the Creative Commons Attribution 4.0 License.
the Creative Commons Attribution 4.0 License.
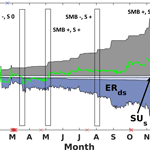
How does the ice sheet surface mass balance relate to snowfall? Insights from a ground-based precipitation radar in East Antarctica
Niels Souverijns
Alexandra Gossart
Irina V. Gorodetskaya
Stef Lhermitte
Alexander Mangold
Quentin Laffineur
Andy Delcloo
Nicole P. M. van Lipzig
Local surface mass balance (SMB) measurements are crucial for understanding changes in the total mass of the Antarctic Ice Sheet, including its contribution to sea level rise. Despite continuous attempts to decipher mechanisms controlling the local and regional SMB, a clear understanding of the separate components is still lacking, while snowfall measurements are almost absent. In this study, the different terms of the SMB are quantified at the Princess Elisabeth (PE) station in Dronning Maud Land, East Antarctica. Furthermore, the relationship between snowfall and accumulation at the surface is investigated. To achieve this, a unique collocated set of ground-based and in situ remote sensing instrumentation (Micro Rain Radar, ceilometer, automatic weather station, among others) was set up and operated for a time period of 37 months. Snowfall originates mainly from moist and warm air advected from lower latitudes associated with cyclone activity. However, snowfall events are not always associated with accumulation. During 38 % of the observed snowfall cases, the freshly fallen snow is ablated by the wind during the course of the event. Generally, snow storms of longer duration and larger spatial extent have a higher chance of resulting in accumulation on a local scale, while shorter events usually result in ablation (on average 17 and 12 h respectively). A large part of the accumulation at the station takes place when preceding snowfall events were occurring in synoptic upstream areas. This fresh snow is easily picked up and transported in shallow drifting snow layers over tens of kilometres, even when wind speeds are relatively low (< 7 ms−1). Ablation events are mainly related to katabatic winds originating from the Antarctic plateau and the mountain ranges in the south. These dry winds are able to remove snow and lead to a decrease in the local SMB. This work highlights that the local SMB is strongly influenced by synoptic upstream conditions.
- Article
(3045 KB) - Full-text XML
-
Supplement
(251 KB) - BibTeX
- EndNote
The Antarctic Ice Sheet (AIS), being currently the largest ice body on earth, is an important regulator of present and future sea level and the global water cycle (Previdi and Polvani, 2016; Vaughan et al., 2013). In order to assess its contribution to sea level rise, understanding the surface mass balance (SMB) of the AIS is of crucial importance. Climate models (potentially coupled to an ocean or ice sheet model) play an important role in understanding and quantifying the contribution of the AIS to sea level (rise) (DeConto and Pollard, 2016; Gregory and Huybrechts, 2006; Ligtenberg et al., 2013; Rignot et al., 2011). Yet, despite their importance, they simplify the different components of the SMB. It has been noted by several authors that, in order to fully understand the impact of the AIS on future sea level rise, information on the individual components of the present-day AIS SMB, including snowfall measurements, is indispensable (Agosta et al., 2013; Gorodetskaya et al., 2015; Grazioli et al., 2017a, b; Lenaerts et al., 2016; Rignot et al., 2011; Shepherd et al., 2012; Souverijns et al., 2017; van Lipzig et al., 2002). A lack of observations of the present behaviour of these different components prevents the validation of climate models. As such, most studies rely on stake and other measurements that register only the total change in snow height (Agosta et al., 2012; Eisen et al., 2008; Favier et al., 2013, 2017; Genthon et al., 2005; Magand et al., 2007; Vaughan et al., 1999; Wang et al., 2016; van Lipzig et al., 2004). Gorodetskaya et al. (2015) quantified the different terms of the local SMB in a systematic way for the Princess Elisabeth (PE) station and determined snowfall from radar measurements. In this study we focus on the relationship and interactions between snowfall and accumulation in order to understand and assess the local SMB components in more detail.
The local SMB is influenced by several processes and can be expressed as the sum of snowfall (S), surface sublimation (SUs), drifting snow sublimation (SUds), surface melt (ME) and wind-induced accumulation or ablation by drifting and blowing snow (ERds) (van den Broeke et al., 2004):
Although previous studies proposed a variety of techniques to calculate the local SMB, most are confined to measuring the sum of all components (net height change at the surface) using stake measurements and ice cores (Favier et al., 2013; Rotschky et al., 2007; Thomas et al., 2017; Vaughan et al., 1999; Wang et al., 2016) or one-dimensional snow models (Groot Zwaaftink et al., 2013). Generally, separately measuring any of the components of the local SMB is considered a difficult task (Amory et al., 2017; Eisen et al., 2008; Gorodetskaya et al., 2015; Grazioli et al., 2017a; King and Turner, 1997; Vaughan et al., 1999; van Lipzig et al., 2002; van den Broeke et al., 2004).
Traditional snowfall measurements using precipitation gauges are inhibited by high wind speeds over the AIS leading to undercatchment, whereas ice cores and stake measurements are poor proxies for snowfall, as they are affected by other components of the local SMB, e.g. blowing snow (Bromwich, 1988; Das et al., 2013; Groot Zwaaftink et al., 2013; van den Broeke et al., 2004). Again this indicates the importance of separately measuring the snowfall component and the existence of a non-linear relationship between the local SMB and snowfall amounts. In recent years, remote sensing applications offer new possibilities regarding the determination of snowfall amounts on remote locations such as the AIS. A first estimate of snowfall rates over the AIS was derived from the CloudSat satellite (Palerme et al., 2014). Its low overpass frequency, narrow swath width and ground clutter are limiting factors (Battaglia and Delanoë, 2013; Casella et al., 2017; Maahn et al., 2014). More locally, the operation of ground-based precipitation radars has proven to be an efficient way to detect snowfall over the AIS at several locations (Gorodetskaya et al., 2015; Grazioli et al., 2017a, b; Souverijns et al., 2017).
Wind-induced accumulation and ablation by blowing or drifting snow over the AIS has a significant impact on the local SMB (Bromwich et al., 2004; Palm et al., 2017; Scarchilli et al., 2010). The ERds component can, however, only be measured accurately using a network of snowdrift instrumentation (Amory et al., 2017; Barral et al., 2014; Leonard et al., 2012; Nishimura and Ishimaru, 2012; Nishimura and Nemoto, 2005; Trouvilliez et al., 2014) and it is difficult to take into account in Antarctic-wide SMB estimates (Das et al., 2013; Genthon and Krinner, 2001; van den Broeke et al., 2004). Atmospheric models have been adapted to take these processes into account (Amory et al., 2015; Gallée et al., 2013; Lenaerts and van den Broeke, 2012a) but depend on parameterisations. Thresholds for drifting snow initiation are very variable and depend on both the meteorological conditions and the snow particle characteristics (Bintanja and Reijmer, 2001; Li and Pomeroy, 1997). Blowing and drifting snow can shape the relief of the ice sheet on a small spatial scale (i.e. sastrugi formation), local scale (i.e. barchan dunes) or kilometre scale (i.e. blue ice zones) (Amory et al., 2017). These processes potentially result in a large variability in snow height on the decametre scale (Libois et al., 2014). Due to the difficulty in measuring ERds, it is often considered the leftover term in the local SMB over the AIS (Gorodetskaya et al., 2015). Recently, some new remote sensing techniques have been developed to detect ERds based on satellite-borne lidar and ground-based ceilometer measurements, adding to the understanding of the ERds component in the SMB (Gossart et al., 2017; Palm et al., 2011).
Both surface and drifting snow sublimation have been quantified for the PE station (Thiery et al., 2012). On a local scale, the significance of the processes can be fairly large (Bliss et al., 2011; Gorodetskaya et al., 2015; Grazioli et al., 2017b; King et al., 2001). For the PE station, sublimation was found to remove 10 % of the total precipitation (Thiery et al., 2012). In this study, the focus is mainly on the relationship between accumulation, ablation and snowfall. The sublimation terms, together with melt, which is only relevant at coastal areas and ice shelves (Lenaerts et al., 2017), are therefore only quantified and not investigated in great depth.
Snow height (changes) can be measured by an automatic weather station (AWS), which is equipped with an acoustic height ranger (van den Broeke et al., 2004). The main advantage of the AWS is that it combines snow height and meteorological observations.
Synoptic- and meso-scale meteorology have a strong impact on the local SMB. For example, cyclone activity in the Antarctic circumpolar trough (5–70∘ S) results in moist air penetrating into the atmosphere above the AIS. This leads to snowfall events and high wind speeds at both coastal and inland locations (Gorodetskaya et al., 2013; Hirasawa et al., 2013; King and Turner, 1997; Schlosser et al., 2010; Simmonds et al., 2003). If large amounts of moisture are transported, these events are identified as atmospheric rivers having a profound impact on the local SMB (Gorodetskaya et al., 2014). Nevertheless, the independent measurement of the snowfall component and the local SMB over the AIS are limited. Gorodetskaya et al. (2015) showed that snowfall events at the PE station do not necessarily contribute to accumulation or an increase in snow height.
This study uses 37 months of independent snowfall and SMB measurements in order to statistically assess the relationship between snowfall and the local SMB. This analysis therefore determines the representativeness of stake measurements as a proxy for snowfall on a local scale and defines the frequency and conditions when snowfall leads to accumulation or ablation. In addition, changes in the local SMB take place without snowfall. A thorough analysis of meteorological conditions during these events adds to the understanding of the behaviour of the ERds component and its impact on the evolution of the local SMB over the AIS.
2.1 Site description and instrumentation
Long-term measurements of the individual components of the SMB over the AIS are scarce due to its harsh environment and difficult accessibility. To tackle this problem, a limited-maintenance and low-power meteorological observatory was established at the PE station in 2009 (Gorodetskaya et al., 2015). The PE station is located in the escarpment zone of the East Antarctic plateau ( S, E; 1392 m above sea level), 173 km from the coast, in Dronning Maud Land, north of the Sør Rondane Mountains (Fig. 1). Meteorological conditions at the station are influenced by both synoptic weather conditions and katabatic winds. A detailed description of the site can be found in Pattyn et al. (2010) and Gorodetskaya et al. (2013). The main aim of this meteorological observatory is to collect a comprehensive database of meteorology, radiative fluxes, snow height changes and cloud and precipitation properties Gorodetskaya et al. (2015).
