and it needs interdisciplinary working” [FS]. Some participants believed that their governmental or institutional strategies impeded progress: “[This government agency] has lots of short-term goals. `I need results for this project in 6 months'. Developing new tools is not part of the strategy” [FS]. In addition, there was a recognition that short-term funding meant that modelling groups had to rely on cheaper labour in short-term employment, such as PhD students and junior postdoctoral researchers. For some participants, this meant that the type of scientific expertise required for model developments could not be met: “You need that longevity of funding within one area. I mean, the idea that you're going to create an Arctic snow model in a PhD is...” (a non-verbal expression was used here and interpreted by CM as “mindboggling”) [SPM]. For others, the short-termism of precarious employment impeded continuity in model building: “you get a PhD student, (…) [they] do great work, (…) then [they're] done and [they] go on to a postdoc somewhere else” [RSS]. The value of what is considered long-term project funding (5 years) was highlighted by an SPM: “[this model development] would not be possible with a 2- to 3-year project. Even in 5 years we won't be finished, but it's still long enough to investigate the problem (…) [and to] trigger some collaborations. We are building [collaborations] between labs which will stay for longer [than our project]”.
Limited resources are also why data are not available, although this is not the only reason. Most Arctic research is conducted by researchers who are not based in the Arctic, which is a logistical reason why “the number of detailed measurements in the Arctic during the entire winter season is close to 0” [FS]. “If you want to study alpine snow (e.g. Col de Porte, France, and Davos, Switzerland, which were set up to support the local tourism industry), you get out of your home, walk in the field or take your car, drive 15 minutes and you see it. If you want to look at Arctic snow, it's more complex” [FS]. The nature of this complexity is manifold. Firstly, although no participant mentioned that meteorological instruments are prone to malfunctioning at low temperatures (see e.g. Fig. 3), it was understood to be the implicit reason why some measurements were not available. Secondly, “we need to find people willing to do this work in total darkness” [FS]; polar nights and harsh winter meteorological conditions make access to Arctic sites difficult, which is why field campaigns often take place in spring and summertime. However, “we need to observe how this happens in the real world. I mean, we certainly have snow pits and we see ice lenses there, but we need to be out there when it's really happening” [SPM].
3.2.2 Adaptability
Public funding is granted to projects that fall within the strategic objectives and research priorities of government funding agencies. As such, “the right to research” (Henkel, 2005) is conditional upon scientists adapting and responding to an evolving funding landscape. Although much literature argues that there is a conflict between academic freedom and solution-based or applied science (e.g. Henkel, 2005; Winter, 2009; Skea, 2021), we found instead that adaptability and shifting priorities were integral to the modellers' research identities. “To some degree, we follow what is being hyped, you know, if something is being hyped in nature” [LSM]. Model developments were presented as being responsive and at the service of others: “There is no master plan. It's opportunity driven, it depends on projects that come in, (…) on what some of the users want to do. It's kind of nice” [SPM]. When questioned about what the priorities for snow model developments are, one SPM answered as follows: “It's not just the snow modellers who can answer that. It is the people who want to use the snow models”. Arguably, performance-based research funding systems like the UK Research Excellence Framework have been in place long enough in some countries for researchers to have adapted to the constraints of the “publish or perish”, “be funded or fade out”, and “impact or pack in” culture.
In fact, interdisciplinary collaborations were the key motivation for model development, demonstrating the modellers' adaptability. The reasons for interdisciplinary collaborations driving snow model developments were manifold. First, they are necessary to address research questions: “Permafrost, snow, wildlife biology (…) These fields have evolved independently over the last 30 or 40 years or whatever (…) [Now] we're working together to do a better job of answering all these interdisciplinary questions” [SPM]. Second, they drive innovations in all fields involved: “if you don't have a good physical snow modelling capability, you can't maximize the value of new [satellite data] retrieval algorithms” [RSS]. Third, they allow model developments to be relevant to a wide range of stakeholders, as is, for example, the case with progress on the many sectors that rely on numerical weather predictions. Fourth, they generate funding: “We wouldn't have enough base funding to pay for a master plan [for model developments] so we are depending on projects that come in and on the interest of individual people” [SPM].
A particularly topical illustration of the significance of interdisciplinary collaborations for snow model development at the time of the interviews was the IVORI project (IVORI, 2023), which aims to develop a new type of snow model that will be able to model the snowpack processes existing models cannot. IVORI was mentioned spontaneously by eight participants other than the project lead (herself a participant in this study). “We had a consultation meeting [at a conference] in 2016. It was really mostly the snow community just saying, `hey, we want something better' (…) The ice core community was also pushing in this direction (…) [as well as] the remote sensing community [because] no model correctly represents snow microstructure [they need]” [SPM]. Although all participants were cautious not to oversell a model at a very early stage of its development, there was a lot of excitement around the project: “[IVORI] is trying to basically rethink the whole snow modelling issue from scratch and come up with a new model that will be the future” [SPM other than the IVORI project lead].
Finally, collaborations provide human resources, especially when models are open-source. From the developers' perspective, open-source means that “the majority of the development work is done [externally. For example,] for the most recent release, we had 50 people involved from 16 different institutions” [LSM]; for the users, it makes models “easy to use. You can just pick up examples and test the model for yourself (…)” and “if something doesn't work or if you have questions, you always find support” [RSS, LSM].
3.2.3 The anchoring bias
Some participants in all but the SMC group argued that many developers misjudged or did not understand the importance of snow when modelling Arctic processes. Four participants stressed the need to design and to implement a long-planned snow model intercomparison project (SnowMIP) focusing on tundra (in both Arctic and Antarctic) snow processes because “the first thing it would do is alert the modellers to the difficulties that they have in the Arctic that, in the absence of these evaluations, they wouldn't even know about…in my sense, large-scale climate modellers aren't sufficiently aware of snow. (…) There are so many people who don't care about that” [LSM].
One of the reasons some modellers “wouldn't even know about (…) the difficulties that they have in the Arctic” is because their existing models served as “anchors” or benchmarks. Anchoring is a common cognitive strategy where individuals, including experts, rely too heavily on an initial piece of information that they use to assess risk and uncertainty, leading to systematic errors in judgements (Tversky and Kahneman, 1974). Within our context, it means that even though some participants acknowledged that existing models represented Arctic snow processes poorly or not at all, the fact that they represented snow at all meant that some participants preferred not to take the risk of investing resources into new models or time-consuming complex developments.