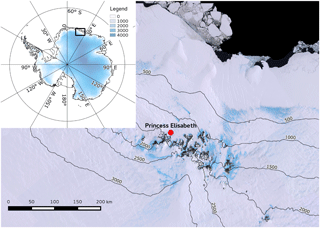
Figure 1Location of the meteorological observatory at the Belgian Princess Elisabeth station, north of the Sør Rondane Mountains. Source: Landsat Image Mosaic of Antarctica (LIMA) Project, http://lima.usgs.gov/ (last access: 8 June 2018). Colours in the inset and contours on the main figure denote topography height (metres).
Snowfall measurements have been recorded since 2010 by using a vertically pointing Micro Rain Radar-2 (MRR) operating at a frequency of 24 GHz (Klugmann et al., 1996; Peters et al., 2002). The potential of millimetre radars to efficiently detect snowfall has been shown by Matrosov et al. (2008) and has been evaluated specifically for this type of radar by Kneifel et al. (2011). As the MRR was originally developed for rain observations, operational MRR procedures used to derive standard radar variables like effective reflectivity factor (Ze) or mean Doppler velocity had to be modified for snowfall using the methodology of Maahn and Kollias (2012). In order to obtain reliable estimates of snowfall rates and their uncertainty, an optical disdrometer (Newman et al., 2009) was installed at the PE station. This instrument is based on a high-speed camera and is able to obtain detailed information about snowflake microphysics (i.e. the particle size distribution). A correction for the horizontal and vertical displacement of snowfall between the MRR data acquisition level and the surface has been applied (Souverijns et al., 2017; Wood, 2011). Using this information, a relationship between radar reflectivity measured by the MRR and snowfall rates was obtained: Ze = 18 SR1.1. Furthermore, a constraint on the uncertainty of the resulting snowfall rates was obtained [−59 + 60 %] (10th–90th percentile) (Souverijns et al., 2017), which is a considerable reduction compared to earlier snowfall rate estimates at the PE station that were retrieved without any information on the snow particle microphysical characteristics (Gorodetskaya et al., 2015).
The net local SMB is calculated based on snow height measurements of an AWS and yearly snow density records (see Sect. 1). The AWS was installed in February 2009 and is located approximately 300 m east of the station (and the MRR) on the windward side of Utsteinen ridge (see Sect. 2.1) in a zone of little accumulation (Pattyn et al., 2010). The AWS records meteorological variables, such as air temperature, pressure, wind speed and direction, relative humidity and radiative fluxes at 2 m above the surface. These measurements are processed following Gorodetskaya et al. (2013). Wind speed and direction are recorded with an anemometer at the top of the AWS. Humidity is recorded with respect to water at the top of the AWS and is converted to humidity with respect to ice using the conversion of Anderson (1994). Broadband radiative fluxes are measured using pyranometers and pyrgeometers. All the above parameters are measured with 6 min resolution and averaged to hourly means. Furthermore, the AWS is equipped with an acoustic height sensor, which measures snow height changes once an hour with an accuracy of 1 cm on an hourly time resolution. A running mean of 24 h is applied to erase the majority of the short-term decametre-scale variability due to sastrugi movement (Libois et al., 2014). Nevertheless, few events may still be related to artefacts caused by the motion of sastrugi. As such, temporary peaks (i.e. with a strong decay within 48 h) in the snow height records are excluded from the analysis. In January 2016, a new AWS was set up to replace the one installed in 2009, which was able to measure snow height changes more accurately. A detailed overview of the specifications of the old AWS, including its uncertainty, can be found in Gorodetskaya et al. (2013) and of the new AWS found in the Supplement (Table S1). Each year, the average density of the snow that has accumulated in the past year is calculated from snow pit measurements at the PE station. Using these average yearly densities (varying between 309 and 375 kg m−3), snow height changes are converted to changes in the local SMB (water equivalent) (Gorodetskaya et al., 2013, 2015). Densification due to snow aging is not taken into account.
Ablation or accumulation resulting in changes in the SMB generally occur in blowing snow layers with a distinct vertical extent. Recently, an algorithm for the detection of blowing snow by the use of a ceilometer was developed (Gossart et al., 2017). Ceilometers are ground-based lidars originally developed to detect cloud-base height (Gossart et al., 2017; Van Tricht et al., 2014). This instrument is able to detect blowing snow particles based on the backscatter signal and is operational during all types of weather conditions (cloudy, snowfall conditions and at night). As such, it complements satellite retrievals of blowing snow from the CALIPSO satellite (Palm et al., 2011). The minimum height of the blowing snow layer that is detected by the ceilometer equals 30 m at the PE station. The temporal resolution equals 15 s, while the vertical height resolution is 10 m. The quantification of blowing snow particle density, shape or number from the ceilometer attenuated backscatter signal is very uncertain (Wiegner et al., 2014). As such, this study is limited to the determination of the blowing snow frequency. Results show that 78 % of the detected events by the ceilometer are in agreement with visual observations at Neumayer III station and that blowing snow occurrence strongly depends on fresh snow availability in addition to wind speed (Gossart et al., 2017).
Surface and snowdrift sublimation are quantified using the approach of Thiery et al. (2012), which determine their magnitude based on parameterisations using meteorological observations such as relative humidity and wind speed (Bintanja and Reijmer, 2001; Déry and Yau, 2001). The necessary data are provided by the AWS. Based on the AWS accuracy, the uncertainty of the surface sublimation term equals approximately 47 %, while for snowdrift sublimation, it equals 20 % based on differences between parameterisations (both 2 standard deviations) (Gorodetskaya et al., 2015).
The sampling period of our study is limited to time periods in which both the AWS and MRR were operational between 2010 and 2016. These time frames are almost always restricted to austral summer months in which the station is manned (Fig. S1 in the Supplement). Data acquisition during the austral winter season is often hampered by power failures at the PE station. The MRR was installed in the season 2009–2010 and a total record of seven austral summer seasons is available for analysis. For austral winter, only one season is available in which both MRR and AWS were operating continuously, i.e. 2012. Apart from this, in January 2015, a problem with the wind vane of the AWS was detected. This problem persisted until December 2015, leading to erroneous wind direction measurements. The year 2015 is therefore also discarded from our analysis. In the end, 37 months of collocated precipitation, total SMB and meteorological data are available at the same location, which is unprecedented for the Antarctic region.
2.2 Snowfall, accumulation and ablation events
The local SMB is composed of the sum of different components (Eq. 1). Snowfall amounts are measured by a Micro Rain Radar, while the two sublimation terms are calculated based on meteorological data from the AWS (see Sect. 2.1). The ERds term is not measured directly at the PE station. Nevertheless, since the total SMB can be deduced from the AWS (see Sect. 1), ERds can be calculated as the residual term after inverting Eq. (1) (Gorodetskaya et al., 2015). Its uncertainty is therefore based on the uncertainty of all other components of the local SMB (Gorodetskaya et al., 2015) and is mainly determined by the uncertainty of the snowfall component at the PE station, as this term has the highest uncertainty range (see Sect 2.1) and absolute contribution to the SMB.
Snowfall is generally considered the main positive term of the local SMB (Davis et al., 2005). One would therefore expect that snowfall results in accumulation or an increase in height. However, this is not necessarily true locally over the AIS, where snowfall often coincides with strong winds. Consequently, snowfall events with strong redistribution can result in a net snow removal on a local scale. As stated in Sect. 1, accumulation and ablation also occur during non-precipitating conditions. In order to define the conditions for these episodes and to assess a relationship between snowfall and accumulation, different types of events were discriminated based on local SMB measurements from the AWS and snowfall records from the MRR:
-
accumulation during snowfall (SMB +, S +)
-
ablation during snowfall (SMB −, S +)
-
accumulation without snowfall (SMB +, S 0)
-
ablation without snowfall (SMB −, S 0).
An event is confined to the period between the start of snowfall, accumulation or ablation and the moment at which no more snowfall, accumulation or ablation is observed. The time step and duration of an event have an hourly time resolution. Snowfall events are defined as exceeding the threshold of 1 mm w.e. during the continuous duration of snowfall measured by the MRR. As the AWS measures snow height changes with a sensitivity of 1 cm, an increase in surface height larger than 1 cm over the duration of an event is considered to be an accumulation event, while an ablation event occurs when a decrease in surface height of more than 1 cm is recorded.
2.3 Local- and large-scale meteorology
In order to understand the mechanisms resulting in snowfall and wind-induced accumulation or ablation, the meteorological conditions of the four types of events defined in Sect. 2.2 are evaluated. For all members within these four types of events, average meteorological conditions are calculated. Wind speed and direction, humidity and radiative fluxes are obtained from the AWS. Snowfall amounts are obtained by applying a relation converting radar reflectivity measurements from the MRR to snowfall rates specifically developed for the PE station using observations from an optical disdrometer (see Sect. 2.1).
Further, the temporal extent of the cloud system is investigated using two different data products. Firstly, cloudy conditions are estimated based on long-wave downward radiation measurements of the AWS. For this, the clear-sky index based on the methodology of Marty and Philipona (2000) and Dürr and Philipona (2004) is used, which calculates the ratio between the apparent and clear-sky long-wave downward radiation. The apparent long-wave downward radiation is calculated using observations of the AWS. For the clear-sky long-wave downward radiation, local coefficients (see Eq. 3 and Sect. 3 in Marty and Philipona, 2000) are optimised for the PE station by comparing calculated clear-sky and observed long-wave downward radiation during visually detected cloud-free conditions (based on camera images and ceilometer data). As a result, based on the ratio of apparent and clear-sky long-wave downward radiation, one can discriminate between cloudy and clear-sky conditions. Secondly, the temporal extent of the cloud system at the PE station was estimated from ERA-Interim (Dee et al., 2011). ERA-Interim is generally considered the best reanalysis product over Antarctica (Bracegirdle and Marshall, 2012). Cloudy conditions over the station are defined as having a total cloud fraction of more than 95 % in the pixel over the PE station. Sensitivity studies have been executed on this threshold (varying between 80 and 100 %) and account for only small relative differences not influencing the general conclusions significantly. Ceilometer cloud observations are not used to determine the temporal extent of the cloud system, as the collocated time period of corresponding measurements is insufficient.
Apart from these local meteorological variables, an analysis of the large-scale circulation over Dronning Maud Land (including a large part of the Southern Ocean) was performed. A cluster analysis was applied to 500 hPa geopotential ERA-Interim reanalysis data covering the period of observations at the PE station (2010–2016). This gives an overview of the climatology and the typical circulation that is present over the region.
Several algorithms are available for a cluster analysis (Philipp et al., 2010). In recent studies, thorough evaluations were performed for each of these algorithms, and the best performance regarding circulation clustering was indicated for optimisation algorithms over different parts of the world (Beck and Philipp, 2010; Casado et al., 2010; Huth et al., 2008; Souverijns et al., 2016). From these optimisation algorithms, the simulated annealing and diversified randomisation (SANDRA) algorithm was chosen, which is based on k-means clustering (Philipp et al., 2007) as it is adequate for different applications over the world regarding circulation clustering (Beck and Philipp, 2010; Casado et al., 2010; Huth et al., 2008; Souverijns et al., 2016). In addition to choosing the classification algorithm, it is necessary to define the total number of circulation patterns that cover the full climatology over Dronning Maud Land. For a range between 2 and 27 total circulation patterns, the quality of the SANDRA algorithm is tested. Using the fast silhouette index, the ability of the SANDRA algorithm to maximise the separability between the members of different circulation patterns while minimising the variances within each circulation pattern was investigated (Rousseeuw, 1987). In this study, a total of six circulation patterns was selected. The fast silhouette index indicates a local minimum value as a further increase in the total number of circulation patterns shows no significant improvement (i.e. decrease) regarding the classification skill (Fig. S2). Based on this climatology, the dominant circulation present during the four types of events resulting in a change in the local SMB defined in Sect. 2.2 are determined. This cluster analysis method has similar results to the local meteorological regimes defined by Gorodetskaya et al. (2013) but increases insight into the large-scale circulation patterns and source regions of air advection.
In addition to this cluster analysis, a back-trajectory analysis was performed for all individual events in order to get insights into the origin and history of the air masses. For this, the FLEXPART software, a Lagrangian transport and dispersion model which makes use of ERA-Interim data is used at a spatial resolution of 0.75∘ × 0.75∘ (Stohl and Seibert, 1998; Stohl et al., 1995).
Changes in snow height are measured by the AWS at a single location, 300 m east of the PE station. However, snow height is highly variable in space and time over the AIS (Eisen et al., 2008; Frezzotti et al., 2005). Observations from the Antarctic plateau show large decametre-scale variability in snow heights due to the formation and movement of sastrugi, highly impacting the snow height record (Libois et al., 2014). In this study, the focus is mainly on accumulation and ablation over larger spatial areas. In order to validate the spatial scale of accumulation and ablation, snow erosion output from the state-of-the-art high-resolution (5.5 km grid) RACMO2.3 simulation is analysed (Lenaerts et al., 2014, 2017; van Wessem et al., 2014), which is coupled to a snow model including drifting snow (Lenaerts and van den Broeke, 2012a). The RACMO2.3 simulation is driven by ERA-Interim and adequately simulates both climatological and meteorological conditions near the surface (Lenaerts et al., 2014). By selecting the time periods during which accumulation and ablation occurred in the RACMO2.3 simulation, an overview of snow erosion spatial structures during these events is achieved.
3.1 Local surface mass balance
The four components of the local SMB, snowfall, surface sublimation, drifting snow sublimation and wind-induced accumulation and ablation, are converted to water equivalent values (Gorodetskaya et al., 2013, 2015). When snow height measurements are available the local SMB can be closed (treating ERds as a residual term; see Eq. 1). For the year 2012, both the AWS and MRR operated year-round, allowing the evolution of the different components through time to be visualised in a cumulative way (Fig. 2; see also Gorodetskaya et al., 2015). Snowfall (S) is identified as a strictly positive term and the stepwise function indicates that the total yearly precipitation amount is strongly influenced by several intense precipitation events per year. The local SMB is denoted by the green line and shows several peaks in both the upper and lower directions (indicating distinct accumulation and ablation events respectively). These events occur both with or without snowfall and allow for easy identification of the individual accumulation and ablation events. Furthermore, it can also be noted that surface and drifting snow sublimation (SUs and SUds respectively) are both ablation terms. The rest of the study ignores these two components, as in this study we focus on the contribution of the ERds term.
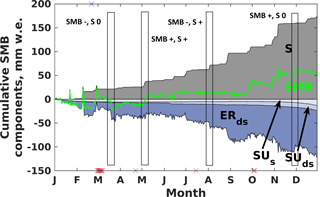
Figure 2Cumulative daily surface mass balance components during 2012 at the Princess Elisabeth station: snowfall (S), surface sublimation (SUs), drifting snow sublimation (SUds), wind-induced accumulation and ablation (ERds), and accumulation and ablation deduced from measured snow height changes since 1 January 2012 (SMB; adapted from Gorodetskaya et al., 2015). SUs and SUds are plotted as ablation terms. ERds is calculated and plotted as a residual term by inverting Eq. (1). Red crosses at the bottom indicate days of missing MRR data, while blue crosses at the top denote missing AWS data. Letters on the x axis mark the first day of each month. Examples of the four types of events defined in Sect. 2.2 are indicated with black rectangles.
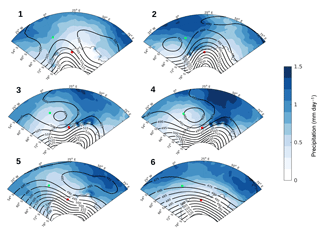
Figure 3Weather atlas illustrating the circulation climatology over Dronning Maud Land. Thick lines denote the 500 hPa geopotential fields, while blue colours show average precipitation amounts linked to this circulation. The red dot indicates the location of the Princess Elisabeth station, while the green dot denotes the location over the ocean used for calculating the pressure gradient (Sect. 3.3). The Antarctic circumpolar trough is identified. The depiction sequence of the circulation types describes the typical west–east movement of the cyclones.
For each of the four types of events defined in Sect. 2.2 one example is highlighted in Fig. 2. It can be seen that some snowfall events account for accumulation (SMB +, S +), but that this is not strictly the case (Gorodetskaya et al., 2015). Wind can also remove mass from the site during a snowfall event, leading to a net removal of mass (SMB −, S +). Additionally, even during time periods without any snowfall, snow height varies continuously and several accumulation (SMB +, S 0) and ablation (SMB −, S 0) events can be identified without the presence of snowfall.
3.2 Large-scale meteorology
Using the SANDRA circulation pattern classification algorithm, it is possible to define the climatology of large-scale circulation over Dronning Maud Land and the nearby Southern Ocean (Fig. 3). Large-scale circulation over Dronning Maud Land is typically characterised by an anticyclone close to the pole and cyclones at latitudes between 50 and 70∘ S, north or near the coast of the AIS. These low-pressure systems form a ring around the Antarctic continent (Antarctic circumpolar trough), resulting in a high variability in meteorological regimes at the coastal areas of the AIS (Gorodetskaya et al., 2013; Hirasawa et al., 2013; King and Turner, 1997; König-Langlo and Loose, 2006; van den Broeke and van Lipzig, 2003). The sequence of circulation patterns depicted in Fig. 3 is typical for the Antarctic region (Simmonds et al., 2003). In circulation type 1 (C1) the Antarctic circumpolar trough is clearly visible, showing a high-pressure bulge over Dronning Maud Land. To the east and west, we can see two low-pressure cells. In C2–C5 the typical movement of a low-pressure cyclone from the west to the east is depicted, largely influencing meteorological conditions at the surface. Apart from the circulation, the average precipitation amounts associated with each circulation pattern are shown (Fig. 3). Precipitation estimates are obtained from ERA-Interim, currently considered the best Antarctic-wide precipitation product, but are still strongly biased (Bromwich et al., 2011). Misrepresentations of large-scale atmospheric flow and precipitation might therefore impact the results. A strong link between the locations of the cyclone and precipitation is present. The cyclone is capable of transporting marine air towards the AIS. These marine air masses have the potential to take up moisture, potentially resulting in precipitation at the continent. A detailed description of each circulation pattern can be retrieved from the Supplement.
3.3 Snowfall
Snowfall is the main positive contributor to the local SMB. During the observational period 2010–2016, a total of 50 independent snowfall episodes were detected resulting in at least 1 mm w.e. at the PE station. Snowfall events are characterised by high wind speeds originating mainly from the east-north-east (ENE; Table 1 and Fig. 4a). Only a limited number of snowfall events correspond to near-surface winds from other directions. This complies with the literature, stating that moist air and precipitation are transported via cyclone activity in the Antarctic circumpolar trough towards the AIS (Sect. 1) and is also confirmed by our cluster analysis. A fraction of more than 70 % of all snowfall events coincide with cyclone activity (Fig. 4b). Over the AIS, these winds are slanted towards the east at the surface due to friction and the location of the PE station in the escarpment zone (Fig. 1).
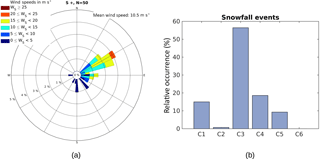
Figure 4(a) Wind rose showing the hourly speed and direction of the wind recorded by the AWS during snowfall events detected by the MRR. N denotes the total number of snowfall events during the observation period. (b) Frequency of occurrence of the circulation patterns depicted in Fig. 3 for snowfall events detected by the MRR.