For example, when an SPM said a “model is never perfect, but is it good enough for what is being done with it?”, what they interpret as being “good enough” is contextual. It depends on the research question to be addressed; on the data, time, and funding available; and on the extent to which what is expected of the model measures against the anchor. As such, what is good enough is dynamic and evolves as the anchor or reference point shifts. For one LSM, the anchor shifted during the interview: “I understand now what you [Cecile B. Menard] have been talking about, how far we are from what people who live in the Arctic really care about”. This insight, along with the historical development context outlined in Sect. 3.1.3, suggests that the anchoring bias in snow modelling partly reflects non-epistemic values (hereafter simply referred to as values), i.e. ethical and social considerations that help scientists make decisions which do not rely on expertise alone (see e.g. Rudner, 1953; Winsberg, 2012). For instance, the historical context outlined in Sect. 3.1.3 echoes value judgements prevalent in early model evolution that prioritized serving the majority of people who live in the mid-latitudes.
The anchor, or benchmark against which to evaluate model priorities, also shifts as a result of community efforts such as MIPs, which motivate developments because they “distil the information and tell [modellers] what are the priorities and what are the sites good for. (…) [SnowMIP] brings together observation experts and other models and modellers. We all learn enormously” [LSM]; “the community does a reasonably good job of trying to develop, incrementally, through different research groups” [FS]. Nevertheless, as “models are not [currently] very well tested for the Arctic, it is not easy to know what they do well” [SPM], anchoring bias plays an important part in the assessment of whether models are good enough or not.
Finally, eight participants spontaneously discussed the risks and benefits of starting models from scratch in view of ongoing projects undertaking this task (e.g. CliMA, 2023, a novel type of climate model, and IVORI). While the time and effort of such an undertaking were the main causes for concern (“With respect to the new model, what I see is that this quest for purity (…) makes things extremely slow”; “the effort of rewriting a climate model [is huge]. I'm not saying it's not worth it (…) but I can understand why people don't do it” [SPM, LSM]), it is because the participants were weighing the value of starting from scratch against, instead, a reference or anchor point, i.e. the existing models, that one concluded that starting from scratch was “totally fraught because you're probably talking about a 5-year project to get even close to the capability of what the current models have. And at the moment, who wants to give up their capabilities?” [LSM]. On the other side of the argument, an FS argued that “trying to improve the candle did not invent electricity. [For tundra snow], existing snow models, there's one thing to do with them. Trash”. Somewhere in the middle, more nuanced opinions were presented: “The community should be endorsing IVORI, but there is such a lag between activities like this and the current suite of models, which people use in high-impact papers, that we also need to spend time understanding what the limitations are and how we can get some improvement out of these models” [RSS].
As mentioned in the Introduction, the premise of this study is rooted in the belief that comprehending the cause of a problem would provide a foundation to address it. The premise found echoes in this RSS's quote: “[You] should never keep doing what you're doing because that's the way it's always been done. (…) What are the priorities? What do we need to learn? What do we need to do that's new?” Section 3.1 and 3.2 show conflicting answers, opinions, and perspectives regarding these three questions. In this section, we aim not to reconcile these opinions, but, based on our reflections on the findings, we will aim to start answering these questions to propose ways forward.
4.1 Opening up research
As mentioned in Sect. 3.2.3, values have contributed to deciding priorities for snow model development over time, such as the importance attributed to their relevance to where “most of the people live” [LSM] e.g. for their survival (e.g. water resources) or leisure (e.g. avalanche forecasting). As mentioned in Sect. 2, SMC, FS, and RSS were interviewed to provide a broad picture of the range of Arctic snow applications and to understand how the absence of an Arctic snow model constrained their own research. Because of the different role that the Arctic snowpack plays in their research, these participants reframed snow models away from their historical model legacies into research areas seen as being underexplored by the Arctic snow community. They proposed how efforts to represent Arctic snowpack processes could pave the way for new interdisciplinary collaborations, yielding benefits such as innovation, stakeholder involvement, and funding.
Permafrost–carbon feedback. “Snow is a kind of blind spot in the international climate modelling community. We know that snow is wrong, but people are not coordinated, people are not really working together” [LSM]. “At the moment, snow structure is not considered for permafrost modelling. It's only how thick the snow is and whether the temperature decouples from the ground or not” [RSS]. Participants from all groups highlighted the importance of snowpack structure to understand soil winter processes. “It's clear that the winter climate is changing even more than the summer climate” [SMC]. For example, “when there is rain on snow, the short-term warming to the ground influences the entire following winter history. What is the magnitude of the impact? Knowing the temperature at the base of the snow is the really crucial information” [RSS]. One participant stressed the importance of upscaling the many in situ soil experiments with the help of suitable snow models: “What manipulation experiments show is that whether we have less snow, or shorter winters, or we have ice layers or something else will have very different, even opposite, effects on soil processes, gas exchanges, plant and soil ecology. (…) For example, when you have ice layers, the ice is disturbing the gas exchange between the soil and atmosphere, but it's still active (…) [so] you get carbon dioxide accumulation. We also found that soil microbes are resilient to late snowpack formation and earlier melt, but the growing season started earlier than usual. (…) [What we now need] is to translate the results of that experiment to larger landscape level” [SMC].
Arctic food webs. Upscaling is also needed to translate local-scale findings to ecosystem scale when investigating fauna biodiversity. “When the snow gets very hard [e.g. after an ROS event or refreezing], lemmings don't move as well through the snow; they cannot access their food anymore and then they starve. (…) [Many] specialized Arctic predators depend on lemmings to survive (…) or to reproduce successfully (e.g. snowy owls, pomarine skuas, Arctic foxes). (…) They also eat a lot and influence the vegetation. (…) If a snow model could reconstitute the snowpack in a reliable way, we could see if there a relationship at the large scale between cyclic lemming populations and snow conditions (…) and address a row of other ecological hypotheses” [SMC].
Reindeer husbandry. For reindeer herders, obtaining near-real-time spatial information on the structure of the snowpack could save their livelihood and their lifestyle: “During the winters of 2020 and 2021, we had thawing, raining, and refreezing in January and there was already a lot of moisture at the ground from the previous fall. So the reindeer have to dig through all that and then there's a layer of ice on the ground. The lichens, blueberries, everything is encased in ice. So there's [sic] two options. They starve or they short-circuit their digestive system because they eat the ice-encrusted vegetation [and] get too much water in their rumen. The Sami herders say that kills the animal anyway. (…) If the herders could get a heads-up [that rain is coming], (…) they [could] immediately communicate with their neighbours to ask: (…) can I go move my herd? East. West. Where is the soft snow?” [SMC].
Remote sensing applications. Remote sensing products are used to tackle many environmental issues, including the three described in this section, and their development is intrinsically linked with physically based models. “Remote sensing doesn't work everywhere all the time so we need to combine information from a model and from satellite data. We need to improve the physical snow models, but in step with developing the remote sensing. If you do one without the other then you're not gonna be able to maximize the value of both” [RSS]. For example, “snow has a confusing effect on retrieval estimates. Some of the signal comes from the atmosphere [e.g. clouds], some comes from the snow, and if you can't disentangle what comes from what then you just throw away millions of satellite data that could potentially be used for numerical weather prediction, better weather forecasts” [RSS].