In order to validate the relationship between large snowfall events and these meteorological conditions, interactions between the transport capacity of the cyclone, the origin of the air mass and the amount of snowfall that is recorded at the PE station are investigated. The transport capacity of the cyclone is parameterised by an index that we constructed based on the pressure difference between the PE station and the typical location of the trough north-west of the station (0∘ E, 62∘ S, Fig. 3) as these cyclones result in the highest snowfall amounts at the PE station. Larger values for this index indicate stronger pressure gradients and wind speeds. The origin of the air masses (5 days prior to the event) are deduced from back trajectories arriving at the PE station at altitudes below 3000 m a.s.l. The most intense snowfall events (> 5 mm w.e. of snowfall per event) are associated with air masses typically originating from areas north of 50∘ N, taking up moisture close to the oceanic surface, and are generally lifted upwards when reaching the AIS continental margin (An example is given in Fig. 5a). A significant relationship between the transport capacity of the cyclone, the origin of the air mass and the amount of snowfall is observed (Fig. 5b). If no cyclone is present NW of the PE station (i.e. negative pressure difference values; left side of the graph), snowfall amounts are generally low. This corresponds to events that are not related to winds originating from the ENE (Fig. 4a) and confirms that snowfall amounts that are not related directly to cyclones are low. If a cyclone is present, a tendency for higher snowfall amounts during larger pressure gradients is present (significant at the 0.05 confidence level). Furthermore, during these conditions, air masses originate from more northern areas (Fig. 5b). As a conclusion, when the cyclone or trough is more developed and high-pressure blocking is present NE of the PE station, moisture from more northern areas is able to be transported, leading to higher snowfall rates at the station. If very high snowfall amounts are observed during this synoptic situation, moisture transport is likely related to atmospheric rivers (Gorodetskaya et al., 2014).
Table 1Average meteorological statistics for the four types of events stated in Sect. 2.2. Only events during coinciding measurements of the AWS and MRR are included. LWcs denotes the long-wave incoming radiation during clear-sky conditions, while SWcs is the short-wave incoming radiation during clear-sky conditions.
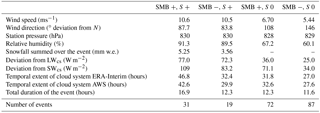
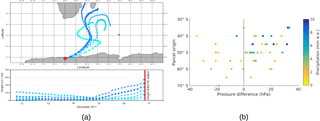
Figure 5(a) Back trajectories for the snowfall event on 17 December 2011 at the Princess Elisabeth station for different heights (start at 06:00 UTC). The red dot (top panel) denotes the location of the Princess Elisabeth station, while the red line (bottom panel) shows the time the snowfall event arrived at the station. (b) Relationship between the transport capacity of the cyclones (based on the pressure difference between the Princess Elisabeth station and 0∘ E, 62∘ S), the origin of the air masses (based on the origin of the back trajectories arriving at the Princess Elisabeth station at altitudes below 3000 m a.s.l. five days prior to the event) and the total amount of snowfall for all detected events at the Princess Elisabeth station during 2010–2016. Positive values for the pressure difference denote the presence of a cyclone north-west of the Princess Elisabeth station, while negative values indicate the absence of a cyclone.
From the total number of snowfall events, 31 resulted in accumulation (62 %, attaining on average for 5.3 mm w.e. of snowfall), while 19 led to ablation (38 %, attaining on average for 3.6 mm w.e. of snowfall; Table 1). Regarding the basic meteorological variables obtained by the AWS, no large differences between the events are observed. Note especially that the mean wind speed is the same. Only the duration of the event and the temporal extent of the cloud structure show clear distinctions regarding their mean values. Accumulation events (SMB +, S +) have a longer duration compared to ablation events (SMB −, S +), which is significant at the 0.1 level (Fig. 6). Furthermore, the persistence of the cloud structure shows a notable difference for both methods described in Sect. 2.3 at the 0.05 level (Table 1 and Fig. 6). It is noted that relatively speaking, the temporal extent of the cloud structure equals on average 260 % of the duration of snowfall for both the accumulation and ablation events using both methods. It is therefore concluded that accumulation events are characterised by a larger temporal extent of the event compared to ablation, which is deduced from both the duration of snowfall and the temporal extent of the cloud structure.
Snowfall events are generally characterised by high wind speeds originating from oceanic areas (ENE at the PE station) and redistribute snow continuously during an event, including a mixture of blowing snow and precipitation particles (Nishimura and Nemoto, 2005). If a snow storm has a large temporal extent, fresh snow precipitates over areas with a large spatial coverage. Therefore, there is a higher chance that snow removed from the PE station is replaced by fresh snow from other areas located upstream. For a shorter event or cloud system, there is a lower probability that snow from other locations replaces snow that is blown away from the station. As such, the larger the cloud system or the duration of the event, the higher the chance that snowfall events result in accumulation on a local scale, rather than ablation. In order to validate this hypothesis, information about the scale of snow displacement is necessary (see Sect. 3.4).
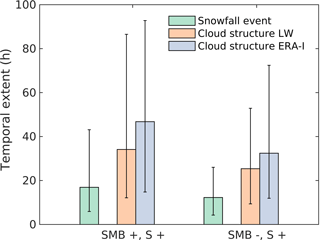
Figure 6Duration (green) and the temporal extent of the cloud structure (red and blue) for snowfall events resulting in accumulation (SMB +, S +) and ablation (SMB −, S +) (hours). Columns denote the average value, while the error bars denote the 25th and 75th percentile based on all events.
Redistribution of snow at the surface by high winds is caused by blowing snow (drifting snow if the height of the snow displacement layer is lower than 2 m). By using information from the ceilometer at the PE station, it is possible to detect blowing snow layers with a minimum vertical extent of 30 m (Gossart et al., 2017). The concurrence of blowing snow and snowfall events is analysed. For snowfall events resulting in accumulation, a blowing snow layer is detected in 95 % of the cases, while for snowfall events resulting in ablation, this concurrence equals 88 %. In total, all 50 snowfall events resulted in changes of 542 cm in height (both accumulation and ablation; detected by the AWS). During the periods in which the ceilometer recorded blowing snow, the AWS detected changes of 486 cm in height (i.e. the sum of all height changes during events for which blowing snow was detected), showing the potential of the ceilometer to detect blowing snow during snowfall events.
Over all snowfall events, a total amount of 230 mm w.e. was recorded. Based on yearly density measurements in the uppermost layers of the snow pack, yearly average values between 309 and 395 kg m−3 are reported. This results in 58–75 cm accumulation at the surface if no other processes are at play. This is significantly lower than the total height changes recorded by the AWS during all snowfall events (542 cm). This indicates the importance of the continuous movement of snow during snowfall events. (Punctual) accumulation records are therefore not advised to be used as a proxy for snowfall over East Antarctica. A direct relationship between snowfall and accumulation is, however, difficult to assess, but snowfall events of a longer duration have a higher chance of resulting in accumulation.
3.4 Wind-induced accumulation and ablation
In the previous section, synoptic events with snowfall lead to both accumulation and ablation, depending on the duration of the event (i.e. the duration of snowfall and temporal extent of the cloud structure). However, snow displacement events also occur without the presence of snowfall. The meteorological conditions during these non-precipitating events are clearly different from snowfall events (Table 1), with clear differences in wind patterns (compare Figs. 7 and 4a). Firstly, the mean wind speed is much lower for non-precipitating events compared to snowfall events, while secondly, two dominant wind directions are detected. Thirdly, in contrast with snowfall events, clear differences between the wind patterns of accumulation and ablation events are visible (Fig. 7). On the one hand there is a dominant ENE flow during accumulation events (SMB +, S 0), while southerly flow is more commonly related to ablation events (SMB −, S 0).
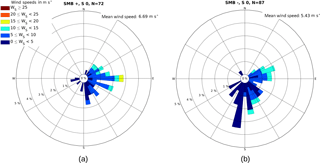
Figure 7Wind speed and direction for the two events resulting in wind-induced accumulation (a) and ablation (b) without snowfall. N denotes the total number of events during our observation period.
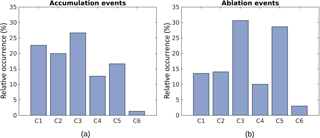
Figure 8Frequency of occurrence of the circulation patterns depicted in Fig. 3 for wind-induced accumulation (a) and ablation (b) without snowfall.
During accumulation events without snowfall (SMB +, S 0), one of the dominant wind directions is from the ENE, similarly to snowfall events. However, wind speeds are much lower (Fig. 7a). Approximately 40 % of all accumulation events occur during a similar circulation to snowfall events (circulation patterns 3 and 4 in Fig. 8a).
To assess the large-scale spatial patterns of snowdrift transport, our observed record is compared with output from a regional climate model. Here, we use the high-resolution RACMO2.3 climate model output. In this simulation, 36 modelled accumulation events attributed for a net snow accumulation of more than 1 kg m−2 at the pixel corresponding to the PE station location in the RACMO2.3 simulation (which equals to height changes between 0.26 and 0.33 cm assuming snow densities between 309–375 kg m−3), while 116 modelled ablation events resulted in net snowfall removal of more than 1 kg m−2. This is approximately 35 % of the observed quantities by the AWS. There are several potential underlying causes for this difference: either part of the snow erosion that is detected by the AWS occurs on the subgrid scale, or RACMO2.3 underestimates the blowing snow amount or its divergence. In fact, Lenaerts et al. (2014) showed that RACMO2.3 underestimates blowing snow amounts around the PE station. Generally, RCMs tend to underestimate blowing snow amounts over the whole of Antarctica (Amory et al., 2015).
Almost all areas north of the mountain ridge generate snow accumulation in the RACMO2.3 simulation when there is accumulation but no snowfall at the PE station (Fig. 9a). A fraction of 43 % of accumulation events occurs within 24 h after a snowfall event at the PE station (i.e. 31 out of 72 events). As shown by the RACMO2.3 simulation and ERA-Interim (compare Fig. 9c with Figs. 3 and 4b), most snowfall occurs upstream of the PE station, north of the Sør Rondane mountain ridge and ENE of the station during the 24 h preceding the accumulation event at the PE station. This freshly fallen snow has a low density and is easily redistributed, as the wind speed threshold for drifting snow erosion is low (Li and Pomeroy, 1997; Mahesh et al., 2003; Trouvilliez et al., 2014). As such, if the wind pattern stays stable from the ENE after the snowfall, it can be transported upstream resulting in accumulation at more westerly locations, such as the PE station.