4.2 A plurality of strategies
Discussions about trade-offs in model building (as in Sect. 3.1.1) precede the development of the first general circulation models (Manabe, 1969), the core components of ESMs, which already included snow. In 1966, Levins argued that, given computational constraints that remain valid 6 decades later, models could not be general, precise, and realistic at the same time; when designing their model building strategy, modellers had to choose which property to trade off. Levins concluded that as no single model strategy could represent a complex system, a plurality of models and model strategies was necessary to provide a more comprehensive picture of the system.
The different opinions expressed throughout this paper suggest that the participants support different strategies. The strategies they endorse are partly dictated by different local epistemologies, i.e. assumptions, methodologies, and aims specific to a community (Longino, 2002), as well as disciplinary identities, i.e. discipline-specific socio-historical norms (Dressen-Hammouda, 2008). For example, ESMs must sacrifice realism and so must, by extension, LSMs: ESMs are precise because they use equations that provide precise outputs and general because these equations must be applicable globally, but they have unrealistic internal processes (e.g. see error compensation in Sect. 3.1.1). However, within-group disagreements and between-group agreements also show that disciplinary identity and local epistemologies do not always dominate the research identity narrative of the participants. As noted in Sects. 3.2.2 and 4.1, collaborations are drivers for model developments, and, when interdisciplinary, these collaborations will also shape the research identities by exposing them to different disciplinary identities and local epistemologies. For example, as mentioned in Sect. 3, one FS declared that they are “sick of modellers who think the world is a computer screen. (…). If you haven't been in the field (…), you just don't understand what's going on”. However, another FS declared that “there are people doing fantastic snow modelling work who don't really see a lot of snow, but they've got the appreciation of understanding what the detail is. It helps to see [on the field] what you're looking at [on your screen], but it's not an absolute essential”. The two FSs show clear differences in their value judgements, with the first one valuing empirical evidence and lived experience over theoretical knowledge and the second having “become a bit more nuanced in [their] thinking” after having been “exposed to different types of models”. Historically, the notion linking value-free science with objectivity and impartiality has prevailed (Pulkkinen et al., 2022) and was an obstacle to bridging the gap between our personal identity, reflected in our values, and our research identity, reflected in our professional decisions (Staddon, 2017). However, the role that non-epistemic values play in climate science was recognized in a dedicated subsection (1.2.3.2) of the IPCC WG1 AR6 (IPCC, 2021), thus providing a space for these conversations to occur in a field historically dominated by epistemic values (e.g. truth, accuracy, falsifiability, replicability).
While Levins' plurality of model strategies was originally aimed at model building in population biology, its relevance has been extended to climate science by, amongst others, Parker (2011), Lloyd (2015), Morrison (2021), Walmsley (2021), and Winsberg (2021). They argue that diversity of opinions, values, epistemic pluralities, and strategies does not need to be resolved but, on the contrary, that a plurality of models that investigate the same phenomenon from different representational perspectives is necessary. One of the most prominent examples in which climate science exploits this plurality is via MIPs, which aim to assess “the robustness, reproducibility, and uncertainty attributable to model internal structure and processes variability” (IPCC, 2021).
However, considered together, existing snow models do not provide this plurality of representational perspectives necessary to understand a complex system. Instead, many of these models are interdependent (Essery et al., 2013) and, rather, provide a plurality of representational complexities all based on the same Anderson–Kojima scheme. Aligning with epistemologists of science, we argue that developing a snow model adapted to Arctic snowpack processes to complement existing models is, therefore, necessary to provide the diversity in model strategies needed to understand complex Arctic processes and interactions.
4.3 Snow model intercomparison projects
Earth System Modelling–SnowMIP (ESM-SnowMIP; Krinner et al., 2018), the fourth snow model intercomparison in 24 years (Slater et al., 2001; Etchevers et al., 2004; Essery et al., 2009; Rutter et al., 2009), is a community effort that aims to evaluate snow schemes in ESMs and to improve our understanding of snow-related feedback in the Earth system. Out of the 10 planned exercises, only 2 have taken place so far (Menard et al., 2021; Essery et al., 2020). During the first exercise, little progress in snow models was found to have occurred since the previous snow MIPs (Menard et al., 2021) because of scientific reasons as well as contextual circumstances that resonate with the findings in this study.
The next planned phase, which aims to test models in the tundra, has suffered a number of setbacks, not least because “the models are not very well tested for the Arctic so it is not easy to know what they do well and it's not easy to ask that question with the available data” [SPM]. In line with discussions about responsible modelling in other sectors (e.g. Saltelli et al., 2020; Nabavi, 2022), we argue that by involving stakeholders (e.g. as represented here by SMC, FS, and RSS) in future snow MIPs, the models would be better prepared to tackle research questions that currently remain unanswered (although there have been attempts to do so with the existing models), thereby unlocking opportunities in new research domains and motivating the collection of the new type of data needed to test models in the Arctic (Sect. 3.1.2 and 3.2.1). The research questions identified in Sect. 4.1 should contribute to determining the focus of the next snow MIP rather than the next snow MIP determining what questions can be answered given the current modelling constraints, with the latter approach failing to challenge the notion that existing models are good enough.
Another consideration would be what legacy a tundra SnowMIP would want to leave behind. In the past, SnowMIP participants were required to provide model results. However, if a tundra SnowMIP is to advance snow modelling, the obstacles that limit the implementation of Arctic tundra snow processes (see Sect. 3.1) should be directly addressed. One suggestion mentioned by participants, although not within a SnowMIP context, was that moving forward, “shareable modules would be strategies that would allow us to make better progress” because “it will be easier for people to take your parameterization, take your model compartment and put it in their model to see what it does”. We argue that future snow MIPs should be vehicles to foster more direct collaborations between modelling teams and with users by advocating for sharing of, amongst others, code, results, and configuration files. This would avoid duplication of efforts and accelerate the model developments required to tackle Arctic snow challenges.
However, “a modelling centre doesn't get money to do a MIP, but they want to do it because it's important to them. So, they end up being involved, but they get MIP-saturated and that's when the errors arise. (…) At the very least, future SnowMIP-like projects need dedicated people whose main responsibility is to take this on, to say `I have funding to do it, I can dedicate time to it' ” [RSS]. Lack of funding for MIP participation is one of the many contextual factors Menard et al. (2021) identified as hindering the first ESM-SnowMIP exercise. Unless the context in which MIPs, SnowMIP or otherwise, operate is reconsidered, the same factors will continue to hinder community efforts.
4.4 Modeller accountability and empowerment
Models are not only the representation of a situation but are also the product of many socio-political interactions (Nabavi, 2022). Even when models lack core government funding, the ability of modellers (as defined here in Sect. 2) to secure competitive funding underscores their alignment with strategic research priorities that often reflect political agendas. Heymann and Dahan Dalmedico (2019) argued that the IPCC ushered in a new era of expertise in which scientists are conditioned and formalized by politically relevant issues; as architects of ESMs, this implies that modellers become vehicles for political agendas.
Participants in this study have provided various reasons for not having prioritized the development of an Arctic snowpack model: data availability, historical context, human resources, lack of funding, competing research priorities, strategic priorities of government agencies, and so on. In Sect. 4.2, we discuss the role of values, which are situated within a social and political context, in these decisions. We argue that they warrant more transparency in revealing the position of modellers within these contexts. We suggest that, following Bourdieu (2001), who argued that scientists should not take a position without acknowledging that they are doing so, natural scientists should position themselves as “insiders” and “outsiders” within the context of the research they conduct and publish (Cecile B. Menard, Sirpa Rasmus, and Ioanna Merkouriadi followed this advice themselves in Sect. 2). “Coming clean” (Lincoln, 1995) about our positionality in our publications would foster a more responsible research environment and contribute to the ongoing discussion about the role of values in climate science, as explored Sect. 4.2. For instance, weaknesses in the reviewing process as described below may be avoided if positionality statements allowed journal editors to identify gaps in the authors' expertise: “Some papers (…) don't make the effort to quantify what the sensitivity of their key result is to how snow is characterized in the model. [For example, if the paper is] (…) about carbon budgets across the Arctic over a 12-month seasonal cycle, [the review] always goes towards the growing season community. (…). So [these papers] don't get scrutinized the way they should” [RSS].
Finally, a “unique practice of sensitive wording” (Gramelsberger et al., 2020) was developed in climate science to describe the information produced by climate models. This practice satisfies the socio-political expectations of climate science to produce trusted information in decision-making, as well as acting as a barrier to accidental or intentional misinterpretation of the same information by climate deniers. An example of such sensitive wording is the “likelihood language” used to describe scientific uncertainties (Landström, 2017; Moss and Schneider, 2000). We suggest that another instance of sensitive wording is the separation between the model and the modeller, which contributes to presenting the information produced as objective and impartial. For example, the IPCC WG1 AR6 mentions “model(s)” 12666 times but “modeller(s)” three times. Such wording makes invisible the role of modellers in the decision-making process of model development and evaluation and, arguably, in some of the information produced in climate science. Yet, models are a product of one or multiple modellers' vision. This was reflected in the interviews during which more participants referred to Richard's model, Glen's model, or Marie's model rather than to FSM, SnowModel, and IVORI, respectively. David Lawrence was named by all participants who mentioned CLM, as was Michael Lehning for SNOWPACK. Crocus was the only model that a large majority of participants did not associate with any particular modeller. The research identity of many modellers is, whether they want it or not, intertwined with their model; inviting authors to reflect about their positionality would allow them to regain control over their own narrative and research identity.
As per more conventional review papers, the novelty in this paper is not in its content but in the medium it chooses with which to present that content. What participants said, they had said, but not necessarily written, before. Conferences, workshops, meetings, and end-of-day visits to more informal venues are places where disagreements about the limits and motivations to model development are debated. But while the written history narrated by our publications does record the arguments presented here in terms of content, it does not record what is presented in terms of context.
In fact, the medium is not novel either. Science and technology studies examine the context within which science is constructed and philosophers of science have long debated the decision-making process of scientists. As such, much of what is non-Arctic snowpack-specific could probably be found in many of these disciplines' seminal texts. However, although one of the participants directly quoted one of Thomas Kuhn's, a pioneer of STS, concepts when they advocated for a change in paradigm (Kuhn, 1962), STS is practised by outsiders looking in on a field, as is philosophy of science. These positions hinder the dissemination of their findings to, and the acceptance of their recommendation by, insiders.
Therefore, the novelty here is that it is an insider's job. It is a reflective exercise which, we hope, will be the start rather than the end point of the conversation. The comments of the participants-turned-co-authors at the paper writing stage certainly suggested as much: “it's interesting that nobody commented on the conventional wisdom that modelling tundra snow is too hard”; “discussions about digital Earth twins are shaking the [LSM] community. Some suggest that many resources, on continental or even global level, should be bundled to create the one big model. Others think this is a recipe for disaster, and some that it is `scientific colonialism”'; “the next step in modelling should be an evolutionary one: we should take the best of each”. The participants were interviewed in their role (or identity) as researchers, but all will have been reviewers of papers and grants, some will have been (co-)editors of journals, and some will have influenced policymakers. We argue that it is our role as insiders to motivate the change to our own practice. We also argue that it is our role as researchers to be more transparent about the contextual factors that influence and restrict our decisions. More importantly, it is our role as reviewers, editors, and policymakers to allow such transparency to happen and to openly challenge the idea that short-term funding can lead to ground-breaking science, that Arctic data can be collected without engaging the people who live there, and that 40-year-old models are good enough to tackle challenges we knew nothing about 10 years ago. If we fail to take on these roles, the reality of our scientific process will remain invisible and silent and, by virtue of it being hidden, unchanged.
The transcripts are not available as they contain sensitive and personal information.
The supplement related to this article is available online at: https://doi.org/10.5194/tc-18-4671-2024-supplement.
CBM, SR, and IM conceptualized the research. CBM conducted the interviews and analysed the data. CBM prepared the original draft with contributions from SR and IM. All other co-authors were interviewed for the research and contributed to the final version of the manuscript.
At least one of the (co-)authors is a member of the editorial board of The Cryosphere. The peer-review process was guided by an independent editor, and the authors also have no other competing interests to declare.
Publisher's note: Copernicus Publications remains neutral with regard to jurisdictional claims made in the text, published maps, institutional affiliations, or any other geographical representation in this paper. While Copernicus Publications makes every effort to include appropriate place names, the final responsibility lies with the authors.
Cecile B. Menard, Sirpa Rasmus, and Ioanna Merkouriadi thank all co-authors, Michael Lehning, Juha Lemmetyinen, and the one anonymous participant who withdrew from the study for being interviewed. We thank Jan Erik Knutsen for providing the photo used in Fig. 3. This project was funded by the European Union's Horizon 2020 programme (CHARTER, grant no. 869471).
This research has been supported by EU Horizon 2020 (grant no. 869471).
This paper was edited by Emily Collier and reviewed by Monica Morrison and two anonymous referees.