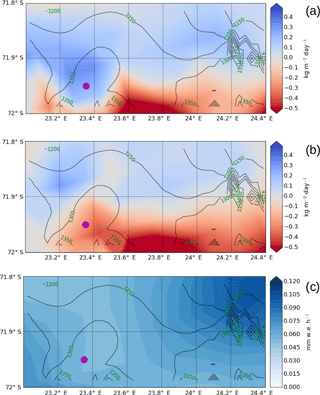
Figure 9Wind-driven snow accumulation and ablation during the two regimes at PE: (a) accumulation regime without snowfall (SMB +, S 0) and (b) ablation regime without snowfall (SMB −, S 0); (c) average amount of snowfall during the 24 h preceding both regimes. The fields are based on RACMO2.3 simulation. The purple dot indicates the location of the PE station, while the contours denote the topography in metres.
For ablation events (SMB −, S 0), the dominant wind direction originates from the south and is characterised by low wind speeds (Fig. 7b). The large-scale circulation analysis shows that there is a dominant occurrence of circulation pattern 5, as well as from circulation pattern 3 (Fig. 8b). Circulation pattern 5 only has a limited influence from low-pressure systems. In order to understand these ablation events, their timing is investigated. A fraction of 46 % of the ablation events take place within 24 h after a snowfall event. As stated in Sect. 3.3, snowfall events are mainly characterised by a ENE flow (Fig. 4a). After these snowfall episodes, the cyclone passes towards the east and winds tend to settle down (this evolution is depicted in Fig. 3). During calm conditions, a katabatic flow manifests, originating from the mountain ridge and the Antarctic plateau (Parish and Cassano, 2003). These areas, south of the PE station, generally received less snowfall during the preceding snowfall event compared to the areas north of the Sør Rondane Mountains (Fig. 9c and Palerme et al., 2014). They are therefore capable of picking up fresh snow at the PE station, resulting in ablation (Scarchilli et al., 2010). Similar conclusions can be drawn from the analysis of the RACMO2.3 climate model output, showing large areas of snow removal at the edge of the mountain ridge (Fig. 9b) and were also identified in RACMO2.3 by Lenaerts and van den Broeke (2012b).
An analysis of the wind roses indicates the occurrence of accumulation and ablation at very low wind speeds (Fig. 7). This points out that the time since the last snowfall event and the availability of low-density fresh snow are of much higher importance than the wind speed when generating blowing snow, confirming the results of Gallée et al. (2001), Mahesh et al. (2003), Scarchilli et al. (2010), Amory et al. (2017), and Gossart et al. (2017). The time since the last snowfall event might also be an important parameter that can explain the large variability in drifting snow wind speed thresholds and threshold friction velocities (Li and Pomeroy, 1997; Trouvilliez et al., 2014).
Based on the ceilometer, which is placed on a small ridge on the roof of the station, and its blowing snow detection algorithm, the concurrence of blowing snow and accumulation or ablation during non-snowfall conditions is analysed. For these accumulation and ablation events, blowing snow is detected by the ceilometer in only 27 and 20 % of the cases. All changes in snow height due to accumulation and ablation resulted in 1125 cm measured by the AWS. When the ceilometer detected blowing snow, only changes of 274 cm in height were detected by the AWS, indicating that the blowing snow layer only has a limited vertical extent and that the transport of snow is restricted to shallow layers close to the ground during these type of events. Furthermore, it is noted that ablation and accumulation events can significantly compensate each other (the total accumulation on a yearly basis due to snowfall and snowdrift is a lot smaller and has a large interannual variability ranging between 23 and 230 mm w.e. at the PE station).
Almost half of the accumulation and ablation events without snowfall occurred shortly after a snowfall event. Furthermore, wind speeds were found to be lower during these events compared to snowfall episodes. In both cases, fresh snow is easily transported towards or away from the station. During these types of events, the vertical extent of the blowing snow layer does not generally reach 30 m and most of the transport of this freshly fallen snow including accumulation and ablation occurs in shallow layers at moderate wind speeds. As such, in order to get a good idea of accumulation and ablation during non-precipitating time periods and their influence on the local SMB, near-ground observations of drifting and blowing snow are indispensable (Barral et al., 2014; Bellot et al., 2011; Leonard and Maksym, 2011; Leonard et al., 2012; Libois et al., 2014; Nishimura and Ishimaru, 2012; Nishimura and Nemoto, 2005; Takahashi, 1985; Trouvilliez et al., 2014).
In this study, snowfall and associated surface mass balance (SMB) changes (accumulation and ablation) are investigated with regard to large-scale atmospheric circulation patterns at the Princess Elisabeth (PE) station in East Antarctica. Using a unique set of remote sensing instruments, such as a Micro Rain Radar and an automatic weather station, which operated concurrently for a period of 37 months, statistical relationships between meteorology and snow erosion are obtained.
Snowfall is the most important source term of the local SMB and originates from oceanic air masses which are transported towards the AIS by cyclones in the Antarctic circumpolar trough. A back-trajectory analysis showed that air masses originating from more northern areas take up higher amounts of moisture, which mainly precipitate at the coastal areas of the AIS. Because of high wind speeds associated with these events, displacement of freshly fallen snow takes place in layers with a vertical extent of usually more than 30 m as detected by the ceilometer at the PE station. The distinction between accumulation and ablation events during snowfall was correlated to the duration of the event (i.e. the duration of snowfall and the temporal cloud extent). Longer and larger events result in bigger areas with fresh snow deposition, allowing snow from synoptically upstream areas to be transported towards the PE stations.
Wind-driven accumulation and ablation also occur without snowfall at the PE station. These accumulation events have a tendency to take place during similar types of circulation to snowfall events, however, they are characterised by lower wind speeds. During most of these accumulation events, snowfall took place upstream of the PE station, ENE of the station and to the north of the Sør Rondane mountain ridge, within the last 24 h. Winds easily pick up the freshly fallen snow from upstream areas and are capable of transporting it over tens of kilometres, leading to accumulation over large spatial areas potentially to higher elevations. Ablation events originate more often from southerly flows and also occur shortly after snowfall events at the station. Katabatic flow originating from the Antarctic plateau is dry, as it contains fewer snow particles and removes the freshly fallen snow at the local site, resulting in ablation. Both these accumulation and ablation events take place at low wind speeds in blowing and drifting snow layers of limited vertical extent as detected by the ceilometer at the PE station.
The results presented in this study may hint at the transport of snow towards more inland locations, instead of the traditional view of transport towards the coast by strong katabatic winds. This mechanism is also seen in RACMO2.3, although the absolute magnitude of accumulation of upstream snow in the absence of snowfall at the PE station only accounts for 35 % of the observed values. It is unclear whether this is due to an underestimation of the mass transport in RACMO2.3, uncertainty in the observations or due to the potential effect of small-scale processes (e.g. sastrugi) in the observational record. A network of blowing snow sensors would be needed to further address this issue.
Observations for this study were limited to one location over the AIS. As such, results might depend on the local topographical and meteorological conditions. However, as the main conclusions are based on both an analysis of synoptic and local meteorology, deductions of our work are also deemed to be valid at other coastal and escarpment areas of the AIS. Future work should expand the measurements of the individual components of the local SMB to other sites over the AIS in order to confirm the role of meteorological conditions at other areas, including their effect on the local SMB.
Data from the instrumentation at the Princess Elisabeth station can be obtained from the database at http://www.aerocloud.be/database/ (Souverijns et al., 2018).
The supplement related to this article is available online at: https://doi.org/10.5194/tc-12-1987-2018-supplement.
The authors declare that they have no conflict of interest.
Two anonymous reviewers are acknowledged for their comments that significantly
improved the manuscript. We thank the logistic teams for executing the
yearly maintenance of our instruments at the Princess Elisabeth station and
for their help by installing and setting up the new AWS. We thank Wim Boot,
Carleen Reijmer, and Michiel van den Broeke (Utrecht University, Institute
for Marine and Atmospheric Research Utrecht) for the development of the
automatic weather station, technical support and raw data processing. Jan
Lenaerts is acknowledged for sharing the data from the RACMO2.3
high-resolution simulation. This work was supported by the Belgian Science
Policy Office (BELSPO; grant number BR/143/A2/AEROCLOUD) and the Research
Foundation Flanders (FWO; grant number
G0C2215N).
Edited by: Robert Arthern
Reviewed by: two anonymous referees
Agosta, C., Favier, V., Genthon, C., Gallée, H., Krinner, G., Lenaerts, J. T. M., and van den Broeke, M. R.: A 40-year accumulation dataset for Adelie Land, Antarctica and its application for model validation, Clim. Dynam., 38, 75–86, https://doi.org/10.1007/s00382-011-1103-4, 2012. a
Agosta, C., Favier, V., Krinner, G., Gallée, H., Fettweis, X., and Genthon, C.: High-resolution modelling of the Antarctic surface mass balance, application for the twentieth, twenty first and twenty second centuries, Clim. Dynam., 41, 3247–3260, https://doi.org/10.1007/s00382-013-1903-9, 2013. a
Amory, C., Trouvilliez, A., Gallée, H., Favier, V., Naaim-Bouvet, F., Genthon, C., Agosta, C., Piard, L., and Bellot, H.: Comparison between observed and simulated aeolian snow mass fluxes in Adélie Land, East Antarctica, The Cryosphere, 9, 1373–1383, https://doi.org/10.5194/tc-9-1373-2015, 2015. a, b
Amory, C., Gallée, H., Naaim-Bouvet, F., Favier, V., Vignon, E., Picard, G., Trouvilliez, A., Piard, L., Genthon, C., and Bellot, H.: Seasonal Variations in Drag Coefficient over a Sastrugi-Covered Snowfield in Coastal East Antarctica, Bound.-Lay. Meteorol., 164, 107–133, https://doi.org/10.1007/s10546-017-0242-5, 2017. a, b, c, d
Anderson, P.: A method for rescaling humidity sensors at temperatures well below freezing, J. Atmos. Ocean. Tech., 11, 1388–1391, https://doi.org/10.1175/1520-0426(1994)011<1388:AMFRHS>2.0.CO;2, 1994. a
Barral, H., Genthon, C., Trouvilliez, A., Brun, C., and Amory, C.: Blowing snow in coastal Adélie Land, Antarctica: three atmospheric-moisture issues, The Cryosphere, 8, 1905–1919, https://doi.org/10.5194/tc-8-1905-2014, 2014. a, b
Battaglia, A. and Delanoë, J.: Synergies and complementarities of Cloudsat-CALIPSO snow observations, J. Geophys. Res.-Atmos., 118, 721–731, https://doi.org/10.1029/2012JD018092, 2013. a
Beck, C. and Philipp, A.: Evaluation and comparison of circulation type classifications for the European domain, Phys. Chem. Earth, 35, 374–387, https://doi.org/10.1016/j.pce.2010.01.001, 2010. a, b
Bellot, H., Trouvilliez, A., Naaim-Bouvet, F., Genthon, C., and Gallée, H.: Present weather-sensor tests for measuring drifting snow, Ann. Glaciol., 52, 176–184, https://doi.org/10.3189/172756411797252356, 2011. a
Bintanja, R. and Reijmer, C. H.: A simple parameterization for snowdrift sublimation, J. Geophys. Res., 106, 31739–31748, https://doi.org/10.1029/2000JD000107, 2001. a, b
Bliss, A. K., Cuffey, K. M., and Kavanaugh, J. L.: Sublimation and surface energy budget of Taylor Glacier, Antarctica, J. Glaciol., 57, 684–696, https://doi.org/10.3189/002214311797409767, 2011. a
Bracegirdle, T. J. and Marshall, G. J.: The Reliability of Antarctic Tropospheric Pressure and Temperature in the Latest Global Reanalyses, J. Climate, 25, 7138–7146, https://doi.org/10.1175/JCLI-D-11-00685.1, 2012. a
Bromwich, D. H.: Snowfall in High Southern Latitudes, Rev. Geophys., 26, 149–168, 1988. a
Bromwich, D. H., Guo, Z., Bai, L., and Chen, Q.-s.: Modeled Antarctic Precipitation. Part I: Spatial and Temporal Variability, J. Climate, 17, 427–447, https://doi.org/10.1175/1520-0442(2004)017<0427:MAPPIS>2.0.CO;2, 2004. a
Bromwich, D. H., Nicolas, J. P., and Monaghan, A. J.: An Assessment of Precipitation Changes over Antarctica and the Southern Ocean since 1989 in Contemporary Global Reanalyses, J. Climate, 24, 4189–4209, https://doi.org/10.1175/2011JCLI4074.1, 2011. a
Casado, M., Pastor, M., and Doblas-Reyes, F.: Links between circulation types and precipitation over Spain, Phys. Chemi. Earth, 35, 437–447, https://doi.org/10.1016/j.pce.2009.12.007, 2010. a, b
Casella, D., Panegrossi, G., Sano, P., Marra, A. C., Dietrich, S., Johnson, B. T., and Kulie, M. S.: Evaluation of the GPM-DPR snowfall detection capability: Comparison with CloudSat-CPR, Atmos. Res., 197, 64–75, https://doi.org/10.1016/j.atmosres.2017.06.018, 2017. a
Das, I., Bell, R. E., Scambos, T. A., Wolovick, M., Creyts, T. T., Studinger, M., Frearson, N., Nicolas, J. P., Lenaerts, J. T. M., and van den Broeke, M. R.: Influence of persistent wind scour on the surface mass balance of Antarctica, Nat. Geosci., 6, 367–371, https://doi.org/10.1038/ngeo1766, 2013. a, b
Davis, C. H., Li, Y., McConnel, J. R., Frey, M. M., and Hanna, E.: Snowfall-Driven Growth in East Antarctic Ice Sheet Mitigates Recent Sea-Level Rise, Science, 308, 1898–1901, https://doi.org/10.1126/science.1110662, 2005. a
DeConto, R. M. and Pollard, D.: Contribution of Antarctica to past and future sea-level rise, Nature, 531, 591–597, https://doi.org/10.1038/nature17145, 2016. a
Dee, D. P., Uppala, S. M., Simmons, A. J., Berrisford, P., Poli, P., and Kobayashi, S.: The ERA-Interim reanalysis: configuration and performance of the data assimilation system, Q. J. Roy. Meteor. Soc., 137, 553–597, https://doi.org/10.1002/qj.828, 2011. a
Déry, S. J. and Yau, M. K.: Simulation of blowing snow in the Canadian Arctic using a double-moment model, Bound.-Lay. Meteorol., 99, 297–316, https://doi.org/10.1023/A:1018965008049, 2001. a
Dürr, B. and Philipona, R.: Automatic cloud amount detection by surface longwave downward radiation measurements, J. Geophys. Res., 109, D05201, https://doi.org/10.1029/2003JD004182, 2004. a
Eisen, O., Frezzotti, M., Genthon, C., Isaksson, E., Magand, O., van den Broeke, M. R., Dixon, D. A., Ekaykin, A., Holmlund, P., Kameda, T., Karlöf, L., Kaspari, S., Lipenkov, V. Y., Oerter, H., Takahashi, S., and Vaughan, D. G.: Ground-based measurements of spatial and temporal variability of snow accumulation in East Antarctica, Rev. Geophys., 46, RG2001, https://doi.org/10.1029/2006RG000218, 2008. a, b, c
Favier, V., Agosta, C., Parouty, S., Durand, G., Delaygue, G., Gallée, H., Drouet, A.-S., Trouvilliez, A., and Krinner, G.: An updated and quality controlled surface mass balance dataset for Antarctica, The Cryosphere, 7, 583–597, https://doi.org/10.5194/tc-7-583-2013, 2013. a, b
Favier, V., Krinner, G., Amory, C., Gallée, H., Beaumet, J., and Agosta, C.: Antarctica-Regional Climate and Surface Mass Budget, Current Climate Change Reports, 3, 303–315, https://doi.org/10.1007/s40641-017-0072-z, 2017. a
Frezzotti, M., Pourchet, M., Flora, O., Gandolfi, S., Gay, M., Urbini, S., Vincent, C., Becagli, S., Gragnani, R., Proposito, M., Severi, M., Traversi, R., Udisti, R., and Fily, M.: Spatial and temporal variability of snow accumulation in East Antarctica from traverse data, J. Glaciol., 51, 113–124, https://doi.org/10.3189/172756505781829502, 2005. a
Gallée, H., Guyomarc'h, G., and Brun, E.: Impact of snow drift on the Antarctic Ice Sheet surface mass balance: Possible sensitivity to snow-surface properties, Bound.-Lay. Meteorol., 99, 1–19, https://doi.org/10.1023/A:1018776422809, 2001. a
Gallée, H., Trouvilliez, A., Agosta, C., Genthon, C., Favier, V., and Naaim-Bouvet, F.: Transport of Snow by the Wind: A Comparison Between Observations in Adélie Land, Antarctica, and Simulations Made with the Regional Climate Model MAR, Bound.-Lay. Meteorol., 146, 133–147, https://doi.org/10.1007/s10546-012-9764-z, 2013. a
Genthon, C. and Krinner, G.: Antarctic surface mass balance and systematic biases in general circulation models, J. Geophys. Res., 106, 20653–20664, https://doi.org/10.1029/2001JD900136, 2001. a
Genthon, C., Kaspari, S., and Mayewski, P. A.: Interannual variability of the surface mass balance of West Antarctica from ITASE cores and ERA40 reanalyses, 1958-2000, Clim. Dynam., 24, 759–770, https://doi.org/10.1007/s00382-005-0019-2, 2005. a
Gorodetskaya, I. V., van Lipzig, N. P. M., van den Broeke, M. R., Mangold, A., Boot, W., and Reijmer, C. H.: Meteorological regimes and accumulation patterns at Utsteinen, Dronning Maud Land, East Antarctica: Analysis of two contrasting years, J. Geophys. Res.-Atmos., 118, 1700–1715, https://doi.org/10.1002/jgrd.50177, 2013. a, b, c, d, e, f, g, h
Gorodetskaya, I. V., Tsukernik, M., Claes, K., Ralph, M., Neff, W., and van Lipzig, N. P. M.: The role of atmospheric rivers in anomalous snow accumulation in East Antarctica, Geophys. Res. Lett., 16, 6199–6206, https://doi.org/10.1002/2014GL060881, 2014. a, b
Gorodetskaya, I. V., Kneifel, S., Maahn, M., Van Tricht, K., Thiery, W., Schween, J. H., Mangold, A., Crewell, S., and Van Lipzig, N. P. M.: Cloud and precipitation properties from ground-based remote-sensing instruments in East Antarctica, The Cryosphere, 9, 285–304, https://doi.org/10.5194/tc-9-285-2015, 2015. a, b, c, d, e, f, g, h, i, j, k, l, m, n, o, p, q, r
Gossart, A., Souverijns, N., Gorodetskaya, I. V., Lhermitte, S., Lenaerts, J. T. M., Schween, J. H., Mangold, A., Laffineur, Q., and van Lipzig, N. P. M.: Blowing snow detection from ground-based ceilometers: application to East Antarctica, The Cryosphere, 11, 2755–2772, https://doi.org/10.5194/tc-11-2755-2017, 2017. a, b, c, d, e, f