ACIA: Arctic Climate Impact Assessment, ACIA Overview report, Cambridge University Press, Cambridge, 1020 pp., ISBN 0521865093, 2005.
Anderson, E. A.: A point energy and mass balance model of a snow cover, Tech. Rep. 19, NOAA, Silver Spring, Md, https://repository.library.noaa.gov/view/noaa/6392 (last access: 30 September 2024), 1976.
Borlaug, S. B., Tellmann, S. M., and Vabø, A.: Nested identities and identification in higher education institutions—the role of organizational and academic identities, High. Educ., 85, 359–377, https://doi.org/10.1007/s10734-022-00837-5, 2023.
Bourdieu, P.: Science de la science et réflexivité, Raisons d'Agir, Paris, 240 pp., ISBN 978-2-912107-14-5, 2001.
Braun, V. and Clarke, V.: Using thematic analysis in psychology, Qual. Res. Psychol., 3, 77–101, https://doi.org/10.1191/1478088706qp063oa, 2008.
CHARTER: https://www.charter-arctic.org/, last access: 29 November 2023.
Clegg, S.: Academic identities under threat?, Br. Educ. Res. J., 34, 329–345, https://doi.org/10.1080/01411920701532269, 2008.
CLiMA: https://clima.caltech.edu/, last access: 29 November 2023.
Descamps, S., Aars, J., Fuglei, E., Kovacs, K. M., Lydersen, C., Pavlova, O., Pedersen, Å. Ø., Ravolainen, V., and Strøm, H.: Climate change impacts on wildlife in a High Arctic archipelago – Svalbard, Norway, Glob. Change Biol., 23, 490–502, https://doi.org/10.1111/gcb.13381, 2016..
DiCicco-Bloom, B. and Crabtree, B. F.: The qualitative research interview, Med. Educ., 40, 314–321, https://doi.org/10.1111/j.1365-2929.2006.02418.x, 2006.
Domine, F., Picard, G., Morin, S., Barrere, M., Madore, J.-B., and Langlois, A.: Major issues in simulating some Arctic snowpack properties using current detailed snow physics models: Consequences for the thermal regime and water budget of permafrost, J. Adv. Model. Earth Sy., 11, 34–44, https://doi.org/10.1029/2018MS001445, 2019.
Domine, F., Lackner, G., Sarrazin, D., Poirier, M., and Belke-Brea, M.: Meteorological, snow and soil data (2013–2019) from a herb tundra permafrost site at Bylot Island, Canadian high Arctic, for driving and testing snow and land surface models, Earth Syst. Sci. Data, 13, 4331–4348, https://doi.org/10.5194/essd-13-4331-2021, 2021.
Dressen-Hammouda, D.: From novice to disciplinary expert: Disciplinary identity and genre mastery, Engl. Specif. Purp., 27, 233–252, https://doi.org/10.1016/j.esp.2007.07.006, 2008.
Essery, R., Rutter, N., Pomeroy, J., Baxter, R., Stähli, M., Gustafsson, D., Barr, A., Bartlett, P., and Elder, K.: SNOWMIP2: An Evaluation of Forest Snow Process Simulations, B. Am. Meteorol. Soc., 90, 1120–1135, https://doi.org/10.1175/2009BAMS2629.1, 2009.
Essery, R., Morin, S., Lejeune, Y., and Menard, C. B.: A comparison of 1701 snow models using observations from an alpine site, Adv. Water Resour., 55, 131–148, https://doi.org/10.1016/j.advwatres.2012.07.013, 2013.
Essery, R., Kim, H., Wang, L., Bartlett, P., Boone, A., Brutel-Vuilmet, C., Burke, E., Cuntz, M., Decharme, B., Dutra, E., Fang, X., Gusev, Y., Hagemann, S., Haverd, V., Kontu, A., Krinner, G., Lafaysse, M., Lejeune, Y., Marke, T., Marks, D., Marty, C., Menard, C. B., Nasonova, O., Nitta, T., Pomeroy, J., Schädler, G., Semenov, V., Smirnova, T., Swenson, S., Turkov, D., Wever, N., and Yuan, H.: Snow cover duration trends observed at sites and predicted by multiple models, The Cryosphere, 14, 4687–4698, https://doi.org/10.5194/tc-14-4687-2020, 2020.
Etchevers, P., Martin, E., Brown, R., Fierz, C., Lejeune, Y., Bazile, E., Boone, A., Dai, Y.-J., Essery, R., Fernandez, A., Gusev, Y., Jordan, R., Koren, V., Kowalczyk, E., Nasonova, N. O., Pyles, R. D., Schlosser, A., Shmakin, A. B., Smirnova, T. G., Strasser, U., Verseghy, D., Yamakazi, T., and Yang, Z.-L.: Validation of the energy budget of an alpine snowpack simulated by several snow models (Snow MIP project), Ann. Glaciol., 38, 150–158, https://doi.org/10.3189/172756404781814825, 2004.
Fitzmaurice, M.: Constructing professional identity as a new academic: a moral endeavour, Stud. High. Educ., 38, 613–622, https://doi.org/10.1080/03075079.2011.594501, 2013.
Fossey, E., Harvey, C., McDermott, F., and Davidson, L.: Understanding and evaluating qualitative research, Aust. N. Z. J. Psychiat., 36, 717–732, https://doi.org/10.1046/j.1440-1614.2002.01100.x, 2002.
Given, L. M.: Participants as co-researchers, in: The SAGE Encyclopedia of Qualitative Research Methods, SAGE Publications, Inc., https://doi.org/10.4135/9781412963909, 600-601, 2008.
Gramelsberger, G.: What do numerical (climate) models really represent?, Stud. Hist. Philos. Sci., 42, 296–302, https://doi.org/10.1016/j.shpsa.2010.11.037, 2011.
Gramelsberger, G. and Mansnerus, E.: The Inner World of Models and Its Epistemic Diversity: Infectious Disease and Climate Modelling, in: Ways of Thinking, Ways of Seeing. Automation, Collaboration, & E-Services, vol 1., edited by: Bissell, C. and Dillon, C., Springer, Berlin, Heidelberg, https://doi.org/10.1007/978-3-642-25209-9_8, 2012..
Gramelsberger, G., Lenhard, J and Parker, W. S. J.: Philosophical Perspectives on Earth System Modeling: Truth, Adequacy, and Understanding, J. Adv. Model. Earth Sy., 12, 2019MS001720, https://doi.org/10.1029/2019MS001720, 2020.
Heggli, M., Köchle, B., Matzl, M., Pinzer, B. R., Riche, F., Steiner, S., Steinfeld, D., and Schneebeli, M.: Measuring snow in 3-D using X-ray tomography: assessment of visualization techniques, Ann. Glaciol., 52, 231–236, https://doi.org/10.3189/172756411797252202, 2011.