Grazioli, J., Genthon, C., Boudevillain, B., Duran-Alarcon, C., Del Guasta, M., Madeleine, J.-B., and Berne, A.: Measurements of precipitation in Dumont d'Urville, Adélie Land, East Antarctica, The Cryosphere, 11, 1797–1811, https://doi.org/10.5194/tc-11-1797-2017, 2017a. a, b, c
Grazioli, J., Madeleine, J.-B., Gallée, H., Forbes, R. M., Genthon, C., Krinner, G., and Berne, A.: Katabatic winds diminish precipitation contribution to the Antarctic ice mass balance, P. Natl. Acad. Sci. USA, 114, 10858–10863, https://doi.org/10.1073/pnas.1707633114, 2017b. a, b, c
Gregory, J. M. and Huybrechts, P.: Ice-sheet contributions to future sea-level change, Philos. T. Roy. Soc. A, 364, 1709–1731, https://doi.org/10.1098/rsta.2006.1796, 2006. a
Groot Zwaaftink, C. D., Cagnati, A., Crepaz, A., Fierz, C., Macelloni, G., Valt, M., and Lehning, M.: Event-driven deposition of snow on the Antarctic Plateau: analyzing field measurements with SNOWPACK, The Cryosphere, 7, 333–347, https://doi.org/10.5194/tc-7-333-2013, 2013. a, b
Hirasawa, N., Nakamura, H., Motoyama, H., Hayashi, M., and Yamanouchi, T.: The role of synoptic-scale features and advection in prolonged warming and generation of different forms of precipitation at Dome Fuji station, Antarctica, following a prominent blocking event, J. Geophys. Res.-Atmos., 118, 6916–6928, https://doi.org/10.1002/jgrd.50532, 2013. a, b
Huth, R., Beck, C., Philipp, A., Demuzere, M., Ustrnul, Z., Cahynová, M., Kyselý, J., and Tveito, O. E.: Classifications of atmospheric circulation patterns: recent advances and applications, Ann. NY Acad. Sci., 1146, 105–152, https://doi.org/10.1196/annals.1446.019, 2008. a, b
King, J. C. and Turner, J.: Antarctic meteorology and climatology, Cambridge University Press, 1997. a, b, c
King, J. C., Anderson, P. S., and Mann, G.: The seasonal cycle of sublimation at Halley, Antarctica, J. Glaciol., 47, 1–8, https://doi.org/10.3189/172756501781832548, 2001. a
Klugmann, D., Heinsohn, K., and Kirtzel, H.-J.: A low cost 24 GHz FM-CW Doppler radar rain profiler, Contributions to Atmospheric Physics, 69, 247–253, 1996. a
Kneifel, S., Maahn, M., Peters, G., and Simmer, C.: Observation of snowfall with a low-power FM-CW K-band radar (Micro Rain Radar), Meteorol. Atmos. Phys., 113, 75–87, https://doi.org/10.1007/s00703-011-0142-z, 2011. a
König-Langlo, G. and Loose, B.: The Meteorological Observatory at Neumayer Stations (GvN and NM-II) Antarctica, Polarforschung, 76, 25–38, 2006. a
Lenaerts, J. T. M. and van den Broeke, M. R.: Modeling drifting snow in Antarctica with a regional climate model: 1. Methods and model evaluation, J. Geophys. Res., 117, D05108, https://doi.org/10.1029/2011JD016145, 2012a. a, b
Lenaerts, J. T. M. and van den Broeke, M. R.: Modeling drifting snow in Antarctica with a regional climate model: 2. Results, J. Geophys. Res.h, 117, D05109, https://doi.org/10.1029/2010JD015419, 2012b. a
Lenaerts, J. T. M., Brown, J., van den Broeke, M. R., Matsuoka, K., Drews, R., Callens, D., Philippe, M., Gorodetskaya, I. V., van Meijgaard, E., Reijmer, C. H., Pattyn, F., and van Lipzig, N. P. M.: High variability of climate and surface mass balance induced by Antarctic ice rises, J. Glaciol., 60, 1126–1134, https://doi.org/10.3189/2014JoG14J040, 2014. a, b, c
Lenaerts, J. T. M., Vizcaino, M., Fyke, J., van Kampenhout, L., and van den Broeke, M. R.: Present-day and future Antarctic ice sheet climate and surface mass balance in the Community Earth System Model, Clim. Dynam., 47, 1367–1381, https://doi.org/10.1007/s00382-015-2907-4, 2016. a
Lenaerts, J. T. M., Lhermitte, S., Drews, R., Ligtenberg, S. R. M., Berger, S., Helm, V., Smeets, C. J. P. P., van den Broeke, M. R., van de Berg, W. J., van Meijgaard, E., Eijkelboom, M., Eisen, O., and Pattyn, F.: Meltwater produced by wind-albedo interaction stored in an East Antarctic ice shelf, Nat. Clim. Change, 7, 58–62, https://doi.org/10.1038/nclimate3180, 2017. a, b
Leonard, K. C. and Maksym, T.: The importance of wind-blown snow redistribution to snow accumulation on Bellingshausen Sea ice, Ann. Glaciol., 52, 271–278, https://doi.org/10.3189/172756411795931651, 2011. a
Leonard, K. C., Tremblay, L. B., Thom, J. E., and MacAyeal, D. R.: Drifting snow threshold measurements near McMurdo station, Antarctica: A sensor comparison study, Cold Reg. Sci. Technol., 70, 71–80, https://doi.org/10.1016/j.coldregions.2011.08.001, 2012. a, b
Li, L. and Pomeroy, J. W.: Estimates of Threshold Wind Speeds for Snow Transport Using Meteorological Data, J. Appl. Meteorol., 36, 205–213, https://doi.org/10.1175/1520-0450(1997)036<0205:EOTWSF>2.0.CO;2, 1997. a, b, c
Libois, Q., Picard, G., Arnaud, L., Morin, S., and Brun, E.: Modeling the impact of snow drift on the decameter-scale variability of snow properties on the Antarctic Plateau, J. Geophys. Res., 119, 11662–11681, https://doi.org/10.1002/2014JD022361, 2014. a, b, c, d
Ligtenberg, S. R. M., van de Berg, W. J., van den Broeke, M. R., Rae, J. G. L., and van Meijgaard, E.: Future surface mass balance of the Antarctic ice sheet and its influence on sea level change, simulated by a regional atmospheric climate model, Clim. Dynam., 41, 867–884, https://doi.org/10.1007/s00382-013-1749-1, 2013. a
Maahn, M. and Kollias, P.: Improved Micro Rain Radar snow measurements using Doppler spectra post-processing, Atmos. Meas. Tech., 5, 2661–2673, https://doi.org/10.5194/amt-5-2661-2012, 2012. a
Maahn, M., Burgard, C., Crewell, S., Gorodetskaya, I. V., Kneifel, S., Lhermitte, S., Van Tricht, K., and van Lipzig, N. P. M.: How does the spaceborne radar blind zone affect derived surface snowfall statistics in polar regions?, J. Geophys. Res.-Atmos., 119, 13604–13620, https://doi.org/10.1002/2014JD022079, 2014. a
Magand, O., Genthon, C., Fily, M., Krinner, G., Picard, G., Frezzotti, M., and Ekaykin, A. A.: An up-to-date quality-controlled surface mass balance data set for the 90∘–180∘ E Antarctica sector and 1950–2005 period, J. Geophys. Res., 112, D12106, https://doi.org/10.1029/2006JD007691, 2007. a
Mahesh, A., Eager, R., Campbell, J. R., and Spinhirne, J. D.: Observations of blowing snow at the South Pole, J. Geophys. Res., 108, 4707, https://doi.org/10.1029/2002JD003327, 2003. a, b
Marty, C. and Philipona, R.: The Clear-Sky Index to separate Clear-Sky from Cloudy-Sky Situations in Climate Research, Geophys. Res. Lett., 27, 2649–2652, 2000. a, b
Matrosov, S. Y., Shupe, M. D., and Djalalova, I. V.: Snowfall retrievals using millimeter-wavelength cloud radars, J. Appl. Meteorol. Clim., 47, 769–777, https://doi.org/10.1175/2007JAMC1768.1, 2008. a
Newman, A. J., Kucera, P. A., and Bliven, L. F.: Presenting the Snowflake Video Imager (SVI), J. Atmos. Ocean. Tech., 26, 167–179, https://doi.org/10.1175/2008JTECHA1148.1, 2009. a
Nishimura, K. and Ishimaru, T.: Development of an automatic blowing-snow station, Cold Reg. Sci. Technol., 82, 30–35, https://doi.org/10.1016/j.coldregions.2012.05.005, 2012. a, b
Nishimura, K. and Nemoto, M.: Blowing snow at Mizuho station, Antarctica, Philos. T. Roy. Soc. A, 363, 1647–1662, https://doi.org/10.1098/rsta.2005.1599, 2005. a, b, c
Palerme, C., Kay, J. E., Genthon, C., L'Ecuyer, T., Wood, N. B., and Claud, C.: How much snow falls on the Antarctic ice sheet?, The Cryosphere, 8, 1577–1587, https://doi.org/10.5194/tc-8-1577-2014, 2014. a, b
Palm, S. P., Yang, Y., Spinhirne, J. D., and Marshak, A.: Satellite remote sensing of blowing snow properties over Antarctica, J. Geophys. Res.-Atmos., 116, D16123, https://doi.org/10.1029/2011JD015828, 2011. a, b
Palm, S. P., Kayetha, V., Yang, Y., and Pauly, R.: Blowing snow sublimation and transport over Antarctica from 11 years of CALIPSO observations, The Cryosphere, 11, 2555–2569, https://doi.org/10.5194/tc-11-2555-2017, 2017. a
Parish, T. R. and Cassano, J. J.: The Role of Katabatic Winds on the Antarctic Surface Wind Regime, Mon. Weather Rev., 131, 317–333, https://doi.org/10.1175/1520-0493(2003)131<0317:TROKWO>2.0.CO;2, 2003. a
Pattyn, F., Matsuoka, K., and Berte, J.: Glacio-meteorological conditions in the vicinity of the Belgian Princess Elisabeth Station, Antarctica, Antarct. Sci., 22, 79–85, https://doi.org/10.1017/S0954102009990344, 2010. a, b
Peters, G., Fischer, B., and Andersson, T.: Rain observations with a vertically looking Micro Rain Radar (MRR), Boreal Environ. Res., 7, 353–362, 2002. a
Philipp, A., Della-Marta, P. M., Jacobeit, J., Fereday, D. R., Jones, P. D., Moberg, A., and Wanner, H.: Long-Term Variability of Daily North Atlantic–European Pressure Patterns since 1850 Classified by Simulated Annealing Clustering, J. Climate, 20, 4065–4095, https://doi.org/10.1175/JCLI4175.1, 2007. a
Philipp, A., Bartholy, J., Beck, C., Erpicum, M., Esteban, P., Fettweis, X., Huth, R., James, P., Jourdain, S., Kreienkamp, F., Krennert, T., Lykoudis, S., Michalides, S. C., Pianko-Kluczynska, K., Post, P., Rasilla Álvarez, D., Schiemann, R., Spekat, A., and Tymvios, F. S.: Cost733cat – A database of weather and circulation type classifications, Phys. Chem. Earth, 35, 360–373, https://doi.org/10.1016/j.pce.2009.12.010, 2010. a
Previdi, M. and Polvani, L. M.: Anthropogenic impact on Antarctic surface mass balance, currently masked by natural variability, to emerge by mid-century, Environ. Res. Lett., 11, 094001, https://doi.org/10.1088/1748-9326/11/9/094001, 2016. a
Rignot, E., Velicogna, I., van den Broeke, M. R., Monaghan, A., and Lenaerts, J. T. M.: Acceleration of the contribution of the Greenland and Antarctic ice sheets to sea level rise, Geophys. Res. Lett., 38, L05503, https://doi.org/10.1029/2011GL046583, 2011. a, b
Rotschky, G., Holmlund, P., Isaksson, E., Mulvaney, R., Oerter, H., van den Broeke, M. R., and Winther, J. G.: A new surface accumulation map for western Dronning Maud Land, Antarctica, from interpolation of point measurements, J. Glaciol., 53, 385–398, https://doi.org/10.3189/002214307783258459, 2007. a
Rousseeuw, P. J.: Silhouettes: A graphical aid to the interpretation and validation of cluster analysis, J. Comput. Appl. Math., 20, 53–65, https://doi.org/10.1016/0377-0427(87)90125-7, 1987. a
Scarchilli, C., Frezzotti, M., Grigioni, P., De Silvestri, L., Agnoletto, L., and Dolci, S.: Extraordinary blowing snow transport events in East Antarctica, Clim. Dynam., 34, 1195–1206, https://doi.org/10.1007/s00382-009-0601-0, 2010. a, b, c
Schlosser, E., Manning, K. W., Powers, J. G., Duda, M. G., Birnbaum, G., and Fujita, K.: Characteristics of high-precipitation events in Dronning Maud Land, Antarctica, J. Geophys. Res., 115, D14107, https://doi.org/10.1029/2009JD013410, 2010. a
Shepherd, A., Ivins, E. R., Geruo, A., Barletta, V. R., Bentley, M. J., Bettadpur, S., Briggs, K. H., Bromwich, D. H., Forsberg, R., Galin, N., Horwath, M., Jacobs, S., Joughin, I., King, M. A., Lenaerts, J. T. M., Li, J., Ligtenberg, S. R. M., Luckman, A., Luthcke, S. B., McMillan, M., Meister, R., Milne, G., Mouginot, J., Muir, A., Nicolas, J. P., Paden, J., Payne, A. J., Pritchard, H., Rignot, E., Rott, H., Sorensen, L. S., Scambos, T. A., Scheuchl, B., Schrama, E. J. O., Smith, B., Sundal, A. V., van Angelen, J. H., van de Berg, W. J., van den Broeke, M. R., Vaughan, D. G., Velicogna, I., Wahr, J., Whitehouse, P. L., Wingham, D. J., Yi, D., Young, D., and Zwally, H. J.: A Reconciled Estimate of Ice-Sheet Mass Balance, Science, 338, 1183–1189, 2012. a
Simmonds, I., Keay, K., and Lim, E.-P.: Synoptic Activity in the Seas around Antarctica, Mon. Weather Rev., 131, 272–288, https://doi.org/10.1175/1520-0493(2003)131<0272:SAITSA>2.0.CO;2, 2003. a, b
Souverijns, N., Thiery, W., Demuzere, M., and Van Lipzig, N. P. M.: Drivers of future changes in East African precipitation, Environ. Res. Lett., 11, 114011, https://doi.org/10.1088/1748-9326/11/11/114011, 2016. a, b
Souverijns, N., Gossart, A., Lhermitte, S., Gorodetskaya, I. V., Kneifel, S., Maahn, M., Bliven, F. L., and van Lipzig, N. P. M.: Estimating radar reflectivity – Snowfall rate relationships and their uncertainties over Antarctica by combining disdrometer and radar observations, Atmos. Res., 196, 211–223, https://doi.org/10.1016/j.atmosres.2017.06.001, 2017. a, b, c, d
Souverijns, N., Gossart, A., Gorodetskaya, I. V., and van Lipzig, N. P. M.: Aerosol, Cloud and Precipitation database at the Princess Elisabeth station, Dronning Maud Land, Antarctica, available at: http://www.aerocloud.be/database/, last access: 8 June 2018. a
Stohl, A. and Seibert, P.: Accuracy of trajectories as determined from the conservation of meteorological tracers, Q. J. Roy. Meteor. Soc., 124, 1465–1484, https://doi.org/10.1002/qj.49712454907, 1998. a
Stohl, A., Wotawa, G., Seibert, P., and Kromp-Kolb, H.: Interpolation Errors in Wind Fields as a Function of Spatial and Temporal Resolution and Their Impact on Different Types of Kinematic Trajectories, J. Appl. Meteorol., 34, 2149–2165, https://doi.org/10.1175/1520-0450(1995)034<2149:IEIWFA>2.0.CO;2, 1995. a
Takahashi, S.: Characteristics of drifting snow at Mizuho Station, Antarctica, Ann. Glaciol., 6, 71–75, https://doi.org/10.1017/S0260305500010028, 1985. a
Thiery, W., Gorodetskaya, I. V., Bintanja, R., Van Lipzig, N. P. M., Van den Broeke, M. R., Reijmer, C. H., and Kuipers Munneke, P.: Surface and snowdrift sublimation at Princess Elisabeth station, East Antarctica, The Cryosphere, 6, 841–857, https://doi.org/10.5194/tc-6-841-2012, 2012. a, b, c
Thomas, E. R., van Wessem, J. M., Roberts, J., Isaksson, E., Schlosser, E., Fudge, T. J., Vallelonga, P., Medley, B., Lenaerts, J., Bertler, N., van den Broeke, M. R., Dixon, D. A., Frezzotti, M., Stenni, B., Curran, M., and Ekaykin, A. A.: Regional Antarctic snow accumulation over the past 1000 years, Clim. Past, 13, 1491–1513, https://doi.org/10.5194/cp-13-1491-2017, 2017. a
Trouvilliez, A., Naaim-Bouvet, F., Genthon, C., Piard, L., Favier, V., Bellot, H., Agosta, C., Palerme, C., Amory, C., and Gallée, H.: A novel experimental study of aeolian snow transport in Adelie Land (Antarctica), Cold Reg. Sci. Technol., 108, 125–138, https://doi.org/10.1016/j.coldregions.2014.09.005, 2014. a, b, c, d
van den Broeke, M. R. and van Lipzig, N. P. M.: Factors Controlling the Near-Surface Wind Field in Antarctica, Mon. Weather Rev., 131, 733–743, https://doi.org/10.1175/1520-0493(2003)131<0733:FCTNSW>2.0.CO;2, 2003. a
van den Broeke, M. R., Reijmer, C. H., and Van de Wal, R. S. W.: A study of the surface mass balance in Dronning Maud Land, Antarctica, using automatic weather stations, J. Glaciol., 50, 565–582, https://doi.org/10.3189/172756504781829756, 2004. a, b, c, d, e
van Lipzig, N. P. M., van Meijgaard, E., and Oerlemans, J.: The spatial and temporal variability of the surface mass balance in Antarctica: results from a regional atmospheric climate model, Int. J. Climatol., 22, 1197–1217, https://doi.org/10.1002/joc.798, 2002. a, b
van Lipzig, N. P. M., King, J. C., Lachlan-Cope, T. A., and van den Broeke, M. R.: Precipitation, sublimation, and snow drift in the Antarctic Peninsula region from a regional atmospheric model, J. Geophys. Res., 109, D24106, https://doi.org/10.1029/2004JD004701, 2004. a
Van Tricht, K., Gorodetskaya, I. V., Lhermitte, S., Turner, D. D., Schween, J. H., and Van Lipzig, N. P. M.: An improved algorithm for polar cloud-base detection by ceilometer over the ice sheets, Atmos. Meas. Tech., 7, 1153–1167, https://doi.org/10.5194/amt-7-1153-2014, 2014. a
van Wessem, J. M., Reijmer, C. H., Lenaerts, J. T. M., van de Berg, W. J., van den Broeke, M. R., and van Meijgaard, E.: Updated cloud physics in a regional atmospheric climate model improves the modelled surface energy balance of Antarctica, The Cryosphere, 8, 125–135, https://doi.org/10.5194/tc-8-125-2014, 2014. a
Vaughan, D., Comiso, J., Allison, I., Carrasco, J., Kaser, G., Kwok, R., Mote, P., Murray, T., Paul, F., Ren, J., Rignot, E., Solomina, O., Steffen, K., and Zhang, T.: Observations: Cryosphere, in: Climate Change 2013: The Physical Science Basis, Contribution of Working Group I to the Fifth Assessment Report of the Intergovernmental Panel on Climate Change, edited by: Stocker, T., Qin, D., Plattner, G.-K., Tignor, M., Allen, S., Boschung, J., Nauels, A., Xia, Y., Bex, V., and Midgley, P., Cambridge University Press, Cambridge, United Kingdom and New York, NY, USA, https://doi.org/10.1017/CBO9781107415324.012, 317–382, 2013. a
Vaughan, D. G., Bamber, J. L., Giovinetto, M., Russell, J., and Cooper, A. P. R.: Reassessment of Net Surface Mass Balance in Antarctica, J. Climate, 12, 933–946, https://doi.org/10.1175/1520-0442(1999)012<0933:RONSMB>2.0.CO;2, 1999. a, b, c
Wang, Y., Ding, M., van Wessem, J. M., Schlosser, E., Altnau, S., van den Broeke, M. R., Lenaerts, J. T., Thomas, E. R., Isaksson, E., Wang, J., and Sun, W.: A comparison of Antarctic ice sheet surface mass balance from atmospheric climate models and in situ observations, J. Climate, 29, 5317–5337, https://doi.org/10.1175/JCLI-D-15-0642.1, 2016. a, b
Wiegner, M., Madonna, F., Binietoglou, I., Forkel, R., Gasteiger, J., Geiß, A., Pappalardo, G., Schäfer, K., and Thomas, W.: What is the benefit of ceilometers for aerosol remote sensing? An answer from EARLINET, Atmos. Meas. Tech., 7, 1979–1997, https://doi.org/10.5194/amt-7-1979-2014, 2014. a
Wood, N. B.: Estimation of snow microphysical properties with application to millimeter-wavelength radar retrievals for snowfall rate, PhD thesis, Colorado State University, Colorado, available at: http://hdl.handle.net/10217/48170 (last access: 8 June 2018), 2011. a