Henkel, M.: Academic identity and autonomy in a changing policy environment, High Educ., 49, 155–176, https://doi.org/10.1007/s10734-004-2919-1, 2005.
Heymann, M. and Dahan Dalmedico, A.: Epistemology and politics in Earth system modeling: Historical perspectives, J. Adv. Model. Earth Sy., 11, 1139–1152, https://doi.org/10.1029/2018MS001526, 2019.
Holmes, A. G. D.: Researcher Positionality – A Consideration of Its Influence and Place in Qualitative Research – A New Researcher Guide, Shanlax Int. J. Educ., 8, 1–10, https://doi.org/10.34293/education.v8i4.3232, 2020.
IPCC: Climate Change: The IPCC Scientific Assessment, edited by: Houghton, J. T., Jenkins, G. J., and Ephraums, J. J., Cambridge University Press, Cambridge, United Kingdom and New York, NY, USA, 414 pp., 1990.
IPCC: Climate Change 1995: The Science of Climate Change, edited by: Houghton, J. T., Meira Filho, L. G., Callander, B. A., Harris, N., Kattenberg, A., and Maskell, K., Cambridge University Press, Cambridge, United Kingdom and New York, NY, USA, 588 pp., 1995.
IPCC: Climate Change 2001: The Scientific Basis. Contribution of Working Group I to the Third Assessment Report of the Intergovernmental Panel on Climate Change, edited by: Houghton, J. T., Ding, Y., Griggs, D. J., Noguer, M., van der Linden, P. J., Dai, X., Maskell, K., and Johnson, C. A., Cambridge University Press, Cambridge, United Kingdom and New York, NY, USA, 881 pp., 2001.
IPCC: Climate Change 2007: The Physical Science Basis. Contribution of Working Group I to the Fourth Assessment Report of the Intergovernmental Panel on Climate Change, edited by: Solomon, S., Qin, D., Manning, M., Chen, Z., Marquis, M., Averyt, K. B., Tignor, M., and Miller, H. L., Cambridge University Press, Cambridge, United Kingdom and New York, NY, USA, 996 pp., 2007.
IPCC: Climate Change 2013: The Physical Science Basis. Contribution of Working Group I to the Fifth Assessment Report of the Intergovernmental Panel on Climate Change, edited by: Stocker, T. F., Qin, D., Plattner, G.-K., Tignor, M., Allen, S. K., Boschung, J., Nauels, A., Xia, Y., Bex, V., and Midgley, P. M., Cambridge University Press, Cambridge, United Kingdom and New York, NY, USA, 1535 pp., ISBN 9781107661820, 2013.
IPCC: IPCC Special Report on the Ocean and Cryosphere in a Changing Climate, edited by: Pörtner, H.-O., Roberts, D. C., Masson-Delmotte, V., Zhai, P., Tignor, M., Poloczanska, E., Mintenbeck, K., Alegría, A., Nicolai, M., Okem, A., Petzold, J., Rama, B., and Weyer, N. M., Cambridge University Press, Cambridge, UK and New York, NY, USA, https://doi.org/10.1017/9781009157964, 755 pp., 2019.
IPCC: Climate Change 2021: The Physical Science Basis. Contribution of Working Group I to the Sixth Assessment Report of the Intergovernmental Panel on Climate Change, edited by: Masson-Delmotte, V., Zhai, P., Pirani, A., Connors, S. L., Péan, C., Berger, S., Caud, N., Chen, Y., Goldfarb, L., Gomis, M. I., Huang, M., Leitzell, K., Lonnoy, E., Matthews, J. B. R., Maycock, T. K., Waterfield, T., Yelekçi, O., Yu, R., and Zhou, B., Cambridge University Press, Cambridge, United Kingdom and New York, NY, USA, 2391 pp., https://doi.org/10.1017/9781009157896, 2021.
IVORI: https://ivori.osug.fr/, last access: 29 November 2023.
Jafari, M., Gouttevin I., Couttet M., Wever N., Michel A., Sharma V., Rossmann L., Maass N., Nicolaus M., and Lehning M.: The Impact of Diffusive Water Vapor Transport on Snow Profiles in Deep and Shallow Snow Covers and on Sea Ice, Front. Earth Sci., 8, 249, https://doi.org/10.3389/feart.2020.00249, 2020.
Krinner, G., Derksen, C., Essery, R., Flanner, M., Hagemann, S., Clark, M., Hall, A., Rott, H., Brutel-Vuilmet, C., Kim, H., Ménard, C. B., Mudryk, L., Thackeray, C., Wang, L., Arduini, G., Balsamo, G., Bartlett, P., Boike, J., Boone, A., Chéruy, F., Colin, J., Cuntz, M., Dai, Y., Decharme, B., Derry, J., Ducharne, A., Dutra, E., Fang, X., Fierz, C., Ghattas, J., Gusev, Y., Haverd, V., Kontu, A., Lafaysse, M., Law, R., Lawrence, D., Li, W., Marke, T., Marks, D., Ménégoz, M., Nasonova, O., Nitta, T., Niwano, M., Pomeroy, J., Raleigh, M. S., Schaedler, G., Semenov, V., Smirnova, T. G., Stacke, T., Strasser, U., Svenson, S., Turkov, D., Wang, T., Wever, N., Yuan, H., Zhou, W., and Zhu, D.: ESM-SnowMIP: assessing snow models and quantifying snow-related climate feedbacks, Geosci. Model Dev., 11, 5027–5049, https://doi.org/10.5194/gmd-11-5027-2018, 2018.
Kojima, K.: Densification of seasonal snow cover, Physics of Snow and Ice: Proceedings, 1, 929–952, 1967.
Kuhn, T. S.: The structure of scientific revolutions, University of Chicago Press, Chicago, 264 pp., ISBN 9780226458120, 1962.
Landström, C.: Tracing uncertainty management through four IPCC Assessment Reports and beyond, in: Cultures of prediction in atmospheric and climate science, edited by: Heymann, M., Gramelsberger, G., and Mahony, M., Routledge, London, 214–230, ISBN 9780367152291, 2017.
Laptander, R., Horstkotte, T., Habeck, J. O., Rasmus, S., Komu, T., Matthes, H., Tømmervik, H., Istomin, K., Eronen, J. T., and Forbes, B. C.: Critical seasonal conditions in the reindeer-herding year: A synopsis of factors and events in Fennoscandia and northwestern Russia, Polar Sci., 39, 101016, https://doi.org/10.1016/j.polar.2023.101016, 2024.
Largeron, C., Dumont, M., Morin, S., Boone, A., Lafaysse, M., Metref, S., Cosme, E., Jonas, T., Winstral, A., and Margulis, S. A.: Toward Snow Cover Estimation in Mountainous Areas Using Modern Data Assimilation Methods: A Review, Front. Earth Sci., 8, 325, https://doi.org/10.3389/feart.2020.00325, 2020.
Latour, B.: Science in Action, Harvard University Press, Cambridge, USA, 288 pp., ISBN 0674792912, 1979.
Levins, R.: The Strategy Of Model Building In Population Biology, Am. Sci., 54, 421–431, 1966.
Lincoln, Y. S.: Emerging criteria for quality in qualitative and interpretive inquiry, Qual. Inq., 1, 275–289, https://doi.org/10.1177/107780049500100301, 1995.
Liston, G. E. and Elder, K.: A distributed snow-evolution modeling system (SnowModel), J. Hydrometeorol., 7, 1259–1276, https://doi.org/10.1175/JHM548.1, 2006.
Liston, G. E., Itkin, P., Stroeve, J., Tschudi, M., Stewart, S., Perdersen, S. H., Reinking, A., and Elder, K.: A Lagrangian snow-evolution system for sea-ice applications (SnowModel-LG): Part I – Model description, J. Geophys. Res., 125, e2019JC015913, https://doi.org/10.1029/2019JC015913, 2020.
Lloyd, E. A.: Model robustness as a confirmatory virtue: The case of climate science, Stud. Hist. Phil. Sci. A, 49, 2015, 58–68, https://doi.org/10.1016/j.shpsa.2014.12.002, 2015.
Longino, H. E.: The Fate of Knowledge, Princeton University Press, Princeton, USA, ISBN 9780691088761, 2002.
Manabe, S.: Climate and the ocean circulation I. The atmospheric circulation and the hydrology of the Earth's surface, Mon. Weather Rev., 97, 739–774, https://doi.org/10.1175/1520-0493(1969)097<0739:CATOC>2.3.CO;2, 1969.
McCune, V.: Academic identities in contemporary higher education: sustaining identities that value teaching, Teach. High. Educ., 26, 20–35, https://doi.org/10.1080/13562517.2019.1632826, 2019.
Menard, C., Essery, R., Krinner, G., Arduini, G., Bartlett, P., Boone, A., Brutel-Vuilmet, C., Burke, E., Cuntz, M., Dai, Y., Decharme, B., Dutra, E., Fang, X., Fierz, C., Gusev, Y., Hagemann, S., Haverd, V., Kim, H., Lafaysse, M., Marke, T., Nasonova, O., Nitta, T., Niwano, M., Pomeroy, J., Schädler, G., Semenov, V., Smirnova, T., Strasser, U., Swenson, S., Turkov, D., Wever, N., and Yuan, H.: Scientific and Human Errors in a Snow Model Intercomparison, B. Am. Meteorol. Soc., 102, E61–E79, 2021.
Morrison, M. A.: The Models Are Alright: A Theory of The Socio-epistemic Landscape of Climate Model Development, PhD Thesis, Indiana University, 2021.
Moss, R. H. and Schneider, S. H.: Uncertainties in the IPCC TAR: Recommendations to lead authors for more consistent assessment and reporting, in: Guidance papers on the cross cutting issues of the Third Assessment Report of the IPCC, edited by: Pachauri, R., Taniguchi, T., and Tanaka, K., World Meteorological Organization, Geneva, 33–51, ISBN 4998090801, 2000.
Nabavi, E.: Computing and Modeling After COVID-19: More Responsible, Less Technical, IEEE T. Technol. Soc., 3, 252–261, 2022.
Parker, W. S.: When Climate Models Agree: The Significance of Robust Model Predictions, Philos. Sci., 78, 579–600. https://doi.org/10.1086/661566, 2011.
Parker, W. S. and Winsberg, E.: Values and evidence: how models make a difference, Eur. J. Philos. Sci., 8, 125–142, https://doi.org/10.1007/s13194-017-0180-6, 2018.
Pope, E. M.: From Participants to Co-Researchers: Methodological Alterations to a Qualitative Case Study, Qual. Rep., 25, 3749–3761, https://doi.org/10.46743/2160-3715/2020.4394, 2020.
Pulkkinen, K., Undorf, S., Bender, F., Wikman-Svahn, P., Doblas-Reyes, F., Flynn, C., Hegerl, G. C., Jönsson, A., Leung, G-K., Roussos, J., Shepherd, T. J., and Thomson, E.: The value of values in climate science, Nat. Clim. Change, 12, 4–6, https://doi.org/10.1038/s41558-021-01238-9, 2022.
Quéno, L., Vionnet, V., Cabot, F., Vrécourt, D., and Dombrowski-Etchevers, I.: Forecasting and modelling ice layer formation on the snowpack due to freezing precipitation in the Pyrenees, Cold Reg. Sci. Technol., 146, 19–31, https://doi.org/10.1016/j.coldregions.2017.11.007., 2018.
Rantanen, M., Karpechko, A. Y., Lipponen, A., Nordling, K, Hyvärinen, O., Ruosteenoja, K., Vihma, T., and Laaksonen, A.: The Arctic has warmed nearly four times faster than the globe since 1979, Commun. Earth Environ., 3, 168, https://doi.org/10.1038/s43247-022-00498-3, 2022.
Rapley, T.: Some Pragmatics of Data Analysis, in: Qualitative Research: Theory, Method & Practice, edited by: Silverman, D., Sage, London, UK, ISBN 9781849204170, 2010.
Rennert, K. J., G. Roe, J. Putkonen, and Bitz, C. M.: Soil Thermal and Ecological Impacts of Rain on Snow Events in the Circumpolar Arctic, J. Climate, 22, 2302–2315, https://doi.org/10.1175/2008JCLI2117.1, 2009.
Rowe, W. E.: Positionality, in: The Sage Encyclopedia of Action Research, edited by: Coghlan, D. and Brydon-Miller, M., Sage, London, United Kingdom, 901 pp., ISBN 978-1-84920-027-1, 2014.
Royer, A., Picard, G., Vargel, C., Langlois, A., Gouttevin, I., and Dumont, M.: Improved Simulation of Arctic Circumpolar Land Area Snow Properties and Soil Temperatures, Front. Earth Sci., 9, 685140, https://doi.org/10.3389/feart.2021.685140, 2021.
Rudner, R.: The Scientist Qua Scientist Makes Value Judgments, Philos. Sci., 20, 1–6, 1953.
Rutter, N., Essery, R., Pomeroy, J., Altimir, N., Andreadis, K., Baker, I., Barr, A., Bartlett, P., Boone, A., Deng, H., Douville, H., Dutra, E., Elder, K., Ellis, C., Feng, X., Gelfan, A., Goodbody, A., Gusev, Y., Gustafsson, D., Hellström, R., Hirabayashi, Y., Hirota, T., Jonas, T., Koren, V., Kuragina, A., Lettenmaier, D., Li, W. P., Luce, C., Martin, E., Nasonova, O., Pumpanen, J., Pyles, R. D., Samuelsson, P., Sandells, M., Schädler, G., Shmakin, A., Smirnova, T. G., Stähli, M., Stöckli, R., Strasser, U., Su, H., Suzuki, K., Takata, K., Tanaka, K., Thompson, E., Vesala, T., Viterbo, P., Wiltshire, A., Xia, K., Xue, Y., and Yamazaki, T.: Evaluation of forest snow processes models (SnowMIP2), J. Geophys. Res.-Atmos., 114, D06111, https://doi.org/10.1029/2008JD011063, 2009.
Saltelli, A., Bammer, G., Bruno, I., Charters, E., Di Fiore, M., Didier, E., Nelson Espeland, W., Kay, J., Lo Piano, S., Mayo, D., Pielke Jr, R., Portaluri, T., Porter, T. M., Puy, A., Rafols, I., Ravetz, J. R., Reinert, E., Sarewitz, D., Stark, P. B., Stirling, A., van der Sluijs, J., and Vineis, P.: Five ways to ensure that models serve society: a manifesto, Nature, 582, 482–484, https://doi.org/10.1038/d41586-020-01812-9, 2020.
Sargeant J.: Qualitative Research Part II: Participants, Analysis, and Quality Assurance, J. Grad. Med. Educ., 4, 1–3, https://doi.org/10.4300/JGME-D-11-00307.1, 2012.
Serreze, M. C., Walsh, J. E., Chapin III, F. S., Osterkamp, T., Dyurgerov, M., Romanovsky, W. C., Oechel, J., Morison, T., Zhang, T., and Barry, R. G.: Observational Evidence of Recent Change in the Northern High-Latitude Environment, Climatic Change, 46, 159–207, https://doi.org/10.1023/A:1005504031923, 2000.
Serreze, M. C., Gustafson, J., Barrett, A. P., Druckenmiller, M. L., Fox, S., Voveris, J., Stroeve, J., Sheffield, B., Forbes, B. C., and Rasmus, S.: Arctic rain on snow events: bridging observations to understand environmental and livelihood impacts, Environ. Res. Lett., 16, 105009, https://doi.org/10.1088/1748-9326/ac269b, 2021.
Skea, C.: Emerging Neoliberal Academic Identities: Looking Beyond Homo economicus, Stud. Philos. Educ., 40, 399–414, https://doi.org/10.1007/s11217-021-09768-7, 2021.
Slater, A. G., Schlosser, C. A., Desborough, C. E., Pitman, A. J., Henderson-Sellers, A., Robock, A., Vinnikov, K. Y., Entin, J., Mitchell, K., Chen, F., Boone, A., Etchevers, P., Habets, F., Noilhan, J., Braden, H., Cox, P. M., de Rosnay, P., Dickinson, R. E., Yang, Z., Dai, Y., Zeng, Q., Duan, Q., Koren, V., Schaake, S., Gedney, N., Gusev, Y. M., Nasonova, O. N., Kim, J., Kowalczyk, E. A., Shmakin, A. B., Smirnova, T. G., Verseghy, D., Wetzel, P., and Xue, Y.: The Representation of Snow in Land Surface Schemes: Results from PILPS 2(d), J. Hydrometeorol., 2, 7–25, https://doi.org/10.1175/1525-7541(2001)002<0007:TROSIL>2.0.CO;2, 2001.
Staddon, S.: Reflecting on personal and professional energy stories in energy demand research, Energy Res. Soc. Sc., 31, 158–163, https://doi.org/10.1016/j.erss.2017.06.013, 2017.
Sturm, M. and Liston, G. E.: Revisiting the Global Seasonal Snow Classification: An Updated Dataset for Earth System Applications, J. Hydrometeorol., 22, 2917–2938, https://doi.org/10.1175/JHM-D-21-0070.1, 2021.
Touzeau, A., Landais, A., Morin, S., Arnaud, L., and Picard, G.: Numerical experiments on vapor diffusion in polar snow and firn and its impact on isotopes using the multi-layer energy balance model Crocus in SURFEX v8.0, Geosci. Model Dev., 11, 2393–2418, https://doi.org/10.5194/gmd-11-2393-2018, 2018.
Tversky, A. and Kahneman, D.: Judgment under uncertainty: Heuristics and biases, Science, 185, 1124–1131, https://doi.org/10.1126/science.185.4157.1124, 1974.
Välimaa, J.: Culture and Identity in Higher Education Research, High. Educ., 36, 119–138, 1998.
Vionnet, V., Brun, E., Morin, S., Boone, A., Faroux, S., Le Moigne, P., Martin, E., and Willemet, J.-M.: The detailed snowpack scheme Crocus and its implementation in SURFEX v7.2, Geosci. Model Dev., 5, 773–791, https://doi.org/10.5194/gmd-5-773-2012, 2012.
Walmsley, L. D.: The strategy of model building in climate science, Synthese, 199, 745–765, https://doi.org/10.1007/s11229-020-02707-y, 2021.
Walsh, J. E.: Intensified warming of the Arctic: Causes and impacts on middle latitudes, Global Planet. Change, 117, 52–63, https://doi.org/10.1016/j.gloplacha.2014.03.003, 2014.
Wever, N., Würzer, S., Fierz, C., and Lehning, M.: Simulating ice layer formation under the presence of preferential flow in layered snowpacks, The Cryosphere, 10, 2731–2744, https://doi.org/10.5194/tc-10-2731-2016, 2016.
Winsberg, E.: Sanctioning Models: The Epistemology of Simulation, Sci. Context, 12, 275–292, https://doi.org/10.1017/S0269889700003422, 1999.
Winsberg, E.: Values and uncertainties in the predictions of global climate models, Kennedy Inst Ethic. J., 22, 111–137, https://doi.org/10.1353/ken.2012.0008, 2012.
Winsberg, E.: What does robustness teach us in climate science: a re-appraisal, Synthese, 198, 5099–5122, https://doi.org/10.1007/s11229-018-01997-7, 2021.
Winter, R.: Academic manager or managed academic? Academic identity schisms in higher education, J. High Educ. Policy M., 31, 121–131, https://doi.org/10.1080/13600800902825835, 2009.
Zhang, T., Osterkamp, T. E., and Stamnes, K.: Influence of the Depth Hoar Layer of the Seasonal Snow Cover on the Ground Thermal Regime, Water Resour. Res., 32, 2075–2086, https://doi.org/10.1029/96WR00996, 1996.